An automatic deep-learning approach for the prediction of post-stroke epilepsy after an initial intracerebral hemorrhage based on non-contrast computed tomography imaging
Introduction
The International League Against Epilepsy defines post-stroke epilepsy (PSE) as epilepsy occurring more than 7 days following a stroke, and sets out the common sequela of this cerebrovascular event (1,2). PSE has an incidence rate of about 4–16%, and it can significantly aggravate neurological symptoms, cerebral tissue damage, and the risk of fatality (3,4). PSE is caused by a combination of multiple risk factors, including intracerebral hemorrhage (ICH) volume, cortical involvement, stroke location, early seizure (seizure occurring within 7 days of stroke), and personal past medical history (5-8). The incidence of epilepsy is higher in hemorrhagic stroke patients than ischemic stroke patients (9,10). Moreover, the risk of PSE development in patients with both hemorrhagic stroke and cortical involvement is approximately 16 times higher than that in other patients (11).
At present, various methods can be used to assess epileptogenesis and diagnose PSE, including computed tomography (CT), electroencephalogram (EEG), magnetic resonance imaging, single-photon emission CT, magnetoencephalogram, and biological biomarkers (12). In recent years, several clinical scoring tools, such as the CAVE score (cortical involvement, age <65 years, ICH volume >10 mL, and early seizures), and the LANE score [lobar hemorrhage, age <65 years, National Institute of Health Stroke Scale (NIHSS) score ≥15, and early seizures], have been developed to identify high-risk PSE patients (13,14). However, these scoring systems rely only on clinical variables, which can be labor intensive to collect. Moreover, the determination of certain clinical parameters, such as cortical involvement, or certain risk factors can be subjective, leading to different scoring outcomes.
Recent studies have shown that deep-learning models can effectively diagnose various diseases, such as brain tumors, skin cancer, lung cancer, prostate tumors, thyroid, and multiple sclerosis (15-21). These diseases involve complex and diverse diagnostic and treatment processes, leading to unpredictable treatment outcomes. Similarly, deep-learning models, especially those that utilize EEG signal patterns, have achieved good results in predicting epileptic seizures (22-24). Notably, deep-learning models can automatically learn task-specific feature representations, and discern intricate hidden patterns directly from data, thus improving large-scale medical imaging evaluations, and enhancing the efficiency and accessibility of medical diagnoses. However, to date, no study has assessed whether deep learning can be used to predict PSE.
Due to its high spatial resolution, rapid imaging speed, and sensitivity to brain hemorrhage, non-contrast computed tomography (NCCT) is the preferred imaging modality for the initial screening of patients with uncertain neurological symptoms or head trauma (25). Thus, this study aimed to develop, train, and validate a novel deep-learning model to accurately identify high-risk patients who may develop PSE after an initial ICH using NCCT data. We present this article in accordance with the TRIPOD + AI reporting checklist (available at https://qims.amegroups.com/article/view/10.21037/qims-24-1345/rc).
Methods
Subjects
This study was approved by the Ethics Committee of The First Affiliated Hospital of Wenzhou Medical University (No. 2020–185). The study was conducted in accordance with the Declaration of Helsinki (as revised in 2013). The subjects or their legally authorized representatives provided verbal informed consent through telephone interviews; the requirement of written informed consent was waived for all participants.
All NCCT scans were retrospectively acquired from patients consecutively diagnosed with ICH at The First Affiliated Hospital of Wenzhou Medical University between January 4, 2010, and July 31, 2020. The corresponding clinicodemographic information was also obtained. Patients were excluded from the study if they met any of the following exclusion criteria: (I) had poor-quality images; (II) had a primary intraventricular hemorrhage or multiple hemorrhagic foci; (III) had a previous history of stroke; (IV) had a history of epilepsy, or other risk factors that can cause epilepsy development, such as intracranial tumors or a history of traumatic brain injury; (V) had not undergone an initial CT scan within 72 hours of the onset of stroke, or had an insufficiently defined initial region of interest (ROI) on the CT; and (VI) had died without a diagnosis of PSE, or had been lost to follow-up within 1 year of the ICH. The patient recruitment process and exclusion criteria are presented in Figure 1.
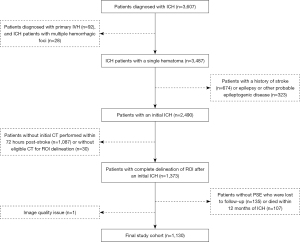
Neuroimaging acquisition and manual ground-truth segmentation
All patients underwent medical imaging examination within 72 hours of ICH onset. The NCCT images were captured using a 64-channel multidetector CT scanner, specifically, the LightSpeed VCT 64 model by GE Healthcare. The following scanning parameters were employed: slice thickness: 5 mm; reconstruction interval: 5 mm; tube voltage: 120 kV; tube current: 80 mAs; and matrix size: 512×512.
Two radiologists with 3 and 6 years of radiology experience manually outlined the ROI along the hematoma profile on each NCCT slice of each patient (a total of 6,390 slices) using 3D Slicer software (version 4.10.2, National Institutes of Health). Notably, only intraparenchymal ICHs were included in the ROIs, and any intraventricular hemorrhages were excluded. The masks were annotated with 1 and 0 for ICH and non-ICH at the pixel level, respectively.
PSE identification and follow-up
PSE identification involved a thorough examination of medical records. Additionally, tele-interviews were conducted using a modified standardized questionnaire (26) (Table S1). Two neurologists (with 5 and 16 years of experience, respectively) reached a consensus on the presence of PSE, and resolved any uncertainties in the clinical data collection. In the event of a disagreement, a senior neurologist (with 29 years of experience) made the final decision.
Deep-learning workflow
In this study, a comprehensive approach was devised for the automated prediction of PSE (Figure 2). This approach comprises two key phases: first, image preprocessing and quantitative analysis (Figure 2A), followed by the PSE prediction task, the constructed deep-learning model, named the post-stroke epilepsy network (PSENet) (Figure 2B). Further details and explanations are provided below.
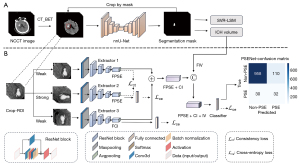
Image preprocessing and quantitative analysis
In the image preprocessing stage (Figure 2A), non-cerebral tissues, such as the skull, scalp, and dura, were first removed from the NCCT scans of each patient using computed tomography_brain extraction tool (CT_BET) (27,28). The resulting processed NCCT scans were then input into the three-dimensional (3D) full-resolution no-new-Net (nnU-Net) to automatically segment the ROI (29) [Supplementary file (Appendix 1)]. Subsequently, each slice image was cropped based on the pixel coordinates’ maximum and minimum values in the ROI to emphasize the focus on the hemorrhage area. Due to variations in ROI sizes across different images, the resulting sizes of the cropped images differed. Therefore, the nearest-neighbor interpolation method was used to re-sample the cropped images to achieve a consistent size of 64×64×8, ensuring uniformity in image dimensions. The cropped image pixels were also normalized using minimum-maximum normalization to scale the values between 0 and 1.
In addition, a quantitative analysis was performed on the segmentation masks, including ICH volume measurement and a multivariate support vector regression for the lesion-symptom mapping (SVR-LSM) analysis, to gain a comprehensive understanding of the relationship between PSE and hemorrhage locations [Supplementary file (Appendix 2)].
Development of the PSENet
For the PSE prediction task, a triple PSENet (Figure 2B) was designed based on ResNet34 (30). Specifically, Extractor1 and Extractor3 applied weak augmentations (RandFlip) to preserve essential image features, while Extractor2 used strong augmentations (RandRotate, RandFlip, and RandomAffine) to introduce more challenging distortions, and thus improve model robustness. Extractor1 and Extractor2 extracted features related to post-stroke epilepsy (FPSE) obtained from the same image after the different enhancements, respectively, and calculated the feature consistency loss (31) to determine the similarity of the images. Extractor3 extracted deep-learning features related to cortical involvement (FCI) after weak image enhancement.
The FPSE and FCI shared the same spatial dimension. A new feature map comprising the FPSE and FCI [the FPSE + cortical involvement (CI)] was established through element-wise addition before fully connected layers. This fusion step aimed to synergistically use the information from both feature maps to enhance the discriminative capabilities of the model in predicting the occurrence of PSE. Finally, we created the feature FPSE + CI + intracerebral hemorrhage volume (IV) by combining the features related to ICH volume (FIV) with the feature FPSE + CI.
To mitigate the risks of overfitting and data distribution imbalance, data augmentation was used. First, the number of patient samples for PSE and non-PSE were determined, followed by random sampling based on their relative weights to ensure a more balanced distribution of positive and negative samples in the training set. As repeated sampling could lead to model overfitting due to the scarcity of PSE patients, 3D data augmentation technology was leveraged under the MONAI libraries (version 1.0.1), specifically targeting minority categories. Moreover, a flip operation was introduced to the PSE patient data with a certain probability to enhance the diversity of the training samples. This strategy ensured that an equal number of samples were randomly selected from each category during each iteration, thereby achieving data balance without the need to expand or shrink the overall dataset size.
The networks used a cross-entropy loss function, consistency loss function, and Adam optimizer, implemented in PyTorch 1.13.1 on an NVIDIA RTX 2080Ti 11 GB GPU. The training spanned 200 epochs with a batch size of 32, and had an initial learning rate of 0.01.
Development of clinical prediction models
To facilitate the comparative analysis with the PSENet, a random-forest model was used to establish three different clinical prediction models because of its interpretable tree-based structure. These models include a baseline clinical model incorporating all variables, the CAVE score model (13), and the LANE score model (14). A comparative analysis was also conducted of the PSENet and the neurological imaging (NIHSS) scoring method, which plays a pivotal role in assessing the condition of stroke patients. Throughout the training process, we adjusted the “CLASS_WEIGHT” parameter of the models to “BALANCED” to address the data imbalance issue (32).
Statistical analysis
The variables were summarized using descriptive statistics. A normality test was conducted on the continuous variables, followed by an independent t-test or Mann-Whitney U test. The Chi-squared test or Fisher’s exact test was used to compare categorical variables. The statistical tests were two-sided. A P value <0.05 indicated a statistically significant difference. SPSS software (version 26, IBM SPSS Statistics, IBM Corporation, Chicago, Illinois, USA) was used for all statistical analyses.
Five-fold cross-validation was used in this study, dividing the dataset into five subsets. Each subset served as the validation set once, with the others forming the training sets, ensuring fair assessment. In addition, the validation set data remained completely separate from the training set data to maintain the independence of each. PSE prediction was evaluated by the receiver operating characteristic (ROC) analysis, using the area under the curve (AUC), accuracy, recall, F1-score, and specificity. Macro-averaging (33) was used to mitigate the impact of the data imbalance on the model evaluation, considering the importance of each category and the sample size, thus providing an accurate assessment of overall model performance. All the results presented are the average of the five iterations conducted. The significant differences between the AUC values were assessed using the DeLong test. The rmda package of R software (version 4.1.2) was used to conduct the decision curve analysis (DCA), and compare the clinical efficacy of the different prediction models under different treatment decision thresholds. The regions that significantly contributed to the prediction results were identified using the gradient-weighted class activation mapping (Grad-CAM) technique to enhance the interpretability of different models (34).
Results
Patient characteristics
The patient demographics, image characteristics, and univariate analysis results are summarized in Table 1. The final dataset comprised 1,130 patients, of whom 62 had PSE and 1,068 did not have PSE. A baseline clinical model was built based on all characteristics.
Table 1
Characteristics | PSE (n=62) | Non-PSE (n=1,068) | P value |
---|---|---|---|
Age (years) | 59.79±12.40 | 59.05±11.084 | 0.667 |
Sex | 0.284 | ||
Male | 47 (75.8) | 741 (69.4) | |
Female | 15 (24.2) | 327 (30.6) | |
ICH volume (mL) | 23.77±21.74 | 10.09±8.70 | <0.001 |
Cortical involvement | 50 (80.6) | 363 (34.0) | <0.001 |
Multilobar involvement | 22 (35.5) | 192 (18.0) | 0.001 |
Extension into ventricle | 17 (27.4) | 226 (21.2) | 0.244 |
Major location | <0.001 | ||
Frontal | 5 (8.1) | 22 (2.1) | |
Temporal | 14 (22.6) | 31 (2.9) | |
Parietal | 9 (14.5) | 27 (2.5) | |
Occipital | 5 (8.1) | 25 (2.3) | |
Deep | 28 (45.2) | 842 (78.8) | |
Cerebellum | 1 (1.6) | 67 (6.3) | |
Brainstem | 0 (0.0) | 54 (5.1) | |
Lacunar infraction | 11 (17.7) | 205 (19.2) | 0.777 |
Stroke to imaging interval | 15.41±23.22 | 12.38±14.76 | 0.280 |
NIHSS score | 12.13±9.90 | 7.68±6.23 | 0.001 |
Early seizure(s) | 19 (30.6) | 19 (1.8) | <0.001 |
Hypertension | 53 (85.5) | 994 (93.1) | 0.026 |
Diabetes | 14 (22.6) | 213 (19.9) | 0.614 |
Cardiac history | 7 (11.3) | 193 (18.1) | 0.230 |
Hyperlipidaemia | 13 (21.0) | 235 (22.0) | 0.848 |
Hyperuricemia | 6 (9.7) | 40 (3.7) | 0.022 |
Infectious complications | 15 (24.2) | 283 (26.5) | 0.689 |
Current drinking | 22 (35.5) | 418 (39.1) | 0.566 |
Current smoking | 25 (40.3) | 425 (39.8) | 0.934 |
Data are presented as mean ± standard deviation or n (%). PSE, post-stroke epilepsy; NIHSS, National Institutes of Health Stroke Scale; ICH, intracerebral hemorrhage.
ICH segmentation and quantitative analysis results
The nnU-Net had a mean Dice score and Intersection over Union (IoU) score of 0.923 and 0.874, respectively (Table 2). The visualization outcomes of two random samples are shown in Figure 3. The results of the quantitative analysis are shown in Figures S1,S2. For the ICH volume calculations, patient-level testing was performed using manual segmentation as the ground truth. The results showed that the nnU-Net was strongly correlated with the ground truth [R2=0.980, 95% confidence interval (CI): 9.934–11.166, P<0.001, Figure S1]. As Figure S2 shows, the distribution of stroke lesions in the PSE patients revealed extensive coverage of the temporal and frontal gyrus. Further, the results demonstrated that the hemorrhagic regions in the PSE patients were predominantly distributed in the inferior temporal gyrus, middle temporal gyrus, and sphenoid gyrus when overlaid with the Automated Anatomical Labeling template (Table S2).
Table 2
Model | Prediction results | Segmentation results | |||||||||
---|---|---|---|---|---|---|---|---|---|---|---|
Accuracy | F1-score | Recall | Specificity | AUC (95% CI) | Dice | IoU | Recall | Specificity | HD95 | ||
Baseline clinical model | 0.769 | 0.538 | 0.665 | 0.782 | 0.787 (0.721–0.835) | ||||||
CAVE | 0.722 | 0.519 | 0.692 | 0.726 | 0.792 (0.744–0.845) | ||||||
LANE | 0.813 | 0.572 | 0.697 | 0.828 | 0.798 (0.743–0.860) | ||||||
C3D | 0.717 | 0.48 | 0.626 | 0.73 | 0.676 (0.540–0.813) | ||||||
AlexNet | 0.746 | 0.497 | 0.618 | 0.759 | 0.699 (0.558–0.840) | ||||||
ViT | 0.677 | 0.475 | 0.622 | 0.688 | 0.758 (0.687–0.826) | ||||||
DenseNet | 0.819 | 0.57 | 0.679 | 0.839 | 0.778 (0.726–0.822) | ||||||
MobileNet | 0.859 | 0.607 | 0.683 | 0.881 | 0.804 (0.760–0.856) | ||||||
PSENet | 0.876 | 0.621 | 0.716 | 0.897 | 0.840* (0.792–0.898) | ||||||
nnU-Net | 0.923 | 0.874 | 0.932 | 1 | 2.333 |
The text with * in the prediction results represents the best performance. PSE, post-stroke epilepsy; ICH, intracerebral hemorrhage; AUC, area under the curve; CI, confidence interval; CAVE, C = cortical involvement, A = age <65 years, V = ICH volume >10 mL, E = early seizures; LANE, L = lobar hemorrhage, A = age <65 years, N = National Institutes of Health Stroke Scale score ≥15, E = early seizures; C3D, convolutional 3D; ViT, vision transformer; nnU-Net, no-new-Net; IoU, intersection over union; HD95, 95th percentile of hausdorff distance.
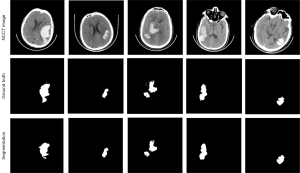
Predictive performance of the PSENet
Using the same parameters, the performance of five popular models, including C3D (35), AlexNet (36), ViT (37), DenseNet (38), and MobileNet (39) models, were assessed and compared with the PSENet. The PSENet showed the highest performance with an AUC of 0.840 (95% CI: 0.792–0.898) and an accuracy, F1-score, recall, and specificity of 0.876 (95% CI: 0.849–0.901), 0.621 (95% CI: 0.609–0.633), 0.716 (95% CI: 0.656–0.799), and 0.897 (95% CI: 0.857–0.933), respectively (Figure 4A and Table 2).
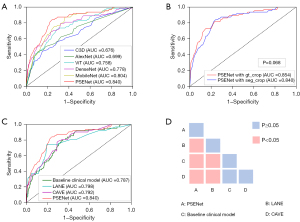
Additionally, the NCCT data were cropped using ROI based on the manual ground truth, and then input into the PSENet for predictions, resulting in an AUC of 0.854 (95% CI: 0.844–0.879). The DeLong test showed that there were no statistically significant differences in the AUC values between the two input data types (P=0.068>0.05, Figure 4B).
Feature ablation experiments on the FCI and FIV were also performed (Table 3). Notably, the combination of the FCI and FIV significantly improved the predictive performance of the model. The AUC increased from 0.782 (95% CI: 0.746–0.838) to 0.840 (95% CI: 0.792–0.898).
Table 3
FCI/FIV/FPSE | AUC (95% CI) |
---|---|
×/×/√ | 0.782 (0.746–0.838) |
√/×/√ | 0.819 (0.794–0.854) |
×/√/√ | 0.790 (0.716–0.870) |
√/√/√ | 0.840 (0.792–0.898) |
FCI, features related to cortical involvement; FIV, features related to intracerebral hemorrhage volume; FPSE, features related to post-stroke epilepsy; AUC, area under the curve; CI, confidence interval.
Comparison of the PSENet to clinical models
The AUC values for the baseline clinical model, CAVE score, and LANE score models were 0.787 (95% CI: 0.721–0.835), 0.792 (95% CI: 0.744–0.845), and 0.798 (95% CI: 0.743–0.860), respectively (Figure 4C and Table 2). While the AUC value of the NIHSS score model was 0.623 (95% CI: 0.542–0.696). The DeLong test revealed significantly differences between the PSENet and all clinical models (P<0.05, Figure 4D). Figure 5 visually represents the comparative performance of different models through a confusion matrix. Figure 6 shows the mean prediction probability histogram for the PSE and non-PSE patients using different models. Notably, the PSENet had the lowest number of incorrect predictions, totaling 140. Additionally, the distribution of the red bars (true negative, label =0) was mostly concentrated within the range of 0.0 to 0.4, indicating that the model had a high accuracy in predicting non-PSE patients. The DCA showed that at a probability threshold of 0.024–0.64, the PSENet had greater clinical benefits than the baseline clinical model (Figure 7).
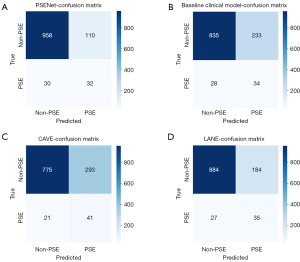
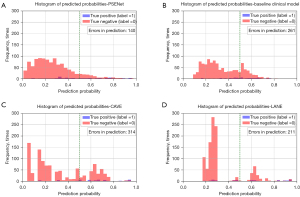
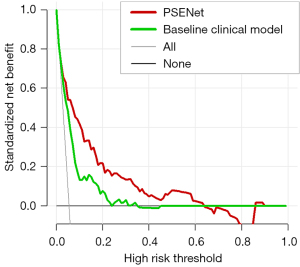
Interpretability of the PSENet
The red-highlighted regions in the Grad-CAM visualization indicated the areas that significantly affected the model’s decision, facilitating a better comprehension of how the model made its predictions or decisions. Representative examples are provided in Figure 8. Two areas were typically valuable in predicting PSE (i.e., the hematoma area and the cortical area) based on the PSENet.
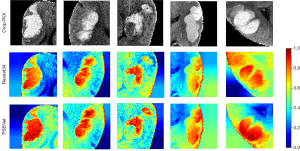
Discussion
The high prevalence of PSE and associated seizures increases the risk of neurological impairments, unfavorable prognoses, extended hospital stays, delayed rehabilitation, and mortality among stroke patients. This study sought to investigate the effectiveness of a deep-learning model in predicting PSE, which was trained and validated using NCCT.
First, the feature importance analysis results showed that ICH volume was the most critical clinical factor for predicting ICH-associated PSE [Figure 9, Supplementary file (Appendix 3)]. A larger ICH volume results in extensive brain tissue damage, triggering abnormal electrical activity in the brain and elevating the risk of seizures. In this study, the nnU-Net enabled the precise segmentation of ICH from normal brain tissue, providing ICH volume measurements comparable to manual delineations performed by radiologists. Further, in the ablation experiments, the combination of FIV and FPSE improved the predictive performance (AUC =0.790, 95% CI: 0.716–0.870, Table 3). Additionally, the ICH locations in patients may influence PSE development. The specific brain regions, particularly the inferior temporal gyrus, middle temporal gyrus, and fusiform gyrus, are more susceptible to PSE based on SVR-LSM (40). These regions play key roles in the brain and are closely related to advanced cognitive functions such as language, memory, and emotion. Damage to these areas may intensify abnormal neuronal electrical activity, thereby exacerbating the development of PSE.
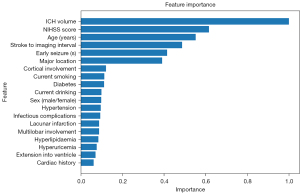
Second, comparing different convolutional neural networks, we found that these models have the potential to predict the occurrence of PSE in ICH patients. Notably, the risk of developing PSE is higher when ICH affects cortical areas, as cortical regions have a lower seizure threshold and can easily trigger abnormal neuronal discharges (41-43). In this study, the predictive performance of the PSENet was enhanced by automatically identifying and incorporating deep-learning FCI, such that the AUC increased from 0.782 to 0.819. Further, when we combined the FCI, the FIV and FPSE, even more significant results were achieved, with the AUC reaching 0.840 (95% CI: 0.792–0.898).
Third, comparisons were made with clinical prediction models that only included clinical features. In a thorough assessment of all metrics, the PSENet consistently outperformed all the clinical models, highlighting the superior capabilities of deep learning in capturing intricate patterns and nuances within the data.
Previous research has used radiomics for PSE prediction, showcasing its advantages over clinical models (26). However, manually crafting features involves time-consuming hematoma region segmentation. Deep learning can address such limitations. For example, the nnU-Net can successfully achieve the automatic segmentation of ICH, reducing the laborious task of manual segmentation and minimizing subjective biases, thereby enhancing efficiency (44). Further, the PSENet was trained in an end-to-end manner, directly extracting highly representative PSE-related features from the cropped ROI without the need for precise hematoma delineation. This direct prediction approach eliminates the four complex steps involved in feature engineering for radiomics (i.e., manual segmentation, feature extraction, feature selection, and model building). Both the cropped ROI based on the nnU-Net segmentation results and ground truth achieved similar AUC values in predicting PSE, and the Delong test showed no significant difference between these two types of input data. Finally, the PSENet can automatically identify and incorporate features that indicate whether the brain hemorrhage has extended into the cortex, which is critical for predicting PSE and determining appropriate treatment strategies.
This study had some limitations. First, it was a single-center study, which limits the generalizability of the results. Therefore, future research should be conducted with larger sample sizes, incorporating patients from diverse centers and foreign-born populations to enhance the robustness and generalizability of the results. Second, the number of PSE cases in our dataset was relatively small, with an incidence rate of approximately 5%, which might have biased the model towards the majority class (non-PSE cases) during training, thereby compromising its predictive performance in relation to the minority class (PSE cases). Measures were implemented to address this class imbalance, but these measures could not completely eliminate this imbalance. In the future, we intend to gather more extensive and comprehensive data to improve the generalizability and accuracy of the model. Further, we will explore more effective data augmentation and class imbalance handling techniques to overcome the limitations of the current dataset. Third, as this was a retrospective study, the prevalence of PSE might have been underestimated. Notably, only patients with motor semiology compatible with focal motor epileptic seizures were diagnosed with PSE, and those with equivocal non-motor symptoms were not so diagnosed, which could have had various etiologies. Therefore, future well-designed prospective studies should include EEG monitoring.
Conclusions
We established an automated deep-learning approach, the PSENet, to accurately predict the development of PSE. Our model could be seamlessly integrated with existing Hospital Information Systems and Picture Archiving and Communication Systems, thus facilitating the automated acquisition and in-depth analysis of NCCT imaging data. The integration of our model could also facilitate the identification of patients at high risk of developing PSE. However, the implementation of this model in clinical practice still faces complex challenges, including those related to ensuring data security and privacy, and those related to gaining the acceptance of and training doctors and technicians. In addition, the performance of the model may vary among different medical institutions and patient populations; thus multi-center validation and continuous optimization are required to ensure the broad applicability and reliability of the model.
Acknowledgments
None.
Footnote
Reporting Checklist: The authors have completed the TRIPOD + AI reporting checklist. Available at https://qims.amegroups.com/article/view/10.21037/qims-24-1345/rc
Funding: This study was supported by
Conflicts of Interest: All authors have completed the ICMJE uniform disclosure form (available at https://qims.amegroups.com/article/view/10.21037/qims-24-1345/coif). The authors have no conflicts of interest to declare.
Ethical Statement: The authors are accountable for all aspects of the work in ensuring that questions related to the accuracy or integrity of any part of the work are appropriately investigated and resolved. This study was approved by the Ethics Committee of The First Affiliated Hospital of Wenzhou Medical University (No. 2020–185). The study was conducted in accordance with the Declaration of Helsinki (as revised in 2013). The subjects or their legally authorized representatives provided verbal informed consent through telephone interviews; the requirement of written informed consent was waived for all participants.
Open Access Statement: This is an Open Access article distributed in accordance with the Creative Commons Attribution-NonCommercial-NoDerivs 4.0 International License (CC BY-NC-ND 4.0), which permits the non-commercial replication and distribution of the article with the strict proviso that no changes or edits are made and the original work is properly cited (including links to both the formal publication through the relevant DOI and the license). See: https://creativecommons.org/licenses/by-nc-nd/4.0/.
References
- Fisher RS, Acevedo C, Arzimanoglou A, Bogacz A, Cross JH, Elger CE, Engel J Jr, Forsgren L, French JA, Glynn M, Hesdorffer DC, Lee BI, Mathern GW, Moshé SL, Perucca E, Scheffer IE, Tomson T, Watanabe M, Wiebe S. ILAE official report: a practical clinical definition of epilepsy. Epilepsia 2014;55:475-82. [Crossref] [PubMed]
- Neri S, Gasparini S, Pascarella A, Santangelo D, Cianci V, Mammì A, Lo Giudice M, Ferlazzo E, Aguglia U. Epilepsy in Cerebrovascular Diseases: A Narrative Review. Curr Neuropharmacol 2023;21:1634-45. [Crossref] [PubMed]
- Rossi C, De Herdt V, Dequatre-Ponchelle N, Hénon H, Leys D, Cordonnier C. Incidence and predictors of late seizures in intracerebral hemorrhages. Stroke 2013;44:1723-5. [Crossref] [PubMed]
- Doria JW, Forgacs PB. Incidence, Implications, and Management of Seizures Following Ischemic and Hemorrhagic Stroke. Curr Neurol Neurosci Rep 2019;19:37. [Crossref] [PubMed]
- Zelano J, Holtkamp M, Agarwal N, Lattanzi S, Trinka E, Brigo F. How to diagnose and treat post-stroke seizures and epilepsy. Epileptic Disord 2020;22:252-63. [Crossref] [PubMed]
- Tanaka T, Ihara M. Post-stroke epilepsy. Neurochem Int 2017;107:219-28. [Crossref] [PubMed]
- Lin R, Yu Y, Wang Y, Foster E, Kwan P, Lin M, Xia N, Xu H, Xie C, Yang Y, Wang X. Risk of Post-stroke Epilepsy Following Stroke-Associated Acute Symptomatic Seizures. Front Aging Neurosci 2021;13:707732. [Crossref] [PubMed]
- Mafla-Mendoza AP, Paredes-Urbano ED, Gea-Izquierdo E. Risk Factors Associated with Epilepsy Related to Cerebrovascular Disease: A Systematic Review and Meta-Analysis. Neuropsychiatr Dis Treat 2023;19:2841-56. [Crossref] [PubMed]
- Chi NF, Kuan YC, Huang YH, Chan L, Hu CJ, Liu HY, Chiou HY, Chien LN. Development and validation of risk score to estimate 1-year late poststroke epilepsy risk in ischemic stroke patients. Clin Epidemiol 2018;10:1001-11. [Crossref] [PubMed]
- Chen TC, Chen YY, Cheng PY, Lai CH. The incidence rate of post-stroke epilepsy: a 5-year follow-up study in Taiwan. Epilepsy Res 2012;102:188-94. [Crossref] [PubMed]
- Yamada S, Nakagawa I, Tamura K, Nishimura F, Motoyama Y, Park YS, Nakase H. Investigation of poststroke epilepsy (INPOSE) study: a multicenter prospective study for prediction of poststroke epilepsy. J Neurol 2020;267:3274-81. [Crossref] [PubMed]
- Tanaka T, Ihara M, Fukuma K, Mishra NK, Koepp MJ, Guekht A, Ikeda A. Pathophysiology, Diagnosis, Prognosis, and Prevention of Poststroke Epilepsy: Clinical and Research Implications. Neurology 2024;102:e209450. [Crossref] [PubMed]
- Haapaniemi E, Strbian D, Rossi C, Putaala J, Sipi T, Mustanoja S, Sairanen T, Curtze S, Satopää J, Roivainen R, Kaste M, Cordonnier C, Tatlisumak T, Meretoja A. The CAVE score for predicting late seizures after intracerebral hemorrhage. Stroke 2014;45:1971-6. [Crossref] [PubMed]
- Wang Y, Li Z, Zhang X, Chen Z, Li D, Chen W, Gu J, Sun D, Rong T, Kwan P. Development and validation of a clinical score to predict late seizures after intracerebral hemorrhage in Chinese. Epilepsy Res 2021;172:106600. [Crossref] [PubMed]
- Chen G, Li Q, Shi F, Rekik I, Pan Z. RFDCR: Automated brain lesion segmentation using cascaded random forests with dense conditional random fields. Neuroimage 2020;211:116620. [Crossref] [PubMed]
- Esteva A, Kuprel B, Novoa RA, Ko J, Swetter SM, Blau HM, Thrun S. Dermatologist-level classification of skin cancer with deep neural networks. Nature 2017;542:115-8. [Crossref] [PubMed]
- Hu J, Heidari AA, Zhang L, et al. Chaotic diffusion-limited aggregation enhanced grey wolf optimizer: Insights, analysis, binarization, and feature selection. International Journal of Intelligent Systems 2021;37:4864-927. [Crossref]
- Hu J, Gui W, Heidari AA, et al. Dispersed foraging slime mould algorithm: Continuous and binary variants for global optimization and wrapper-based feature selection. Knowledge-Based Systems 2022;237. [Crossref]
- Pan Z, Chen H, Ye Y, et al. Enhanced Moth-flame Optimizer with Quasi-Reflection and Refraction Learning with Application to Image Segmentation and Medical Diagnosis. Current Bioinformatics 2023;18:109-42. [Crossref]
- Xia J, Zhang H, Li R, et al. Generalized Oppositional Moth Flame Optimization with Crossover Strategy: An Approach for Medical Diagnosis. Journal of Bionic Engineering 2021;18:991-1010. [Crossref]
- Pan Z, Lu H, Cheng Q. Activities of daily living and lesion position among multiple sclerosis patients by Bayes network. Neural Regen Res 2013;8:1327-36. [Crossref] [PubMed]
- Qiu X, Yan F, Liu H. A difference attention ResNet-LSTM network for epileptic seizure detection using EEG signal. Biomedical Signal Processing and Control 2023;83. [Crossref]
- Lih OS, Jahmunah V, Palmer EE, Barua PD, Dogan S, Tuncer T, García S, Molinari F, Acharya UR. EpilepsyNet: Novel automated detection of epilepsy using transformer model with EEG signals from 121 patient population. Comput Biol Med 2023;164:107312. [Crossref] [PubMed]
- Anita M, Meena Kowshalya A. Automatic epileptic seizure detection using MSA-DCNN and LSTM techniques with EEG signals. Expert Systems with Applications 2024;238. [Crossref]
- Kyung S, Shin K, Jeong H, Kim KD, Park J, Cho K, Lee JH, Hong G, Kim N. Improved performance and robustness of multi-task representation learning with consistency loss between pretexts for intracranial hemorrhage identification in head CT. Med Image Anal 2022;81:102489. [Crossref] [PubMed]
- Lin R, Lin J, Xu Y, Yu Y, Foster E, Lin M, Xu H, Li X, Ye J, Yao F, Xu H, Chen M, Chen J, Zheng H, Li J, Zheng M, Huang S, Zhu S, Yang Y, Wang X. Development and validation of a novel radiomics-clinical model for predicting post-stroke epilepsy after first-ever intracerebral haemorrhage. Eur Radiol 2023;33:4526-36. [Crossref] [PubMed]
- Landman BA, Angelini ED, Erickson BJ, Philbrick KA, Kostandy P, Akkus Z. Extraction of brain tissue from CT head images using fully convolutional neural networks. Medical Imaging 2018: Image Processing 2018:514-20.
- Akkus Z, Kostandy P, Philbrick KA, Erickson BJ. Robust brain extraction tool for CT head images. Neurocomputing 2020;392:189-95. [Crossref]
- Isensee F, Jaeger PF, Kohl SAA, Petersen J, Maier-Hein KH. nnU-Net: a self-configuring method for deep learning-based biomedical image segmentation. Nat Methods 2021;18:203-11. [Crossref] [PubMed]
- Peng J, Kang S, Ning Z, Deng H, Shen J, Xu Y, Zhang J, Zhao W, Li X, Gong W, Huang J, Liu L. Residual convolutional neural network for predicting response of transarterial chemoembolization in hepatocellular carcinoma from CT imaging. Eur Radiol 2020;30:413-24. [Crossref] [PubMed]
- Oord Avd, Li Y, Vinyals O. Representation learning with contrastive predictive coding. arXiv preprint arXiv:180703748 2018.
- Qu W, Balki I, Mendez M, Valen J, Levman J, Tyrrell PN. Assessing and mitigating the effects of class imbalance in machine learning with application to X-ray imaging. Int J Comput Assist Radiol Surg 2020;15:2041-8. [Crossref] [PubMed]
- Sokolova M, Lapalme G. A systematic analysis of performance measures for classification tasks. Information Processing & Management 2009;45:427-37. [Crossref]
- Selvaraju RR, Cogswell M, Das A, Vedantam R, Parikh D, Batra D. Grad-CAM: Visual Explanations from Deep Networks via Gradient-Based Localization. International Journal of Computer Vision 2019;128:336-59. [Crossref]
- Du Tran, Lubomir Bourdev, Rob Fergus, Lorenzo Torresani, Paluri M. Learning Spatiotemporal Features with 3D Convolutional Networks. Proceedings of the IEEE International Conference on Computer Vision 2015:4489-97.
- Krizhevsky A, Sutskever I, Hinton GE. ImageNet classification with deep convolutional neural networks. Communications of the ACM 2017;60:84-90. [Crossref]
- Liu Y, Zhang Y, Wang Y, Hou F, Yuan J, Tian J, Zhang Y, Shi Z, Fan J, He Z. A Survey of Visual Transformers. IEEE Trans Neural Netw Learn Syst 2024;35:7478-98. [Crossref] [PubMed]
- Huang G, Liu Z, van der Maaten L, Weinberger KQ. Densely Connected Convolutional Networks. Proceedings of the IEEE Conference on Computer Vision and Pattern Recognition 2017:4700-8.
- Howard AG, Zhu M, Chen B, Kalenichenko D, Wang W, Weyand T, Andreetto M, Adam H. MobileNets Efficient Convolutional Neural Networks for Mobile Vision. arXiv preprint 2017;arXiv:1704.04861.
- Dziadkowiak E, Guziński M, Chojdak-Łukasiewicz J, Wieczorek M, Paradowski B. Predictive factors in post-stroke epilepsy: Retrospective analysis. Adv Clin Exp Med 2021;30:29-34. [Crossref] [PubMed]
- Zou S, Wu X, Zhu B, Yu J, Yang B, Shi J. The pooled incidence of post-stroke seizure in 102 008 patients. Top Stroke Rehabil 2015;22:460-7. [Crossref] [PubMed]
- Zhang C, Wang X, Wang Y, Zhang JG, Hu W, Ge M, Zhang K, Shao X. Risk factors for post-stroke seizures: a systematic review and meta-analysis. Epilepsy Res 2014;108:1806-16. [Crossref] [PubMed]
- Lee SH, Aw KL, Banik S, Myint PK. Post-stroke seizure risk prediction models: a systematic review and meta-analysis. Epileptic Disord 2022;24:302-14. [Crossref] [PubMed]
- Wang S, Shi J, Ye Z, Dong D, Yu D, Zhou M, Liu Y, Gevaert O, Wang K, Zhu Y, Zhou H, Liu Z, Tian J. Predicting EGFR mutation status in lung adenocarcinoma on computed tomography image using deep learning. Eur Respir J 2019;53:1800986. [Crossref] [PubMed]