Diffusion tensor image analysis along the perivascular space and quantitative susceptibility mapping in the diagnosis and severity assessment of Parkinson’s disease
Introduction
The incidence of Parkinson’s disease (PD), a prevalent neurodegenerative disorder, notably increases between the ages of 55 and 79 years. Its pathological hallmarks lie in the loss of dopaminergic neurons in the substantia nigra (SN), coupled with intracellular inclusions containing aggregates of α-synuclein (1). Accurate diagnosis and severity assessment of PD remain crucial to the disease’s efficient treatment and management. Conventional diagnostic methodologies largely depend on clinical scores, primarily the Movement Disorder Society-Unified Parkinson’s Disease Rating Scale (MDS-UPDRS), with increasing emphasis on motor symptoms. Nonetheless, these approaches exhibit limitations in capably capturing the complex pathophysiology of PD.
Recently, there has been a surge of interest in studies focusing on glymphatic dysfunction in PD. Emerging evidence underscores the vital part that the glymphatic system plays in removing neurotoxic protein aggregates often found in neurodegenerative diseases, such as α-synuclein in PD (2). Dysfunction in glymphatic clearance can potentially lead to secondary damage to dopaminergic neurons due to increased α-synuclein accumulation. Extant research has demonstrated that obstructing the glymphatic pathway, consequently causing drainage dysfunction, could enhance α-synuclein pathology and intensify motor symptoms (3). Hence, glymphatic dysfunction is a significant factor in the pathology and physiology of PD, making it crucial to develop a non-invasive and reliable method to assess glymphatic functionality in vivo. The diffusion tensor image analysis along the perivascular space (DTI-ALPS) index, a non-invasive measure, demonstrates substantial representation and reproducibility of glymphatic clearance function (4,5). This DTI-ALPS index has been correlated with aging, PD, and multiple sclerosis (4,6,7). In PD patients, the DTI-ALPS index has been reported as being notably lower than that seen in healthy individuals, indicating the presence of glymphatic dysfunction in PD. Additionally, some studies in PD patients have found that a lower DTI-ALPS index showed a significant association with the higher MDS-UPDRS III score (8) and its subscore for rigidity (9), and longitudinal cognitive decline and worse longitudinal disease severity (10).
Meanwhile, a growing body of evidence suggests a significant role of abnormal iron homeostasis in PD pathology (11). Excessive iron can generate reactive oxygen species (ROS) that subsequently lead to neuronal death through iron-mediated mechanisms (12,13). Moreover, surplus iron in the brain is also implicated in various critical pathophysiological pathways specific to PD (12,14). In PD patients, post-mortem examinations most frequently reveal heightened iron levels in the SN (12,15). As a result, iron deposition is considered a contributor to brain damage mechanisms in PD, leading to the evaluation of in vivo brain iron as a prominent research focus. High magnetic susceptibility of iron allows for its detection through magnetic resonance imaging (MRI), especially via an advanced imaging technique known as quantitative susceptibility mapping (QSM). QSM provides quantitative estimates of voxel-level iron distribution in the brain (16). Across various brain regions, QSM has reliably quantified changes in iron content (17-19). Post-mortem studies further validate the accuracy of QSM in identifying iron deposition in brain regions, showing strong correlations between QSM contrast and histochemical measurements of iron (17,20,21). Previous research showed that susceptibility measures in PD patients were significantly higher than in healthy individuals across various brain regions, especially in SN, pointing to increased iron accumulation in PD (22). Another study found that the susceptibility measure was significantly associated with the MDS-UPDRS III score (23).
DTI-ALPS and QSM are fundamental quantitative imaging techniques in PD research. DTI-ALPS captures dysfunction in the glymphatic system, whereas QSM quantifies iron accumulation across various brain regions. However, research that compares or integrates these techniques in diagnosing and determining the severity of PD is scant. Consequently, we postulate that the diagnostic accuracies between these two methods may vary. Moreover, their strategic combination may enhance PD diagnosis. Therefore, this study was designed to assess the diagnostic utility of these two techniques in PD. Additionally, we also evaluated the correlation between DTI-ALPS and QSM with MDS-UPDRS III. We present this article in accordance with the STROBE reporting checklist (available at https://qims.amegroups.com/article/view/10.21037/qims-24-1605/rc).
Methods
Participants
Patients with idiopathic PD were identified and evaluated retrospectively from the Department of Neurology in Fujian Medical University Union Hospital between March 2019 and April 2023. The diagnosis of PD was determined based on the diagnostic criteria from the International Parkinson & Movement Disorder Society (24) and confirmed by two senior physicians, each with more than 10 years of experience. For this study, age- and gender-matched healthy control (HC) participants were also recruited. HC participants had no familial history of PD or any associated neurodegenerative disorders. Participants were randomly enrolled. Data such as sex and age of all participants, and disease duration, Hoehn and Yahr scale, and MDS-UPDRS III (Med-OFF state) of PD patients were compiled for further analysis. We established the following exclusion criteria: (I) a history of significant medical conditions, such as pronounced cardiovascular disorders that could potentially affect cardiovascular brain impulses influencing glymphatic flux (25); (II) other prevalent systemic, psychiatric, or neurological diseases; (III) contraindications to MRI and poor quality of MRI. The study was conducted in accordance with the Declaration of Helsinki (as revised in 2013) and approved by the Ethics Committee of the Fujian Medical University Union Hospital (No. 2023KJCX058). Informed consent was provided by all individual participants. Figure 1 provides a visual depiction of the screening process implemented for the inclusion of PD patients and HC participants.
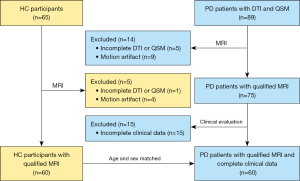
MRI acquisition
MRI was conducted on a 3.0-Tesla MR scanner (MAGNETOM Prisma; Siemens Healthineers, Erlangen, Germany) utilizing a 64-channel receive-only head coil. The utilized structural MRI protocols comprised a sagittal T1-weighted magnetization prepared rapid gradient echo sequence (T1-MPRAGE) with a voxel size of 0.9375×0.9375×0.9 mm3, axial T2-weighted fast spin-echo images, and fluid attenuated inversion recovery (FLAIR). The scan parameters of sagittal T1-MPRAGE were as follows: repetition time (TR) =2,300 ms; echo time (TE) =2.32 ms; inversion time, 900 ms; acquisition matrix, 256×256; field of view (FOV) =240 mm × 240 mm; number of averages =1; voxel size =0.9375×0.9375×0.9 mm3. DTI was performed with a spinning-echo echo-planar imaging (SE-EPI) sequence, which consisted of 9 b-values (250, 350, 400, 550, 750, 950, 1,100, 1,150, and 1,500 s/mm2), corresponding with 3, 2, 4, 4, 3, 12, 8, 4, and 6 directions, respectively. The sequence parameters were as follows: TR =3,900 ms; TE =88 ms; FOV =230 mm × 230 mm; generalized autocalibrating partial parallel acquisition (GRAPPA) =2; slice acceleration factor =2; number of averages =1; voxel size =2.5×2.5×2.5 mm3, with no gap; and a bipolar pulse. QSM imaging was performed based on three-dimensional flow-compensated multi-echo gradient-echo (GRE) images in the axial plane (TR =35 ms; first TE =6.67 ms; uniform echo spacing =6.24 ms; last TE =25.39 ms; number of echoes =4; flip angle =15◦; FOV =280 mm × 320 mm; voxel size =0.72×0.72×2 mm3).
Quantification of QSM
The software STIsuite (https://chunleiliulab.github.io/software.html) was employed for the computation of the QSM maps from the phase images. Specifically, the raw phase was unwrapped using a Laplacian-based phase unwrapping (26) and the normalized phase was calculated. The normalized background phase was removed using the spherical-mean-value filtering (V_SHARP) (27). QSM images were calculated using STreaking Artifact Reduction for QSM (STAR-QSM) method (28).
Images derived from the T1-MPRAGE images were co-registered with QSM images by using rigid-body registering to the initial echo magnitude image drawn from the GRE pulse sequence. This procedure utilized SPM12 (www.fil.ion.ucl.ac.uk/spm/software/spm12), followed by skull stripping through multiplication with a brain binary mask, which was created in MRIcron (https://www.nitrc.org/projects/mricron/) based on the initial echo magnitude image from the GRE pulse sequence. QSM and T1WI, which had been co-registered and skull-stripped, then underwent an automated multi-atlas segmentation procedure using both QSM and T1 contrast to delineate bilateral regions of SN (29). Briefly, the automated multi-atlas segmentation procedure involves the use of a multi-atlas library consisting of 23 atlases with T1-weighted images, gradient echo magnitude images, and QSM images. Each atlas includes manually labeled regions of interest (ROIs), such as the SN, based on QSM contrast. The target QSM and T1WI are preprocessed, including scaling QSM values and bias correction of T1WI. The pipeline then maps the target images to the atlas space using affine transformations and large deformation diffeomorphic mapping (LDDMM) to account for local deformations. The manual segmentations from the atlases are warped into the target space, and a multi-atlas fusion algorithm combines these to create a target segmentation. This approach leverages both QSM and T1 contrasts, enhancing segmentation accuracy, particularly in iron-rich deep gray matter nuclei. This method has been made publicly available through the MRICloud platform (https://braingps.mricloud.org/), facilitating accessible analysis of QSM data (30). The subsequent steps involved measuring the average QSM values for the left and right SN. The bilateral average QSM values for both left and right sides were further computed for extended analysis.
Quantification of DTI-ALPS index
The DTI-ALPS index was computed as a measure of the glymphatic function, a method that is consistent with prior reports (4,31). To accomplish this task, all diffusion-weighted images were subjected to motion correction using the Eddymotion tool (https://github.com/nipreps/eddymotion) before being utilized for fitting the diffusion tensor model via FMRIB’s Diffusion Toolbox (https://fsl.fmrib.ox.ac.uk/fsl/fslwiki/FSL). This color-coded fractional anisotropy (FA) map along with diffusivity maps in the x-, y-, and z-axis directions were calculated. A location slice, in which deep medullary veins ran vertical to the ventricle body, was determined and categorized on the QSM map. The subsequent QSM images then underwent co-registration with b0 images. This was accomplished by rigidly registering the first echo magnitude image from the GRE pulse sequence to b0 images utilizing SPM12. According to previous research, the size of the ROI does not influence the DTI-ALPS index calculation (32). Therefore, four circular ROIs of equivalent diameter were independently positioned in the bilateral projection fiber and the association fiber, on the labeled color-coded FA map slice. This process was performed by a seasoned radiologist with seven years of experience, who was unaware of the underlying clinical data, by utilizing ITK-snap software (http://www.itksnap.org/pmwiki/pmwiki.php), as shown in Figure 2A. It is advisable to avoid placing the ROIs within T2-FLAIR lesions. Upon computing the ROIs’ diffusivities in the x-, y-, and z-axis directions on the labeled slice, the DTI-ALPS index for both the left and the right hemispheres was calculated based on the following formula: DTI-ALPS index = mean (Dxproj, Dxassoc)/mean (Dyproj, Dzassoc). The mean DTI-ALPS index for the bilateral hemisphere was subsequently derived by averaging the calculated indexes of the left and right DTI-ALPS.
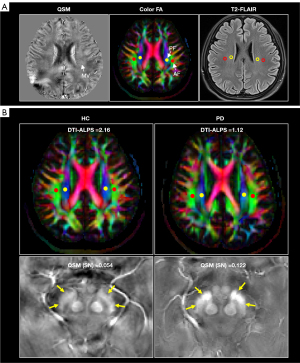
Statistical analysis
Statistical analysis was carried out utilizing the software SPSS 27.0 (IBM Corp., Armonk, NY, USA). Demographic and clinical traits, along with DTI-ALPS and QSM values, were recorded as either raw numbers or median values with the associated interquartile range. The Mann-Whitney U test served to highlight any differences in the DTI-ALPS and QSM values of SN between PD patients and HC participants. To evaluate the correlation between the MDS-UPDRS III and the DTI-ALPS and QSM values, a Spearman correlation analysis was employed. Binary logistic regression analysis was utilized in leveraging these parameters to generate a regression equation, thus determining the corresponding prediction probability for distinguishing PD from HC. Receiver operating characteristic (ROC) curves were plotted, with the area under the curve (AUC), sensitivity, and specificity metrics employed in assessing the diagnostic performance for PD. The DeLong tests were used for further comparison of the AUCs. A predetermined significance threshold was applied throughout the statistical analysis.
Results
Clinical and demographic characteristics of participants
After the selection process as shown in Figure 1, a total of 60 PD patients and 60 HC participants, matched by age and gender, were included in the study. From the pool of PD patients, 34 (56.7%) were male, with a median disease duration of 84.0 months. The median Hoehn and Yahr scale was 3.0, and the MDS-UPDRS III also showed a median value of 46.0. Table 1 presents the clinical characteristics, along with a comparison between the two groups. There was no significant variation found in terms of age and gender between the PD patients and the HC participants, with P values of 0.299 and 0.201, respectively.
Table 1
Characteristics | PD patients (n=60) | HC participants (n=60) | P value |
---|---|---|---|
Age (years) | 63.0 (58.5, 68.0) | 62.0 (57.0, 66.0) | 0.299 |
Gender (male/female) | 34/26 | 27/33 | 0.201 |
Disease duration (months) | 84.0 (61.5, 125.0) | NA | NA |
MDS-UPDRS III | 46.0 (37.6, 58.8) | NA | NA |
Hoehn and Yahr scale | 3.0 (2.5, 3.0) | NA | NA |
Data were presented as median (interquartile range) or number. PD, Parkinson’s disease; HC, healthy control; MDS-UPDRS, Movement Disorder Society-Unified Parkinson’s Disease Rating Scale; NA, not applicable.
Comparison between PD patients and HC participants
The representative HC participant and PD patient demonstrating the difference between HC and PD are shown in Figure 2B. Comparative analysis indicated significantly lower left, right, and average DTI-ALPS for PD patients when matched with HC participants (all P<0.001). A similar disparity was observed in the average QSM value of SN. The values for PD patients were significantly higher than those of HC participants for the left, right, and bilateral regions (P=0.018, 0.033, and 0.020, respectively). These results are represented in Table 2 and Figure 3.
Table 2
Metric | PD patients (n=60) | HC participants (n=60) | Z | P value |
---|---|---|---|---|
DTI-ALPS | ||||
Left DTI-ALPS | 1.41 (1.27, 1.53) | 1.52 (1.37, 1.75) | −3.690 | <0.001** |
Right DTI-ALPS | 1.33 (1.19, 1.59) | 1.55 (1.43, 1.72) | −4.304 | <0.001** |
Average DTI-ALPS | 1.38 (1.26, 1.53) | 1.52 (1.41, 1.71) | −4.325 | <0.001** |
QSM (ppm) | ||||
Left SN | 0.09 (0.07, 0.10) | 0.07 (0.07, 0.08) | −2.372 | 0.018* |
Right SN | 0.09 (0.08, 0.10) | 0.08 (0.07, 0.09) | −2.136 | 0.033* |
Bilateral SN | 0.09 (0.07, 0.10) | 0.08 (0.07, 0.09) | −2.336 | 0.020* |
Data were presented as median (interquartile range). *, P<0.05; **, P<0.01. PD, Parkinson’s disease; HC, healthy control; DTI-ALPS, diffusion tensor image analysis along the perivascular space; SN, substantia nigra; QSM, quantitative susceptibility mapping.
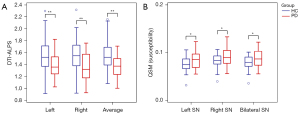
Logistic regression
The age, gender, bilateral average DTI-ALPS, and average QSM value of the bilateral SN were selected and incorporated into a binary logistic regression equation (Eq. [1]) to differentiate between PD and HC. The subsequent calculation yielded a prediction probability. Both the bilateral average DTI-ALPS and the bilateral average QSM value of SN contributed significantly to PD diagnosis (P<0.001 and P=0.004, respectively). The odds ratios (OR) and 95% confidence intervals (CI) were 0.001 (0.000–0.021) for the bilateral average DTI-ALPS and 1.573 (1.153–2.145) for the average QSM of bilateral SN, with each 0.01 increase in QSM value.
The logistic regression equation of the bilateral average DTI-ALPS and the average QSM value of the bilateral SN predicts the occurrence of PD.
In this equation, ‘p’ represents the probability of PD being the case, whereas ‘(1−p)’ signifies the probability that PD is not the case.
Evaluation and comparison of diagnostic performance
In Table 3, we present the results of ROC analyses of the DTI-ALPS and QSM of SN in differentiating PD from HC. The associated ROC curves are visualized in Figure 4. The average values of left, right, and bilateral DTI-ALPS and QSM of SN in diagnosing PD had AUCs ranging from 0.613 to 0.729. The bilateral average DTI-ALPS demonstrated an AUC of 0.729, with a diagnostic sensitivity of 71.67% and a specificity of 65.00%. Correspondingly, the bilateral average QSM of SN displayed an AUC of 0.624, with a sensitivity of 33.33% and a specificity of 96.67%. The inclusion of age, sex, bilateral average DTI-ALPS, and bilateral average QSM of SN in a logistic regression equation improved the predictive probability, yielding the highest AUC of 0.801, and establishing a sensitivity and specificity of 68.33% and 80.00%, respectively.
Table 3
Metric | AUC (95% CI) | Threshold value | Sensitivity | Specificity |
---|---|---|---|---|
DTI-ALPS | ||||
Left DTI-ALPS | 0.695 (0.605–0.776) | 1.433 | 63.33% | 66.67% |
Right DTI-ALPS | 0.728 (0.639–0.805) | 1.402 | 63.33% | 73.33% |
Average DTI-ALPS | 0.729 (0.640–0.806) | 1.483 | 71.67% | 65.00% |
QSM (ppm) | ||||
Left SN | 0.626 (0.533–0.712) | 0.089 | 45.00% | 83.33% |
Right SN | 0.613 (0.520–0.701) | 0.096 | 41.67% | 83.33% |
Bilateral SN | 0.624 (0.531–0.710) | 0.099 | 33.33% | 96.67% |
Combination | 0.801 (0.719–0.869) | 0.579 | 68.33% | 80.00% |
Note that the term ‘combination’ refers to the predictive probability derived from the binary logistic regression equation that combines age, gender, bilateral average DTI-ALPS and the average QSM value of the bilateral SN to distinguish PD from HC. DTI-ALPS, diffusion tensor image analysis along the perivascular space; QSM, quantitative susceptibility mapping; SN, substantia nigra; PD, Parkinson’s disease; HC, healthy control; AUC, area under the curve; CI, confidence interval.
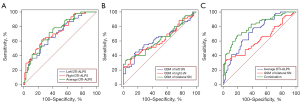
The DeLong tests revealed that in discriminating PD from HC, the AUC of the bilateral average DTI-ALPS exceeded that of the bilateral average QSM of SN. However, this did not achieve statistical significance (P=0.122). Nonetheless, the AUC of the prediction probability of the logistic regression equation markedly surpassed those of bilateral average DTI-ALPS (P=0.034), left DTI-ALPS (P=0.008), bilateral average QSM of SN (P<0.001), left QSM of SN (P<0.001), and right QSM of SN (P<0.001). These findings are detailed in Table 4.
Table 4
AUC comparisons | P value |
---|---|
Average DTI-ALPS - Average QSM of bilateral SN | 0.122 |
Average DTI-ALPS - QSM of left SN | 0.121 |
Average DTI-ALPS - QSM of right SN | 0.091 |
Left DTI-ALPS - Average QSM of bilateral SN | 0.301 |
Left DTI-ALPS - QSM of left SN | 0.308 |
Left DTI-ALPS - QSM of right SN | 0.239 |
Right DTI-ALPS - Average QSM of bilateral SN | 0.126 |
Right DTI-ALPS - QSM of left SN | 0.125 |
Right DTI-ALPS - QSM of right SN | 0.096 |
Combination - Average DTI-ALPS | 0.034* |
Combination - Left DTI-ALPS | 0.008** |
Combination - Right DTI-ALPS | 0.054 |
Combination - Average QSM of bilateral SN | <0.001** |
Combination - QSM of left SN | <0.001** |
Combination - QSM of right SN | <0.001** |
*, P<0.05; **, P<0.01. Note that the term ‘combination’ refers to the predictive probability derived from a binary logistic regression equation that incorporates age, gender, bilateral average DTI-ALPS, and average QSM value of bilateral SN, used for distinguishing PD from HC. AUC, area under the curve; PD, Parkinson’s disease; HC, healthy control; DTI-ALPS, diffusion tensor image analysis along the perivascular space; QSM, quantitative susceptibility mapping; SN, substantia nigra.
Correlation of MDS-UPDRS III with DTI-ALPS and QSM values of SN
Table 5 presents the results of the correlation between MDS-UPDRS III and the bilateral average DTI-ALPS values, as well as the bilateral average QSM value of SN. The corresponding scatter diagrams can be viewed in Figure 5. Significant correlations were identified between MDS-UPDRS III and the QSM values of the right SN (rho =−0.285, P=0.029), in addition to the bilateral average QSM values of SN (rho =−0.276, P=0.034). However, no significant correlation was observed between the MDS-UPDRS III and the QSM values of the left SN (rho =−0.211, P=0.108). No significant correlation was also found between MDS-UPDRS III and the left, right, or bilateral average DTI-ALPS (P>0.05 for all).
Table 5
Metric | rho | P value |
---|---|---|
DTI-ALPS | ||
Left DTI-ALPS | −0.155 | 0.240 |
Right DTI-ALPS | 0.079 | 0.552 |
Average DTI-ALPS | −0.025 | 0.850 |
QSM of SN (ppm) | ||
Left QSM | −0.211 | 0.108 |
Right QSM | −0.285 | 0.029* |
Average QSM
−0.276
0.034*
*, P<0.05. MDS-UPDRS, Movement Disorder Society-Unified Parkinson’s Disease Rating Scale; DTI-ALPS, diffusion tensor image analysis along the perivascular space; QSM, quantitative susceptibility mapping; SN, substantia nigra.
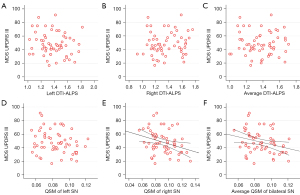
Discussion
In this study, we assessed glymphatic dysfunction using DTI-ALPS and measured SN iron accumulation using QSM. Our aim was to diagnose and assess the severity of PD. Additionally, we combined these assessments to distinguish PD patients from HC. Our findings were threefold: (I) we observed significant glymphatic dysfunction and increased iron accumulation in the SN of PD patients; (II) glymphatic dysfunction offered higher diagnostic accuracy when differentiating PD patients from HC, whereas SN iron accumulation demonstrated a stronger correlation with the MDS-UPDRS III score; (III) the combined assessment of glymphatic dysfunction and SN iron accumulation enhanced the diagnostic accuracy of PD.
The DTI-ALPS index is a novel non-invasive method developed for assessing brain glymphatic functionality. This index is attained by the ratio calculation of diffusion in the perivascular direction along the medullary veins to the diffusion perpendicular to the primary fiber tract direction, thereby providing an indicative measure of global glymphatic functionality (31,33). Prior to this, Chen et al. computed the DTI-ALPS index in the dominant left hemisphere, particularly in PD patients, leading to a key finding of a decreased index throughout different stages of the disease (34). In a similar vein to these findings, our study mirrored glymphatic dysfunction in both hemispheres in PD patients, which is presumably due to their advanced disease stage as indicated by a median score of 3 on the Hoehn and Yahr scale. In the context of PD, the potential breakdown of perivascular clearance pathways is considered a facilitator for α-synuclein accumulation (2,35), a pivotal pathological characteristic of PD implicated in deleterious effects on dopaminergic neurons. Additionally, glymphatic clearance dysfunction may instigate the degeneration of dopaminergic neurons as a secondary effect of heightened α-synuclein accumulation.
In this study, QSM values were observed to be significantly elevated in the SN of patients with PD, when compared with HC participants. This suggests a heightened level of iron deposition within the SN of PD patients, which is generally consistent with previous studies (16,19,36-38). The accumulation of iron in the SN is pivotal to the evolution and progression of PD. An excessive deposition of iron can instigate neurotoxicity and may even lead to iron-mediated cell death, resulting in the loss of dopaminergic neurons through various mechanisms, including oxidative stress, the aggregation of α-synuclein, and neuroinflammation. Thereafter, the reduction of dopaminergic neurons leads to diminished dopamine secretion in the SN. This sequence of events culminates in motor symptoms such as resting tremor, bradykinesia, rigidity, and postural instability, which are commonly observed in PD patients. Of note, in this study, the diagnostic accuracy of QSM values in the SN was lower than that reported in some other studies (19). This discrepancy could be due to several factors. Our QSM imaging protocol, primarily designed for clinical practicality, may not have achieved the same resolution or TEs as studies that reported higher accuracies. Additionally, although the automated ROI delineation used in this study reduces bias, it might not capture the same level of detail as the manual delineation methods employed in some high-accuracy studies. Furthermore, the inherent variability in the PD population and disease progression could also affect diagnostic accuracy. Despite these considerations, our study provides a practical approach to diagnostic accuracy that balances clinical feasibility, making it valuable for the adoption of these imaging techniques in routine clinical practice.
Regarding the loss of swallow tail sign (STS), a marker indicating iron deposition within the nigrosome 1 territory of the SN, most existing literature (39,40) uses GRE sequences with a slice thickness of approximately 1 mm for assessment. In contrast, our QSM sequence had a slice thickness of 2 mm, which may not be optimal for accurately assessing this STS sign (41). Moreover, although some studies have found that the diagnostic accuracy of the loss of STS is high (86.66%) in diagnosing PD or progressive supranuclear palsy (40), others have found that the abnormal STS is not a reliable biomarker for idiopathic PD (42). Consequently, we did not include an evaluation of the loss of STS in our research data.
This study importantly identified higher diagnostic accuracy in distinguishing PD from HC through the detection of glymphatic dysfunction as compared to the identification of iron accumulation within the SN. This could be attributed to the tendency of glymphatic dysfunction to manifest in the early stages of PD, whereas iron accumulation in the SN typically appears during later stages. The resultant decrease in glymphatic functionality thus emerges as more significant than the associated increase in SN iron accumulation. Consequently, the diagnostic efficacy of identifying glymphatic dysfunction surpasses that of detecting increased iron accumulation in the SN. Nevertheless, the overlap in AUC values for both conditions registered as statistically insignificant within this study. This observation could possibly result from an inadequate sample size. The study further established a stronger correlation between iron accumulation in the SN and clinical motor symptoms. This may be explained by the fact that increased iron accumulation signifies the degeneration and necrosis of dopaminergic neurons. Consequentially, a significant reduction in dopamine levels corresponds more directly with Parkinson’s motor symptoms. On the contrary, ailments such as glymphatic dysfunction that manifest in the early stages of PD are not necessarily accompanied by dopaminergic neuronal degeneration and necrosis. Hence, their correlation with motor symptoms may not be as direct or as substantial as that of iron accumulation.
Additionally, our study discerned that the concurrent identification of glymphatic dysfunction and iron accumulation in the SN enhances the diagnostic accuracy of PD. This effectively suggests that the consideration of both glymphatic dysfunction and SN iron accumulation provides a complementary diagnostic approach that potentially compounds the precision of PD diagnosis. The integration of DTI-ALPS and QSM in clinical practice could significantly enhance the diagnostic accuracy of PD, facilitating earlier and more confident diagnoses. This non-invasive approach could improve patient compliance and reduce risks associated with diagnostic procedures, while also enabling a more personalized treatment strategy tailored to individual patient needs. The adoption of DTI-ALPS and QSM may ultimately lead to improved patient outcomes and quality of life.
In addition to QSM values in the SN, QSM values in the striatum or cerebral cortex are also potential imaging biomarkers that can reveal increased brain iron load in patients with PD, and further demonstrate that PD patients with cognitive impairments may have a unique brain iron load pattern (37,43,44). Moreover, some previous papers have reported the potential of advanced MRI techniques for neurodegenerative biomarkers, such as arterial spin labeling based blood-brain barrier imaging. Physiologically, the blood-brain barrier is an integral component of the glymphatic system, and it plays a crucial role in maintaining brain homeostasis by clearing metabolic waste and other neurotoxic compounds from the central nervous system into meningeal lymphatics and systemic circulation (45). Consequently, alterations in the blood-brain barrier may also occur in neurodegenerative diseases such as PD. However, these possibilities require further research to be fully understood.
Our study possesses several limitations. Initially, this study was conducted within a single center. Hence, the results warrant further validation within future multi-centered studies. Secondly, this study was designed retrospectively; our QSM imaging protocol, primarily designed for clinical practicality, may not have achieved the same resolution or TEs as more specialized studies. Consequently, this could limit our ability to achieve high diagnostic accuracy and may not be the most suitable for assessing the loss of the STS. In light of these considerations, we plan to conduct prospective studies in the future, optimizing sequence parameters to enhance diagnostic accuracy. Finally, our study employed the use of the DTI-ALPS index as a surrogate for glymphatic function, rather than measuring the glymphatic flux or clearance rate of metabolic waste products in vivo. Although this indirect index offers a simplistic approach, future investigations employing more direct and sensitive methods for assessing glymphatic function are recommended.
Conclusions
Our study indicates that DTI-ALPS and QSM may potentially serve as biomarkers and tools for diagnosing and assessing the severity of PD. Notably, we observed a significant glymphatic dysfunction and increased iron accumulation in the SN among PD patients. The former appeared to have a higher diagnostic accuracy in distinguishing PD from HC. Meanwhile, the latter showed a more robust correlation with motor symptoms in PD. Furthermore, integrating these two markers markedly enhanced the diagnostic performance. However, additional studies are required to substantiate our findings.
Acknowledgments
None.
Footnote
Reporting Checklist: The authors have completed the STROBE reporting checklist. Available at https://qims.amegroups.com/article/view/10.21037/qims-24-1605/rc
Funding: This work was supported by grants from
Conflicts of Interest: All authors have completed the ICMJE uniform disclosure form (available at https://qims.amegroups.com/article/view/10.21037/qims-24-1605/coif). Y.X.J.W. serves as the Editor-In-Chief of Quantitative Imaging in Medicine and Surgery. The other authors have no conflicts of interest to declare.
Ethical Statement: The authors are accountable for all aspects of the work in ensuring that questions related to the accuracy or integrity of any part of the work are appropriately investigated and resolved. The study was conducted in accordance with the Declaration of Helsinki (as revised in 2013) and was approved by the Ethics Committee of the Fujian Medical University Union Hospital (approval No. 2023KJCX058). Informed consent was provided by all individual participants.
Open Access Statement: This is an Open Access article distributed in accordance with the Creative Commons Attribution-NonCommercial-NoDerivs 4.0 International License (CC BY-NC-ND 4.0), which permits the non-commercial replication and distribution of the article with the strict proviso that no changes or edits are made and the original work is properly cited (including links to both the formal publication through the relevant DOI and the license). See: https://creativecommons.org/licenses/by-nc-nd/4.0/.
References
- Arya R, Haque AKMA, Shakya H, Billah MM, Parvin A, Rahman MM, Sakib KM, Faruquee HM, Kumar V, Kim JJ. Parkinson's Disease: Biomarkers for Diagnosis and Disease Progression. Int J Mol Sci 2024;25:12379. [Crossref] [PubMed]
- Zou W, Pu T, Feng W, Lu M, Zheng Y, Du R, Xiao M, Hu G. Blocking meningeal lymphatic drainage aggravates Parkinson's disease-like pathology in mice overexpressing mutated α-synuclein. Transl Neurodegener 2019;8:7. [Crossref] [PubMed]
- Ding XB, Wang XX, Xia DH, Liu H, Tian HY, Fu Y, Chen YK, Qin C, Wang JQ, Xiang Z, Zhang ZX, Cao QC, Wang W, Li JY, Wu E, Tang BS, Ma MM, Teng JF, Wang XJ. Impaired meningeal lymphatic drainage in patients with idiopathic Parkinson's disease. Nat Med 2021;27:411-8. [Crossref] [PubMed]
- Carotenuto A, Cacciaguerra L, Pagani E, Preziosa P, Filippi M, Rocca MA. Glymphatic system impairment in multiple sclerosis: relation with brain damage and disability. Brain 2022;145:2785-95. [Crossref] [PubMed]
- Zhang W, Zhou Y, Wang J, Gong X, Chen Z, Zhang X, Cai J, Chen S, Fang L, Sun J, Lou M. Glymphatic clearance function in patients with cerebral small vessel disease. Neuroimage 2021;238:118257. [Crossref] [PubMed]
- Cai X, Chen Z, He C, Zhang P, Nie K, Qiu Y, Wang L, Wang L, Jing P, Zhang Y. Diffusion along perivascular spaces provides evidence interlinking compromised glymphatic function with aging in Parkinson's disease. CNS Neurosci Ther 2023;29:111-21. [Crossref] [PubMed]
- Shen T, Yue Y, Ba F, He T, Tang X, Hu X, Pu J, Huang C, Lv W, Zhang B, Lai HY. Diffusion along perivascular spaces as marker for impairment of glymphatic system in Parkinson's disease. NPJ Parkinsons Dis 2022;8:174. [Crossref] [PubMed]
- Meng JC, Shen MQ, Lu YL, Feng HX, Chen XY, Xu DQ, Wu GH, Cheng QZ, Wang LH, Gui Q. Correlation of glymphatic system abnormalities with Parkinson's disease progression: a clinical study based on non-invasive fMRI. J Neurol 2024;271:457-71. [Crossref] [PubMed]
- Qin Y, He R, Chen J, Zhou X, Zhou X, Liu Z, Xu Q, Guo JF, Yan XX, Jiang N, Liao W, Taoka T, Wang D, Tang B. Neuroimaging uncovers distinct relationships of glymphatic dysfunction and motor symptoms in Parkinson's disease. J Neurol 2023;270:2649-58. [Crossref] [PubMed]
- Wood KH, Nenert R, Miften AM, Kent GW, Sleyster M, Memon RA, Joop A, Pilkington J, Memon AA, Wilson RN, Catiul C, Szaflarski J, Amara AW. Diffusion Tensor Imaging-Along the Perivascular-Space Index Is Associated with Disease Progression in Parkinson's Disease. Mov Disord 2024;39:1504-13. [Crossref] [PubMed]
- Ward RJ, Zucca FA, Duyn JH, Crichton RR, Zecca L. The role of iron in brain ageing and neurodegenerative disorders. Lancet Neurol 2014;13:1045-60. [Crossref] [PubMed]
- Thomas GEC, Leyland LA, Schrag AE, Lees AJ, Acosta-Cabronero J, Weil RS. Brain iron deposition is linked with cognitive severity in Parkinson's disease. J Neurol Neurosurg Psychiatry 2020;91:418-25. [Crossref] [PubMed]
- Cozzi A, Orellana DI, Santambrogio P, Rubio A, Cancellieri C, Giannelli S, Ripamonti M, Taverna S, Di Lullo G, Rovida E, Ferrari M, Forni GL, Fiorillo C, Broccoli V, Levi S. Stem Cell Modeling of Neuroferritinopathy Reveals Iron as a Determinant of Senescence and Ferroptosis during Neuronal Aging. Stem Cell Reports 2019;13:832-46. [Crossref] [PubMed]
- Ndayisaba A, Kaindlstorfer C, Wenning GK. Iron in Neurodegeneration - Cause or Consequence? Front Neurosci 2019;13:180. [Crossref] [PubMed]
- Dexter DT, Jenner P, Schapira AH, Marsden CD. Alterations in levels of iron, ferritin, and other trace metals in neurodegenerative diseases affecting the basal ganglia. The Royal Kings and Queens Parkinson's Disease Research Group. Ann Neurol 1992;32:S94-100. [Crossref] [PubMed]
- Deistung A, Schweser F, Reichenbach JR. Overview of quantitative susceptibility mapping. NMR Biomed 2017;
- Langkammer C, Schweser F, Krebs N, Deistung A, Goessler W, Scheurer E, Sommer K, Reishofer G, Yen K, Fazekas F, Ropele S, Reichenbach JR. Quantitative susceptibility mapping (QSM) as a means to measure brain iron? A post mortem validation study. Neuroimage 2012;62:1593-9. [Crossref] [PubMed]
- Zang Z, Song T, Li J, Yan S, Nie B, Mei S, Ma J, Yang Y, Shan B, Zhang Y, Lu J. Modulation effect of substantia nigra iron deposition and functional connectivity on putamen glucose metabolism in Parkinson's disease. Hum Brain Mapp 2022;43:3735-44. [Crossref] [PubMed]
- Murakami Y, Kakeda S, Watanabe K, Ueda I, Ogasawara A, Moriya J, Ide S, Futatsuya K, Sato T, Okada K, Uozumi T, Tsuji S, Liu T, Wang Y, Korogi Y. Usefulness of quantitative susceptibility mapping for the diagnosis of Parkinson disease. AJNR Am J Neuroradiol 2015;36:1102-8. [Crossref] [PubMed]
- Lee H, Baek SY, Chun SY, Lee JH, Cho H. Specific visualization of neuromelanin-iron complex and ferric iron in the human post-mortem substantia nigra using MR relaxometry at 7T. Neuroimage 2018;172:874-85. [Crossref] [PubMed]
- Wang C, Foxley S, Ansorge O, Bangerter-Christensen S, Chiew M, Leonte A, Menke RA, Mollink J, Pallebage-Gamarallage M, Turner MR, Miller KL, Tendler BC. Methods for quantitative susceptibility and R2* mapping in whole post-mortem brains at 7T applied to amyotrophic lateral sclerosis. Neuroimage 2020;222:117216. [Crossref] [PubMed]
- Azuma M, Hirai T, Yamada K, Yamashita S, Ando Y, Tateishi M, Iryo Y, Yoneda T, Kitajima M, Wang Y, Yamashita Y. Lateral Asymmetry and Spatial Difference of Iron Deposition in the Substantia Nigra of Patients with Parkinson Disease Measured with Quantitative Susceptibility Mapping. AJNR Am J Neuroradiol 2016;37:782-8. [Crossref] [PubMed]
- He N, Ling H, Ding B, Huang J, Zhang Y, Zhang Z, Liu C, Chen K, Yan F. Region-specific disturbed iron distribution in early idiopathic Parkinson's disease measured by quantitative susceptibility mapping. Hum Brain Mapp 2015;36:4407-20. [Crossref] [PubMed]
- Postuma RB, Berg D, Stern M, Poewe W, Olanow CW, Oertel W, Obeso J, Marek K, Litvan I, Lang AE, Halliday G, Goetz CG, Gasser T, Dubois B, Chan P, Bloem BR, Adler CH, Deuschl G. MDS clinical diagnostic criteria for Parkinson's disease. Mov Disord 2015;30:1591-601. [Crossref] [PubMed]
- Rajna Z, Mattila H, Huotari N, Tuovinen T, Krüger J, Holst SC, Korhonen V, Remes AM, Seppänen T, Hennig J, Nedergaard M, Kiviniemi V. Cardiovascular brain impulses in Alzheimer's disease. Brain 2021;144:2214-26. [Crossref] [PubMed]
- Li W, Avram AV, Wu B, Xiao X, Liu C. Integrated Laplacian-based phase unwrapping and background phase removal for quantitative susceptibility mapping. NMR Biomed 2014;27:219-27. [Crossref] [PubMed]
- Wu B, Li W, Guidon A, Liu C. Whole brain susceptibility mapping using compressed sensing. Magn Reson Med 2012;67:137-47. [Crossref] [PubMed]
- Wei H, Dibb R, Zhou Y, Sun Y, Xu J, Wang N, Liu C. Streaking artifact reduction for quantitative susceptibility mapping of sources with large dynamic range. NMR Biomed 2015;28:1294-303. [Crossref] [PubMed]
- Li X, Chen L, Kutten K, Ceritoglu C, Li Y, Kang N, Hsu JT, Qiao Y, Wei H, Liu C, Miller MI, Mori S, Yousem DM, van Zijl PCM, Faria AV. Multi-atlas tool for automated segmentation of brain gray matter nuclei and quantification of their magnetic susceptibility. Neuroimage 2019;191:337-49. [Crossref] [PubMed]
- Mori S, Wu D, Ceritoglu C, Li Y, Kolasny A, Vaillant MA, Faria AV, Oishi K, Miller MI. MRICloud: Delivering High-Throughput MRI Neuroinformatics as Cloud-Based Software as a Service. Computing in Science & Engineering 2016;18:21-35.
- Taoka T, Masutani Y, Kawai H, Nakane T, Matsuoka K, Yasuno F, Kishimoto T, Naganawa S. Evaluation of glymphatic system activity with the diffusion MR technique: diffusion tensor image analysis along the perivascular space (DTI-ALPS) in Alzheimer's disease cases. Jpn J Radiol 2017;35:172-8. [Crossref] [PubMed]
- Taoka T, Ito R, Nakamichi R, Kamagata K, Sakai M, Kawai H, Nakane T, Abe T, Ichikawa K, Kikuta J, Aoki S, Naganawa S. Reproducibility of diffusion tensor image analysis along the perivascular space (DTI-ALPS) for evaluating interstitial fluid diffusivity and glymphatic function: CHanges in Alps index on Multiple conditiON acquIsition eXperiment (CHAMONIX) study. Jpn J Radiol 2022;40:147-58. [Crossref] [PubMed]
- McKnight CD, Trujillo P, Lopez AM, Petersen K, Considine C, Lin YC, Yan Y, Kang H, Donahue MJ, Claassen DO. Diffusion along perivascular spaces reveals evidence supportive of glymphatic function impairment in Parkinson disease. Parkinsonism Relat Disord 2021;89:98-104. [Crossref] [PubMed]
- Chen HL, Chen PC, Lu CH, Tsai NW, Yu CC, Chou KH, Lai YR, Taoka T, Lin WC. Associations among Cognitive Functions, Plasma DNA, and Diffusion Tensor Image along the Perivascular Space (DTI-ALPS) in Patients with Parkinson's Disease. Oxid Med Cell Longev 2021;2021:4034509. [Crossref] [PubMed]
- Sundaram S, Hughes RL, Peterson E, Müller-Oehring EM, Brontë-Stewart HM, Poston KL, Faerman A, Bhowmick C, Schulte T. Establishing a framework for neuropathological correlates and glymphatic system functioning in Parkinson's disease. Neurosci Biobehav Rev 2019;103:305-15. [Crossref] [PubMed]
- He N, Langley J, Huddleston DE, Chen S, Huang P, Ling H, Yan F, Hu X. Increased iron-deposition in lateral-ventral substantia nigra pars compacta: A promising neuroimaging marker for Parkinson's disease. Neuroimage Clin 2020;28:102391. [Crossref] [PubMed]
- Uchida Y, Kan H, Sakurai K, Inui S, Kobayashi S, Akagawa Y, Shibuya K, Ueki Y, Matsukawa N. Magnetic Susceptibility Associates With Dopaminergic Deficits and Cognition in Parkinson's Disease. Mov Disord 2020;35:1396-405. [Crossref] [PubMed]
- Yan Y, Wang Z, Wei W, Yang Z, Guo L, Wang Z, Wei X. Correlation of brain iron deposition and freezing of gait in Parkinson's disease: a cross-sectional study. Quant Imaging Med Surg 2023;13:7961-72. [Crossref] [PubMed]
- Cheng Z, He N, Huang P, Li Y, Tang R, Sethi SK, Ghassaban K, Yerramsetty KK, Palutla VK, Chen S, Yan F, Haacke EM. Imaging the Nigrosome 1 in the substantia nigra using susceptibility weighted imaging and quantitative susceptibility mapping: An application to Parkinson's disease. Neuroimage Clin 2020;25:102103. [Crossref] [PubMed]
- Gupta R, Kumar G, Kumar S, Thakur B, Tiwari R, Verma AK. The Swallow Tail Sign of Substantia Nigra: A Case-Control Study to Establish Its Role in Diagnosis of Parkinson Disease on 3T MRI. J Neurosci Rural Pract 2022;13:181-5. [Crossref] [PubMed]
- Shams S, Fällmar D, Schwarz S, Wahlund LO, van Westen D, Hansson O, Larsson EM, Haller S. MRI of the Swallow Tail Sign: A Useful Marker in the Diagnosis of Lewy Body Dementia? AJNR Am J Neuroradiol 2017;38:1737-41. [Crossref] [PubMed]
- Kim DS, Tung GA, Akbar U, Friedman JH. The evaluation of the swallow tail sign in patients with parkinsonism and gait disorders. J Neurol Sci 2021;428:117581. [Crossref] [PubMed]
- Uchida Y, Kan H, Sakurai K, Arai N, Kato D, Kawashima S, Ueki Y, Matsukawa N. Voxel-based quantitative susceptibility mapping in Parkinson's disease with mild cognitive impairment. Mov Disord 2019;34:1164-73. [Crossref] [PubMed]
- Uchida Y, Kan H, Sakurai K, Oishi K, Matsukawa N. Quantitative susceptibility mapping as an imaging biomarker for Alzheimer's disease: The expectations and limitations. Front Neurosci 2022;16:938092. [Crossref] [PubMed]
- Uchida Y, Kan H, Sakurai K, Oishi K, Matsukawa N. Contributions of blood-brain barrier imaging to neurovascular unit pathophysiology of Alzheimer's disease and related dementias. Front Aging Neurosci 2023;15:1111448. [Crossref] [PubMed]