Diagnostic value of deep learning reconstruction-based subtraction CT-FFR in patients with calcified-related stenosis or stent implantation
Introduction
Fractional flow reserve (FFR) is a well-established standard for identifying hemodynamically significant stenosis that causes ischemia. The application of FFR is limited by the invasive procedure and high cost. With the great technological improvements of coronary computed tomography angiography (CTA), coronary CTA-derived fractional flow reserve (CT-FFR) allows for the noninvasive interpretation of anatomical stenosis and the hemodynamic severity of coronary lesions (1). Clinical trials have shown a high correlation between CT-FFR and invasive FFR (2-5). However, calcium-related blooming artifacts may affect lumen segmentation in the process of CT-FFR computation, and the diagnostic performance of CT-FFR would decrease due to severe coronary calcium burden (6). In addition, the measurement of FFR after coronary stenting may have an important role in clinical outcome follow-up (7), but vessels with coronary stents are not suitable for CT-FFR analysis (8).
Subtraction coronary CTA has shown the potential to reduce blooming artifacts caused by severe calcification or stents, which would improve the diagnostic performance in anatomical stenosis (9-11). Moreover, deep learning is widely used in biomedical studies (12-15). The image quality can be improved by deep learning reconstruction (DLR) with reduced image noise and sharper coronary artery edges. The DLR algorithm (Advanced Intelligent Clear-IQ Engine, AiCE, Canon Medical Systems) utilized in this study was developed by integrating convolutional neural networks trained on high-quality target images generated through model-based iterative reconstruction. This training process involved iteratively executing input-forward and error-backpropagation operations, utilizing millions of image patches to achieve optimal results. As a result, the DLR algorithm harnesses the advantages of model-based iterative reconstruction, transforming low-quality input data into high-quality images that are devoid of noise contamination. Therefore, when subtraction coronary CTA is combined with DLR, the diagnostic performance could be further enhanced in terms of visualization of the coronary lumen (16,17). However, the application of subtraction CT-FFR combined with DLR has not been fully investigated.
Therefore, we conducted this study to investigate the diagnostic value of DLR-based subtraction CT-FFR in detecting calcified-related hemodynamically significant stenosis and the feasibility of DLR-based subtraction CT-FFR in the application of coronary stents. We present this article in accordance with the STARD reporting checklist (available at https://qims.amegroups.com/article/view/10.21037/qims-24-1366/rc).
Methods
Patient population
Between March 2020 and January 2022, consecutive patients with known or suspected coronary artery disease (CAD) who were scheduled for invasive coronary angiography (ICA) and agreed to subtraction coronary CTA were eligible for study participation. Those who had a history of coronary bypass graft surgery, impaired renal function, or were unable to sustain a breath hold were excluded from coronary CTA. Patients without moderate to severe coronary calcification (Agatston score ≥100) or stent implantation on calcium scoring CT were further excluded from the subtraction protocol. Pre-test probability of CAD (clinical CAD consortium) was calculated based on age, sex, symptoms, and cardiovascular risk factors. FFR was provided for participation after comprehensive evaluation by cardiologists and radiologists. Figure 1 shows the patient inclusion flowchart.
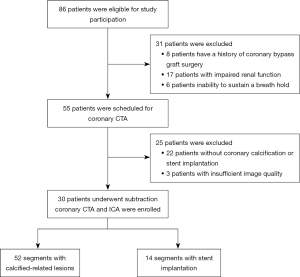
The study complied with the Declaration of Helsinki (as revised in 2013). This prospective study protocol was approved by the Institutional Ethical Committee of the Peking Union Medical College Hospital (No. HS-2427), and written informed consents were obtained from all the patients.
Subtraction coronary CTA acquisition
Subtraction coronary CTA was conducted using a 320-row detector CT scanner (Aquilion ONE GENESIS Edition; Canon Medical Systems Corp, Otawara, Japan) with a collimation of 320 mm × 0.5 mm, a gantry rotation time of 275 ms and a z-coverage of 120–160 mm. Breathing training was given before the CTA scan. Sublingual nitroglycerin (0.5–1.0 mg) was used 1–2 min before the CTA scan. Patients with a heart rate ≥70 bpm were administered β blockers (metoprolol tartrate tablets, 25–50 mg, AstraZeneca) before the examination. A two-breath-hold subtraction protocol (18) including a noncontrast scan and contrast-enhanced scan was used with prospective ECG triggering. Iodinated contrast agent (Iopamidol Injection, 370 mg I/mL, Shanghai Bracco Sine Pharmaceutical Corp, Shanghai, China) was injected at a rate of body weight (kg) × 0.053 mL/s in 10 seconds (fixed), followed by 30 mL of saline at the same injection rate. A bolus-tracking technique was used with a trigger threshold of 280 Hounsfield units in the ascending aorta. The tube voltage was set at 100 kVp, while the tube current was adjusted automatically with a noise index SD =33, ranging from 250 to 900 mA in this study.
CT image reconstruction
The coronary CTA image data were reconstructed using hybrid iterative reconstruction [HIR, Adaptive Iterative Dose Reduction (AIDR) 3D, Cardiac kernel, FC09] and DLR [Advanced Intelligent Clear-IQ Engine (AiCE), Cardiac kernel, Canon Medical Systems] with a slice thickness of 0.5 mm and an interval of 0.25 mm. Subtraction images were derived using dedicated post-processing software (SURESubtraction Canon Medical Systems, Otawara, Japan). The effective dose was calculated by multiplying the dose length product (DLP) with a conversion coefficient k for cardiac examination (k=0.026) (19).
Quantitative and qualitative image quality evaluation
For quantitative image quality evaluation, the mean attenuation value and adjacent adipose tissue in aortic root and in proximal segment of the three main coronary arteries. The signal-to-noise ratio (SNR) and contrast-to-noise ratio (CNR) of each part were calculated (16). The circular region of interest (ROI) should be as large as possible but avoid the tube wall and calcification. For qualitative image quality evaluation, the image quality was qualitatively evaluated using the Likert 4 stage score, with score 1 means excellent and score 4 means nondiagnostic (16).
Coronary CTA and CT-FFR analysis
All images were transferred to a dedicated workstation (Syngo.via VB10 coronary CTA, Siemens Healthcare, Forchheim, Germany) (20). For CT-FFR analysis, the conventional and subtraction coronary CTA images of HIR and DLR were also transferred to a dedicated software prototype (cFFR, Version 3.2.5, Siemens, currently not commercially available). The lumen centerlines or lumen contours were extracted and delineated automatically and then corrected by an experienced observer. After severe stenoses were identified, a patient-specific model of the coronary artery tree was generated, and the CT-FFR values were available. CT-FFR value measurements were initiated 20 mm distal to the stenotic area. A CT-FFR value ≤0.80 was defined as hemodynamically significant stenosis. Two observers with six and two years of experience performed stenosis and CT-FFR measurements blinded to the results of invasive coronary angiography or FFR values.
Invasive coronary angiography and FFR
ICA was performed by experienced cardiologists (with more than 10 years of experience) on an Allura Xper UNIQ FD10 system (Philips Medical Systems). During angiography, images were acquired in multiple projections, where at least two orthogonal projections were obtained to assess target vessels. FFR is provided for moderate-to-severe stenosis caused by calcification or after stent implantation. FFR was measured using a sensor-tipped 0.014-inch pressure wire at maximal hyperemia induced by the venous infusion of adenosine triphosphate (140 µg/kg/min). Cardiologists performed FFR blinded to the CT-FFR results. Hemodynamically significant stenosis was defined as FFR ≤0.8.
Statistical analysis
Statistical analyses were performed using R software (version 3.6.1; http://www.R-project.org). Diagnostic performance comparisons were conducted among different approaches, namely, CT-FFRHIR or DLR, and subtraction CT-FFRHIR or DLR, in three groups, including the calcified-related stenosis group, coronary stent group and all patient groups. Diagnostic performance, including sensitivity, specificity, negative predictive value (NPV), positive predictive value (PPV), diagnostic accuracy, and area under the area under receiver-operator characteristics curve (ROC-AUC) for each approach to identify hemodynamically significant stenosis, were calculated and compared, with invasive FFR serving as a reference standard. The R package DtCompair (https://cran.r-project.org/web/packages/DTComPair/index.html) was used to compute P values for the binary diagnostic tests. In this package, the statistical method for comparison of sensitivity and specificity was McNemar; and the method for comparison of PPV and NPV was weighted generalized score statistical. ROC-AUCs were compared by the Delong method. Benjamini-Hochberg correction was used for multiple testing, P<0.05 was considered significant.
Results
Patient sample
A total of 96 patients with known or suspected CAD were eligible for study participation. Based on predefined exclusion criteria, 8 patients were excluded due to a history of coronary bypass graft surgery, 17 patients were excluded due to impaired renal function, and 6 patients were excluded due to the inability to sustain a breath hold. A total of 22 patients without coronary calcification or stent implantation on calcium scoring CT were excluded from the subtraction protocol. A total of 3 patients were excluded due to insufficient image quality. Eleven patients failed to undergo invasive coronary and FFR within 4 weeks. Finally, 30 patients (20 men, 10 women; mean age, 63.9±6.5 years) with 52 calcified-related lesions and 14 coronary stents were included in this study. The clinical CAD consortium showed the mean pre-test probabilities was 45%±20% (ranging from 17% to 85%). The median Agatston score (25%, 75%) of patients without stent implantation was 795.4 (447.4, 2,050.5), ranged from 110.4 to 3,070.8. The median time between CT and ICA/FFR was 3 days (IQR, 1–7 days). Table 1 shows the characteristics of the 30 patients.
Table 1
Characteristic | Value |
---|---|
Age, years | 63.9±6.5 |
Male | 20 (66.7) |
Body mass index, kg/m2 | 25.9±3.8 |
Diabetes | 16 (53.3) |
Hypertension | 21 (70) |
Hypercholesterolemia | 17 (56.7) |
Smoking | 19 (63.3) |
CAD family history | 10 (33.3) |
CTDIvol (mGy) | 38.6±9.5 |
DLP (mGy×cm) | 142.8±75.9 |
Effective dose (mSv) | 3.7±2.0 |
Heart rate, bpm | 65.7±12.1 |
Number of patients with lesions | |
One lesion | 8 (26.7) |
Two lesions | 9 (30.0) |
Three lesions | 13 (43.4) |
Diabetes was defined as fasting glucose levels ≥7 mmol/L or postprandial blood sugar ≥11.1 mmol/L. Hypertension was defined as a blood pressure ≥140/90 mmHg or use of antihypertensive medication. Hypercholesterolemia was defined as a total cholesterol ≥5.2 mmol/L) or treatment with cholesterol-lowering medications. Smoking was defined as current or past smoking. DLP and Effective dose were for the sum of two CT scans. The value of age, body mass index, CTDIvol, DLP, effective dose and heart rate were present with mean ± SD; other information were presented with number (percentage). CAD, coronary artery disease; CTDIvol, volume CT dose index; DLP, dose length product; CT, computed tomography; SD, standard deviation.
Quantitative and qualitative image quality evaluation
There was significant difference in noise at the aortic root between DLR and HIR-based CTA images (18.38±2.67 vs. 27.21±3.67, P<0.001), and between DLR and HIR-based subtraction images (25.03±4.02 vs. 36.27±6.18, P<0.001).
Tables 2,3 show the quantitative and qualitative image quality evaluation.
Table 2
Variables | CTAHIR | CTADLR | CTAsHIR | CTAsDLR | P values | |
---|---|---|---|---|---|---|
CTADLR vs. CTAHIR | CTAsHIR vs. CTAsDLR | |||||
CT attenuation | ||||||
Ao | 486.78±93.22 | 475.62±85.43 | 435.71±92.25 | 428.91±91.30 | <0.001 | 0.002 |
LM | 445.67±89.90 | 438.04±91.83 | 416.17±91.97 | 396.67±80.57 | 0.21 | 0.007 |
LAD | 374.78±123.47 | 365.73±117.49 | 348.91±101.63 | 332.70±97.90 | 0.26 | 0.005 |
LCX | 385.45±88.97 | 374.41±89.34 | 351.58±92.89 | 307.24±120.48 | 0.02 | 0.001 |
RCA | 413.17±84.68 | 417.94±85.71 | 389.34±83.08 | 384.27±85.09 | 0.66 | 0.31 |
Noise | ||||||
Ao | 27.21±3.67 | 18.38±2.67 | 36.27±6.18 | 25.03±4.02 | <0.001 | <0.001 |
LM | 25.63±8.16 | 16.82±7.55 | 28.70±10.58 | 21.03±9.92 | <0.001 | <0.001 |
LAD | 24.85±10.31 | 18.51±8.72 | 26.26±8.55 | 18.73±6.43 | 0.01 | <0.001 |
LCX | 22.66±8.65 | 17.55±8.54 | 25.83±10.46 | 21.77±10.59 | 0.001 | 0.02 |
RCA | 23.09±8.22 | 20.79±7.52 | 26.93±9.53 | 23.80±9.08 | 0.24 | 0.05 |
SNR | ||||||
Ao | 18.18±4.08 | 26.51±6.43 | 12.47±3.81 | 18.11±8.11 | <0.001 | <0.001 |
LM | 16.65±3.88 | 24.40±6.45 | 11.96±3.95 | 16.78±7.52 | <0.001 | <0.001 |
LAD | 13.82±4.47 | 20.32±7.73 | 10.10±4.11 | 13.87±6.89 | <0.001 | <0.001 |
LCX | 14.40±3.82 | 20.97±6.83 | 10.13±3.78 | 13.08±7.24 | <0.001 | <0.001 |
RCA | 15.51±4.18 | 23.15±5.50 | 10.99±2.70 | 15.86±5.03 | <0.001 | <0.001 |
CNR | ||||||
Ao | 22.01±4.56 | 32.39±7.34 | 15.39±4.20 | 22.27±9.09 | <0.001 | <0.001 |
LM | 20.45±4.15 | 30.17±7.26 | 14.90±4.35 | 20.73±8.31 | <0.001 | <0.001 |
LAD | 17.61±4.51 | 25.63±8.06 | 13.12±4.45 | 18.00±7.54 | <0.001 | <0.001 |
LCX | 18.28±3.95 | 26.50±7.33 | 13.03±4.15 | 16.80±7.30 | <0.001 | <0.001 |
RCA | 19.38±4.48 | 28.54±5.76 | 13.79±2.91 | 19.82±5.79 | <0.001 | <0.001 |
The value of CT attenuation, noise, SNR and CNR were present with mean ± SD. CTA, CT angiography; DLR, deep learning-based image restoration; HIR, hybrid iterative reconstruction; sDLR, subtraction deep learning-based image restoration; sHIR, subtraction hybrid iterative reconstruction; Ao, aorta root; LM, left main artery; LAD, left anterior descending; LCX, left circumflex; RCA, right coronary artery; CT, computed tomography; SD, standard deviation.
Table 3
Variables | Mean | Diagnostic segments | Non-diagnostic segments | P values | |||||
---|---|---|---|---|---|---|---|---|---|
S1 | S2 | S3 | S4 | CTAHIR vs. CTADLR | CTAsHIR vs. CTAsDLR | ||||
CTAHIR | 1.47±0.63 | 18 (60%) | 10 (33%) | 2 (7%) | 0 (0%) | <0.001 | <0.001 | ||
CTAsHIR | 1.77±0.73 | 12 (40%) | 13 (43%) | 5 (17%) | 0 (0%) | ||||
CTADLR | 1.10±0.41 | 28 (93%) | 1 (3%) | 1 (3%) | 0 (0%) | ||||
CTAsDLR | 1.13±0.35 | 26 (87%) | 4 (13%) | 0 (0%) | 0 (0%) |
The value of mean score was present with mean ± SD. The number of diagnostic or non-diagnostic segments was present with number (percentage). CTA, CT angiography; DLR, deep learning-based image restoration; HIR, hybrid iterative reconstruction; sDLR, subtraction deep learning-based image restoration; sHIR, subtraction hybrid iterative reconstruction; CT, computed tomography; SD, standard deviation.
Diagnostic performance in calcified-related stenoses
For calcified-related lesions, 17/52 (32.7%) lesions showed invasive FFR ≤0.8. Subtraction CT-FFRDLR showed highest diagnostic performance than other approaches at the lesion-based level, and the ROC-AUCs for subtraction CT-FFRDLR, CT-FFRDLR, subtraction CT-FFRHIR and CT-FFRHIR were 0.90, 0.70, 0.80 and 0.61, respectively. DLR or HIR-based subtraction CT-FFR outperformed CT-FFR in detecting calcified-related hemodynamically significant stenosis (P=0.01 for DLR; P=0.01 for HIR). The sensitivity, specificity, PPV, NPV, and accuracy in detecting hemodynamically significant stenosis were 100.00%, 71.43%, 62.96%, 100.00% and 80.77%, respectively for subtraction CT-FFRDLR, and 94.12%, 62.86%, 55.17%, 95.65% and 73.08%, respectively for subtraction CT-FFRHIR. Although the diagnostic accuracy was improved from 73.08% to 80.77%, there was no significant difference between DLR or HIR-based subtraction CT-FFR (P=0.15). For patient based level, DLR or HIR-based subtraction CT-FFR shown no significant difference in diagnostic accuracy.
Diagnostic performance in coronary stents
A total of 8/14 (57.1%) stents showed invasive FFR ≤0.8. Subtraction CT-FFRDLR achieved higher diagnostic performance than the other approaches in lesion-based analysis, and the ROC-AUCs for subtraction CT-FFRDLR were 0.85. Subtraction CT-FFRDLR showed equal sensitivity (100.00% vs. 100.00%, P>0.99) and NPV (100.00% vs. 100.00%, P>0.99), higher specificity (83.33% vs. 16.67%, P<0.001), PPV (88.89% vs. 61.54%, P=0.10), and accuracy (92.86% vs. 64.29%, P=0.04) than CT-FFRDLR. Although the diagnostic specificity was improved from 50.00% to 83.33%, there was no significant difference between DLR or HIR-based subtraction CT-FFR. In patient-based analysis for coronary stents, both DLR and HIR-based subtraction CT-FFR shown excellent diagnostic performance.
Diagnostic performance in all patients
A total of 25/66 (37.9%) lesions showed invasive FFR ≤0.8. At the lesion-based level, the ROC-AUCs for subtraction CT-FFRDLR in detecting hemodynamically significant stenosis were superior to CT-FFRDLR (0.89 vs. 0.74, P=0.01). The sensitivity, specificity, PPV, NPV, and accuracy for subtraction CT-FFRDLR were 100.00%, 73.17%, 69.44%, 100.00% and 83.33%, respectively. The ROC-AUCs for subtraction CT-FFRHIR in detecting hemodynamically significant stenosis were superior to CT-FFRHIR (0.81 vs. 0.61, P=0.008). The sensitivity, specificity, PPV, NPV, and accuracy for subtraction CT-FFRHIR were 96.00%, 60.98%, 60.00%, 96.15% and 74.24%, respectively. Although the diagnostic accuracy was improved from 74.24% to 83.33%, there was no significant difference between DLR or HIR-based subtraction CT-FFR (P=0.07). Tables 4,5 summarize the diagnostic performance of different approaches at the lesion-based level and corresponding P values. Tables 6,7 summarize the diagnostic performance of different approaches at the patient-based level and corresponding P values. Figure 2 shows the area under the curve comparison. Figures 3,4 show the representative cases.
Table 4
Variables | TP | FP | TN | FN | Sensitivity (%) | Specificity (%) | PPV (%) | NPV (%) | Accuracy (%) | AUC |
---|---|---|---|---|---|---|---|---|---|---|
Calcified-related stenoses (n=52) | ||||||||||
CT-FFRHIR | 14 | 18 | 17 | 3 | 82.35 (60.59, 100.00) |
48.57 (31.87, 67.09) |
43.75 (26.67, 60.71) |
85.00 (66.07, 100.00) |
59.62 (46.15, 73.08) |
0.61 (0.44, 0.77) |
Subtraction CT-FFRHIR | 16 | 13 | 22 | 1 | 94.12 (80.00, 100.00) |
62.86 (47.06, 78.86) |
55.17 (36.53, 71.93) |
95.65 (85.71, 100.00) |
73.08 (61.54, 84.62) |
0.80 (0.66, 0.93) |
CT-FFRDLR | 13 | 14 | 21 | 4 | 76.47 (51.40, 94.44) |
60.00 (43.33, 76.32) |
48.15 (29.83, 66.67) |
84.00 (67.93, 96.36) |
65.38 (51.92, 78.85) |
0.70 (0.55, 0.86) |
Subtraction CT-FFRDLR | 17 | 10 | 25 | 0 | 100.00 (100.00, 100.00) |
71.43 (54.42, 86.75) |
62.96 (43.62, 81.48) |
100.00 (100.00, 100.00) |
80.77 (69.23, 90.38) |
0.90 (0.81, 0.98) |
Stents (n=14) | ||||||||||
CT-FFRHIR | 7 | 6 | 0 | 1 | 87.50 (58.67, 100.00) |
0.00 (0.00, 0.00) |
53.85 (26.21, 83.33) |
0.00 (0.00, 0.00) |
50.00 (21.43, 78.57) |
0.50 (0.16, 0.84) |
Subtraction CT-FFRHIR | 8 | 3 | 3 | 0 | 100.00 (100.00, 100.00) |
50.00 (0.00, 100.00) |
72.73 (45.45, 100.00) |
100.00 (100.00, 100.00) |
78.57 (57.14, 100.00) |
0.75 (0.41, 1.00) |
CT-FFRDLR | 8 | 5 | 1 | 0 | 100.00 (100.00, 100.00) |
16.67 (0.00, 50.00) |
61.54 (35.71, 91.67) |
100.00 (100.00, 100.00) |
64.29 (42.86, 92.86) |
0.82 (0.59, 1.00) |
Subtraction CT-FFRDLR | 8 | 1 | 5 | 0 | 100.00 (100.00, 100.00) |
83.33 (40.00. 100.00) |
88.89 (35.71, 91.67) |
100.00 (100.00, 100.00) |
92.86 (78.57, 100.00) |
0.85 (0.57, 1.00) |
All (n=66) | ||||||||||
CT-FFRHIR | 21 | 24 | 17 | 4 | 84.00 (66.67, 96.15) |
41.46 (26.58, 57.51) |
46.67 (31.35, 60.47) |
80.95 (60.87, 95.65) |
57.58 (45.45, 70.40) |
0.61 (0.47, 0.75) |
Subtraction CT-FFRHIR | 24 | 16 | 25 | 1 | 96.00 (86.67, 100.00) |
60.98 (46.43, 76.92) |
60.00 (43.21, 74.62) |
96.15 (86.96, 100.00) |
74.24 (63.64, 84.85) |
0.81 (0.69, 0.92) |
CT-FFRDLR | 21 | 19 | 22 | 4 | 84.00 (68.74, 96.07) |
53.66 (37.36, 68.29) |
52.50 (35.05, 67.06) |
84.62 (70.37, 96.30) |
65.15 (53.03, 75.76) |
0.74 (0.61, 0.86) |
Subtraction CT-FFRDLR | 25 | 11 | 30 | 0 | 100.00 (100.00, 100.00) |
73.17 (59.46, 86.29) |
69.44 (54.55, 83.98) |
100.00 (100.00, 100.00) |
83.33 (74.24, 90.91) |
0.89 (0.82, 0.97) |
The values for sensitivity, specificity, PPV, NPV and accuracy are presented with 95% CIs. TP, true positive; FP, false positive; TN, true negative; FN, false negative; PPV, positive predictive value; NPV, negative predictive value; AUC, area under the curve; CT-FFR, CT angiography-derived fractional flow reserve; HIR, hybrid iterative reconstruction; DLR, deep learning reconstruction; CT, computed tomography; CI, confidence interval.
Table 5
Variables | Sensitivity | Specificity | PPV | NPV | Accuracy | AUC |
---|---|---|---|---|---|---|
Calcified-related stenoses (n=52) | ||||||
CT-FFRHIRvs. subtraction CT-FFRHIR | 0.32 | 0.21 | 0.06 | 0.16 | 0.06 | 0.01 |
CT-FFRDLRvs. subtraction CT-FFRDLR | 0.20 | 0.21 | 0.06 | 0.12 | 0.06 | 0.01 |
CT-FFRHIRvs. CT-FFRDLR | 0.56 | 0.21 | 0.42 | 0.88 | 0.39 | 0.15 |
Subtraction CT-FFRHIRvs. subtraction CT-FFRDLR | 0.43 | 0.32 | 0.31 | 0.39 | 0.28 | 0.15 |
Stents (n=14) | ||||||
CT-FFRHIRvs. subtraction CT-FFRHIR | 0.64 | 0.16 | 0.10 | <0.001 | 0.04 | 0.39 |
CT-FFRDLRvs. subtraction CT-FFRDLR | >0.99 | <0.001 | 0.10 | >0.99 | 0.04 | 0.85 |
CT-FFRHIRvs. CT-FFRDLR | 0.64 | 0.32 | 0.16 | <0.001 | 0.15 | 0.32 |
Subtraction CT-FFRHIRvs. subtraction CT-FFRDLR | >0.99 | 0.21 | 0.16 | >0.99 | 0.15 | 0.39 |
All (n=66) | ||||||
CT-FFRHIRvs. subtraction CT-FFRHIR | 0.16 | 0.04 | 0.008 | 0.04 | <0.001 | 0.008 |
CT-FFRDLRvs. subtraction CT-FFRDLR | 0.16 | 0.04 | 0.008 | 0.04 | 0.004 | 0.01 |
CT-FFRHIRvs. CT-FFRDLR | >0.99 | 0.13 | 0.17 | 0.62 | 0.15 | 0.04 |
Subtraction CT-FFRHIRvs. subtraction CT-FFRDLR | 0.43 | 0.13 | 0.13 | 0.36 | 0.07 | 0.09 |
The P values for sensitivity, specificity, PPV, NPV and accuracy are presented. Benjamini-Hochberg correction was used for multiple testing, P<0.05 was considered significant. PPV, positive predictive value; NPV, negative predictive value; AUC, area under the curve; CT-FFR, coronary CT angiography-derived fractional flow reserve; HIR, hybrid iterative reconstruction; DLR, deep learning reconstruction; CT, computed tomography.
Table 6
Variables | TP | FP | TN | FN | Sensitivity (%) | Specificity (%) | PPV (%) | NPV (%) | Accuracy (%) | AUC |
---|---|---|---|---|---|---|---|---|---|---|
Calcified-related stenoses (n=28) | ||||||||||
CT-FFRHIR | 11 | 13 | 2 | 2 | 84.62 (61.54, 100.00) |
13.33 (0.00, 34.25) |
45.83 (25.49, 65.22) |
50.00 (0.00, 100.00) |
46.43 (28.57, 64.29) |
0.66 (0.44, 0.87) |
Subtraction CT-FFRHIR | 13 | 10 | 5 | 0 | 100.00 (100.00, 100.00) |
33.33 (0.11, 60.00) |
56.52 (34.78, 78.26) |
100.00 (100.00, 100.00) |
64.29 (44.76, 82.14) |
0.72 (0.52, 0.93) |
CT-FFRDLR | 11 | 12 | 3 | 2 | 84.62 (62.05, 100.00) |
20.00 (0.00, 41.18) |
47.83 (28.30, 68.18) |
60.00 (0.00, 100.00) |
50.00 (32.14, 67.86) |
0.57 (0.35, 0.79) |
Subtraction CT-FFRDLR | 13 | 10 | 5 | 0 | 100.00 (100.00, 100.00) |
33.33 (9.10, 59.11) |
56.52 (36.36, 77.51) |
100.00 (100.00, 100.00) |
64.29 (46.43, 82.14) |
0.82 (0.66, 0.97) |
Stents (n=11) | ||||||||||
CT-FFRHIR | 8 | 3 | 0 | 0 | 100.00 (100.00, 100.00) |
0.00 (0.00, 100.00) |
72.73 (45.45, 95.15) |
0.00 (0.00, 0.00) |
72.73 (45.45, 95.15) |
0.67 (0.01, 1.00) |
Subtraction CT-FFRHIR | 8 | 0 | 3 | 0 | 100.00 (100.00, 100.00) |
100.00 (100.00, 100.00) |
100.00 (100.00, 100.00) |
100.00 (100.00, 100.00) |
100.00 (100.00, 100.00) |
1.00 (1.00, 1.00) |
CT-FFRDLR | 8 | 2 | 1 | 0 | 100.00 (100.00, 100.00) |
33.33 (0.11, 60.00) |
80.00 (52.42, 100.00) |
100.00 (100.00, 100.00) |
81.82 (54.55, 100.00) |
0.92 (0.73, 1.00) |
Subtraction CT-FFRDLR | 8 | 0 | 3 | 0 | 100.00 (100.00, 100.00) |
100.00 (100.00, 100.00) |
100.00 (100.00, 100.00) |
100.00 (100.00, 100.00) |
100.00 (100.00, 100.00) |
1.00 (1.00, 1.00) |
All (n=30) | ||||||||||
CT-FFRHIR | 17 | 10 | 1 | 2 | 89.47 (75.00, 100.00) |
9.09 (0.00, 33.33) |
62.96 (44.44, 80.77) |
33.33 (0.00, 100.00) |
60.00 (43.33, 76.67) |
0.64 (0.42, 0.86) |
Subtraction CT-FFRHIR | 19 | 8 | 3 | 0 | 100.00 (100.00, 100.00) |
27.27 (0.00, 57.14) |
70.37 (51.79, 87.06) |
100.00 (100.00, 100.00) |
73.33 (56.67, 88.22) |
0.72 (0.49, 0.95) |
CT-FFRDLR | 17 | 8 | 3 | 2 | 89.47 (72.22, 100.00) |
27.27 (0.00, 57.14) |
68.00 (48.08, 8.43) |
60.00 (0.00, 100.00) |
66.67 (48.44, 83.33) |
0.70 (0.49, 0.91) |
Subtraction CT-FFRDLR | 19 | 8 | 3 | 0 | 100.00 (100.00, 100.00) |
27.27 (0.00, 57.14) |
70.37 (52.77, 86.80) |
100.00 (100.00, 100.00) |
73.33 (56.67, 88.22) |
0.78 (0.60, 0.96) |
The values for sensitivity, specificity, PPV, NPV and accuracy are presented with 95% CIs. TP, true positive; FP, false positive; TN, true negative; FN, false negative; PPV, positive predictive value; NPV, negative predictive value; AUC, area under the curve; CT-FFR, CT angiography-derived fractional flow reserve; HIR, hybrid iterative reconstruction; DLR, deep learning reconstruction; CT, computed tomography; CI, confidence interval.
Table 7
Variables | Sensitivity | Specificity | PPV | NPV | Accuracy | AUC |
---|---|---|---|---|---|---|
Calcified-related stenoses (n=28) | ||||||
CT-FFRHIRvs. subtraction CT-FFRHIR | 0.32 | 0.32 | 0.10 | 0.18 | 0.04 | 0.69 |
CT-FFRDLRvs. subtraction CT-FFRDLR | 0.32 | 0.32 | 0.10 | 0.18 | 0.04 | 0.16 |
CT-FFRHIRvs. CT-FFRDLR | >0.99 | 0.43 | 0.79 | 0.83 | 0.76 | 0.69 |
Subtraction CT-FFRHIRvs. subtraction CT-FFRDLR | >0.99 | >0.99 | >0.99 | >0.99 | >0.99 | 0.69 |
Stents (n=11) | ||||||
CT-FFRHIRvs. subtraction CT-FFRHIR | 0.64 | <0.001 | 0.12 | <0.001 | 0.16 | 0.69 |
CT-FFRDLRvs. subtraction CT-FFRDLR | >0.99 | <0.001 | 0.30 | >0.99 | 0.24 | 0.69 |
CT-FFRHIRvs. CT-FFRDLR | >0.99 | 0.43 | 0.80 | >0.99 | 0.35 | 0.69 |
Subtraction CT-FFRHIRvs. subtraction CT-FFRDLR | >0.99 | >0.99 | >0.99 | >0.99 | >0.99 | >0.99 |
All (n=30) | ||||||
CT-FFRHIRvs. subtraction CT-FFRHIR | 0.32 | 0.32 | 0.24 | 0.20 | 0.16 | 0.76 |
CT-FFRDLRvs. subtraction CT-FFRDLR | 0.32 | >0.99 | 0.28 | 0.20 | 0.26 | 0.76 |
CT-FFRHIRvs. CT-FFRDLR | >0.99 | 0.32 | 0.28 | 0.40 | 0.41 | 0.76 |
Subtraction CT-FFRHIRvs. subtraction CT-FFRDLR | >0.99 | >0.99 | >0.99 | >0.99 | >0.99 | 0.76 |
The P values for sensitivity, specificity, PPV, NPV and accuracy are presented. Benjamini-Hochberg correction was used for multiple testing, P<0.05 was considered significant. PPV, positive predictive value; NPV, negative predictive value; AUC, area under the curve; CT-FFR, CT angiography-derived fractional flow reserve; HIR, hybrid Iterative reconstruction; DLR, deep learning reconstruction; CT, computed tomography.
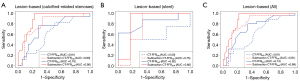
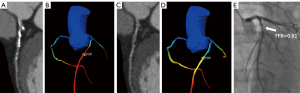
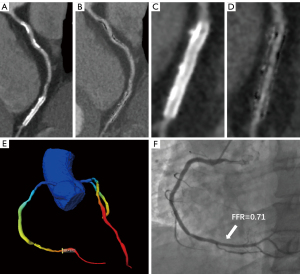
Discussion
This study compared different approaches in the evaluation of hemodynamically significant stenosis and demonstrated that subtraction CT-FFR yielded optimal diagnostic performance for calcified-related stenosis. The application of subtraction CT-FFR in the evaluation of coronary stents was feasible. The diagnostic performance of subtraction CT-FFRDLR was better than the subtraction CT-FFRHIR, but there was no significant difference.
As the correlation between anatomical and functional significance of coronary lesions is poor (21), previous study CT-FFR yielded superior diagnostic performance for hemodynamically significant stenosis to coronary CTA (22). In the presence of blooming artifacts caused by severe calcification or stents, the coronary lumen could be obscured and would result in inappropriate CT-FFR assessment. When the Agatston score is larger than 400, the specificity of CT-FFR is significantly decreased (6). In line with Kamo et al. (22), calcification was eliminated on subtraction images, and we showed that FFR derived from HIR-based subtraction CTA images yielded optimal diagnostic performance in hemodynamic significant stenosis assessment. Compared to CT-FFRHIR, the lesion-based diagnostic accuracy of subtraction CT-FFRHIR improved from 59.62% to 73.08%, the specificity improved from 48.57% to 62.86%. Moreover, DLR could improve edge delineation, the edges of calcifications and stents become sharper compared to the normal reconstruction approach (23). Whether DLR combined with subtraction techniques could yield higher diagnostic performance has not been investigated. In the present study, the lesion-based false positive rate of CT-FFRHIR (36.4%), CT-FFRDLR and subtraction CT-FFRHIR were 36.4%, 28.2% and 24.2%, respectively, subtraction CT-FFRDLR could reduce the false positive rate to 18.3%, and reduced unnecessary invasive tests. However, there was no significant difference between DLR or HIR-based subtraction CT-FFR, while the diagnostic accuracy was improved from 73.08% to 80.77% (P=0.28). This situation could be attributed to the relatively small sample size and the stability of machine learning-based CT-FFR, which was trained with a large scale of data. The difference in image quality did not affect the value of machine learning-based CT-FFR (24).
To our knowledge, this is the first study to apply subtraction CT-FFR to coronary stent evaluation. Previous studies have shown an association between post-intervention FFR values and adverse clinical outcomes (25,26). The applications of CT-FFR in patients with stent implantation are rare. Andreini et al. (27) showed that CT-FFR can be used after stent implantation in a case report. Tang et al. (28) demonstrated the feasibility and prognostic implications of CT-FFR in patients with stent implantation. In our study, DLR-based subtraction CT-FFR outperformed CT-FFR in detecting hemodynamic ISR. The specificity of subtraction CT-FFRDLR improved from 16.67% to 83.33% when compared to CT-FFRDLR (P<0.001). Collison et al. (29) showed FFR after percutaneous coronary intervention had a correlation with angina frequency score at follow-up. However, the application of FFR is limited by the invasive procedure and high cost. With the high diagnostic performance and affordable price, noninvasive hemodynamic assessment using subtraction CT-FFR could inform expectations of angina relief and quality of life improvement after stent implantation. The wide use of subtraction images was limited by severe misregistration artifacts and additional radiation exposure. Breathing training before subtraction coronary CTA ensures good image quality of CTA and subtraction CTA. Three patients were excluded due to insufficient image quality (misregistration), which was lower than previously reported (11). DLR allows a reduction in radiation exposure with improved image quality, and the combination of DLR and subtraction CTA may have potential for expanding applications of subtraction protocols. The mean effective radiation dose in this study was 3.7±2.0 mSv. DLR-based low-radiation dose subtraction protocols could be investigated in future studies. In addition, with the development of deep learning algorithms, its application in image reconstruction may have different performance, the comparison of different reconstruction methods could also be investigated in future studies.
Conclusions
This study had some limitations. First, our study was performed at a single center, the sample size was relatively small and our study performed on a single vendor and used a single CT-FFR calculation method. Multicenter studies and analysis with a large cohort, different vendors and different CT-FFR calculation methods are needed. Second, using invasive FFR as a reference standard could lead to selection bias. Third, patients with high heart rates may not be suitable for subtraction method, increased radiation dose and misregistration artifact of subtraction images cannot be ignored, modified subtraction protocol (i.e., DLR-based low-radiation dose) should be investigated to further optimize subtraction CT-FFR application. Forth, a two breath-hold technique was used in this study due to old people could not hold their breath for too long, breath training was performed before CT scans.
In conclusion, our study demonstrated that subtraction CT-FFR yielded optimal diagnostic performance for hemodynamically significant calcified-related stenosis, and the application of subtraction CT-FFR in the evaluation of coronary stents were feasible. The diagnostic performance of subtraction CT-FFRDLR was better than that of subtraction CT-FFRHIR, but there was no significant difference.
Acknowledgments
Footnote
Reporting Checklist: The authors have completed the STARD reporting checklist. Available at https://qims.amegroups.com/article/view/10.21037/qims-24-1366/rc
Funding: This work was supported by
Conflicts of Interest: All authors have completed the ICMJE uniform disclosure form (available at https://qims.amegroups.com/article/view/10.21037/qims-24-1366/coif). M.X. is an employee of Canon Medical System. The other authors have no conflicts of interest to declare.
Ethical Statement: The authors are accountable for all aspects of the work in ensuring that questions related to the accuracy or integrity of any part of the work are appropriately investigated and resolved. The study complied with the Declaration of Helsinki. This study was approved by the Institutional Ethics Committee of the Peking Union Medical College Hospital (No. HS-2427). Written informed consents were obtained from all the patients.
Open Access Statement: This is an Open Access article distributed in accordance with the Creative Commons Attribution-NonCommercial-NoDerivs 4.0 International License (CC BY-NC-ND 4.0), which permits the non-commercial replication and distribution of the article with the strict proviso that no changes or edits are made and the original work is properly cited (including links to both the formal publication through the relevant DOI and the license). See: https://creativecommons.org/licenses/by-nc-nd/4.0/.
References
- Serruys PW, Hara H, Garg S, Kawashima H, Nørgaard BL, Dweck MR, Bax JJ, Knuuti J, Nieman K, Leipsic JA, Mushtaq S, Andreini D, Onuma Y. Coronary Computed Tomographic Angiography for Complete Assessment of Coronary Artery Disease: JACC State-of-the-Art Review. J Am Coll Cardiol 2021;78:713-36. [Crossref] [PubMed]
- Min JK, Leipsic J, Pencina MJ, Berman DS, Koo BK, van Mieghem C, Erglis A, Lin FY, Dunning AM, Apruzzese P, Budoff MJ, Cole JH, Jaffer FA, Leon MB, Malpeso J, Mancini GB, Park SJ, Schwartz RS, Shaw LJ, Mauri L. Diagnostic accuracy of fractional flow reserve from anatomic CT angiography. JAMA 2012;308:1237-45. [Crossref] [PubMed]
- Koo BK, Erglis A, Doh JH, Daniels DV, Jegere S, Kim HS, Dunning A, DeFrance T, Lansky A, Leipsic J, Min JK. Diagnosis of ischemia-causing coronary stenoses by noninvasive fractional flow reserve computed from coronary computed tomographic angiograms. Results from the prospective multicenter DISCOVER-FLOW (Diagnosis of Ischemia-Causing Stenoses Obtained Via Noninvasive Fractional Flow Reserve) study. J Am Coll Cardiol 2011;58:1989-97. [Crossref] [PubMed]
- Nørgaard BL, Leipsic J, Gaur S, Seneviratne S, Ko BS, Ito H, et al. Diagnostic performance of noninvasive fractional flow reserve derived from coronary computed tomography angiography in suspected coronary artery disease: the NXT trial (Analysis of Coronary Blood Flow Using CT Angiography: Next Steps). J Am Coll Cardiol 2014;63:1145-55. [Crossref] [PubMed]
- Coenen A, Lubbers MM, Kurata A, Kono A, Dedic A, Chelu RG, Dijkshoorn ML, Gijsen FJ, Ouhlous M, van Geuns RJ, Nieman K. Fractional flow reserve computed from noninvasive CT angiography data: diagnostic performance of an on-site clinician-operated computational fluid dynamics algorithm. Radiology 2015;274:674-83. [Crossref] [PubMed]
- Han D, Lin A, Gransar H, Dey D, Berman DS. Influence of Coronary Artery Calcium Score on Computed Tomography-Derived Fractional Flow Reserve: A Meta-Analysis. JACC Cardiovasc Imaging 2021;14:702-3. [Crossref] [PubMed]
- Thakur U, Khav N, Comella A, Michail M, Ihdayhid AR, Poon E, Nicholls SJ, Ko B, Brown AJ. Fractional Flow Reserve following Percutaneous Coronary Intervention. J Interv Cardiol 2020;2020:7467943. [Crossref] [PubMed]
- Nazir MS, Mittal TK, Weir-McCall J, Nieman K, Channon K, Nicol ED. Opportunities and challenges of implementing computed tomography fractional flow reserve into clinical practice. Heart 2020;106:1387-93. [Crossref] [PubMed]
- Viladés Medel D, Leta R, Alomar Serralach X, Carreras Costa F, Pons-Lladó G. Reliability of a new method for coronary artery calcium or metal subtraction by 320-row cardiac CT. Eur Radiol 2016;26:3208-14. [Crossref] [PubMed]
- Fuchs A, Kühl JT, Chen MY, Helqvist S, Razeto M, Arakita K, Steveson C, Arai AE, Kofoed KF. Feasibility of coronary calcium and stent image subtraction using 320-detector row CT angiography. J Cardiovasc Comput Tomogr 2015;9:393-8. [Crossref] [PubMed]
- Fuchs A, Kühl JT, Chen MY, Viladés Medel D, Alomar X, Shanbhag SM, Helqvist S, Kofoed KF. Subtraction CT angiography improves evaluation of significant coronary artery disease in patients with severe calcifications or stents-the C-Sub 320 multicenter trial. Eur Radiol 2018;28:4077-85. [Crossref] [PubMed]
- Le NQK. Predicting emerging drug interactions using GNNs. Nat Comput Sci 2023;3:1007-8. [Crossref] [PubMed]
- Kha QH, Tran TO, Nguyen TT, Nguyen VN, Than K, Le NQK. An interpretable deep learning model for classifying adaptor protein complexes from sequence information. Methods 2022;207:90-6. [Crossref] [PubMed]
- Kerdvibulvech C, Dong ZY. Roles of Artificial Intelligence and Extended Reality Development in the Post-COVID-19 Era. In: Stephanidis C, et al. HCI International 2021 - Late Breaking Papers: Multimodality, eXtended Reality, and Artificial Intelligence. HCII 2021. Lecture Notes in Computer Science, vol 13095. Springer, Cham 2021.
- Kerdvibulvech C, Chen LL. The Power of Augmented Reality and Artificial Intelligence During the Covid-19 Outbreak. In: Stephanidis C, Kurosu M, Degen H, Reinerman-Jones L, editors. HCI International 2020 - Late Breaking Papers: Multimodality and Intelligence. HCII 2020. Lecture Notes in Computer Science, vol 12424. Springer, Cham.
- Yi Y, Xu C, Xu M, Yan J, Li YY, Wang J, Yang SJ, Guo YB, Wang Y, Li YM, Jin ZY, Wang YN. Diagnostic Improvements of Deep Learning-Based Image Reconstruction for Assessing Calcification-Related Obstructive Coronary Artery Disease. Front Cardiovasc Med 2021;8:758793. [Crossref] [PubMed]
- Xu C, Yi Y, Xu M, Yan J, Guo YB, Wang J, Wang Y, Li YM, Jin ZY, Wang YN. Coronary Artery Stent Evaluation by CTA: Impact of Deep Learning Reconstruction and Subtraction Technique. AJR Am J Roentgenol 2023;220:63-72. [Crossref] [PubMed]
- Yoshioka K, Tanaka R, Muranaka K. Subtraction coronary CT angiography for calcified lesions. Cardiol Clin 2012;30:93-102. [Crossref] [PubMed]
- Trattner S, Halliburton S, Thompson CM, Xu Y, Chelliah A, Jambawalikar SR, Peng B, Peters MR, Jacobs JE, Ghesani M, Jang JJ, Al-Khalidi H, Einstein AJ. Cardiac-Specific Conversion Factors to Estimate Radiation Effective Dose From Dose-Length Product in Computed Tomography. JACC Cardiovasc Imaging 2018;11:64-74. [Crossref] [PubMed]
- Tesche C, De Cecco CN, Baumann S, Renker M, McLaurin TW, Duguay TM, Bayer RR 2nd, Steinberg DH, Grant KL, Canstein C, Schwemmer C, Schoebinger M, Itu LM, Rapaka S, Sharma P, Schoepf UJ, Coronary CT. Angiography-derived Fractional Flow Reserve: Machine Learning Algorithm versus Computational Fluid Dynamics Modeling. Radiology 2018;288:64-72. [Crossref] [PubMed]
- Tonino PA, Fearon WF, De Bruyne B, Oldroyd KG, Leesar MA, Ver Lee PN, Maccarthy PA, Van't Veer M, Pijls NH. Angiographic versus functional severity of coronary artery stenoses in the FAME study fractional flow reserve versus angiography in multivessel evaluation. J Am Coll Cardiol 2010;55:2816-21. [Crossref] [PubMed]
- Kamo Y, Fujimoto S, Nozaki YO, Aoshima C, Kawaguchi YO, Dohi T, Kudo A, Takahashi D, Takamura K, Hiki M, Okai I, Okazaki S, Tomizawa N, Kumamaru KK, Aoki S, Minamino T. Incremental Diagnostic Value of CT Fractional Flow Reserve Using Subtraction Method in Patients with Severe Calcification: A Pilot Study. J Clin Med 2021;10:4398. [Crossref] [PubMed]
- Tatsugami F, Higaki T, Nakamura Y, Yu Z, Zhou J, Lu Y, Fujioka C, Kitagawa T, Kihara Y, Iida M, Awai K. Deep learning-based image restoration algorithm for coronary CT angiography. Eur Radiol 2019;29:5322-9. [Crossref] [PubMed]
- Xu C, Xu M, Yan J, Li YY, Yi Y, Guo YB, Wang M, Li YM, Jin ZY, Wang YN. The impact of deep learning reconstruction on image quality and coronary CT angiography-derived fractional flow reserve values. Eur Radiol 2022;32:7918-26. [Crossref] [PubMed]
- Li SJ, Ge Z, Kan J, Zhang JJ, Ye F, Kwan TW, Santoso T, Yang S, Sheiban I, Qian XS, Tian NL, Rab TS, Tao L, Chen SL. Cutoff Value and Long-Term Prediction of Clinical Events by FFR Measured Immediately After Implantation of a Drug-Eluting Stent in Patients With Coronary Artery Disease: 1- to 3-Year Results From the DKCRUSH VII Registry Study. JACC Cardiovasc Interv 2017;10:986-95. [Crossref] [PubMed]
- Agarwal SK, Kasula S, Hacioglu Y, Ahmed Z, Uretsky BF, Hakeem A. Utilizing Post-Intervention Fractional Flow Reserve to Optimize Acute Results and the Relationship to Long-Term Outcomes. JACC Cardiovasc Interv 2016;9:1022-31. [Crossref] [PubMed]
- Andreini D, Mushtaq S, Pontone G, Rogers C, Pepi M, Bartorelli AL. Severe in-stent restenosis missed by coronary CT angiography and accurately detected with FFR(CT). Int J Cardiovasc Imaging 2017;33:119-20. [Crossref] [PubMed]
- Tang CX, Guo BJ, Schoepf JU, Bayer RR 2nd, Liu CY, Qiao HY, Zhou F, Lu GM, Zhou CS, Zhang LJ. Feasibility and prognostic role of machine learning-based FFR(CT) in patients with stent implantation. Eur Radiol 2021;31:6592-604. [Crossref] [PubMed]
- Collison D, Copt S, Mizukami T, Collet C, McLaren R, Didagelos M, et al. Angina After Percutaneous Coronary Intervention: Patient and Procedural Predictors. Circ Cardiovasc Interv 2023;16:e012511. [Crossref] [PubMed]