Association of diffusion tensor imaging along the perivascular space index with cognitive impairment in type 2 diabetes mellitus
Introduction
Type 2 diabetes mellitus (T2DM) comprises a group of metabolic diseases characterized by abnormal blood glucose levels due to insufficient insulin secretion and utilization. T2DM is associated with a series of chronic complications, including microvascular lesions, macrovascular lesions, neuropathy, diabetic foot, and other diseases (1). Cognitive impairment also is a severe complication of T2DM (2), which may be caused by brain microvascular lesions due to metabolic disorders of glucose and lipids. T2DM increases the risk of dementia by nearly 37% and the risk of mild cognitive impairment (MCI) by about 20% (3).
In 2012, Iliff et al. (4) were first to report the existence of the glymphatic system within the central nervous system (CNS) in a mouse model using tracer tracking methods. The glymphatic system is a pathway essential to clearing metabolic waste from the brain. The glymphatic system consists of periarterial cerebrospinal fluid (CSF) inflow running in the same direction as blood flow. The CSF mixes with interstitial fluid (ISF) in a process facilitated by aquaporin-4 (AQP4) water channels (5,6). The mixture of CSF and ISF leaves the brain via the perivenous space and along cranial and spinal nerves (7). The AQP4 is a key component of the glymphatic system; in one study, when the polarity of AQP4 in diabetic rats was destroyed, there was a decrease in the efficiency of metabolic waste clearance in the brain (8). Taoka et al. (9) reported that diffusion tensor imaging analysis along the perivascular space (DTI-ALPS) method can assess the clearance function of the glymphatic system by measuring the diffusion rate of water molecules in different directions within the perivascular space surrounding the deep medullary veins. This method has also been widely used in the research of various neurological diseases such as Alzheimer disease (10), Parkinson disease (11), and multiple sclerosis (12).
Some studies have indicated that alterations in glymphatic system function are associated with cognitive impairments caused by diseases such as Parkinson’ disease, insomnia, and age-related hearing loss (13-15). It has also been confirmed that T2DM may cause damage to the CNS, leading to MCI and dementia. Previous animal and clinical studies have found that glymphatic system activity is reduced in the T2DM condition (16-18); however, thus far, little research had been conducted on the glymphatic system in patients with T2DM with MCI (T2DM-MCI). MCI is a reversible intermediate state between normal and dementia, while dementia is an irreversible stage (19,20). Therefore, early detection and effective treatment of T2DM-MCI are particularly important.
For this study, we hypothesized the following: (I) the DTI-ALPS index is lower in patients with T2DM than in healthy controls (HCs), especially in patients with T2DM-MCI; (II) the DTI-ALPS index is associated with white-matter damage and cognitive impairment in patients with T2DM. We present this article in accordance with the STROBE reporting checklist (available at https://qims.amegroups.com/article/view/10.21037/qims-24-1591/rc).
Methods
Participants
In this study, we enrolled patients with T2DM diagnosed in The Second Affiliated Hospital of Nanchang University from June 2022 to April 2024. All participants were over 40 years old and had at least 6 years of education. The inclusion criteria for patients with T2DM were as follows: (I) meeting the diabetes diagnosis criteria issued by the World Health Organization in 1999 (21); (II) a history of diabetes for at least 1 year; and (III) right-handedness. The exclusion criteria were as follows: (I) brain tumors, trauma, or neurodegenerative diseases; (II) severe depression, schizophrenia, sleep disorders, or other diseases affecting the function of the glymphatic system; (III) dementia and any other systemic diseases unrelated to diabetes but affecting cognitive function; and (IV) contraindications to magnetic resonance imaging (MRI) examination and large motion artifacts. All participants were assessed for cognitive function using a Mini-Mental State Examination (MMSE) and Montreal Cognitive Assessment (MoCA) (20). As all participants had more than 6 years of education, the inclusion criteria for MCI were a MoCA score <26 and a simple MMSE score >24 (21). We recruited 35 HCs matched for age, sex, and education. This study was conducted in accordance with the Declaration of Helsinki (as revised in 2013) and was approved by the Ethics Committee of The Second Affiliated Hospital of Nanchang University (No. O-2024-73). All participants signed a written consent form.
MRI acquisition
All participants underwent scanning with the same 3.0-T MRI device (SIGNA Architect; GE HealthCare, Chicago, IL, USA) equipped with a 64-channel standard head and neck coil. During the MRI examination, all participants were kept in a clear state, and their heads were stabilized with foam pillows to reduce motion artifacts. The imaging parameters of the 3D T1-weighted imaging were as follows: repetition time/echo time (TR/TE), 2,000/2.47 ms, flip angle, 12°; field of view (FOV), 230 mm × 230 mm; slice thickness, 1.0 mm; number of slices, 176; matrix size, 256×256; and voxel size, 0.9 mm × 0.9 mm × 1.0 mm. DTI was acquired using a spin-echo echo-planar imaging (EPI) sequence under the following parameters: TR, 8,200 ms; TE, 69 ms; FOV, 224 mm × 224 mm; matrix size, 122×122; slice thickness, 2.0 mm; number of slices, 70; voxel size, 1.8 mm × 1.8 mm × 2.0 mm; b-value, 0 and 1,000 s/mm2; number of b0, 2; and number of diffusion gradient directions, 64. Finally, the susceptibility-weighted imaging (SWI) imaging parameters were as follows: TR, 42.7 ms; TE, 7.5 ms; FOV, 220 mm × 220 mm; matrix size, 256×256; slice thickness, 2.0; number of slices, 70; and voxel size, 0.86 mm × 0.86 mm × 2.0 mm.
DTI processing
All DTI data of the T2DM and HC participants were processed and analyzed using DSI Studio software (version 2021). Initially, the participants’ DTI data were converted from the Digital Imaging and Communications in Medicine (DICOM) format to the SRC file format specifically used by DSI Studio software. Subsequently, each generated SRC file was checked through a quality control procedure, the participants with significant motion artifacts were excluded, and corrections were made for eddy-current and phase-distortion artifacts. A mask was created using the built-in functions of thresholding, smoothing, extension, erosion, and debris sorting provided by DSI Studio to filter out the background area, improve reconstruction efficiency, and facilitate further visualization. The DTI reconstruction method was used to characterize the main direction of water diffusion, which was followed by fiber tracking under default parameters. We used the default warping in the DSI Studio software to register individual DTI to the Human Connectome Project (HCP) 1065 template and evaluated the extremum point for each white-matter fiber. The HCP 1065 template was generated using DWI data from a cohort of 1,065 HCP participants, aligned to the standard Montreal Neurological Institute (MNI) reference space (22). We used DSI Studio software to perform fiber tracking across the whole-brain region based on a deterministic fiber tracking algorithm, calculating measures of whole-brain DTI parameters, including the average values of whole-brain fractional anisotropy (FA), mean diffusivity (MD), axial diffusivity (AD), and radial diffusivity (RD). Subsequently, a connectivity matrix was generated for each participant, and the global structural connectivity was analyzed using graph theoretical analysis methods. We used weighted network measures and normalized the connectivity matrix, yielding a maximum value of 1. We used the automated anatomical labelling atlas 3 provided by DSI Studio to match the participant data and performed spatial normalization using a linear transformation. Finally, from the connectivity matrices at a set density, we derived measures such as the average clustering coefficient (Cp), characteristic path length (Lp), global efficiency (Eglob), and small-world index (Sigma) to evaluate the global structural connectivity.
DTI-ALPS index calculation
The acquisition process of the DTI-ALPS index is provided in Figure 1, and it is also described in our previous paper (23). We performed rigid registration between participants’ DTI images and SWI sequences, which facilitated the identification of deep medullary veins perpendicular to the walls of the lateral ventricles (along the x-axis), thereby guiding the placement of regions of interest (ROIs) on bilateral association and projection fibers. The projection fibers adjacent to the lateral ventricle run in the head-to-foot (z-axis) direction, and the superior longitudinal fascicle representing the association fibers runs in the anterior-posterior (y-axis) direction outside the projection fibers (24). Two neuroradiologists used the DSI Studio software to draw four ROIs (comprising 3×3 voxels) in the same slice of the FA-weighted color map. Two ROIs were drawn within the area of projection fibers, where the major fibers were oriented along the z-axis. Additionally, another two ROIs were drawn within the area of association fibers, with the major fibers running along the y-axis in the bilateral hemispheres. The diffusivity parameters of the projection and association fibers extracted along the x-, y-, and z-axes of the ROI voxel level were obtained from DTI. The DTI-ALPS was computed as the ratio between the mean of x-axis diffusivity on the projection fibers (Dxproj) and association fibers (Dxassoc) and the mean of y-axis diffusivity on the projection fibers (Dyproj) and z-axis diffusivity on association fibers (Dzassoc). The DTI-ALPS index was calculated using the following formula: DTI-ALPS index = mean (Dxproj, Dxassoc)/mean (Dyproj, Dzassoc). Finally, we used the average DTI-ALPS index values from both hemispheres for further analysis.
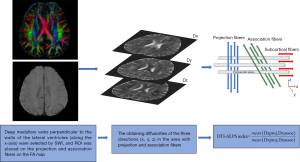
Evaluation of conventional MRI markers of small cerebral vessel disease
Conventional MRI markers of cerebral small vessel disease (SVD) include white-matter hyperintensities (WMHs), lacunes, microbleeds, and enlarged perivascular spaces (EPVSz). WMH is characterized as hypointensity or isointensity on T1-weighted imaging and hyperintensity on T2-weighted fluid-attenuated inversion recovery (FLAIR) imaging. The severity of WMH was graded according to the modified Fazekas scale as follows: 0, normal; 1, mild; 2, moderate; and 3, severe (25). Lacunae are defined as CSF signal holes with a diameter of 3–15 mm. Microbleeds were defined as round or oval hypointense lesions with a diameter of 2–10 mm on SWI sequences. EPVSz are defined as fluid-filled spaces with a diameter of <3 mm that exhibit signal intensity similar to that of CSF on all MRI sequences (26). The assessment of EPVS in the basal ganglia and centrum semiovale was estimated using a semiquantitative scale as follows (27): no EPVS, 0; 1–10 EPVSz, 1; 11–20 EPVSz, 2; 21–40 EPVSz, 4; and more than 40 EPVSz, 4. These conventional MRI markers of CSVD (WMH, lacunae, microbleeds, and EPVS) were measured using uAI discovery-CSVD software (United Imaging Intelligence, Shanghai, China) (28). When MRI markers of CSVD measurements were completed, the results were evaluated by a neuroradiologist. After evaluation, all automatic measurement results are approved without correction.
Whole-brain volume and intracranial volume (ICV) segmentation
We used the DPABISurf_V3.1 software (http://rfmri.org/DPABISurf) to calculate the whole-brain volume and ICV of the 3D T1-weighted images in all patients with T2DM and HCs (29). This software operates based on FreeSurfer software (version 7.3.2). FreeSurfer software provides complete data processing and automatic segmentation of the brain structure, as previously described (30). Subsequently, the results of the whole-brain volume, and the ICV segmentation of all patients with T2DM and HCs were quality-checked by a radiologist. All the automatic segmentations were confirmed to be accurate without the need for manual corrections.
Statistical analysis
Statistical analyses were performed using the SPSS software version 26.0 (IBM Corp., Armonk, NY, USA). Continuous variables and categorical variables are represented as the mean ± standard deviation and as frequency (%), respectively. Continuous variables of demographic and neuropsychological test results between the T2DM and the HCs were compared using the Student t-test or Mann-Whitney test, while categorical variables were analyzed using the Chi-squared test. The interclass correlation coefficient (ICC) was used to evaluate the interobserver agreement in the measurements of the DTI-ALPS index by two neuroradiologists. A general linear model was used to compare the DTI-ALPS index and global MRI parameters between the HC and T2DM groups after adjustments were made for age and sex. Additionally, to further adjust for the effects of the whole-brain volume and conventional MRI markers of CSVD, such as WMH, lacunae, EPVS, and microbleeds on the DTI-ALPS index, these factors were included as confounding variables in the general linear model to compare the DTI-ALPS index.
The multivariate linear regression model was used to analyze the relationship between the DTI-ALPS index or other MRI parameters and MoCA or MMSE score in the T2DM group. In this analysis, potential confounding variables such as age, sex, years of education, body mass index (BMI), triglycerides, total cholesterol, high-density lipoprotein (HDL), low-density lipoprotein (LDL), whole-brain volume, smoking, and hypertension status were adjusted to control for their effects. The PROCESS macro of SPSS was used for mediation analysis to characterize the role of FA in the association between glymphatic system damage and cognitive function. In each analysis, the Benjamini-Hochberg false discovery rate (BHFDR) correction method was used for multiple comparison corrections. A two-tailed PFDR<0.05 was considered statistically significant.
Results
Participant characteristics
This study enrolled 70 patients with T2DM (age 66.37±9.85 years; 48 males) and 35 HCs (age 64.03±11.26 years; 21 males), including 35 patients with T2DM-MCI and 35 patients with T2DM but not MCI (T2DM-nMCI). The demographic and clinical characteristics, whole-brain volume, ICV, and conventional CSVD markers are shown in Table 1. The glycated hemoglobin A1c (HbA1c), fasting glucose, and postprandial glucose levels in the T2DM group were significantly higher than those of the HCs, while the MoCA score and BMI were lower. There were no significant differences in HbA1c, fasting glucose, or postprandial glucose levels between the T2DM-MCI and the T2DM-nMCI groups. There were no significant differences in age, sex, years of education, smoking history, hypertension history, triglyceride levels, cholesterol levels, HDL, LDL, MMSE score, whole-brain volume, ICV, or conventional CSVD markers between the T2DM and HC groups.
Table 1
Variables | HC | T2DM | PFDR | T2DM-nMCI | T2DM-MCI | PFDR |
---|---|---|---|---|---|---|
Age (years) | 64.03±11.26 | 66.37±9.85 | 0.276† | 64.54±10.30 | 68.20±9.15 | 0.121† |
Male | 21 (60.00) | 48 (68.57) | 0.383‡ | 25 (71.48) | 23 (65.71) | 0.607‡ |
Education (years) | 11.49±2.68 | 11.74±2.37 | 0.502§ | 11.86±2.49 | 11.63±2.28 | 0.664§ |
BMI (kg/m2) | 24.93±2.22 | 23.92±2.44 | 0.044† | 23.93±2.62 | 23.91±2.29 | 0.967§ |
Smoking | 9 (25.71) | 15 (21.42) | 0.733‡ | 7 (20.00) | 8 (22.86) | 0.771‡ |
Hypertension | 17 (48.57) | 44 (62.85) | 0.162‡ | 23 (65.71) | 21 (60.00) | 0.621‡ |
Fasting glucose (mmol/L) | 5.17±0.42 | 8.47±2.26 | <0.001§ | 8.36±2.38 | 8.59±2.16 | 0.391§ |
Postprandial glucose (mmol/L) | 6.71±0.78 | 11.77±2.84 | <0.001§ | 11.09±2.08 | 12.44±3.34 | 0.104§ |
HbA1c (%) | 5.62±0.30 | 7.07±1.39 | <0.001§ | 6.99±1.29 | 7.16±1.49 | 0.445§ |
Triglyceride (mmol/L) | 1.41±0.87 | 1.60±1.00 | 0.265§ | 1.45±0.69 | 1.76±1.23 | 0.860§ |
Total cholesterol (mmol/L) | 4.30±0.98 | 4.13±1.17 | 0.251§ | 4.03±1.15 | 4.24±1.19 | 0.561§ |
HDL (mmol/L) | 1.24±0.37 | 1.15±0.31 | 0.239§ | 1.19±0.34 | 1.12±0.28 | 0.481§ |
LDL (mmol/L) | 2.30±0.76 | 2.25±0.87 | 0.463§ | 2.20±0.81 | 2.29±0.95 | 0.733§ |
MoCA score | 27.80±1.18 | 24.59±3.14 | <0.001§ | 27.34±1.11 | 21.83±1.76 | <0.001§ |
MMSE score | 28.69±0.96 | 28.11±1.57 | 0.121§ | 28.63±1.00 | 27.60±1.85 | 0.020§ |
Fazekas score | 1.63±0.97 | 1.64±0.80 | 0.795§ | 1.71±0.75 | 1.57±0.85 | 0.280§ |
Basal ganglia EPVS | 1.80±0.96 | 1.87±0.93 | 0.636§ | 1.86±1.00 | 1.89±0.87 | 0.707§ |
Centrum semiovale EPVS | 1.74±0.85 | 1.99±0.99 | 0.269§ | 1.94±0.97 | 2.02±1.04 | 0.784§ |
Lacunae | 9 (25.71) | 30 (42.86) | 0.087‡ | 14 (40.00) | 16 (45.71) | 0.629‡ |
Microbleeds | 7 (20.00) | 22 (31.43) | 0.217‡ | 10 (28.57) | 12 (34.29) | 0.607‡ |
Whole brain volume (mL) | 1,060.25±118.85 | 1,043.05±106.56 | 0.455† | 1,060.74±81.06 | 1,025.36±125.81 | 0.167† |
ICV (mL) | 1,396.56±138.21 | 1,432.21±128.74 | 0.195† | 1,435.01±112.86 | 1,429.42±144.50 | 0.857† |
Values are reported as the mean ± standard deviation for quantitative variables and as the frequency (percentage) for categorical variables. †, the P value was obtained using the two-sample t-test; ‡, the P value was obtained using the χ2 test; §, the P value was obtained using the Mann-Whitney test. MRI, magnetic resonance imaging; T2DM, type 2 diabetes mellitus; HC, healthy control; FDR, false discovery rate; T2DM-nMCI, T2DM without mild cognitive impairment; T2DM-MCI, patients with T2DM with mild cognitive impairment; BMI, body mass index; HbA1c, hemoglobin A1c; HDL, high-density lipoprotein; LDL, low-density lipoprotein; MoCA, Montreal Cognitive Assessment; MMSE, Mini-Mental State Examination; EPVS, enlarged perivascular space; ICV, intracranial volume.
DTI-ALPS index and global MRI parameters in patients with T2DM and HCs
The DTI-ALPS index demonstrated excellent interobserver agreement, with an ICC of 0.918 [95% confidence interval (CI): 0.864–0.948; P<0.001]. Table 2 shows the comparison of the DTI-ALPS index and the global MRI parameters between the T2DM and the HC after adjustments were made for age and sex. The DTI-ALPS index of patients with T2DM was significantly lower than that of HCs (1.2858 vs. 1.5276; PFDR<0.001). Additionally, the DTI-ALPS index of patients with T2DM-MCI was significantly lower than that of patients with T2DM-nMCI (1.2428 vs. 1.3287; PFDR=0.008) (Figure 2). Additionally, to further adjust for the effects of the whole brain volume and conventional MRI markers of CSVD, such as WMH, lacunae, EPVS, and microbleeds on the DTI-ALPS index, these factors were included as confounding variables in the general linear model to compare the DTI-ALPS index. The results showed that the DTI-ALPS index of patients with T2DM was lower than that of HCs (P<0.001), while the DTI-ALPS index of patients with T2DM-MCI was lower than that of patients with T2DM-nMCI (P=0.003).
Table 2
HC | T2DM | PFDR | T2DM-nMCI | T2DM-MCI | PFDR | |
---|---|---|---|---|---|---|
DTI-ALPS index | 1.5276±0.0549 | 1.2858±0.1156 | <0.001 | 1.3287±0.0878† | 1.2428±0.1250†,‡ | 0.008 |
Cp | 0.0135±0.0034 | 0.0123±0.0026 | 0.115 | 0.01308±0.0031 | 0.0115±0.0018† | 0.086 |
Lp | 43.2964±11.2263 | 55.4947±22.8403 | 0.030 | 52.2859±21.1964 | 58.7035±24.2532† | 0.492 |
Eglob | 0.0507±0.0076 | 0.0450±0.0083 | 0.001 | 0.0460±0.0073† | 0.0441±0.0092† | 0.670 |
Sigma | 0.0029±0.0011 | 0.0027±0.0010 | 0.785 | 0.0029±0.0010 | 0.0026±0.0010 | 0.698 |
FA | 0.4570±0.0593 | 0.4290±0.0538 | <0.001 | 0.4494±0.0511† | 0.4085±0.0491†,‡ | <0.001 |
MD | 0.8753±0.0716 | 0.9024±0.0590 | <0.001 | 0.8969±0.0607† | 0.9078±0.0577†,‡ | 0.011 |
AD | 1.2620±0.0633 | 1.2920±0.0560 | <0.001 | 1.2816±0.0610† | 1.3023±0.0491†,‡ | 0.008 |
RD | 0.6819±0.0785 | 0.7076±0.0640 | <0.001 | 0.7046±0.0633† | 0.7106±0.0655†,‡ | 0.018 |
Values are reported as the mean ± standard deviation for quantitative variables. MD, AD, and RD are expressed in units of ×10−3 mm2/s. †, PFDR<0.05 compared with HCs; ‡, PFDR<0.05 compared with patients with T2DM-nMCI. MRI, magnetic resonance imaging; T2DM, type 2 diabetes mellitus; HC, healthy control; FDR, false discovery rate; T2DM-nMCI, T2DM without mild cognitive impairment; T2DM-MCI, patients with T2DM with mild cognitive impairment; DTI-ALPS, diffusion tensor imaging analysis along the perivascular space; Cp, clustering coefficient; Lp, characteristic path length; Eglob, global efficiency; Sigma, small-worldness index; FA, fractional anisotropy; MD, mean diffusivity; AD, axial diffusivity; RD, radial diffusivity.
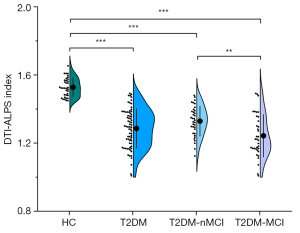
The Lp of patients with T2DM was higher than that of HCs (55.4947 vs. 43.2964; PFDR=0.030), while the Eglob of patients withT2DM was lower than that of HCs (0.0450 vs. 0.0507; PFDR=0.001). There were no significant differences in the Cp or Sigma between the T2DM and HC groups. However, the Cp of T2DM-MCI group was lower than that of HCs (0.0115 vs. 0.0135; PFDR=0.026). No significant differences were found in the Cp, Lp, Eglob, or Sigma values between the T2DM-MCI and T2DM-nMCI groups.
Compared to HCs, patients with T2DM had higher whole-brain MD (0.9024 vs. 0.8753; PFDR<0.001), AD (1.2920 vs. 1.2620; PFDR<0.001), and RD (0.7076 vs. 0.6819; PFDR<0.001) values but lower whole-brain FA values (0.4290 vs. 0.4570, PFDR<0.001). The whole-brain MD (0.9078 vs. 0.8969; PFDR=0.011), AD (1.3023 vs. 1.2816; PFDR=0.008), and RD (0.7106 vs. 0.7046; PFDR=0.018) values of the patients with T2DM-MCI were all higher than those of patients with T2DM-nMCI, while the whole-brain FA value was lower (0.4085 vs. 0.4494; PFDR<0.001).
Analysis of associations
After adjustments were made for age, sex, years of education, BMI, whole-brain volume, triglycerides, total cholesterol, HDL, LDL, smoking, and hypertension status, a lower MoCA score was associated with a lower DTI-ALPS index (standardized β =0.577; 95% CI: 0.360 to 0.793; PFDR<0.001) and a lower FA (standardized β =0.448; 95% CI: 0.171 to 0.725; PFDR=0.009) in patients with T2DM (Table 3, Figure 3). However, no significant associations were observed between MMSE score and MRI parameters in patients with T2DM (PFDR>0.05). In patients with T2DM, a lower DTI-ALPS index was associated with a lower FA (standardized β =0.340; 95% CI: 0.069 to 0.611; PFDR=0.043) and higher MD values (standardized β =−0.338; 95% CI: −0.610 to −0.065; PFDR=0.043), AD values (standardized β =−0.440; 95% CI: −0.712 to −0.168; PFDR=0.016), and RD values (standardized β =−0.313; 95% CI: −0.581 to −0.044; PFDR=0.046) (Table 4 and Figure 3). No significant associations were observed between the DTI-ALPS index and other MRI parameters in the HC groups (Table 4).
Table 3
MoCA | MMSE | ||||||
---|---|---|---|---|---|---|---|
β | 95% CI | PFDR | β | 95% CI | PFDR | ||
DTI-ALPS index | 0.577 | 0.360, 0.793 | <0.001 | 0.057 | −0.213, 0.326 | 0.675 | |
Cp | 0.176 | −0.085, 0.438 | 0.328 | 0.011 | −0.260, 0.282 | 0.937 | |
Lp | −0.062 | −0.323, 0.198 | 0.635 | −0.097 | −0.362, 0.168 | 0.466 | |
Eglob | 0.108 | −0.156, 0.373 | 0.580 | 0.122 | −0.147, 0.391 | 0.368 | |
Sigma | 0.171 | −0.075, 0.418 | 0.328 | 0.001 | −0.255, 0.256 | 0.988 | |
FA | 0.448 | 0.171, 0.725 | 0.009 | −0.065 | −0.372, 0.242 | 0.672 | |
MD | −0.112 | −0.406, 0.183 | 0.580 | 0.041 | −0.260, 0.343 | 0.784 | |
AD | −0.209 | −0.505, 0.087 | 0.328 | 0.031 | −0.276, 0.338 | 0.841 | |
RD | −0.084 | −0.374, 0.207 | 0.625 | 0.097 | −0.199, 0.393 | 0.657 |
MoCA, Montreal Cognitive Assessment; MMSE, Mini-Mental State Examination; MRI, magnetic resonance imaging; T2DM, type 2 diabetes mellitus; CI, confidence interval; FDR, false discovery rate; DTI-ALPS, diffusion tensor imaging analysis along the perivascular space; Cp, clustering coefficient; Lp, characteristic path length; Eglob, global Efficiency; Sigma, small-worldness index; FA, fractional anisotropy; MD, mean diffusivity; AD, axial diffusivity; RD, radial diffusivity.
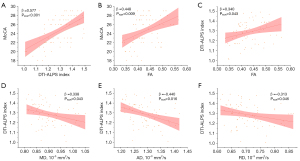
Table 4
HC | T2DM | ||||||
---|---|---|---|---|---|---|---|
β | 95% CI | PFDR | β | 95% CI | PFDR | ||
Cp | 0.057 | −0.363, 0.476 | 0.783 | 0.065 | −0.197, 0.327 | 0.622 | |
Lp | −0.070 | −0.512, 0.373 | 0.748 | 0.041 | −0.217, 0.300 | 0.750 | |
Eglob | 0.162 | −0.371, 0.694 | 0.536 | 0.053 | −0.209, 0.315 | 0.686 | |
Sigma | −0.103 | −0.567, 0.359 | 0.647 | 0.144 | −0.102, 0.384 | 0.249 | |
FA | −0.104 | −0.542, 0.334 | 0.627 | 0.340 | 0.069, 0.611 | 0.043 | |
MD | −0.169 | −0.763, 0.437 | 0.579 | −0.338 | −0.610, −0.065 | 0.043 | |
AD | −0.179 | −0.780, 0.423 | 0.544 | −0.440 | −0.712, −0.168 | 0.016 | |
RD | −0.148 | −0.743, 0.446 | 0.610 | −0.313 | −0.581, −0.044 | 0.046 |
DTI-ALPS, diffusion tensor imaging analysis along the perivascular space; MRI, magnetic resonance imaging; HC, healthy control; T2DM, type 2 diabetes mellitus; CI, confidence interval; FDR, false discovery rate; Cp, clustering coefficient; Lp, characteristic path length; Eglob, global efficiency; Sigma, small-worldness index; FA, fractional anisotropy; MD, mean diffusivity; AD, axial diffusivity; RD, radial diffusivity.
Mediation analysis
To further clarify the relationship between the DTI-ALPS index, whole-brain FA, and cognition, we conducted a mediation analysis with the DTI-ALPS index as the independent variable (X) and MoCA scores as the dependent variable (Y). After adjustments for age and sex, the mediation analysis between the DTI-ALPS index and MoCA revealed that FA partially mediated the effect of the DTI-ALPS index on cognitive impairment in patients with T2DM (standardized β coefficient =0.086; mediation effect 14.86%) (Figure 4).

Discussion
In this study, we aimed to evaluate the glymphatic system function using the DTI-ALPS method and to clarify the relationship among the DTI-ALPS index, whole-brain white-matter damage, and cognitive impairment in patients with T2DM. We found that the DTI-ALPS index was decreased in patients with T2DM, particularly in those with T2DM-MCI. Moreover, cognitive impairment was associated with decreased DTI-ALPS index and white-matter damage in patients with T2DM; meanwhile, the DTI-ALPS index was associated with white-matter damage and cognitive impairment in patients with T2DM.
The glymphatic system is a highly organized pathway for fluid transport (31), facilitating the distribution of glucose, growth factors, and neurotrophic factors in the brain (32) and serving as a key route for the clearance of metabolic waste from the brain (6). One animal study reported that the rate of clearance of gadolinium-diethylenetriamine-penta-acetic acid from the interstitial space was three times slower in T2DM rats, suggesting that the glymphatic system is impaired (18). Although intrathecal injection of contrast agents is considered the gold standard for assessing glymphatic function, it is invasive and can cause gadolinium deposition in the brain (33). Therefore, we chose the noninvasive DTI-ALPS method to evaluate the glymphatic system function in patients with T2DM. Taoka et al. (9) proposed that calculating the DTI-ALPS index could serve as a noninvasive method for assessing glymphatic function. This DTI-ALPS method provides a nearly independent analysis of the water diffusivity along the perivascular space by taking advantage of the perpendicular conditions of veins (x-axis), association fibers (y-axis), and projection fibers (z-axis) at the level of the lateral ventricle body and thus reflecting glymphatic system activity (9). MRI studies using intrathecal gadolinium-based contrast agent have demonstrated a robust correlation between the DTI-ALPS index and glymphatic clearance rates (34).
We found that the DTI-ALPS index has good interobserver agreement, indicating that the DTI-ALPS index calculated by manually defined ROIs can provide good reliability and repeatability. Previous studies have found that the DTI-ALPS value calculated by manually placed ROIs has good reliability and repeatability (12,13). Our findings indicate that the DTI-ALPS index of patients with T2DM-MCI or T2DM-nMCI is significantly lower than that of HCs, suggesting the glymphatic system function may be reduced in patients with T2DM. Yang et al. (17) used the DTI-ALPS method to assess glymphatic function in patients with T2DM and found that the DTI-ALPS index of patients with T2DM was lower than that of HC. In a recently published study, they found that patients with T2DM had a significantly lower DTI-ALPS index and that the DTI-ALPS index was correlated with MoCA (16). It is worth noting that their study did not stratify patients with T2DM based on cognitive levels, whereas our study specifically extended this exploration to those withT2DM-MCI and further investigated the association between glymphatic function, cognitive function, and microstructural integrity of white matter. Our study found that the DTI-ALPS index in patients with T2DM-MCI was lower than that of T2DM-nMCI, indicating that the glymphatic system activity was more severely decreased in patients with T2DM-MCI. In addition, a lower DTI-ALPS index was associated with lower MoCA scores, suggesting that glymphatic system activity may be related to cognitive function in patients with T2DM. There was no significant association between DTI-ALPS index and MMSE score in patients with T2DM. This may be related to the fact that the broader MCI score range on the MoCA could be useful for earlier detection when cognitive deficits are more subtle, making it significantly better than MMSE in identifying patients with MCI (35).
T2DM is recognized as a major risk factor for cognitive dysfunction (3). Insulin resistance is a major pathogenic mechanism in T2DM and is often associated with dysfunction in the phosphoinositide 3 kinase/protein kinase B (PI3K/Akt) insulin signaling pathway, leading to reduced Aβ clearance and resulting in cognitive impairment (36). The glymphatic system plays an important role in removing metabolic waste and solutes from the brain parenchyma, including Aβ protein, which has been implicated in the pathogenesis of cognitive impairment (4). Therefore, it is reasonable to speculate that the DTI-ALPS index is lower in patients with T2DM and that the decrease in DTI-ALPS index is more significant in patients with T2DM-MCI.
The human brain is composed of multiple functionally diverse and interconnected brain regions, forming a complex network. Network analysis is an effective method for studying the neurobiological mechanisms of diseases (37). Previous studies had also found changes in the brain’s structural network in patients with T2DM, but the results have been inconsistent. Our study found that Cp and Eglob values were decreased, while Lp was increased in patients with T2DM-MCI. However, patients with T2DM-nMCI only showed a decrease in Eglob. We found no differences in the Cp, Lp, Eglob, or Sigma values between the T2DM-MCI and T2DM-nMCI groups. This suggest that global structural connectivity in patients with T2DM may be associated with cognitive impairment and a variety of other factors. It is also possible that the measurements of global structural connectivity are not sufficiently sensitive to detect subtle changes in patients with T2DM (38). Furthermore, we performed a correlation analysis to examine the relationship between the DTI-ALPS index and global structural connectivity parameters in patients with T2DM. We did not find any correlation between them, which may be related to the insensitivity of the global structural connectivity parameter measurements.
DTI can be used to reflect microstructural changes in the white matter of the brain. FA and MD can reflect the integrity of white-matter fiber bundles. The increase in AD is associated with axonal degeneration, while the increase in RD is associated with demyelination (39). It has been suggested that damage to the white-matter structure of the brain is associated with cognitive impairment in patients with T2DM (40). In our study, we found that patients with T2DM had increased MD, RD, and AD, as well as decreased FA, indicating whole-brain white-matter structural damage in those with T2DM. Furthermore, we observed a positive correlation between FA and MoCA score, suggesting that patients with T2DM have white-matter damage, which is in turn associated with cognitive impairment in T2DM. In a recent study on patients with multiple sclerosis and cognitive impairment, it was found that the DTI-ALPS index was positively correlated with FA and negatively correlated with MD (41). Furthermore, Lee et al. (42) reported that the DTI-ALPS index was positively correlated with whole-brain FA and negatively correlated with MD, AD, and RD in patients with newly diagnosed focal epilepsy, suggesting that glymphatic system dysfunction is associated with white-matter damage. Our study found the DTI-ALPS index was positively correlated with whole-brain FA and negatively correlated with MD and AD, which indicates that glymphatic system dysfunction is related to white-matter damage in patients with T2DM. To further characterize the complex relationships between the glymphatic system, white-matter damage, and cognitive function, we conducted a mediation analysis. Our results showed that FA played a partial mediating role, accounting for 14.86% of the total effect, indicating that glymphatic dysfunction may lead to white-matter damage, thereby affecting cognitive function in patients with T2DM. Our study supports a potential relationship between the DTI-ALPS index, white-matter damage, and cognitive function in patients with T2DM, which may provide a new direction for studying the underlying pathogenesis of T2DM-MCI patients.
The DTI-ALPS method has become a popular technique for indirectly evaluating the dynamics of ISF by differentiating water diffusivity in white matter along PVSs and in axonal fibers perpendicular to PVSs, but the extent to which DTI-ALPS actually reflects glymphatic function remains a subject of active debate (43). The DTI-ALPS index may not distinguish perivascular water diffusivity from other sources of directional water motion, such as diffusion along fiber tracts, which are included in the ROI. Although many studies have reported an association between the DTI-ALPS index and glymphatic function in neurological diseases, the association is not equivalent with causality. It is possible that the DTI-ALPS index merely reflects features of white matter (44). Taoka et al. (45) speculated that since the brain’s system for clearing waste can function in various regions, the DTI-ALPS method, which is limited to assessing specific areas, might not accurately reflect the overall functionality of the brain’s glymphatic system. The relationship between DTI-ALPS index and the glymphatic function should be interpreted with caution. A recent study indicates that the decrease in DTI-ALPS index may be related to the perivascular space diffusivity and the intrinsic structure of the white-matter tracts, such as changes in the radial asymmetry of white matter; therefore, we should be wary of attributing the change in the ALPS index solely to the change in the perivascular space diffusion rate or glymphatic function (46). We observed that the ALPS index was decreased in patients with T2DM, which may also reflect changes in the intrinsic structure of the brain’s white-matter fiber bundles. Therefore, we did not use a lower DTI-ALPS index to directly reflect the glymphatic dysfunction. In summary, further research is needed to clarify the correlation between the DTI-ALPS index and the function of the glymphatic system.
Our study involved several limitations which should be addressed. First, although our research provides valuable insights into the relationship between glymphatic system dysfunction, brain white-matter damage, and cognitive impairment in T2DM, the presence of a correlation does not imply causation. Second, we only used the MoCA and MMSE scales to assess cognition and did not conduct a detailed assessment of multiple domains of cognition, which may not reflect the full cognition of the participants and may require more extensive neuropsychological assessment in future research. Additionally, our sample size was relatively small. In the future, multicenter studies with larger sample sizes can be conducted to further validate the validity and accuracy of the results of this study. Finally, the DTI-ALPS index has certain limitations as a marker for assessing brain clearance function (44), and future studies could combine other biomarkers and multiple imaging techniques to investigate changes in glymphatic system function in patients with T2DM.
Conclusions
The DTI-ALPS index was decreased in patients with T2DM, especially in those with T2DM-MCI. The reduction in DTI-ALPS index was associated with cognitive impairment and whole-brain white-matter damage in patients with T2DM. Our findings may provide a better understanding of the mechanisms underlying cognitive impairment in patients with T2DM.
Acknowledgments
None.
Footnote
Reporting Checklist: The authors have completed the STROBE reporting checklist. Available at https://qims.amegroups.com/article/view/10.21037/qims-24-1591/rc
Funding: This work was supported by
Conflicts of Interest: All authors have completed the ICMJE uniform disclosure form (available at https://qims.amegroups.com/article/view/10.21037/qims-24-1591/coif). J.D. is an employee of GE HealthCare and has a collaborative relationship with this study. The other authors have no other conflicts of interest to declare.
Ethical Statement: The authors are accountable for all aspects of the work in ensuring that questions related to the accuracy or integrity of any part of the work are appropriately investigated and resolved. This study was conducted in accordance with the Declaration of Helsinki (as revised in 2013) and was approved by the Ethics Committee of The Second Affiliated Hospital of Nanchang University (No. O-2024-73). All participants signed written informed consent.
Open Access Statement: This is an Open Access article distributed in accordance with the Creative Commons Attribution-NonCommercial-NoDerivs 4.0 International License (CC BY-NC-ND 4.0), which permits the non-commercial replication and distribution of the article with the strict proviso that no changes or edits are made and the original work is properly cited (including links to both the formal publication through the relevant DOI and the license). See: https://creativecommons.org/licenses/by-nc-nd/4.0/.
References
- Zaccardi F, Webb DR, Yates T, Davies MJ. Pathophysiology of type 1 and type 2 diabetes mellitus: a 90-year perspective. Postgrad Med J 2016;92:63-9. [Crossref] [PubMed]
- McCrimmon RJ, Ryan CM, Frier BM. Diabetes and cognitive dysfunction. Lancet 2012;379:2291-9. [Crossref] [PubMed]
- Biessels GJ, Whitmer RA. Cognitive dysfunction in diabetes: how to implement emerging guidelines. Diabetologia 2020;63:3-9. [Crossref] [PubMed]
- Iliff JJ, Wang M, Liao Y, Plogg BA, Peng W, Gundersen GA, Benveniste H, Vates GE, Deane R, Goldman SA, Nagelhus EA, Nedergaard M. A paravascular pathway facilitates CSF flow through the brain parenchyma and the clearance of interstitial solutes, including amyloid β. Sci Transl Med 2012;4:147ra111. [Crossref] [PubMed]
- Mestre H, Hablitz LM, Xavier AL, Feng W, Zou W, Pu T. etal. Aquaporin-4-dependent glymphatic solute transport in the rodent brain. Elife 2018;7:e40070. [Crossref] [PubMed]
- Hablitz LM, Nedergaard M. The glymphatic system. Curr Biol 2021;31:R1371-5. [Crossref] [PubMed]
- Mestre H, Mori Y, Nedergaard M. The Brain's Glymphatic System: Current Controversies. Trends Neurosci 2020;43:458-66. [Crossref] [PubMed]
- Ward R, Li W, Abdul Y, Jackson L, Dong G, Jamil S, Filosa J, Fagan SC, Ergul A. NLRP3 inflammasome inhibition with MCC950 improves diabetes-mediated cognitive impairment and vasoneuronal remodeling after ischemia. Pharmacol Res 2019;142:237-50. [Crossref] [PubMed]
- Taoka T, Masutani Y, Kawai H, Nakane T, Matsuoka K, Yasuno F, Kishimoto T, Naganawa S. Evaluation of glymphatic system activity with the diffusion MR technique: diffusion tensor image analysis along the perivascular space (DTI-ALPS) in Alzheimer's disease cases. Jpn J Radiol 2017;35:172-8. [Crossref] [PubMed]
- Zhang X, Wang Y, Jiao B, Wang Z, Shi J, Zhang Y, Bai X, Li Z, Li S, Bai R, Sui B. Glymphatic system impairment in Alzheimer's disease: associations with perivascular space volume and cognitive function. Eur Radiol 2024;34:1314-23. [Crossref] [PubMed]
- Shen T, Yue Y, Ba F, He T, Tang X, Hu X, Pu J, Huang C, Lv W, Zhang B, Lai HY. Diffusion along perivascular spaces as marker for impairment of glymphatic system in Parkinson's disease. NPJ Parkinsons Dis 2022;8:174. [Crossref] [PubMed]
- Carotenuto A, Cacciaguerra L, Pagani E, Preziosa P, Filippi M, Rocca MA. Glymphatic system impairment in multiple sclerosis: relation with brain damage and disability. Brain 2022;145:2785-95. [Crossref] [PubMed]
- Pang H, Wang J, Yu Z, Yu H, Li X, Bu S, Zhao M, Jiang Y, Liu Y, Fan G. Glymphatic function from diffusion-tensor MRI to predict conversion from mild cognitive impairment to dementia in Parkinson's disease. J Neurol 2024;271:5598-609. [Crossref] [PubMed]
- Jin Y, Zhang W, Yu M, Li J, Du Y, Wang W, Chen G, Ding X, Ding J. Glymphatic system dysfunction in middle-aged and elderly chronic insomnia patients with cognitive impairment evidenced by diffusion tensor imaging along the perivascular space (DTI-ALPS). Sleep Med 2024;115:145-51. [Crossref] [PubMed]
- Xu K, Zhang J, Xing C, Xu X, Yin X, Wu Y, Chen X, Chen YC. Evaluation of glymphatic system activity by diffusion tensor image analysis along the perivascular space in presbycusis. CNS Neurosci Ther 2024;30:e14458. [Crossref] [PubMed]
- Yu S, Jiang H, Yu L, Liu T, Yang C, Cao J, Song Q, Miao Y, Wang W. DTI-ALPS index decreased in patients with Type 2 Diabetes Mellitus. Front Neurosci 2024;18:1383780. [Crossref] [PubMed]
- Yang G, Deng N, Liu Y, Gu Y, Yao X. Evaluation of Glymphatic System Using Diffusion MR Technique in T2DM Cases. Front Hum Neurosci 2020;14:300. [Crossref] [PubMed]
- Jiang Q, Zhang L, Ding G, Davoodi-Bojd E, Li Q, Li L, Sadry N, Nedergaard M, Chopp M, Zhang Z. Impairment of the glymphatic system after diabetes. J Cereb Blood Flow Metab 2017;37:1326-37. [Crossref] [PubMed]
- Luis CA, Loewenstein DA, Acevedo A, Barker WW, Duara R. Mild cognitive impairment: directions for future research. Neurology 2003;61:438-44. [Crossref] [PubMed]
- Biessels GJ, Deary IJ, Ryan CM. Cognition and diabetes: a lifespan perspective. Lancet Neurol 2008;7:184-90. [Crossref] [PubMed]
- Mayfield J. Diagnosis and classification of diabetes mellitus: new criteria. Am Fam Physician 1998;58:1355-62, 1369-70.
- Glasser MF, Smith SM, Marcus DS, Andersson JL, Auerbach EJ, Behrens TE, Coalson TS, Harms MP, Jenkinson M, Moeller S, Robinson EC, Sotiropoulos SN, Xu J, Yacoub E, Ugurbil K, Van Essen DC. The Human Connectome Project's neuroimaging approach. Nat Neurosci 2016;19:1175-87. [Crossref] [PubMed]
- Hu P, Yuan Y, Zou Y, Xiong R, Dai J, Zhao X, Xie L, Tang X. Alterations in the DTI-ALPS index and choroid plexus volume are associated with clinical symptoms in participants with narcolepsy type 1. Sleep Med 2024;124:471-8. [Crossref] [PubMed]
- Taoka T, Naganawa S. Glymphatic imaging using MRI. J Magn Reson Imaging 2020;51:11-24. [Crossref] [PubMed]
- Fazekas F, Chawluk JB, Alavi A, Hurtig HI, Zimmerman RA. MR signal abnormalities at 1.5 T in Alzheimer's dementia and normal aging. AJR Am J Roentgenol 1987;149:351-6. [Crossref] [PubMed]
- Wu L, Wang Z, Zhou X, Kong Q, Zhang Y, Xu S, Huang H, Luo X. Mismatch of MRI White Matter Hyperintensities and Gait Function in Patients With Cerebral Small Vessel Disease. J Magn Reson Imaging 2024;60:550-8. [Crossref] [PubMed]
- Doubal FN, MacLullich AM, Ferguson KJ, Dennis MS, Wardlaw JM. Enlarged perivascular spaces on MRI are a feature of cerebral small vessel disease. Stroke 2010;41:450-4. [Crossref] [PubMed]
- Wu J, Xia Y, Wang X, Wei Y, Liu A, Innanje A, Zheng M, Chen L, Shi J, Wang L, Zhan Y, Zhou XS, Xue Z, Shi F, Shen D. uRP: An integrated research platform for one-stop analysis of medical images. Front Radiol 2023;3:1153784. [Crossref] [PubMed]
- Yan CG, Wang XD, Lu B. DPABISurf: data processing & analysis for brain imaging on surface. Sci Bull (Beijing) 2021;66:2453-5. [Crossref] [PubMed]
- Fischl B. FreeSurfer. Neuroimage 2012;62:774-81. [Crossref] [PubMed]
- Rasmussen MK, Mestre H, Nedergaard M. The glymphatic pathway in neurological disorders. Lancet Neurol 2018;17:1016-24. [Crossref] [PubMed]
- Yan T, Qiu Y, Yu X, Yang L. Glymphatic Dysfunction: A Bridge Between Sleep Disturbance and Mood Disorders. Front Psychiatry 2021;12:658340. [Crossref] [PubMed]
- Nguyen NC, Molnar TT, Cummin LG, Kanal E. Dentate Nucleus Signal Intensity Increases Following Repeated Gadobenate Dimeglumine Administrations: A Retrospective Analysis. Radiology 2020;296:122-30. [Crossref] [PubMed]
- Zhang W, Zhou Y, Wang J, Gong X, Chen Z, Zhang X, Cai J, Chen S, Fang L, Sun J, Lou M. Glymphatic clearance function in patients with cerebral small vessel disease. Neuroimage 2021;238:118257. [Crossref] [PubMed]
- Trzepacz PT, Hochstetler H, Wang S, Walker B, Saykin AJAlzheimer’s Disease Neuroimaging Initiative. Relationship between the Montreal Cognitive Assessment and Mini-mental State Examination for assessment of mild cognitive impairment in older adults. BMC Geriatr 2015;15:107. [Crossref] [PubMed]
- Yamamoto N, Ishikuro R, Tanida M, Suzuki K, Ikeda-Matsuo Y, Sobue K. Insulin-signaling Pathway Regulates the Degradation of Amyloid β-protein via Astrocytes. Neuroscience 2018;385:227-36. [Crossref] [PubMed]
- Sporns O, Tononi G, Kötter R. The human connectome: A structural description of the human brain. PLoS Comput Biol 2005;1:e42. [Crossref] [PubMed]
- Zhou B, Wang X, Yang Q, Wu F, Tang L, Wang J, Li C. Topological Alterations of the Brain Functional Network in Type 2 Diabetes Mellitus Patients With and Without Mild Cognitive Impairment. Front Aging Neurosci 2022;14:834319. [Crossref] [PubMed]
- Gao J, Pan P, Li J, Tang M, Yan X, Zhang X, Wang M, Ai K, Lei X, Zhang X, Zhang D. Analysis of white matter tract integrity using diffusion kurtosis imaging reveals the correlation of white matter microstructural abnormalities with cognitive impairment in type 2 diabetes mellitus. Front Endocrinol (Lausanne) 2024;15:1327339. [Crossref] [PubMed]
- Zhang J, Wang Y, Wang J, Zhou X, Shu N, Wang Y, Zhang Z. White matter integrity disruptions associated with cognitive impairments in type 2 diabetic patients. Diabetes 2014;63:3596-605. [Crossref] [PubMed]
- Margoni M, Pagani E, Meani A, Preziosa P, Mistri D, Gueye M, Moiola L, Filippi M, Rocca MA. Cognitive Impairment Is Related to Glymphatic System Dysfunction in Pediatric Multiple Sclerosis. Ann Neurol 2024;95:1080-92. [Crossref] [PubMed]
- Lee DA, Park BS, Ko J, Park SH, Lee YJ, Kim IH, Park JH, Park KM. Glymphatic system dysfunction in temporal lobe epilepsy patients with hippocampal sclerosis. Epilepsia Open 2022;7:306-14. [Crossref] [PubMed]
- Agarwal N, Lewis LD, Hirschler L, Rivera LR, Naganawa S, Levendovszky SR, et al. Current Understanding of the Anatomy, Physiology, and Magnetic Resonance Imaging of Neurofluids: Update From the 2022 "ISMRM Imaging Neurofluids Study group" Workshop in Rome. J Magn Reson Imaging 2024;59:431-49. [Crossref] [PubMed]
- Ringstad G. Glymphatic imaging: a critical look at the DTI-ALPS index. Neuroradiology 2024;66:157-60. [Crossref] [PubMed]
- Taoka T, Ito R, Nakamichi R, Nakane T, Kawai H, Naganawa S. Diffusion Tensor Image Analysis ALong the Perivascular Space (DTI-ALPS): Revisiting the Meaning and Significance of the Method. Magn Reson Med Sci 2024;23:268-90. [Crossref] [PubMed]
- Wright AM, Wu YC, Chen NK, Wen Q. Exploring Radial Asymmetry in MR Diffusion Tensor Imaging and Its Impact on the Interpretation of Glymphatic Mechanisms. J Magn Reson Imaging 2024;60:1432-41. [Crossref] [PubMed]