Development and validation of a dynamic nomogram for predicting central lymph node metastasis in papillary thyroid carcinoma patients based on clinical and ultrasound features
Introduction
The incidence of thyroid carcinoma, the most prevalent endocrine malignancy, is increasing globally (1). According to the International Agency for Research on Cancer (IARC) of the World Health Organization (WHO), thyroid cancer ranks seventh in terms of global incidence, contributing to a total of 821,214 cases and resulting in 47,507 deaths in 2022 (2). Among the various histopathological subtypes of thyroid carcinoma, papillary thyroid carcinoma (PTC) is most frequently diagnosed and accounts for approximately 80% of all cases (3). Despite being considered an indolent tumor (4), approximately 20–50% of PTC patients exhibit central lymph node metastasis (CLNM), which significantly increases the risk of recurrence (5). CLNM serves as the strongest risk factor for both recurrence and prognosis among PTC patients but also plays a crucial role in determining appropriate surgical approaches preoperatively (6). Prophylactic cervical lymph node dissection (CLND) has long been a subject of controversy (7,8). Some scholars argue that CLND alters tumor-node-metastasis (TNM) staging and reduces the risk of postoperative recurrence in patients (9). Conversely, other studies have indicated insufficient evidence supporting the benefits of prophylactic CLND in reducing recurrence rates and improving prognosis. Moreover, it poses potential surgical complications such as recurrent laryngeal nerve injury and hypoparathyroidism (10). Consequently, it is imperative to accurately evaluate the presence of CLNM prior to surgery.
Ultrasound is favored as a valuable tool for the examination of cervical lymph nodes due to its convenience and noninvasiveness. However, complex anatomical positioning limits the ability of ultrasound imaging to detect CLNM. Conventional ultrasound diagnosis exhibits suboptimal sensitivity in detecting CLNM, with rates ranging from 20% to 33% (11). Furthermore, the ability of ultrasound to identify latent lymph node metastases is limited. Therefore, there is a pressing need for an effective and noninvasive tool to assess CLNM prior to surgery. Biffoni et al. (12) employed structured high-resolution ultrasound examination to accurately map the location of suspicious lymph nodes on anatomical models, in combination with endocrinology written reports, to establish a mapping model that more accurately locates suspicious lymph nodes and demonstrates high specificity for preoperative detection of CLNM. However, the sensitivity of this approach remains relatively limited. In recent years, research has shown a close correlation between the clinical and ultrasound characteristics of PTC patients and the occurrence of CLNM and researchers have attempted to establish a predictive model using clinical and ultrasound features to estimate the likelihood of CLNM occurrence (13,14). However, consistent results have not yet been achieved, and certain models are hindered by practicality issues and lack validation (15).
In this study, we collected clinical information, ultrasound features, American College of Radiology Thyroid Imaging Reporting and Data System (ACR TI-RADS) (16) scores and the Chinese version of the Thyroid Imaging Reporting and Data System (C TI-RADS) (17) scores of patients with PTC to develop and validate a preoperative prediction nomogram model for CLNM. It is envisioned that this tool will be used to accurately perform preoperative staging and risk stratification of PTC, which can guide surgical resection and postoperative adjuvant therapy. We present this article in accordance with the TRIPOD reporting checklist (available at https://qims.amegroups.com/article/view/10.21037/qims-24-618/rc).
Methods
Study population
This retrospective study was approved by the Ethics Committee of the Second Affiliated Hospital of Shantou University Medical College (No. 2023-23). The requirement for informed consent was waived due to the retrospective nature of the study. The study was conducted in accordance with the Declaration of Helsinki (as revised in 2013).
Patients with PTC confirmed by surgery and histopathology combined with CLND were retrospectively evaluated from the institutional database of The Second Affiliated Hospital of Shantou University Medical College from January 2019 to September 2023. All the data available in the database were utilized to optimize the statistical power and enhance the generalizability of the findings. The inclusion criteria were as follows: (I) PTC was first pathologically diagnosed; (II) all patients underwent lobectomy or total thyroidectomy and CLND; (III) CLNM status was confirmed by postoperative pathology; and (IV) ultrasound examination was performed in the ultrasound department within one month prior to surgery. The exclusion criteria were as follows: (I) the presence of other thyroid malignancies; (II) the occurrence of distant metastases or other malignant tumors; (III) a history of thyroid cancer or other head and neck surgeries; and (IV) incomplete ultrasonic image information or poor image quality. The process of patient enrollment is illustrated in Figure 1.
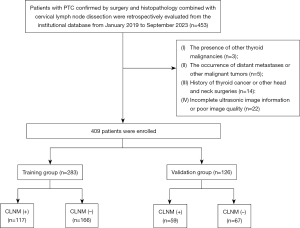
The patient recruitment process is shown in Figure 1. Overall, 409 patients were enrolled and divided into training and validation groups according to chronological order at a ratio of 7:3. A total of 283 patients treated between January 2019 and July 2022 composed the training group, while 126 patients treated between August 2022 and September 2023 composed the validation group. A CLNM (+) and CLNM (−) group was formed based on the pathological results of the patients.
Instruments and methods
Preoperative ultrasound was performed on the thyroid nodules and cervical lymph nodes of all patients using a high-resolution ultrasound system (LOGIQ E9, GE Healthcare, Chicago, IL, USA) with a 6 to 12 MHz high-frequency linear probe. The ultrasound features were independently evaluated by two experienced radiologists with more than 10 years of thyroid diagnostic experience. If there was disagreement, the final decision was made by consensus. Both radiologists were unaware of the patients’ information and pathological outcomes.
The following features were collected: sex; age; ultrasound features, including multifocality, bilaterality, tumor size, location, composition, echogenicity, aspect ratio, shape, margin, boundary, calcification, and capsule contact; Hashimoto’s thyroiditis (HT); ultrasound-based CLNM status; ACR scores; and C scores. Multifocality was defined as having more than one suspected malignant tumor in the thyroid, with the largest tumor being evaluated. Bilaterality was defined as suspected malignant tumors in both lobes of the thyroid. Tumor size was defined as the maximum diameter (D) of the tumor, which was categorized as either D ≤1 cm or D >1 cm. The location was classified as upper, middle, lower or isthmus. The composition was categorized as cystic or spongiform, mixed cystic and solid, or solid. Echogenicity was classified as anechoic, hyperechoic or isoechoic, hypoechoic, or very hypoechoic. The aspect ratio was defined as the height divided by the width of the transverse section, divided into <1 or >1. Shape and margin were classified as regular or irregular, while boundary was classified as smooth or ambiguous. Calcifications were classified as none, macro-calcifications, peripheral calcifications or micro-calcifications. Capsular contact was defined as the proportion of the tumor contacting the thyroid capsule exceeding 20% or the absence of the echo of the thyroid capsule shown by ultrasound. Ultrasound-based CLNM was considered suspicious in patients with one of the following characteristics: hyperechogenicity, round shape or necrosis, loss of the fatty hilum, or micro-calcifications. HT diagnosis was based on the pathological outcome. Using the ACR TI-RADS and C TI-RADS, the tumor scores were calculated, and a receiver operating characteristic (ROC) curve was used to calculate the best cutoff point as the basis for grouping.
The pathological results of the status of CLNM were provided by the professional pathology department. All pathological examinations were independently evaluated by two experienced pathologists, and final decisions are made through consensus in case of disagreement. These two physicians were not privy to the patient’s information or ultrasound examination results.
The ultrasound features and pathological results collected in both the training group and validation group adhered to consistent standards.
Statistical analysis
Statistical analyses were conducted with R software (version 4.2.2). Quantitative data were expressed as the mean ± standard deviation, and a t-test was used for comparisons. Categorical data were presented as frequencies and percentages (%), and the Chi-squared test was used for comparisons. A two-sided P<0.05 was considered to indicate statistical significance.
To avoid the influence of confounding factors, the features in the training group were selected by least absolute shrinkage and selection operator (LASSO) regression with penalty parameter adjustment by 10-fold cross-validation. The potential features with nonzero coefficients were included in multivariate logistic regression analysis to calculate the odds ratio (OR) for identifying independent risk factors for CLNM. All the independent risk factors were incorporated into the prediction model to construct a nomogram.
A ROC curve and area under the ROC curve (AUC) were used to estimate the performance of the prediction model. The Delong test was used to compare the ROC curve and AUC. The calibration curve and Hosmer-Lemeshow test were used to evaluate the goodness of fit of the model. Decision curve analysis (DCA) and clinical impact curve analysis were performed to evaluate the clinical usefulness of the prediction model.
Results
Patient characteristics
The study included a total of 409 patients with PTC, comprising 65 male and 344 female, with a mean age of 45.68±12.93 years. Table 1 summarizes the clinical and ultrasound features of all patients. There was no difference in features between the two groups except for margin and echogenicity (P>0.05). The incidence of CLNM in the training and validation groups was 41.3% (117/283) and 46.8% (59/126), respectively, with no significant difference between the two groups (P=0.3546).
Table 1
Variables | Subgroups | Training group (N=283) | Validation group(N=126) | P value |
---|---|---|---|---|
CLNM | (−) | 166 (58.7) | 67 (53.2) | 0.3546 |
(+) | 117 (41.3) | 59 (46.8) | ||
Sex | Male | 45 (15.9) | 20 (15.9) | 1 |
Female | 238 (84.1) | 106 (84.1) | ||
Age (years) | – | 45.69±12.89 | 45.67±13.01 | 0.9892 |
Location | Upper | 65 (23.0) | 36 (28.6) | 0.6438 |
Middle | 138 (48.8) | 55 (43.7) | ||
Lower | 64 (22.6) | 28 (22.2) | ||
Isthmus | 16 (5.7) | 7 (5.6) | ||
Tumor size | ≤1 cm | 146 (51.6) | 70 (55.6) | 0.5258 |
>1 cm | 137 (48.4) | 56 (44.4) | ||
Multifocality | No | 220 (77.7) | 102 (81.0) | 0.5469 |
Yes | 63 (22.3) | 24 (19.0) | ||
Bilaterality | No | 239 (84.5) | 110 (87.3) | 0.5481 |
Yes | 44 (15.5) | 16 (12.7) | ||
Capsule contact | No | 144 (50.9) | 57 (45.2) | 0.3435 |
Yes | 139 (49.1) | 69 (54.8) | ||
Composition | Mixed cystic and solid | 7 (2.5) | 7 (5.6) | 0.1135 |
Solid | 276 (97.5) | 119 (94.4) | ||
Echogenicity | Isoechoic | 19 (6.7) | 3 (2.4) | 0.002717 |
Hypoechoic | 224 (79.2) | 117 (92.9) | ||
Very hypoechoic | 40 (14.1) | 6 (4.8) | ||
Aspect ratio | ≤1 | 159 (56.2) | 60 (47.6) | 0.1346 |
>1 | 124 (43.8) | 66 (52.4) | ||
Margin | Regular | 180 (63.6) | 64 (50.8) | 0.01986 |
Irregular | 103 (36.4) | 62 (49.2) | ||
Macro-calcifications | No | 226 (79.9) | 90 (71.4) | 0.08008 |
Yes | 57 (20.1) | 36 (28.6) | ||
Peripheral calcifications | No | 275 (97.2) | 121 (96.0) | 0.5435 |
Yes | 8 (2.8) | 5 (4.0) | ||
Micro-calcifications | No | 100 (35.3) | 52 (41.3) | 0.3003 |
Yes | 183 (64.7) | 74 (58.7) | ||
Shape | Regular | 169 (59.7) | 73 (57.9) | 0.8186 |
Irregular | 114 (40.3) | 53 (42.1) | ||
Boundary | Smooth | 198 (70.0) | 76 (60.3) | 0.07159 |
Ambiguous | 85 (30.0) | 50 (39.7) | ||
ACR TI-RADS scores | <9 | 127 (44.9) | 54 (42.9) | 0.7858 |
≥9 | 156 (55.1) | 72 (57.1) | ||
C TI-RADS scores | <3 | 116 (41.0) | 58 (46.0) | 0.3987 |
≥3 | 167 (59.0) | 68 (54.0) | ||
US-based CLNM status | No | 244 (86.2) | 115 (91.3) | 0.2019 |
Yes | 39 (13.8) | 11 (8.7) | ||
Hashimoto’s thyroiditis | No | 200 (70.7) | 99 (78.6) | 0.1229 |
Yes | 83 (29.3) | 27 (21.4) |
Quantitative data are expressed as the mean ± standard deviation. Categorical data are presented as number (%). CLNM, central lymph node metastasis; ACR TI-RADS, American College of Radiology Thyroid Imaging Reporting and Data System; C TI-RADS, the Chinese version of the Thyroid Imaging Reporting and Data System; US, ultrasound; N, number.
Correlation between clinical and ultrasound features and CLNM
As shown in Table 2, significant differences were observed in sex, age, tumor size, multifocality, bilaterality, capsule contact, margin, micro-calcification, shape, boundary, ACR TI-RADS score, C TI-RADS score, and ultrasound-based CLNM status between the CLNM (+) and CLNM (−) in the training groups (P<0.05). ROC curve analysis indicated that 9 and 3 were the optimal cutoff values for the ACR TI-RADS score and C TI-RADS score, respectively.
Table 2
Characteristics | Subgroups | Training group (N=283) | Validation group (N=126) | |||||
---|---|---|---|---|---|---|---|---|
CLNM (−) (N=166) |
CLNM (+) (N=117) | P value | CLNM (−) (N=67) |
CLNM (+) (N=59) |
P value | |||
Sex | Male | 18 (10.8) | 27 (23.1) | 0.009 | 8 (11.9) | 12 (20.3) | 0.297 | |
Female | 148 (89.2) | 90 (76.9) | 59 (88.1) | 47 (79.7) | ||||
Age (years) | – | 48.02±12.41 | 42.38±12.88 | <0.001 | 48.22±12.85 | 42.76±12.66 | 0.018 | |
Location | Upper | 43 (25.9) | 22 (18.8) | 0.108 | 17 (25.4) | 19 (32.2) | 0.753 | |
Middle | 84 (50.6) | 54 (46.2) | 29 (43.3) | 26 (44.1) | ||||
Lower | 33 (19.9) | 31 (26.5) | 17 (25.4) | 11 (18.6) | ||||
Isthmus | 6 (3.6) | 10 (8.5) | 4 (6.0) | 3 (5.1) | ||||
Tumor size | ≤1 cm | 114 (68.7) | 32 (27.4) | <0.001 | 43 (64.2) | 27 (45.8) | 0.058 | |
>1 cm | 52 (31.3) | 85 (72.6) | 24 (35.8) | 32 (54.2) | ||||
Multifocality | No | 142 (85.5) | 78 (66.7) | <0.001 | 57 (85.1) | 45 (76.3) | 0.304 | |
Yes | 24 (14.5) | 39 (33.3) | 10 (14.9) | 14 (23.7) | ||||
Bilaterality | No | 152 (91.6) | 87 (74.4) | <0.001 | 60 (89.6) | 50 (84.7) | 0.589 | |
Yes | 14 (8.4) | 30 (25.6) | 7 (10.4) | 9 (15.3) | ||||
Capsule contact | No | 122 (73.5) | 22 (18.8) | <0.001 | 44 (65.7) | 13 (22.0) | <0.001 | |
Yes | 44 (26.5) | 95 (81.2) | 23 (34.3) | 46 (78.0) | ||||
Composition | Mixed cystic and solid | 5 (3.0) | 2 (1.7) | 0.759 | 3 (4.5) | 4 (6.8) | 0.862 | |
Solid | 161 (97.0) | 115 (98.3) | 64 (95.5) | 55 (93.2) | ||||
Echogenicity | Isoechoic | 12 (7.2) | 7 (6.0) | 0.163 | 2 (3.0) | 1 (1.7) | 0.552 | |
Hypoechoic | 136 (81.9) | 88 (75.2) | 63 (94.0) | 54 (91.5) | ||||
Very hypoechoic | 18 (10.8) | 22 (18.8) | 2 (3.0) | 4 (6.8) | ||||
Aspect ratio | ≤1 | 88 (53.0) | 71 (60.7) | 0.246 | 35 (52.2) | 25 (42.4) | 0.354 | |
>1 | 78 (47.0) | 46 (39.3) | 32 (47.8) | 34 (57.6) | ||||
Margin | Regular | 142 (85.5) | 38 (32.5) | <0.001 | 50 (74.6) | 14 (23.7) | <0.001 | |
Irregular | 24 (14.5) | 79 (67.5) | 17 (25.4) | 45 (76.3) | ||||
Macro-calcifications | No | 133 (80.1) | 93 (79.5) | 1 | 53 (79.1) | 37 (62.7) | 0.067 | |
Yes | 33 (19.9) | 24 (20.5) | 14 (20.9) | 22 (37.3) | ||||
Peripheral calcifications | No | 162 (97.6) | 113 (96.6) | 0.888 | 64 (95.5) | 57 (96.6) | 1 | |
Yes | 4 (2.4) | 4 (3.4) | 3 (4.5) | 2 (3.4) | ||||
Micro-calcifications | No | 80 (48.2) | 20 (17.1) | <0.001 | 39 (58.2) | 13 (22.0) | <0.001 | |
Yes | 86 (51.8) | 97 (82.9) | 28 (41.8) | 46 (78.0) | ||||
Shape | Regular | 124 (74.7) | 45 (38.5) | <0.001 | 50 (74.6) | 23 (39.0) | <0.001 | |
Irregular | 42 (25.3) | 72 (61.5) | 17 (25.4) | 36 (61.0) | ||||
Boundary | Smooth | 144 (86.7) | 54 (46.2) | <0.001 | 58 (86.6) | 18 (30.5) | <0.001 | |
Ambiguous | 22 (13.3) | 63 (53.8) | 9 (13.4) | 41 (69.5) | ||||
ACR TI-RADS scores | <9 | 101 (60.8) | 26 (22.2) | <0.001 | 40 (59.7) | 14 (23.7) | <0.001 | |
≥9 | 65 (39.2) | 91 (77.8) | 27 (40.3) | 45 (76.3) | ||||
C TI-RADS scores | <3 | 92 (55.4) | 24 (20.5) | <0.001 | 42 (62.7) | 16 (27.1) | <0.001 | |
≥3 | 74 (44.6) | 93 (79.5) | 25 (37.3) | 43 (72.9) | ||||
US-based CLNM status | No | 162 (97.6) | 84 (71.8) | <0.001 | 67 (100.0) | 48 (81.4) | 0.001 | |
Yes | 4 (2.4) | 33 (28.2) | 0 (0.0) | 11 (18.6) | ||||
Hashimoto’s thyroiditis | No | 117 (70.5) | 83 (70.9) | 1 | 51 (76.1) | 48 (81.4) | 0.619 | |
Yes | 49 (29.5) | 34 (29.1) | 16 (23.9) | 11 (18.6) |
Quantitative data are expressed as the mean ± standard deviation. Categorical data are presented as number (%). CLNM, central lymph node metastasis; ACR TI-RADS, American College of Radiology Thyroid Imaging Reporting and Data System; C TI-RADS, the Chinese version of the Thyroid Imaging Reporting and Data System; US, ultrasound; N, number.
Feature selection and model construction
To avoid the influence of confounding factors, nine nonzero factors, including sex, age, tumor size, multifocality, capsule contact, margin, micro-calcification, boundary and ultrasound-based CLNM status, were selected using LASSO regression analysis (Figure 2). These factors were included in the multivariate logistic regression analysis, and the results revealed that sex, age, multifocality, capsule contact, margin, micro-calcification and ultrasound-based CLNM status were independent risk factors for CLNM (Table 3). Based on the above independent risk factors, a nomogram prediction model was developed (Figures 3,4). The prediction model is presented in the form of a dynamic nomogram (https://predictionmodel2.shinyapps.io/CLNMprediction/) (Figure 5). By inputting the clinical and ultrasound features of the patient into the aforementioned website, one can obtain the probability and 95% confidence interval (CI) of CLNM occurrence.
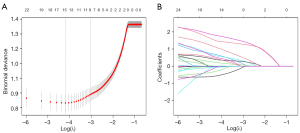
Table 3
Risk factors | OR | 95% CI | P value |
---|---|---|---|
Sex | 0.37 | 0.14–0.92 | 0.036 |
Age (years) | 0.97 | 0.94–1.00 | 0.045 |
Multifocality | 2.48 | 1.14–5.51 | 0.023 |
Capsule contact | 8.3 | 4.19–17.16 | <0.001 |
Margin | 5.79 | 2.83–12.22 | <0.001 |
Micro-calcifications | 2.33 | 1.09–5.11 | 0.031 |
US-based CLNM status | 4.34 | 1.32–17.80 | 0.024 |
CLNM, central lymph node metastasis; OR, odds ratio; CI, confidence interval; US, ultrasound.
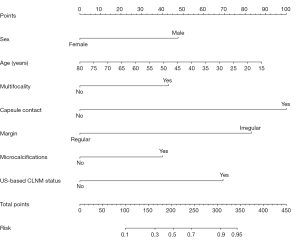
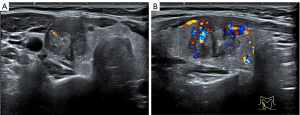
Prediction model validation
ROC curves of the prediction model were generated for the training and validation groups. The model showed good discriminability, with an AUC of 0.905 (95% CI: 0.870–0.940) in the training group and 0.865 (95% CI: 0.799–0.932) in the validation group. In addition, the AUCs of the single ultrasound features for predicting CLNM were 0.629 (95% CI: 0.586–0.672) in the training group and 0.593 (95% CI: 0.543–0.643) in the validation group, both of which were smaller than those of the prediction model (P<0.001) (Figure 6).
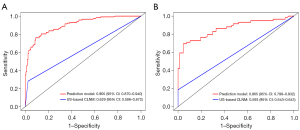
Based on the calibration curve (Figure 7) and Hosmer-Lemeshow test, the prediction model was found to have good concordance in both the training group (P=0.6259) and validation group (P=0.1182).
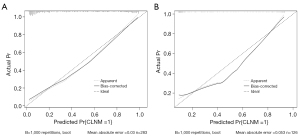
The DCA demonstrated that the prediction model had greater clinical benefit than either the treat-all-patients scheme or the treat-none scheme in both the training group and validation group when the threshold probability was between 15% and 90% (Figure 8).
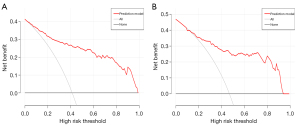
The clinical impact curves of the training group and validation group showed that when the threshold probability was ≤80%, the patients classified as positive by the prediction model were more likely to be truly positive (Figure 9).
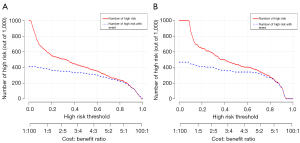
Discussion
According to the 2015 American Thyroid Association Management Guidelines for Adult Patients with Thyroid Nodules and Differentiated Thyroid Cancer, CLNM is as an important indicator of risk stratification and intimately associated with the risks of postoperative recurrence and distant metastasis (18). The guidelines recommend performing CLND for patients with high-risk PTC, while prophylactic CLND is suggested for those with low-risk PTC to accurately determine postoperative staging and to guide subsequent treatment decisions (18). However, some studies have shown that prophylactic CLND does not significantly improve long-term outcomes for patients but rather increases the potential risks of surgical complications such as recurrent laryngeal nerve injury and hypoparathyroidism (10). Accurate preoperative assessment of CLNM is crucial. Ultrasound is the preferred method for the preoperative assessment of PTC, but has limited sensitivity to detect CLNM due to the anatomical location of cervical lymph nodes (19). To achieve a reliable and noninvasive preoperative assessment of CLNM risk in PTC patients, our study developed and validated a prediction model that combines clinical and ultrasound characteristics. The model is presented as a dynamic nomogram, benefiting PTC patients by quantifying the likelihood of CLNM.
The present study indicated that sex, age, multifocality, capsule contact, margin, micro-calcification, and ultrasound-based CLNM status were independent predictors of CLNM.
PTC incidence is significantly greater in females than in males, with a female-to-male ratio of approximately 3.7:1 (20). However, an observational cohort study by Zhu et al. (21) showed that male sex in PTC patients was significantly associated with the risk of CLNM. The results of this study are consistent with the aforementioned conclusion, but to date, no research has provided a reasonable explanation for this phenomenon.
In the studies conducted by Zheng et al. (22) and Liu et al. (23), age was identified as an independent risk factor for CLNM, which aligns with our findings. Specifically, a younger age was associated with a heightened susceptibility to CLNM. This could be attributed to the fact that young age is correlated with a greater probability of experiencing multiple genetic abnormalities, that younger patients are more susceptible to encountering multiple genetic events, or that the early onset and disease progression are influenced by multiple genetic events (24).
This study indicated that multifocality plays a significant role as a risk factor for CLNM in PTC patients, which is in agreement with Liu et al. (23) and Mao et al. (25). This association can be attributed to the extensive lymphatic network within the thyroid, where multifocality may arise from tumor cells disseminating through lymphatic vessels within the gland (26). As a result, multifocal PTC often exhibits heightened invasiveness.
In our study, the probability of CLNM was high in PTC nodules that were in contact with the capsule, with an OR of 8.71 (95% CI: 4.39–18.10). Kamaya et al. suggested that ultrasonography revealed nodule contact with the capsule, contour bulging, and the absence of a thyroid capsule echo, which has good predictive value for detecting extracapsular extension (27). Xue et al. quantified the invasion of the capsule based on the contact proportion of the nodule with its perimeter, and the results showed that an adjacent capsule contact range exceeding 50% was an independent risk factor for CLNM (28). A higher degree of nodular contact with the capsule indicates a greater likelihood of tumor invasion. CLNM occurs when a tumor invades surrounding tissue through an interrupted capsule.
The findings of this study also demonstrate the significance of irregular margins in predicting CLNM, consistent with the findings of Li et al. (29). The process of metastasis requires cancer cells to invade the extracellular matrix, migrate through blood vessels and proliferate within lymph nodes. This aggressive behavior can result in the disruption of distinct demarcations between the tumor and adjacent normal tissue (15).
Micro-calcification involves the deposition of calcium salts resulting from vascular and fibrous hyperplasia, reflecting the rapid proliferation of cancer cells and represents a characteristic sign of PTC on ultrasound (13,30). This study suggested that micro-calcifications independently contribute to the risk of CLNM, consistent with previous research findings (31). Some cytokines, such as osteopontin (OPN), are involved in calcification, and the overexpression of OPN has been found to stimulate cell proliferation, migration, motility, and invasion in thyroid cancer cells, which is closely associated with the aggressive nature of PTC (32).
In our study, there were significant differences in the ACR TI-RADS and C TI-RADS scores between the CLNM (+) and CLNM (−) subgroups of patients with PTC. However, these scores did not emerge as independent risk factors for CLNM. This may be attributed to the fact that some scoring details, such as the composition, echogenicity and aspect ratio, of the ACR TI-RADS and C TI-RADS scores did not significantly differ between the CLNM (+) and CLNM (−) subgroups and did not have a predictive effect on CLNM.
Due to the intricate anatomical location of CLNM and differences in physicians’ examination techniques, the preoperative detection sensitivity of CLNM in PTC patients is limited (19). In this study, relying solely on ultrasound diagnosis for CLNM yielded an AUC of 0.629 (95% CI: 0.586–0.672) in the training group and 0.593 (95% CI: 0.543–0.643) in the validation group. However, the prediction model constructed using seven independent risk factors, namely, sex, age, multifocality, capsule contact, margin, micro-calcification, and ultrasound-based CLNM status, achieved superior performance, with an AUC of 0.905 (95% CI: 0.870–0.940) in the training group and 0.865 (95% CI: 0.799–0.932) in the validation group. Moreover, based on the calibration curve and Hosmer-Lemeshow test, the prediction model was found to have good concordance in both the training group (P=0.6259) and validation group (P=0.1182). The DCA and clinical impact curves illustrate the model’s significant clinical utility value. Recently, there have been studies conducted on the predictive model for CLNM in PTC patients (33). He et al. (34) developed a CLNM prediction model based on contrast-enhanced ultrasound score, achieving AUC values of 0.72 and 0.79 in the training and validation group, respectively. The model utilizing contrast-enhanced ultrasound is valuable for predicting CLNM, but its invasive nature and challenges in application limit its use in local hospitals. Wang et al. (35) developed a convolutional neural network prediction model using an artificial intelligence (AI) deep learning algorithm, achieving AUCs of 0.89 in the training set and 0.78 in the test set, indicating high predictive efficacy for CLNM. A meta-analysis of 107 studies involving 136,245 patients also demonstrated the outstanding performance of various machine learning-based predictive CLNM models, indicating their wide-ranging potential applications (36). However, the complexity of AI-based models, which require extensive image extraction and substantial technical support, similarly restricts their utilization in local hospitals. Furthermore, Zhao et al. (37) had shown that the quantitative parameters of dual-energy computed tomography (CT) and the morphology of PTC can be effective tools for predicting occult central cervical lymph node metastasis, with the model achieving an AUC index of 0.83. However, dual-energy CT is associated with higher costs, increased radiation exposure, and potential delays in radioactive iodine treatment for patients in need of adjuvant therapy (38). The predictive model was presented in the form of a dynamic nomogram and included seven noninvasive and easily obtainable clinical and ultrasound variables. It can be used by clinicians to visually and quickly assess CLNM risk and aids in the individualized treatment decision-making process.
There are several limitations in this study. First, the study was a retrospective study conducted at a single center, potentially introducing selection bias. This design, based on existing data and commonly used for association analysis, struggled to control for confounding factors, thus impacting the reliability and applicability of the model in new populations. Additionally, retrospective validation did not assess the practical application of the model in clinical settings, potentially leading to overfitting and instability when applied to independent prospective datasets. Second, the sample size was relatively limited. Third, for multifocal tumors, only the characteristics of the largest tumor were analyzed, while details about other tumors remain unknown. Finally, the criteria used to evaluate ultrasound features were subjective and may introduce errors when conducted by inexperienced radiologists. Nevertheless, in our study, the ultrasound features were evaluated by two radiologists with more than 10 years of experience in thyroid diagnosis, and a consensus was reached on each feature to minimize subjective errors. In future studies, it is imperative to conduct multicenter large-scale prospective studies to externally validate the nomogram and separately analyze solitary and multifocal tumors to derive more definitive conclusions. The nomogram model can also be improved by combining with more advanced technical parameters such as AI-assisted diagnostic systems.
Conclusions
In conclusion, this study indicated that sex, age, multifocality, capsule contact, margin, micro-calcification, and ultrasound-based CLNM status were independent predictors of CLNM. Dynamic nomograms developed using clinical and ultrasound characteristics show great predictive ability for CLNM risk in PTC patients, thereby providing valuable support to clinicians for making personalized treatment decisions.
Acknowledgments
Funding: This study was supported by
Footnote
Reporting Checklist: The authors have completed the TRIPOD reporting checklist. Available at https://qims.amegroups.com/article/view/10.21037/qims-24-618/rc
Conflicts of Interest: All authors have completed the ICMJE uniform disclosure form (available at https://qims.amegroups.com/article/view/10.21037/qims-24-618/coif). The authors have no conflicts of interest to declare.
Ethical Statement: The authors are accountable for all aspects of the work in ensuring that questions related to the accuracy or integrity of any part of the work are appropriately investigated and resolved. This retrospective study was approved by the Ethics Committee of the Second Affiliated Hospital of Shantou University Medical College (No. 2023-23). The requirement for informed consent was waived due to the retrospective nature of the study. The study was conducted in accordance with the Declaration of Helsinki (as revised in 2013).
Open Access Statement: This is an Open Access article distributed in accordance with the Creative Commons Attribution-NonCommercial-NoDerivs 4.0 International License (CC BY-NC-ND 4.0), which permits the non-commercial replication and distribution of the article with the strict proviso that no changes or edits are made and the original work is properly cited (including links to both the formal publication through the relevant DOI and the license). See: https://creativecommons.org/licenses/by-nc-nd/4.0/.
References
- Gong Y, Jiang Q, Zhai M, Tang T, Liu S. Thyroid cancer trends in China and its comparative analysis with G20 countries: Projections for 2020-2040. J Glob Health 2024;14:04131. [Crossref] [PubMed]
- Cheng F, Xiao J, Shao C, Huang F, Wang L, Ju Y, Jia H. Burden of Thyroid Cancer From 1990 to 2019 and Projections of Incidence and Mortality Until 2039 in China: Findings From Global Burden of Disease Study. Front Endocrinol (Lausanne) 2021;12:738213. [Crossref] [PubMed]
- Huang J, Ngai CH, Deng Y, Pun CN, Lok V, Zhang L, Xu Q, Lucero-Prisno DE, Xu W, Zheng ZJ, Elcarte E, Withers M, Wong MCSNCD Research Group, Global Health, Association of Pacific Rim Universities (APRU). Incidence and mortality of thyroid cancer in 50 countries: a joinpoint regression analysis of global trends. Endocrine 2023;80:355-65. [Crossref] [PubMed]
- Houten PV, Netea-Maier RT, Smit JW. Differentiated thyroid carcinoma: An update. Best Pract Res Clin Endocrinol Metab 2023;37:101687. [Crossref] [PubMed]
- Wang Y, Nie F, Wang G, Liu T, Dong T, Sun Y. Value of Combining Clinical Factors, Conventional Ultrasound, and Contrast-Enhanced Ultrasound Features in Preoperative Prediction of Central Lymph Node Metastases of Different Sized Papillary Thyroid Carcinomas. Cancer Manag Res 2021;13:3403-15. [Crossref] [PubMed]
- Zheng CM, Ji YB, Song CM, Ge MH, Tae K. Number of Metastatic Lymph Nodes and Ratio of Metastatic Lymph Nodes to Total Number of Retrieved Lymph Nodes Are Risk Factors for Recurrence in Patients With Clinically Node Negative Papillary Thyroid Carcinoma. Clin Exp Otorhinolaryngol 2018;11:58-64. [Crossref] [PubMed]
- Taniuchi M, Kawata R, Terada T, Higashino M, Aihara T, Jinnin T. Central node dissection from the perspective of lateral neck node metastasis in papillary thyroid carcinoma. Auris Nasus Larynx 2024;51:266-70. [Crossref] [PubMed]
- Zhang D, Wu J, Chen L. Surgical management of papillary thyroid carcinoma coexisting with Hashimoto's disease: a single-center retrospective cohort study. Front Endocrinol (Lausanne) 2024;15:1383945. [Crossref] [PubMed]
- Shen Y, Li X, Tao L, Chen Y, Xie R. Clinical Efficacy of Intraoperative Ultrasound for Prophylactic Lymphadenectomy of the Lateral Cervical Neck in Stage CN0 Papillary Thyroid Cancer: A Prospective Study. J Invest Surg 2023;36:2154416. [Crossref] [PubMed]
- Yang J, Han Y, Min Y, Chen C, Chen J, Xiang K, Liao J, Feng Y, Hu D, Yin G. Prophylactic central neck dissection for cN0 papillary thyroid carcinoma: is there any difference between western countries and China? A systematic review and meta-analysis. Front Endocrinol (Lausanne) 2023;14:1176512. [Crossref] [PubMed]
- Zhao H, Li H. Meta-analysis of ultrasound for cervical lymph nodes in papillary thyroid cancer: Diagnosis of central and lateral compartment nodal metastases. Eur J Radiol 2019;112:14-21. [Crossref] [PubMed]
- Biffoni M, Grani G, Melcarne R, Geronzi V, Consorti F, Ruggieri G, Galvano A, Razlighi MH, Iannuzzi E, Engel TD, Pace D, Di Gioia CRT, Boniardi M, Durante C, Giacomelli L. Drawing as a Way of Knowing: How a Mapping Model Assists Preoperative Evaluation of Patients with Thyroid Carcinoma. J Clin Med 2024;13:1389. [Crossref] [PubMed]
- Gao X, Luo W, He L, Cheng J, Yang L. Predictors and a Prediction Model for Central Cervical Lymph Node Metastasis in Papillary Thyroid Carcinoma (cN0). Front Endocrinol (Lausanne) 2021;12:789310. [Crossref] [PubMed]
- Wan F, He W, Zhang W, Zhang H, Zhang Y, Guang Y. Application of decision tree algorithms to predict central lymph node metastasis in well-differentiated papillary thyroid carcinoma based on multimodal ultrasound parameters: a retrospective study. Quant Imaging Med Surg 2023;13:2081-97. [Crossref] [PubMed]
- Hu Q, Zhang WJ, Liang L, Li LL, Yin W, Su QL, Lin FF. Establishing a Predictive Nomogram for Cervical Lymph Node Metastasis in Patients With Papillary Thyroid Carcinoma. Front Oncol 2021;11:766650. [Crossref] [PubMed]
- Tessler FN, Middleton WD, Grant EG, Hoang JK, Berland LL, Teefey SA, Cronan JJ, Beland MD, Desser TS, Frates MC, Hammers LW, Hamper UM, Langer JE, Reading CC, Scoutt LM, Stavros AT. ACR Thyroid Imaging, Reporting and Data System (TI-RADS): White Paper of the ACR TI-RADS Committee. J Am Coll Radiol 2017;14:587-95. [Crossref] [PubMed]
- Zhou J, Yin L, Wei X, Zhang S, Song Y, Luo B, et al. 2020 Chinese guidelines for ultrasound malignancy risk stratification of thyroid nodules: the C-TIRADS. Endocrine 2020;70:256-79. [Crossref] [PubMed]
- Haugen BR, Alexander EK, Bible KC, Doherty GM, Mandel SJ, Nikiforov YE, Pacini F, Randolph GW, Sawka AM, Schlumberger M, Schuff KG, Sherman SI, Sosa JA, Steward DL, Tuttle RM, Wartofsky L. 2015 American Thyroid Association Management Guidelines for Adult Patients with Thyroid Nodules and Differentiated Thyroid Cancer: The American Thyroid Association Guidelines Task Force on Thyroid Nodules and Differentiated Thyroid Cancer. Thyroid 2016;26:1-133. [Crossref] [PubMed]
- Yao F, Yang Z, Li Y, Chen W, Wu T, Peng J, Jiao Z, Yang A. Real-World Evidence on the Sensitivity of Preoperative Ultrasound in Evaluating Central Lymph Node Metastasis of Papillary Thyroid Carcinoma. Front Endocrinol (Lausanne) 2022;13:865911. [Crossref] [PubMed]
- Feng JW, Hong LZ, Wang F, Wu WX, Hu J, Liu SY, Jiang Y, Ye J. A Nomogram Based on Clinical and Ultrasound Characteristics to Predict Central Lymph Node Metastasis of Papillary Thyroid Carcinoma. Front Endocrinol (Lausanne) 2021;12:666315. [Crossref] [PubMed]
- Zhu J, Huang R, Yu P, Ren H, Su X. Male Gender Is Associated with Lymph Node Metastasis but Not with Recurrence in Papillary Thyroid Carcinoma. Int J Endocrinol 2022;2022:3534783. [Crossref] [PubMed]
- Zheng X, Peng C, Gao M, Zhi J, Hou X, Zhao J, Wei X, Chi J, Li D, Qian B. Risk factors for cervical lymph node metastasis in papillary thyroid microcarcinoma: a study of 1,587 patients. Cancer Biol Med 2019;16:121-30. [Crossref] [PubMed]
- Liu W, Cheng R, Ma Y, Wang D, Su Y, Diao C, Zhang J, Qian J, Liu J. Establishment and validation of the scoring system for preoperative prediction of central lymph node metastasis in papillary thyroid carcinoma. Sci Rep 2018;8:6962. [Crossref] [PubMed]
- Shukla N, Osazuwa-Peters N, Megwalu UC. Association Between Age and Nodal Metastasis in Papillary Thyroid Carcinoma. Otolaryngol Head Neck Surg 2021;165:43-9. [Crossref] [PubMed]
- Mao J, Zhang Q, Zhang H, Zheng K, Wang R, Wang G. Risk Factors for Lymph Node Metastasis in Papillary Thyroid Carcinoma: A Systematic Review and Meta-Analysis. Front Endocrinol (Lausanne) 2020;11:265. [Crossref] [PubMed]
- Gui CY, Qiu SL, Peng ZH, Wang M. Clinical and pathologic predictors of central lymph node metastasis in papillary thyroid microcarcinoma: a retrospective cohort study. J Endocrinol Invest 2018;41:403-9. [Crossref] [PubMed]
- Kamaya A, Tahvildari AM, Patel BN, Willmann JK, Jeffrey RB, Desser TS. Sonographic Detection of Extracapsular Extension in Papillary Thyroid Cancer. J Ultrasound Med 2015;34:2225-30. [Crossref] [PubMed]
- Xue T, Liu C, Liu JJ, Hao YH, Shi YP, Zhang XX, Zhang YJ, Zhao YF, Liu LP. Analysis of the Relevance of the Ultrasonographic Features of Papillary Thyroid Carcinoma and Cervical Lymph Node Metastasis on Conventional and Contrast-Enhanced Ultrasonography. Front Oncol 2021;11:794399. [Crossref] [PubMed]
- Li J, Sun P, Huang T, Li L, He S, Ai X, Xiao H, Xue G. Preoperative prediction of central lymph node metastasis in cN0T1/T2 papillary thyroid carcinoma: A nomogram based on clinical and ultrasound characteristics. Eur J Surg Oncol 2022;48:1272-9. [Crossref] [PubMed]
- Ning CP, Ji QL, Fang SB, Wang HQ, Zhong YM, Niu HT. Distribution patterns of microcalcifications in suspected thyroid carcinoma: a classification method helpful for diagnosis. Eur Radiol 2018;28:2612-9. [Crossref] [PubMed]
- Liu J, Jia X, Gu Y, Chen X, Guan L, Yan J, Zhai H, Zhou N, Dong Y, Zhan W, Luo X, Zhou J. Thyroid Parenchyma Microcalcifications on Ultrasound for Predicting Lymph Node Metastasis in Papillary Thyroid Carcinoma: A Prospective Multicenter Study in China. Front Oncol 2021;11:609075. [Crossref] [PubMed]
- Ferreira LB, Lima RT, Bastos ACSDF, Silva AM, Tavares C, Pestana A, Rios E, Eloy C, Sobrinho-Simões M, Gimba ERP, Soares P. OPNa Overexpression Is Associated with Matrix Calcification in Thyroid Cancer Cell Lines. Int J Mol Sci 2018;19:2990. [Crossref] [PubMed]
- Zou Y, Shi Y, Bi H, Tan J, Guo Q, Qin Y, Lu X, Ma X, Yang S, Liu J. A nomogram for risk stratification of central cervical lymph node metastasis in patients with papillary thyroid carcinoma. Quant Imaging Med Surg 2024;14:5084-98. [Crossref] [PubMed]
- He L, Chen X, Hu J, Meng Y, Zhang Y, Chen W, Fan Y, Li T, Fang J. Score based on contrast-enhanced ultrasound predict central lymph node metastasis in papillary thyroid cancer. Front Endocrinol (Lausanne) 2024;15:1336787. [Crossref] [PubMed]
- Wang Z, Qu L, Chen Q, Zhou Y, Duan H, Li B, Weng Y, Su J, Yi W. Deep learning-based multifeature integration robustly predicts central lymph node metastasis in papillary thyroid cancer. BMC Cancer 2023;23:128. [Crossref] [PubMed]
- Liu F, Han F, Lu L, Chen Y, Guo Z, Yao J. Meta-analysis of prediction models for predicting lymph node metastasis in thyroid cancer. World J Surg Oncol 2024;22:278. [Crossref] [PubMed]
- Zhao W, Shen S, Ke T, Jiang J, Wang Y, Xie X, Hu X, Tang X, Han D, Chen J. Clinical value of dual-energy CT for predicting occult metastasis in central neck lymph nodes of papillary thyroid carcinoma. Eur Radiol 2024;34:16-25. [Crossref] [PubMed]
- Fu J, Liu J, Wang Z, Qian L. Predictive Values of Clinical Features and Multimodal Ultrasound for Central Lymph Node Metastases in Papillary Thyroid Carcinoma. Diagnostics (Basel) 2024;14:1770. [Crossref] [PubMed]