Predicting progression of cerebral small vessel disease: relevance of carotid perivascular fat density based on computed tomography angiography
Introduction
Cerebral small vessel disease (CSVD) is the most common pathological and neurological syndrome caused by cerebrovascular lesions, involving the small arteries, arterioles, capillaries, and venules (1). CSVD causes about 25% of ischemic strokes, most hemorrhagic strokes, and about 50% vascular dementia worldwide, imposing a massive public health burden (2). Characteristic magnetic resonance imaging (MRI) features of CSVD include white matter hyperintensities (WMH), lacunes, cerebral microbleeds (CMBs), enlarged perivascular spaces (EPVS), and cortical superficial siderosis (cSS) (3).
The progression of CSVD could lead to an increased risk of stroke and dementia (4). Thus, the identification of CSVD progression would be of great significance for managing and reducing the risk of cognitive impairment in patients. CSVD results from the interaction of several mechanisms including cerebral microangiopathy, endothelial cell dysfunction, neuroinflammation, and blood-brain barrier (BBB) breakdown (5). Many studies have found that inflammation is related to CSVD (6,7). Longitudinal studies have shown that elevated levels of systemic inflammatory markers at baseline were associated with future CSVD severity and progression (8,9). Nam et al. (10) demonstrated that higher levels of neutrophil/lymphocyte ratio were associated with increased WMH volume.
A study by Moss and Ramji indicated that pro-inflammatory cytokines are fundamental drivers of carotid atherosclerosis and associated with lesion progression (11). Although carotid atherosclerosis and CSVD represent distinct vascular conditions, they frequently coexist in patients presenting with cerebrovascular symptoms (12,13). Li et al. (14) reported that vulnerable carotid plaques coexisting with CSVDs have higher a probability to cause an acute ischemic stroke. Additionally, Conijn et al. (15) have proposed that CSVDs are risk factors for mortality and ischemic stroke in patients with atherosclerotic disease. Given the association between carotid artery lesions and CSVD, we hypothesized that the inflammatory state around the carotid artery may be linked to CSVD.
The variation in computed tomography (CT) attenuation values of perivascular fat density (PFD) derived from carotid computed tomography angiography (CTA) images can reflect changes in the composition of perivascular adipose tissue (PVAT) induced by underlying inflamed, vulnerable plaques (16). PVAT around carotid arteries without an intervening fascial barrier is a metabolically and immunologically active fat deposit, which is closely related to vascular inflammatory responses (17). The increased PFD levels are associated with various risk characteristics of carotid plaque (18,19). PFD can also serve as an imaging marker of cerebrovascular ischemic symptoms (20). Regarding its association with CSVD, Zhang et al. (21) demonstrated that elevated PFD correlates with higher WMH grades and the presence of lacunes. To our knowledge, few studies have assessed the association between PFD and CSVD in the absence of carotid stenosis.
Thus, this study aimed to investigate the possible association of the metabolically active pericarotid adipose tissue with the presence and the progression of CSVD, in patients without carotid stenosis. Additionally, to explore the potential diagnostic value of PVAT, this study developed and validated predictive models for CSVD progression based on clinical risk factors, PFD, and radiomic features of PVAT. We present this article in accordance with the TRIPOD reporting checklist (available at https://qims.amegroups.com/article/view/10.21037/qims-24-1512/rc).
Methods
Data
This retrospective study collected 263 patients that received carotid CTA, computed tomographic perfusion (CTP), and brain MRI from January 2019 to March 2024 at Zhejiang Provincial People’s Hospital (hospital I). The inclusion criteria were as follows: (I) carotid stenosis less than 30%, as assessed by the North American Symptomatic Carotid Endarterectomy Trial (NASCET) criteria (22); (II) age 50 years or above; (III) at least 3 months’ interval between two MRI and susceptibility-weighted imaging (SWI) examinations, and at most 15 days’ interval between CTA and the first MRI examination; (IV) MRI examinations including complete T1-weighted imaging (T1WI), T2-weighted imaging (T2WI), T2-fluid attenuated inversion recovery imaging (T2-FLAIR), and diffusion-weighted imaging (DWI). The exclusion criteria were as follows: (I) a history of carotid endarterectomy or stenting; (II) arterial lesions other than atherosclerotic disease (e.g., dissection, aneurysm); (III) other neurological symptoms (e.g., Alzheimer’s disease, multiple sclerosis, or major brain injuries); (IV) poor image quality of CTA and MRI; (V) duplicate cases in the cohort. Based on the above inclusion and exclusion criteria, 65 patients who received CTA and brain MRI at Hangzhou Traditional Chinese Medicine Hospital (hospital II) were collected for external validation of the models. Figure 1 displays the patient distribution and overall study design.
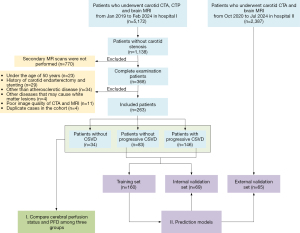
The study was conducted in accordance with the Declaration of Helsinki (as revised in 2013). The study was approved by the Ethics Committees of Zhejiang Provincial People’s Hospital (No. QT2024163) and Hangzhou Traditional Chinese Medicine Hospital (No. 202412100953000019995), and the requirement for informed consent was waived due to the retrospective nature of the study.
Demographic and clinical characteristics
Demographic and clinical data were obtained from medical records, including age, sex, body mass index (BMI), history of hypertension, systolic pressure, diastolic pressure, hyperlipidemia, diabetes, coronary heart disease, current stroke, previous stroke, smoking or drinking history, antihypertensive treatment, statin use, and antiplatelet use. For diabetic patients, glycosylated hemoglobin (HbA1c) levels were collected to categorize them into well-controlled (HbA1c <7%) and poorly controlled (HbA1c ≥7%) groups (23). Additionally, the levels of the following blood biomarkers were recorded: total cholesterol (TC), triglycerides (TG), high density lipoprotein cholesterol (HDL-C), low-density lipoprotein cholesterol (LDL-C), white blood cell count (WBC), and C-reactive protein (CRP).
Head and neck CTA and CTP image acquisition and analysis
CTA and CTP protocol
Head and neck CTA examinations were conducted using a dual-energy CT (DECT) scanner (Siemens, Erlangen, Germany) and 640-slice CT (Toshiba, Tokyo, Japan). The scanning parameters were as follows: tube voltage 100 kV, tube current 300 mA, pitch 1.0, layer thickness 0.5 mm, and matrix 512×512. The patient was instructed to fast before the examination, and the patient’s heart rate, height, and weight were measured. The scanning range was from the ascending aorta to the top of the skull. After the acquisition of the localization image, the nonionic isotonic contrast agent iodixanol (320 mg/mL) was injected into the right median vein at a rate of 5 mL/s (50 mL of contrast agent plus 30 mL of saline). The region of interest (ROI) was set at the aortic arch level, with a trigger threshold of 150 Hounsfield unit (HU), and the CTA scan was started using the contrast agent Intelligent tracking technology (Surstar, Ulrich GmbH & Co. KG, Ulm, Germany). Simultaneously, CTP images were acquired, the acquisition parameter was 128 mAs, 120 kV, the scanning width was 5 mm, and the scanning coverage was 240 mm.
Assessment of cerebral perfusion status on CTP
The CTP raw data were processed by commercial software MIStar (Apollo Medical Imaging Technology, Melbourne, Australia). A delay time (DT) >3 seconds indicated the presence of ischemic injury, with tissues exhibiting cerebral blood flow (CBF) ≤30% identified as the ischemic core (24). CTP results were assessed by two experienced radiologists who were blinded to clinical information. According to CTP images, perfusion results were categorized into perfusion normalcy and perfusion abnormality (Figure 2).
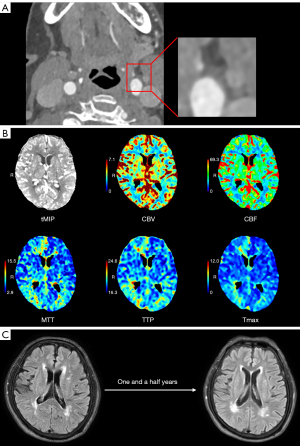
Quantification of pericarotid adipose tissue density
Assessment of the density of the perivascular fat surrounding the carotid artery was conducted by a dedicated software (Perivascular Fat Analysis Tool, Shukun Technology, Beijing, China) (19,25). All head and neck CTA images were first processed using an automated imaging reconstruction system for vascular segmentation and reconstruction (26). Perivascular adipose tissue was defined as the adipose tissue located within a radial distance from the outer vessel wall, equivalent to the luminal diameter of the artery (27) (Figures 2,3). Based on a previously employed pericoronary fat measurement approach, PFD was measured in a semi-automated manner by tracking the contour of the inner and outer vessel wall border (28). In cases with carotid plaque, the ROI was defined as all voxels in the −190 to −30 HU range surrounding the plaque (29,30). In cases without plaque, the ROI was selected in the same density range at the carotid bifurcation. All fat density measurements were reported in HU.
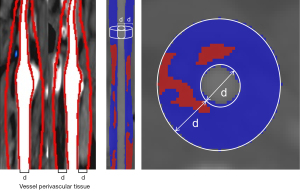
MRI image acquisition and analysis
MRI protocol
MRI examinations were performed on two 3.0-tesla MRI scanners (Siemens Trio3.0 T and GE Discovery MR 750, GE Healthcare, Chicago, IL, USA) using the same magnetic resonance (MR) parameters and eight-channel coils. The routine scanning parameters of MRI images were as follows: repetition time (TR) of 1,750 ms, echo time (TE) of 24 ms, slice thickness (ST) of 5.5 mm, and field of view (FOV) of 220 cm × 220 cm for T1WI; TR of 5,614 ms, TE of 102 ms, ST of 5.5 mm, and FOV of 220 cm × 220 cm for T2WI; TR of 9,000 ms, TE of 120 ms, ST of 5.5 mm, and FOV of 220 cm × 220 cm for T2-FLAIR; and TR of 3,047 ms, TE of 63 ms, ST of 5.5 mm, FOV of 220 cm × 220 cm, and b value of 0, 1,000 s/mm2 for DWI. In addition, SWI was used for CMB observation, with the following parameters: TR of 25 ms, TE of 25 ms, ST of 5.5 mm, and FOV of 240 cm × 240 cm.
Assessment of MRI markers of CSVD
All neuroimaging markers of CSVD were defined according to Standards for Reporting Vascular Changes on Neuroimaging 2 (STRIVE-2) consensus criteria (3). WMH was defined as hyperintense signal changes on T2-weighted (preferably FLAIR) MRI sequences (31). The severity of WMH in periventricular WMH (PWMH) and deep WMH (DWMH) was evaluated according to the Fazekas rating scale (32). The total WMH burden was calculated as the sum of PWMH and DWMH scores and ranged between 0 and 6 points. The volume of WMH was calculated using specialized software (uAI Discover CSVD, United Imaging, Shanghai, China) in milliliters. The measured WMH regions included PWMH and DWMH, with their volumes summed to calculate the total WMH volume. Lacune was defined as a round or ovoid, subcortical, cerebrospinal fluid-like cavity with a diameter of 3–15 mm, appearing hypointense on T1 imaging or hyperintense on FLAIR images (3). CMBs were defined as small, generally 2–5 mm diameter, low-signal lesions on SWI (3). EPVS were fluid-filled round, ovoid, or linear hyperintensities on T2 images (33). The total CSVD burden was rated on an ordinal scale from 0 to 6 according to the above MRI markers (34). The presence of the following manifestations was counted as one point: (I) ≥1 lacunes; (II) 1–4 CMBs; (III) >20 EPVS; (IV) moderate WMHs (PWMH + DWMH grade 3–4). Two points were awarded for the following presentations: (I) ≥5 CMBs; (II) severe WMHs (PWMH + DWMH grade 5–6) (34,35).
CSVD burden was assessed by two experienced radiologists who were blinded to clinical information. In case of discrepancy, a third senior radiologist was consulted to achieve consensus. CSVD scores and total WMH volumes of two MRIs were recorded by an experienced radiologist. Based on CSVD burden and total WMH volume, CSVD fluctuations were categorized into (I) progression and (II) non-progression. Progression of CSVD was defined as an increase in total score by more than 1 point or when the total scores were equal but the WMH volume increased by more than 0.25 mL (36,37), Figure 2 shows the progression of WMH; otherwise, it was considered non-progression. In addition, if the CSVD score was 0, it was considered without CSVD.
Radiomics feature extraction and selection
To minimize the center effect of CT images from different scanners and hospitals (38), all original CT images were appropriately pre-processed. All images were resampled to 1×1×1 mm3 (x, y, z) using a linear interpolation algorithm to standardize the voxel spacing (39). ROI segmentation of the PVAT around carotid plaque was conducted using the Perivascular Fat Analysis Tool. An experienced neuroradiologist, who was blinded to the clinical data, performed manual adjustments to ensure accurate delineation of the ROIs using ITK-SNAP software (www.itksnap.org). PyRadiomics (version 3.0.1) was applied to extract radiomics features of the PVAT segmentation, which conformed to the Image Biomarker Standardization Initiative guideline (40). The extracted features included shape, first-order, texture, wavelet, exponential, and square transform features (41).
ComBaTool and z-scores were then applied to normalize the features. ComBaTool, a free online application (https://forlhac.shinyapps.io/Shiny_ComBat/) (42), was used to pool features and reduce inter-scanner variability. Principal component analysis (PCA) was used to visualize the effects of Combat on feature harmonization (Figure S1). Next, all radiomic features were standardized using z-scores. An independent-samples t-test or Mann-Whitney U test was then applied for initial feature selection. Finally, Spearman correlation analysis and elastic-net logistic regression were used to filter out redundant features.
Development of the predictive models
Patients with CSVD without progression and with CSVD progression enrolled in hospital I were randomly divided into a training set and internal validation set according to the ratio of 7:3, and patients in hospital II were included in the external validation set.
Univariate logistic regression analysis was conducted to identify significant factors from potential predictors (including demographic and clinical characteristics, cerebral perfusion status, PFD, and radiomic features). The selected factors were then included in a multivariate analysis to determine the independent predictors of CSVD progression for model construction.
To evaluate the progression of CSVD, six different models were developed based on clinical risk factors, PFD, and PVAT radiomic signatures. Model A was a clinical model using only significant clinical factors, Model B used only PFD, and Model C used only radiomic signatures. Model D combined significant clinical factors and PFD, and Model E combined significant clinical factors and radiomic signatures. Model F was a hybrid model constructed using the XGBoost algorithm, integrating significant clinical factors, PFD, and radiomic signatures. The XGBoost algorithm, a supervised ensemble learning method, is recognized for its scalability, flexibility, and parallel processing, making it effective for optimizing model performance by combining multiple weak learners.
Statistical analysis
Statistical analysis was conducted using the software SPSS 25.0 (IBM Corp., Armonk, NY, USA) and MedCalc 19.3.1 (MedCalc Software, Ostend, Belgium). A P value <0.05 was considered statistically significant.
Continuous variables were expressed as mean ± standard deviation (SD), and categorical variables were presented as n (%). Kolmogorov-Smirnov was used to test the normality of measurement data. For continuous variable comparisons, the Student’s t-test (normal distributions) or Mann-Whitney test (nonnormal distributions) was used between two groups, whereas analysis of variance (ANOVA) test (normal distributions) or Kruskal-Wallis test (nonnormal distributions) was used among three groups. For categorical variable comparisons, chi-square or Fisher’s exact test was used. The Spearman test was used to assess the correlations between PFD value and neuroimaging markers of CSVD. The performance of different models was evaluated according to area under the receiver operating characteristic curve (AUC), accuracy, sensitivity, and specificity.
Results
Baseline characteristics of the patients
Of 368 patients who underwent both CTA and CTP and two MRIs in hospital I, 105 were excluded due to various reasons (see Figure 1). Consequently, 263 patients, including 34 without CSVD, 83 with CSVD without progression, and 146 with CSVD progression were enrolled in the study. Additionally, 65 patients from hospital II were enrolled for external validation, including 22 without CSVD progression and 43 with CSVD progression. The mean interval between the two MRI examinations was 608.05±367.21 days in hospital I and 578.32±262.63 days in hospital II. The interval between CTA/CTP and MRI examinations was 2.77±3.46 days in hospital I and 2.28±3.28 days in hospital II.
There were significant differences in age, coronary heart disease, TC, TG, and LDL-C between patients without and with CSVD (Table 1—in the table, the latter are shown subdivided in the two subgroups with and without progression). Instead, most clinical characteristics were not different between the “without CSVD progression” group and the “with CSVD progression” group for the training set, internal validation set, and external validation set (Table 2).
Table 1
Variable | All (n=263) | Without CSVD (n=34) | Without progression (n=83) | With progression (n=146) | P value |
---|---|---|---|---|---|
Age (years) | 67.00±10.81 | 61.97±8.07 | 67.07±9.76 | 68.14±10.55 | 0.006* |
Male | 147 (55.9) | 17 (50.0) | 50 (60.24) | 80 (54.79) | 0.552 |
BMI (kg/m2) | 24.17±3.35 | 24.82±3.06 | 23.76±3.14 | 24.25±3.52 | 0.276 |
Hypertension | 181 (68.82) | 23 (67.65) | 53 (63.86) | 105 (71.93) | 0.443 |
Systolic pressure (mmHg) | 140.98±20.10 | 137.82±19.13 | 140.35±20.20 | 142.07±20.31 | 0.511 |
Diastolic pressure (mmHg) | 80.44±12.95 | 78.32±10.86 | 81.16±14.89 | 80.52±12.23 | 0.560 |
Hyperlipidemia | 15 (5.70) | 4 (11.76) | 3 (3.61) | 8 (5.48) | 0.228 |
Diabetes | |||||
Non-diabetic | 196 (74.52) | 25 (73.53) | 68 (81.93) | 103 (70.55) | 0.385 |
Well-controlled diabetic | 35 (13.31) | 5 (14.71) | 9 (10.84) | 21 (14.38) | |
Poorly-controlled diabetic | 32 (12.17) | 4 (11.76) | 6 (7.23) | 22 (15.07) | |
Coronary heart disease | 15 (5.70) | 4 (11.76) | 7 (8.43) | 4 (2.74) | 0.031* |
Current stroke | 124 (47.15) | 13 (38.24) | 39 (46.99) | 72 (49.32) | 0.507 |
Previous stroke | 53 (20.15) | 2 (5.88) | 19 (22.89) | 32 (21.92) | 0.083 |
Current smoking | 59 (22.43) | 10 (29.41) | 19 (22.89) | 30 (20.55) | 0.533 |
Drinking | 48 (18.25) | 6 (17.65) | 16 (19.28) | 26 (17.81) | 0.958 |
Medication | |||||
Antihypertension use | 160 (60.84) | 20 (58.82) | 45 (54.22) | 95 (65.07) | 0.262 |
Statin use | 42 (15.97) | 4 (11.76) | 16 (19.28) | 22 (15.07) | 0.545 |
Antiplatelet use | 47 (17.87) | 5 (14.71) | 17 (20.48) | 25 (17.12) | 0.714 |
Serum biomarkers | |||||
TC (mmol/L) | 3.95±1.05 | 4.64±1.30 | 3.87±1.00 | 3.83±0.94 | <0.001* |
TG (mmol/L) | 1.46±0.98 | 1.78±1.07 | 1.57±1.14 | 1.33±0.82 | 0.025* |
HDL-C (mmol/L) | 1.07±0.30 | 1.10±0.26 | 1.08±0.31 | 1.05±0.30 | 0.612 |
LDL-C (mmol/L) | 2.18±0.82 | 2.67±1.01 | 2.09±0.78 | 2.11±0.75 | 0.001* |
WBC (×109/L) | 6.24±2.09 | 6.34±1.85 | 6.49±2.49 | 6.08±1.88 | 0.343 |
CRP (mg/L) | 5.74±13.01 | 5.81±12.07 | 7.09±18.68 | 4.95±8.59 | 0.491 |
Data are presented as mean ± SD or n (%). *, P<0.05. Hospital I, Zhejiang Provincial People’s Hospital. CSVD, cerebral small vessel disease; BMI, body mass index; TC, total cholesterol; TG, triglycerides; HDL-C, high-density lipoprotein cholesterol; LDL-C, low-density lipoprotein cholesterol; WBC, white blood cell count; CRP, C-reactive protein; SD, standard deviation.
Table 2
Variable | Training set | Internal validation set | External validation set | ||||||||
---|---|---|---|---|---|---|---|---|---|---|---|
Without progression (n=58) | With progression (n=102) | P value | Without progression (n=25) | With progression (n=44) | P value | Without progression (n=22) | With progression (n=43) | P value | |||
Age (years) | 67.55±9.90 | 68.58±10.46 | 0.666 | 65.96±9.54 | 67.11±10.81 | 0.698 | 70.18±10.44 | 72.33±7.90 | 0.358 | ||
Male | 34 (58.6) | 54 (52.9) | 0.597 | 16 (64.0) | 26 (59.1) | 0.885 | 10 (45.5) | 26 (60.5) | 0.374 | ||
BMI (kg/m2) | 23.38±2.87 | 24.38±1.02 | 0.077 | 24.66±3.60 | 23.95±3.19 | 0.402 | 24.12±3.38 | 23.02±3.71 | 0.248 | ||
Hypertension | 38 (65.5) | 74 (72.5) | 0.451 | 15 (60.0) | 31 (70.5) | 0.535 | 12 (54.5) | 27 (62.8) | 0.708 | ||
Systolic pressure (mmHg) | 138.09±21.49 | 143.07±20.63 | 0.150 | 145.60±16.01 | 139.75±19.59 | 0.208 | 134.45±18.25 | 145.26±21.32 | 0.047 | ||
Diastolic pressure (mmHg) | 81.24±14.74 | 81.28±12.47 | 0.984 | 80.96±15.55 | 78.75±11.60 | 0.504 | 75.77±15.27 | 77.37±11.84 | 0.643 | ||
Hyperlipidemia | 3 (5.2) | 5 (4.9) | >0.99 | 0 (0.0) | 3 (6.8) | 0.471 | 5 (22.7) | 7 (16.3) | 0.767 | ||
Diabetes | |||||||||||
Non-diabetic | 46 (79.3) | 69 (67.6) | 0.225 | 22 (88.0) | 34 (77.3) | 0.540 | 20 (90.9) | 27 (62.8) | 0.045* | ||
Well-controlled diabetic | 7 (12.1) | 15 (14.7) | 2 (8.0) | 6 (13.6) | 1 (4.5) | 3 (7.0) | |||||
Poorly-controlled diabetic | 5 (8.6) | 18 (17.6) | 1 (4.0) | 4 (9.1) | 1 (4.5) | 13 (30.2) | |||||
Coronary heart disease | 5 (8.6) | 3 (2.9) | 0.227 | 2 (8.0) | 1 (2.3) | 0.612 | 1 (4.5) | 5 (11.6) | 0.631 | ||
Current stroke | 24 (41.4) | 52 (51.0) | 0.242 | 15 (60.0) | 20 (45.5) | 0.245 | 7 (31.8) | 16 (37.2) | 0.667 | ||
Previous stroke | 15 (25.9) | 23 (22.5) | 0.779 | 4 (16.0) | 9 (20.5) | 0.893 | 6 (27.3) | 13 (30.2) | >0.99 | ||
Current smoking | 13 (22.4) | 19 (18.6) | 0.711 | 6 (24.0) | 11 (25.0) | >0.99 | 5 (22.7) | 11 (25.6) | >0.99 | ||
Drinking | 11 (19.0) | 16 (15.7) | 0.754 | 5 (20.0) | 10 (22.7) | >0.99 | 2 (9.1) | 6 (14.0) | 0.868 | ||
Antihypertension use | 33 (56.9) | 67 (65.7) | 0.350 | 12 (48.0) | 28 (63.6) | 0.312 | 10 (45.5) | 24 (55.8) | 0.597 | ||
Statin use | 10 (17.2) | 18 (17.6) | >0.99 | 6 (24.0) | 4 (9.1) | 0.182 | 6 (27.3) | 10 (23.3) | 0.959 | ||
Antiplatelet use | 10 (17.2) | 22 (21.6) | 0.651 | 7 (28.0) | 3 (6.8) | 0.041 | 5 (22.7) | 8 (18.6) | 0.948 | ||
TC (mmol/L) | 3.92±1.02 | 3.72±0.84 | 0.109 | 3.77±0.98 | 4.06±1.12 | 0.273 | 2.66±0.83 | 2.58±0.92 | 0.741 | ||
TG (mmol/L) | 1.58±1.06 | 1.36±0.90 | 0.137 | 1.53±1.37 | 1.25±0.60 | 0.446 | 4.26±1.17 | 3.99±1.15 | 0.377 | ||
HDL-C (mmol/L) | 1.14±0.31 | 1.05±0.30 | 0.043* | 0.96±0.25 | 1.07±0.31 | 0.132 | 1.05±0.45 | 1.24±0.84 | 0.642 | ||
LDL-C (mmol/L) | 2.08±0.78 | 2.02±0.66 | 0.807 | 2.10±0.82 | 2.33±0.88 | 0.306 | 1.21±0.66 | 1.00±0.19 | 0.142 | ||
WBC (×109/L) | 6.60±2.77 | 6.03±1.89 | 0.284 | 6.25±1.68 | 6.19±1.87 | 0.885 | 6.23±1.98 | 6.19±1.97 | 0.734 | ||
CRP (mg/L) | 7.22±18.77 | 4.83±8.58 | 0.156 | 6.79±18.86 | 5.25±8.70 | 0.949 | 2.21±3.59 | 4.62±11.6 | 0.249 |
Data are presented as mean ± SD or n (%). *, P<0.05. CSVD, cerebral small vessel disease; BMI, body mass index; TC, total cholesterol; TG, triglycerides; HDL-C, high-density lipoprotein cholesterol; LDL-C, low-density lipoprotein cholesterol; WBC, white blood cell count; CRP, C-reactive protein; SD, standard deviation.
Comparison of cerebral perfusion status among non-CSVD, non-CSVD progression, and CSVD progression groups
Among patients without CSVD, the majority exhibited normal perfusion (94.1%) compared to only 5.9% displaying perfusion abnormality, which was statistically different from the groups with CSVD (P<0.05). There was no statistical difference observed in perfusion status between patients with and without CSVD progression. Specifically, 28.8% of individuals with CSVD progression displayed perfusion abnormality, comparable to the 25.3% observed in individuals without CSVD progression (Table 3).
Table 3
Patient group | n | Perfusion normalcy, n (%) | Perfusion abnormality, n (%) | χ2 test | P value |
---|---|---|---|---|---|
Without CSVD | 34 | 32 (94.1) | 2 (5.9)*# | 7.785 | 0.020 |
Without progression | 83 | 62 (74.7) | 21 (25.3)^ | ||
With progression | 146 | 104 (71.2) | 42 (28.8) |
*, without CSVD vs. without CSVD progression, P<0.05; #, without CSVD vs. with CSVD progression, P<0.05; ^, without CSVD vs. with CSVD progression, P>0.05. CSVD, cerebral small vessel disease.
Correlation between PFD and CSVD
Using the Spearman correlation coefficient to evaluate the quantitative correlation between PFD value and imaging markers of CSVD, we found that PFD value was substantially associated with the presence of lacunes, CMB and WMH (P<0.05). Links were also observed between PFD value and the volume of PWMH and DWMH. However, there was no significant correlation between PFD value and EPVS. The specific results are displayed in Figure 4.
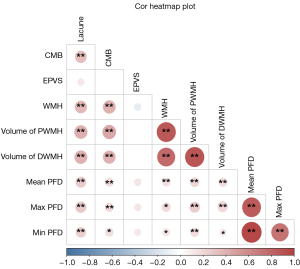
By comparing PFD values among patients without CSVD, without CSVD progression, and with CSVD progression, statistically significant differences were found in the maximum, minimum, and mean PFD values (P<0.001). The detailed results for association between PFD and CSVD are summarized in Figure 5 and Table S1.
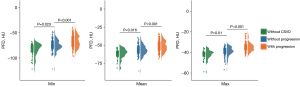
Performance of different prediction models
A total of 1,073 radiomics features were extracted from the ROI. For feature selection, 201 features were coarsely selected by an independent-samples t-test or Mann-Whitney U test. Subsequently, 185 redundant features were excluded using spearman correlation analysis. Finally, 10 predictive features were determined using elastic-net logistic regression analysis (Table S2 and Figure S2).
Univariate and multivariate logistic regression analyses revealed that diabetes, coronary heart disease, PFD value, and radiomics signature were independent predictors of CSVD progression, as detailed in Table 4.
Table 4
Variable | Univariate logistic regression | Multivariate logistic regression | |||
---|---|---|---|---|---|
OR (95% CI) | P value | OR (95% CI) | P value | ||
Age | 1.01 (0.98–1.04) | 0.625 | – | – | |
Sex | 0.76 (0.43–1.32) | 0.323 | – | – | |
BMI | 1.11 (1.01–1.21) | 0.023 | 1.07 (0.98–1.18) | 0.144 | |
Hypertension | 1.21 (0.66–2.21) | 0.539 | – | – | |
Systolic pressure | 1.01 (0.99–1.02) | 0.387 | – | – | |
Diastolic pressure | 0.99 (0.97–1.01) | 0.600 | – | – | |
Hyperlipidemia | 0.82 (0.24–2.79) | 0.757 | – | – | |
Diabetes | |||||
Non-diabetic | – | – | – | – | |
Well-controlled diabetic | 1.39 (0.62–3.12)* | 0.427 | 1.86 (0.78–4.45) | 0.164 | |
Poorly-controlled diabetic | 3.16(1.36–9.59)# | 0.010 | 4.09 (1.48–11.30) | 0.007 | |
Coronary heart disease | 0.23(0.06–0.83) | 0.025 | 0.18 (0.04–0.67) | 0.011 | |
Current stroke | 1.47 (0.77–2.83) | 0.243 | – | – | |
Previous stroke | 0.81 (0.43–1.53) | 0.515 | – | – | |
Current smoking | 0.67 (0.34–1.31) | 0.239 | – | – | |
Drinking | 0.72 (0.35–1.47) | 0.365 | – | – | |
Antihypertension use | 1.24 (0.70–2.18) | 0.468 | – | – | |
Statin use | 1.15 (0.55–2.41) | 0.707 | – | – | |
Antiplatelet use | 1.48 (0.73–3.01) | 0.282 | – | – | |
TC | 0.76 (0.56–1.03) | 0.077 | – | – | |
TG | 0.80 (0.58–1.09) | 0.162 | – | – | |
HDL-C | 0.33 (0.13–0.86) | 0.023 | 0.49 (0.18–1.32) | 0.159 | |
LDL-C | 0.88 (0.60–1.30) | 0.527 | – | – | |
WBC | 0.87 (0.77–0.99) | 0.039 | 0.90 (0.78–1.03) | 0.125 | |
CRP | 0.99 (0.97–1.01) | 0.196 | – | – | |
Cerebral perfusion status | 0.66 (0.37–1.20) | 0.177 | – | – | |
PFD value | 1.11 (1.06–1.16) | <0.001 | 1.13 (1.07–1.19) | <0.001 | |
Radiomics signature | 14.51 (5.86–35.89) | <0.001 | 12.31 (4.65–32.61) | <0.001 |
*, comparison between the non-diabetic group and the well-controlled diabetic group; #, comparison between the non-diabetic group and the poorly-controlled diabetic group. CSVD, cerebral small vessel disease; OR, odds ratio; CI, confidence interval; BMI, body mass index; TC, total cholesterol; TG, triglycerides; HDL-C, high-density lipoprotein cholesterol; LDL-C, low-density lipoprotein cholesterol; WBC, white blood cell count; CRP, C-reactive protein; PFD, perivascular fat density.
The predictive performance of the models is displayed in Table 5 and Figure 6. The single PFD model showed moderate performance (AUCs of 0.702, 0.734, and 0.681 in the training, internal, and external validation sets, respectively); the radiomic model also demonstrated moderate performance (AUCs of 0.756, 0.675, and 0.679 in the training, internal, and external validation sets, respectively). In comparison, the hybrid model F demonstrated the highest predictive ability, with AUC values of 0.818, 0.805, and 0.807 in the training, internal validation, and external validation sets, respectively.
Table 5
Dataset | Model | Included variable | AUC (95% CI) | Accuracy | Sensitivity | Specificity |
---|---|---|---|---|---|---|
Training | Model A | Clinical risk factors | 0.598 (0.522–0.671) | 0.506 | 0.314 | 0.845 |
Model B | PFD | 0.702 (0.618–0.782) | 0.675 | 0.686 | 0.655 | |
Model C | Radiomics signatures | 0.756 (0.672–0.828) | 0.700 | 0.686 | 0.724 | |
Model D | Clinical risk factors + PFD | 0.735 (0.655–0.811) | 0.725 | 0.814 | 0.569 | |
Model E | Clinical risk factors + radiomics signatures | 0.763 (0.682–0.835) | 0.731 | 0.794 | 0.621 | |
Model F | Clinical risk factors + PFD + radiomics signatures | 0.818 (0.758–0.876) | 0.725 | 0.657 | 0.845 | |
Internal validation | Model A | Clinical risk factors | 0.575 (0.472–0.675) | 0.464 | 0.227 | 0.880 |
Model B | PFD | 0.734 (0.616–0.839) | 0.638 | 0.682 | 0.560 | |
Model C | Radiomics signatures | 0.675 (0.543–0.813) | 0.623 | 0.591 | 0.680 | |
Model D | Clinical risk factors + PFD | 0.694 (0.566–0.825) | 0.681 | 0.750 | 0.560 | |
Model E | Clinical risk factors + radiomics signatures | 0.755 (0.631–0.870) | 0.681 | 0.750 | 0.560 | |
Model F | Clinical risk factors + PFD + radiomics signatures | 0.805 (0.690–0.908) | 0.696 | 0.659 | 0.760 | |
External validation | Model A | Clinical risk factors | 0.574 (0.469–0.677) | 0.508 | 0.302 | 0.909 |
Model B | PFD | 0.681 (0.538–0.820) | 0.523 | 0.372 | 0.818 | |
Model C | Radiomics signatures | 0.679 (0.525–0.816) | 0.662 | 0.698 | 0.591 | |
Model D | Clinical risk factors + PFD | 0.699 (0.539–0.828) | 0.677 | 0.791 | 0.455 | |
Model E | Clinical risk factors + radiomics signatures | 0.722 (0.592–0.848) | 0.585 | 0.488 | 0.773 | |
Model F | Clinical risk factors + PFD + radiomics signatures | 0.807 (0.676–0.921) | 0.723 | 0.698 | 0.773 |
Clinical risk factors include diabetes and coronary heart disease. CSVD, cerebral small vessel disease; PFD, perivascular fat density; AUC, area under the receiver operating characteristic curve; CI, confidence interval.
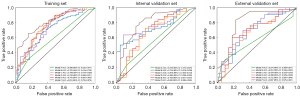
Discussion
Identifying reliable biomarkers to predict the progression of CSVD holds significant clinical relevance, as it enables early intervention and reduces the risk of complications for patients. The present study has revealed a correlation between an increased PFD value and the degree of lacunes, CMB, and WMH. More importantly, we found a significant association between PFD values and the progression of CSVD. Furthermore, the hybrid model incorporating clinical risk factors, PFD value, and radiomic features performed well in predicting CSVD progression. The inclusion of PFD value and radiomic signatures significantly improved the model’s AUC (P<0.05) in training, internal validation, and external validation sets.
Cerebral ischemia and hypoperfusion have been linked to CSVD pathogenesis (43). In a cross-sectional study, larger WMH burden has been consistently associated with lower resting CBF (44). Symptomatic carotid artery stenosis would manifest as perfusion abnormalities on CTP, which could lead to CSVD on MRI (45). To assess the possible contribution of PVAT to the pathogenesis of CSVD in the absence of cerebral hypoperfusion, our study focused on patients without carotid stenosis, and in fact, the results of CTP revealed no significant differences in perfusion between the CSVD progression and non-progression groups. This suggests that the progression of CSVD in patients with PVAT without carotid stenosis may be associated with the inflammation present in PVAT. Our results also suggest that PFD values could serve as an independent predictive factor for CSVD progression.
Previous studies have found that changes in the structure and function of the upstream large blood vessels may also have downstream effects on the cerebral microvasculature (46,47). Sustained inflammatory stimuli induce endothelial dysfunction (48). On the one hand, this promotes platelet aggregation and increases vasoconstriction, further exacerbating the condition by enhancing inflammation and pathological remodeling, contributing to the narrowing and stiffening of arteries (49,50). On the other hand, within the cerebrovascular system, endothelial dysfunction significantly affects the integrity of the BBB, further resulting in microglial activation, neuroinflammation, neuronal and synaptic dysfunction, and white matter damage (51,52). We found that density variations in PVAT around the carotid artery were associated with lacunes, CMBs, and WMH, which was also found in Zhang et al.’s study (21). We took a step further to employ an automated segmentation method to measure WMH volume in our study, providing a more objective, accurate, and consistent approach compared to the visual Fazekas scale used in earlier research.
Our study discovered that the PFD value independently influences the occurrence of CSVD progression. Inflammation emerges as a critical factor in the progression of both atherosclerosis and CSVD, acting as intertwined pathological processes (8,51). However, circulatory inflammatory indicators such as neutrophils and C-reactive protein showed no significant correlation with CSVD progression in our study, potentially indicating that this local inflammation might be independent of any generalized systemic inflammation. We used techniques already applied in coronary artery studies, measuring HU to assess PVAT density on CT scans, which was therefore highly reproducible. This approach facilitates the measurement and identification of high-risk patients.
A previous study constructed a predictive model using radiomics features of PVAT around carotid plaque to evaluate the risk of plaque with a training set AUC of 0.834 and a validation set AUC of 0.837 (53). CT-based radiomics can classify diseases by extracting quantifiable radiomic features imperceptible to the human eye (54). In this study, the hybrid model that integrates clinical risk factors, PFD value, and radiomic features of PVAT demonstrated good performance in terms of accuracy, sensitivity, and specificity, achieving the best AUC values of 0.818, 0.805, and 0.807 in the training, internal validation, and external validation sets, respectively. Radiomics could integrate various imaging features to provide more comprehensive biomarker information, offering additional benefits for the identification of the progressive CSVD.
This study has several limitations. Firstly, it is retrospective and thus cannot establish causal relationships between perivascular fat and CSVD progression. Future prospective studies with larger sample sizes are needed. Secondly, inflammatory changes in PVAT were not histologically validated. This study provides partial texture feature information through radiomics analysis. Finally, we did not assess the association between PFD and occurrence of cerebrovascular events in our study.
Conclusions
Our study has shown that, in patients without carotid stenosis, PFD can predict the progression of CSVD, which suggests that inflammatory mechanisms related to pericarotid adipose tissue may be involved in the pathogenesis of CSVD. Incorporating PFD and PVAT radiomics signatures into the predictive model could improve the ability to predict the progression of the disease in CSVD patients.
Acknowledgments
We would like to thank all the participants for their cooperation.
Footnote
Reporting Checklist: The authors have completed the TRIPOD reporting checklist. Available at https://qims.amegroups.com/article/view/10.21037/qims-24-1512/rc
Funding: This study was funded by
Conflicts of Interest: All authors have completed the ICMJE uniform disclosure form (available at https://qims.amegroups.com/article/view/10.21037/qims-24-1512/coif). Z.Y. is the employee of ShuKun Technology and has a collaborative relationship with this study. The other authors have no conflicts of interest to declare.
Ethical Statement: The authors are accountable for all aspects of the work in ensuring that questions related to the accuracy or integrity of any part of the work are appropriately investigated and resolved. The study was conducted in accordance with the Declaration of Helsinki (as revised in 2013). The study was approved by the Ethics Committees of Zhejiang Provincial People’s Hospital (No. QT2024163) and Hangzhou Traditional Chinese Medicine Hospital (No. 202412100953000019995), and the requirement for informed consent was waived due to the retrospective nature of the study.
Open Access Statement: This is an Open Access article distributed in accordance with the Creative Commons Attribution-NonCommercial-NoDerivs 4.0 International License (CC BY-NC-ND 4.0), which permits the non-commercial replication and distribution of the article with the strict proviso that no changes or edits are made and the original work is properly cited (including links to both the formal publication through the relevant DOI and the license). See: https://creativecommons.org/licenses/by-nc-nd/4.0/.
References
- Li Q, Yang Y, Reis C, Tao T, Li W, Li X, Zhang JH. Cerebral Small Vessel Disease. Cell Transplant 2018;27:1711-22. [Crossref] [PubMed]
- Wardlaw JM, Smith C, Dichgans M. Small vessel disease: mechanisms and clinical implications. Lancet Neurol 2019;18:684-96. [Crossref] [PubMed]
- Duering M, Biessels GJ, Brodtmann A, Chen C, Cordonnier C, de Leeuw FE, et al. Neuroimaging standards for research into small vessel disease-advances since 2013. Lancet Neurol 2023;22:602-18. [Crossref] [PubMed]
- Markus HS, de Leeuw FE. Cerebral small vessel disease: Recent advances and future directions. Int J Stroke 2023;18:4-14. [Crossref] [PubMed]
- Ihara M, Yamamoto Y. Emerging Evidence for Pathogenesis of Sporadic Cerebral Small Vessel Disease. Stroke 2016;47:554-60. [Crossref] [PubMed]
- Cai J, Zeng X, Huang X, Dong H, Liu J, Lin J, Xie M, Wei X. Relationship of neutrophil/lymphocyte ratio with cerebral small vessel disease and its common imaging markers. Immun Inflamm Dis 2024;12:e1228. [Crossref] [PubMed]
- Xiao Y, Teng Z, Xu J, Qi Q, Guan T, Jiang X, Chen H, Xie X, Dong Y, Lv P. Systemic Immune-Inflammation Index is Associated with Cerebral Small Vessel Disease Burden and Cognitive Impairment. Neuropsychiatr Dis Treat 2023;19:403-13. [Crossref] [PubMed]
- Low A, Mak E, Rowe JB, Markus HS, O'Brien JT. Inflammation and cerebral small vessel disease: A systematic review. Ageing Res Rev 2019;53:100916. [Crossref] [PubMed]
- Walker KA, Windham BG, Power MC, Hoogeveen RC, Folsom AR, Ballantyne CM, Knopman DS, Selvin E, Jack CR Jr, Gottesman RF. The association of mid-to late-life systemic inflammation with white matter structure in older adults: The Atherosclerosis Risk in Communities Study. Neurobiol Aging 2018;68:26-33. [Crossref] [PubMed]
- Nam KW, Kwon HM, Jeong HY, Park JH, Kim SH, Jeong SM, Yoo TG, Kim S. High neutrophil to lymphocyte ratio is associated with white matter hyperintensity in a healthy population. J Neurol Sci 2017;380:128-31. [Crossref] [PubMed]
- Moss JW, Ramji DP. Cytokines: roles in atherosclerosis disease progression and potential therapeutic targets. Future Med Chem 2016;8:1317-30. [Crossref] [PubMed]
- Altaf N, Morgan PS, Moody A, MacSweeney ST, Gladman JR, Auer DP. Brain white matter hyperintensities are associated with carotid intraplaque hemorrhage. Radiology 2008;248:202-9. [Crossref] [PubMed]
- Fan X, Zhang X, Lai Z, Lin T, You H, Liu C, Feng F. Cerebral Small Vessel Disease Burden Related to Carotid Intraplaque Hemorrhage Serves as an Imaging Marker for Clinical Symptoms in Carotid Stenosis. Front Neurol 2021;12:731237. [Crossref] [PubMed]
- Li J, Wu H, Hang H, Sun B, Zhao H, Chen Z, Zhou Y, Xu J, Chen J, Zhou D, Zhao X, Yuan C. Carotid vulnerable plaque coexisting with cerebral small vessel disease and acute ischemic stroke: a Chinese Atherosclerosis Risk Evaluation study. Eur Radiol 2022;32:6080-9. [Crossref] [PubMed]
- Conijn MM, Kloppenborg RP, Algra A, Mali WP, Kappelle LJ, Vincken KL, van der Graaf Y, Geerlings MISMART Study Group. Cerebral small vessel disease and risk of death, ischemic stroke, and cardiac complications in patients with atherosclerotic disease: the Second Manifestations of ARTerial disease-Magnetic Resonance (SMART-MR) study. Stroke 2011;42:3105-9. [Crossref] [PubMed]
- Antonopoulos AS, Sanna F, Sabharwal N, Thomas S, Oikonomou EK, Herdman L, et al. Detecting human coronary inflammation by imaging perivascular fat. Sci Transl Med 2017;9:eaal2658. [Crossref] [PubMed]
- Katsiki N, Mikhailidis DP. Perivascular Adipose Tissue: Pathophysiological Links With Inflammation, Atherosclerosis, and Thrombosis. Angiology 2022;73:195-6. [Crossref] [PubMed]
- Yu M, Meng Y, Zhang H, Wang W, Qiu S, Wang B, Bao Y, Du B, Zhu S, Ge Y, Zhu L, Xu K. Associations between pericarotid fat density and image-based risk characteristics of carotid plaque. Eur J Radiol 2022;153:110364. [Crossref] [PubMed]
- Zhang S, Yu X, Gu H, Kang B, Guo N, Wang X. Identification of high-risk carotid plaque by using carotid perivascular fat density on computed tomography angiography. Eur J Radiol 2022;150:110269. [Crossref] [PubMed]
- Baradaran H, Myneni PK, Patel P, Askin G, Gialdini G, Al-Dasuqi K, Kamel H, Gupta A. Association Between Carotid Artery Perivascular Fat Density and Cerebrovascular Ischemic Events. J Am Heart Assoc 2018;7:e010383. [Crossref] [PubMed]
- Zhang DH, Jin JL, Zhu CF, Chen QY, He XW. Association between carotid artery perivascular fat density and cerebral small vessel disease. Aging (Albany NY) 2021;13:18839-51. [Crossref] [PubMed]
- Barnett HJM, Taylor DW, Haynes RB, Sackett DL, Peerless SJ, Ferguson GG, Fox AJ, Rankin RN, Hachinski VC, Wiebers DO, Eliasziw M. Beneficial effect of carotid endarterectomy in symptomatic patients with high-grade carotid stenosis. N Engl J Med 1991;325:445-53. [Crossref] [PubMed]
- Handelsman Y, Bloomgarden ZT, Grunberger G, Umpierrez G, Zimmerman RS, Bailey TS, et al. American association of clinical endocrinologists and american college of endocrinology - clinical practice guidelines for developing a diabetes mellitus comprehensive care plan - 2015. Endocr Pract 2015;21:1-87. [Crossref] [PubMed]
- Lin L, Bivard A, Krishnamurthy V, Levi CR, Parsons MW, Whole-Brain CT. Perfusion to Quantify Acute Ischemic Penumbra and Core. Radiology 2016;279:876-87. [Crossref] [PubMed]
- Lan Y, Shang J, Ma Y, Zhen Y, Dang Y, Ren D, Liu T, Ju R, Guo N, Wang X, Hou Y. A new predictor of coronary artery disease in acute ischemic stroke or transient ischemic attack patients: pericarotid fat density. Eur Radiol 2024;34:1667-76. [Crossref] [PubMed]
- Fu F, Wei J, Zhang M, Yu F, Xiao Y, Rong D, Shan Y, Li Y, Zhao C, Liao F, Yang Z, Li Y, Chen Y, Wang X, Lu J. Rapid vessel segmentation and reconstruction of head and neck angiograms using 3D convolutional neural network. Nat Commun 2020;11:4829. [Crossref] [PubMed]
- Antoniades C, Tousoulis D, Vavlukis M, Fleming I, Duncker DJ, Eringa E, Manfrini O, Antonopoulos AS, Oikonomou E, Padró T, Trifunovic-Zamaklar D, De Luca G, Guzik T, Cenko E, Djordjevic-Dikic A, Crea F. Perivascular adipose tissue as a source of therapeutic targets and clinical biomarkers. Eur Heart J 2023;44:3827-44. [Crossref] [PubMed]
- Tu YB, Gu M, Zhou SQ, Xie G, Liu LL, Deng FB, Li K. Pericoronary adipose tissue attenuation in patients with acute aortic dissection based on coronary computed tomography angiography. Quant Imaging Med Surg 2024;14:31-42. [Crossref] [PubMed]
- Oikonomou EK, Marwan M, Desai MY, Mancio J, Alashi A, Hutt Centeno E, et al. Non-invasive detection of coronary inflammation using computed tomography and prediction of residual cardiovascular risk (the CRISP CT study): a post-hoc analysis of prospective outcome data. Lancet 2018;392:929-39. [Crossref] [PubMed]
- Zhang WZ, Li PL, Gao Y, Chen XY, He LY, Zhang Q, Yu JQ. Relationships between the coronary fat attenuation index for patients with heart-related disease measured automatically on coronary computed tomography angiography and coronary adverse events and degree of coronary stenosis. Quant Imaging Med Surg 2023;13:8218-29. [Crossref] [PubMed]
- Wardlaw JM, Valdés Hernández MC, Muñoz-Maniega S. What are white matter hyperintensities made of? Relevance to vascular cognitive impairment. J Am Heart Assoc 2015;4:001140. [Crossref] [PubMed]
- Fazekas F, Barkhof F, Wahlund LO, Pantoni L, Erkinjuntti T, Scheltens P, Schmidt R. AMECT and MRI rating of white matter lesions. Cerebrovasc Dis 2002;13:31-6. [Crossref] [PubMed]
- Wardlaw JM, Benveniste H, Nedergaard M, Zlokovic BV, Mestre H, Lee H, et al. Perivascular spaces in the brain: anatomy, physiology and pathology. Nat Rev Neurol 2020;16:137-53. [Crossref] [PubMed]
- Lau KK, Li L, Schulz U, Simoni M, Chan KH, Ho SL, Cheung RTF, Küker W, Mak HKF, Rothwell PM. Total small vessel disease score and risk of recurrent stroke: Validation in 2 large cohorts. Neurology 2017;88:2260-7. [Crossref] [PubMed]
- Pasi M, Sugita L, Xiong L, Charidimou A, Boulouis G, Pongpitakmetha T, Singh S, Kourkoulis C, Schwab K, Greenberg SM, Anderson CD, Gurol ME, Rosand J, Viswanathan A, Biffi A. Association of Cerebral Small Vessel Disease and Cognitive Decline After Intracerebral Hemorrhage. Neurology 2021;96:e182-92. [Crossref] [PubMed]
- Cho AH, Kim HR, Kim W, Yang DW. White matter hyperintensity in ischemic stroke patients: it may regress over time. J Stroke 2015;17:60-6. [Crossref] [PubMed]
- Jin H, Hou J, Qin X, Du X, Zheng G, Meng Y, Shu Z, Wei Y, Gong X. Predicting progression of white matter hyperintensity using coronary artery calcium score based on coronary CT angiography-feasibility and accuracy. Front Aging Neurosci 2023;15:1256228. [Crossref] [PubMed]
- Dissaux G, Visvikis D, Da-Ano R, Pradier O, Chajon E, Barillot I, Duvergé L, Masson I, Abgral R, Santiago Ribeiro MJ, Devillers A, Pallardy A, Fleury V, Mahé MA, De Crevoisier R, Hatt M, Schick U. Pretreatment (18)F-FDG PET/CT Radiomics Predict Local Recurrence in Patients Treated with Stereotactic Body Radiotherapy for Early-Stage Non-Small Cell Lung Cancer: A Multicentric Study. J Nucl Med 2020;61:814-20. [Crossref] [PubMed]
- Ligero M, Jordi-Ollero O, Bernatowicz K, Garcia-Ruiz A, Delgado-Muñoz E, Leiva D, Mast R, Suarez C, Sala-Llonch R, Calvo N, Escobar M, Navarro-Martin A, Villacampa G, Dienstmann R, Perez-Lopez R. Minimizing acquisition-related radiomics variability by image resampling and batch effect correction to allow for large-scale data analysis. Eur Radiol 2021;31:1460-70. [Crossref] [PubMed]
- Zwanenburg A, Vallières M, Abdalah MA, Aerts HJWL, Andrearczyk V, Apte A, et al. The Image Biomarker Standardization Initiative: Standardized Quantitative Radiomics for High-Throughput Image-based Phenotyping. Radiology 2020;295:328-38. [Crossref] [PubMed]
- van Griethuysen JJM, Fedorov A, Parmar C, Hosny A, Aucoin N, Narayan V, Beets-Tan RGH, Fillion-Robin JC, Pieper S, Aerts HJWL. Computational Radiomics System to Decode the Radiographic Phenotype. Cancer Res 2017;77:e104-7. [Crossref] [PubMed]
- Orlhac F, Lecler A, Savatovski J, Goya-Outi J, Nioche C, Charbonneau F, Ayache N, Frouin F, Duron L, Buvat I. How can we combat multicenter variability in MR radiomics? Validation of a correction procedure. Eur Radiol 2021;31:2272-80. [Crossref] [PubMed]
- Pantoni L. Cerebral small vessel disease: from pathogenesis and clinical characteristics to therapeutic challenges. Lancet Neurol 2010;9:689-701. [Crossref] [PubMed]
- Shi Y, Thrippleton MJ, Makin SD, Marshall I, Geerlings MI, de Craen AJM, van Buchem MA, Wardlaw JM. Cerebral blood flow in small vessel disease: A systematic review and meta-analysis. J Cereb Blood Flow Metab 2016;36:1653-67. [Crossref] [PubMed]
- Mohimen A, Gupta A, Gill S, Sahu S, Anadure R. Correlation of CT perfusion with MRI brain in symptomatic carotid artery stenosis. Med J Armed Forces India 2023;79:421-7. [Crossref] [PubMed]
- Nyúl-Tóth Á, Patai R, Csiszar A, Ungvari A, Gulej R, Mukli P, Yabluchanskiy A, Benyo Z, Sotonyi P, Prodan CI, Liotta EM, Toth P, Elahi F, Barsi P, Maurovich-Horvat P, Sorond FA, Tarantini S, Ungvari Z. Linking peripheral atherosclerosis to blood-brain barrier disruption: elucidating its role as a manifestation of cerebral small vessel disease in vascular cognitive impairment. Geroscience 2024;46:6511-36. [Crossref] [PubMed]
- Zhai FF, Yang M, Wei Y, Wang M, Gui Y, Han F, Zhou LX, Ni J, Yao M, Zhang SY, Jin ZY, Cui LY, Dai Q, Zhu YC. Carotid atherosclerosis, dilation, and stiffness relate to cerebral small vessel disease. Neurology 2020;94:e1811-9. [Crossref] [PubMed]
- Hannawi Y. Cerebral Small Vessel Disease: a Review of the Pathophysiological Mechanisms. Transl Stroke Res 2024;15:1050-69. [Crossref] [PubMed]
- Cooper A, Heagerty AM. Endothelial dysfunction in human intramyocardial small arteries in atherosclerosis and hypercholesterolemia. Am J Physiol 1998;275:H1482-8. [Crossref] [PubMed]
- Vanhoutte PM. Endothelial dysfunction and atherosclerosis. Eur Heart J 1997;18 Suppl E:E19-29.
- Toth P, Tarantini S, Csiszar A, Ungvari Z. Functional vascular contributions to cognitive impairment and dementia: mechanisms and consequences of cerebral autoregulatory dysfunction, endothelial impairment, and neurovascular uncoupling in aging. Am J Physiol Heart Circ Physiol 2017;312:H1-H20. [Crossref] [PubMed]
- Elahi FM, Wang MM, Meschia JF. Cerebral Small Vessel Disease-Related Dementia: More Questions Than Answers. Stroke 2023;54:648-60. [Crossref] [PubMed]
- Nie JY, Chen WX, Zhu Z, Zhang MY, Zheng YJ, Wu QD. Initial experience with radiomics of carotid perivascular adipose tissue in identifying symptomatic plaque. Front Neurol 2024;15:1340202. [Crossref] [PubMed]
- Xia H, Yuan L, Zhao W, Zhang C, Zhao L, Hou J, Luan Y, Bi Y, Feng Y. Predicting transient ischemic attack risk in patients with mild carotid stenosis using machine learning and CT radiomics. Front Neurol 2023;14:1105616. [Crossref] [PubMed]