Association of decreased visibility on deep medullary vein gray-matter volume mediated by increased extracellular fluid in the white matter of patients with cerebral small vessel disease
Introduction
Atrophy is a common imaging hallmark of cerebral small vessel disease (CSVD) (1) and is correlated to cognitive impairment (2). However, the underlying mechanisms driving its occurrence and progression remain unclear, presenting challenges for the prevention and management of brain atrophy.
Diffusion tensor imaging (DTI) with a bicompartmental model has emerged as a novel method for assessing extracellular fluid (ECF) through the metric of free water (FW) (3,4), providing a sensitive marker for microstructural changes associated with CSVD. FW specifically reflects the extracellular environment and correlates strongly with clinical status in patients with CSVD (5). Notably, FW is sensitive to microstructural changes in normal-appearing white matter (NAWM) (6) unlike other diffusion metrics of fractional anisotropy (5).
DTI, combined with advancements in magnetic resonance imaging (MRI) techniques, particularly susceptibility-weighted imaging (SWI), has led to increased recognition of associations between changes in cerebral venous structures (7,8) and the development of CSVD. Several studies have shown that the visibility and signal continuity of deep medullary veins (DMVs) on SWI images can reflect the condition of DMV lumens or oxygen metabolism and are correlated with the severity of CSVD (9,10). Furthermore, research indicates that the dysfunction of the DMV can elevate venous pressure, leading to interstitial edema with increased ECF and the progression of white-matter hyperintensities (WMHs) (11). Liu et al. (12) demonstrated that DMV dysfunction in patients with CSVD is widespread and contributes to microstructural brain damage, including gray-matter atrophy. Moreover, Mao et al. (13) found that decreased visibility of DMV on SWI images were independently associated with gray-matter loss. However, the precise mechanisms by which DMV dysfunction leads to gray-matter atrophy remain unclear. Building upon these findings, our study further examined the relationship between DMV scores, NAWM FW, and brain gray-matter volume using multimodal MRI. We present this article in accordance with the STROBE reporting checklist (available at https://qims.amegroups.com/article/view/10.21037/qims-24-957/rc).
Methods
Participants
This secondary exploratory analysis was approved by Tongde Hospital of Zhejiang Province (No. 2022-42-JY) and was conducted in accordance with the Declaration of Helsinki (as revised in 2013). All participants provided informed consent. We collected the clinical and multimodal MRI data of patients diagnosed with CSVD in 2022. Inclusion criteria included individuals aged 40 years and above, MRI scans demonstrating typical CSVD imaging markers according to neuroimaging standards for research into small vessel disease (1), and at least one vascular risk factor. The exclusion criteria included the presence of image artifacts affecting crucial imaging parameters, severe stenosis or occlusion of intracranial or cervical vessels, clear evidence of alternative causes for brain demyelinating lesions such as infection or genetic factors, coexisting intracranial pathologies including stroke [excluding old lacunar infarcts (LI)], trauma, tumor, or vascular malformation as well as concurrent heart, lung, or kidney dysfunction. To better reflect the relationship between DMV score, FW in NAWM and gray matter (GM) atrophy in different severities of CSVD, we included patients with varying levels of CSVD burden, including those with mild CSVD (total CSVD MR score of 0). This approach allows us to explore the changes in these relationships across a broader spectrum of CSVD severity, as shown in Table 1, where the median CSVD burden is 1 (IQR, 0–2). Figure 1 presents a flowchart detailing the enrollment of study participants.
Table 1
Variable | Value |
---|---|
n | 123 |
Male, n (%) | 57 (46.3) |
Age, years, mean ± SD | 60±12 |
Hypertension, n (%) | 67 (54.5) |
Diabetes mellitus, n (%) | 22 (17.9) |
Current smoking, n (%) | 27 (22.0) |
Hyperlipidemia, n (%) | 31 (25.2) |
DMV score, median (IQR) | 3 (1, 7) |
LI, n (%) | 48 (39.0) |
CMB, n (%) | 27 (22.0) |
WMH volume, mL, mean ± SD | 11.97±11.36 |
PWMH Fazekas score, median (IQR) | 1 (1, 2) |
DWMH Fazekas score, median (IQR) | 1 (1, 2) |
CSVD burden, median (IQR) | 1 (0, 2) |
NAWM free water, median (IQR) | 0.23 (0.22, 0.24) |
GM volume, mL, mean ± SD | 309.27±31.67 |
Whole-brain volume, mL, mean ± SD | 1,344.69±135.39 |
GM_N, median (IQR) | 0.27 (0.26, 0.29) |
SD, standard deviation; DMV, deep medullary vein; IQR, interquartile range; LI, lacunar infarcts; CMB, cerebral microbleed; WMH, white-matter hyperintensity; PWMH, periventricular WMH; DWMH, deep WMH; CSVD, cerebral small vessel disease; NAWM, normal-appearing white matter; GM, gray matter; GM_N, normalized gray-matter volume.
MRI protocol
All participants underwent comprehensive imaging assessments with a 1.5-T MRI scanner (Siemens Healthineers, Erlangen, Germany). The imaging protocols encompassed a variety of sequences, including standard sequences [T1-weighted imaging (T1WI), T2-weighted imaging (T2WI), and T2 fluid-attenuated inversion recovery (FLAIR)], as well as 3D-T1WI, time-of-flight magnetic resonance angiography, SWI, and DTI. The specific parameters for 3D T1WI were a repetition time (TR) of 2,000 ms, an echo time (TE) of 2.84 ms, a 1-mm slice thickness (SLT), and a 256×256 matrix. For T2 FLAIR, parameters included a TR of 3,800 ms, a TE of 88 ms, a 5-mm SLT, and a 256×256 matrix. The SWI settings were a TR of 27 ms, a TE of 20 ms, a flip angle of 10°, a 2-mm SLT, and a 512×512 matrix. For DTI, the parameters included a TR of 3,600 ms, a TE of 95 ms, a 3-mm SLT, a 128×128 matrix, and b values of 0, 1,000, and 2,000 s/mm2, with 30 diffusion directions for each b value.
Imaging analyses
CSVD evaluation
We assessed WMH, cerebral microbleeds (CMBs), perivascular spaces (PVS), and LI following the STRIVE guidelines. WMHs were characterized by abnormal hyperintensities observed in periventricular or deep white matter on T2 FLAIR images. Its severity was graded using the Fazekas system, in which high-grade WMH (HWMH) is indicated by a Fazekas score of 2 higher in either the periventricular WMH (PWMH) or deep WMH (DWMH). CMBs were identified as homogeneous hypointense lesions measuring 3 to 5 mm identified on SWI following the exclusion of calcification, abnormal iron deposits, and vascular cross-sections. Enlarged PVS were defined as small dot-like or linear fluid signals on MR images, with high-grade PVS (HPVS) indicating more than 10 enlarged PVSs in the unilateral basal ganglia. LI was considered to be to round or ovoid lesions measuring 3 to 15 mm, appearing hyperintense on T2WI and hypointense on T1WI.
The total CSVD burden was evaluated on a scale of 0 to 4 based on the presence (score of 1) or absence (score of 0) of HWMH, CMB, HPVS, and LI. For example, a patient with all four features (CMB, HWMH, HPVS, and LI) would have a total score of 4.
DMV score
The DMV score was reviewed by two seasoned neuroradiologists (Z.X. and M.Y.), both with over 5 years of experience in neuroimaging. Both radiologists were blinded to other imaging and clinical data to avoid bias. In cases in which there was disagreement between the two initial neuroradiologists, a third experienced neuroradiologist (S.C.) was consulted. The three radiologists then discussed the case together to reach a consensus on the final DMV score. The assessment of DMV score was conducted using SWI images, with five periventricular slices selected starting from the level just above the basal ganglia. DMV visibility on SWI images served as the basis for scoring. Initially, six regions were delineated according to the anatomical distribution of DMV (14), encompassing the bilateral frontal, parietal, and occipital lobes (see Figure 2). Subsequently, each of these six regions underwent individual scoring for the visibility of DMV. The criteria used for scoring DMV visibility were delineated as follows (15) (refer to Figure 3 for details): 0 points for significantly clear visibility, 1 point for clear visibility with at least one discontinuity; 2 points for faintly discernible, unclear visibility; and 3 points for an entirely invisible DMV. Finally, the scores from the six regions were aggregated to derive the patient’s DMV score, which could range between 0 and 18 points. A higher DMV score reflected poorer visibility.
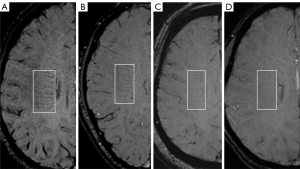
Normalized gray-matter volume
Normalized gray-matter volume (GM_N) was used to quantify the volume of GM in the brain, defined as the ratio of gray-matter volume to intracranial volume (16). Initially, 3D T1WI sequence images were converted from the Digital Imaging and Communications in Medicine (DICOM) format to the Neuroimaging Informatics Technology Initiative (NIfTI) format. Subsequently, the fsl_anat (https://fsl.fmrib.ox.ac.uk/fsl/fslwiki/fsl_anat) functionality within the FSL software was employed to segment the GM, including both cortical and deep GM, and white matter and to generate an intracranial mask (see Figure 4). Finally, GM and intracranial volumes were computed using these masks to derive GM_N.
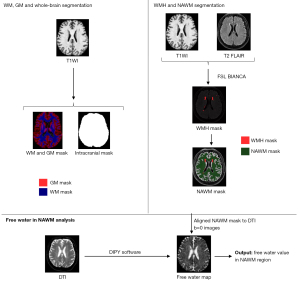
WMH volume and NAWM mask
The BIANCA algorithm was trained on a dataset of 390 cases with imaging protocols that included 3D T1WI, T2 FLAIR, and manually segmented WMH masks. The WMH volume was then determined using the BIANCA (17) function within the FSL software (https://fsl.fmrib.ox.ac.uk/fsl/fslwiki/BIANCA) for the automated segmentation of WMH from T2 FLAIR images under a threshold of 0.90 for the WMH mask. The resulting WMH mask was then manually checked, verified, and edited, if necessary, which was followed by the quantification of WMH volume.
Subsequently, both the WMH mask and white matter masks were independently aligned with the DTI b=0 s/mm2 images, which served as the reference space, using the FSL FLIRT (https://fsl.fmrib.ox.ac.uk/fsl/fslwiki/FLIRT) function in FSL software. Following this, the NAWM mask was derived by subtracting the WMH mask from the white-matter mask, thereby enabling the computation of NAWM FW.
FW in NAWM
Initially, DTI images underwent preprocessing using Mrtrix3 (18), including tasks such as elimination of Gibbs artifacts, denoising, repair of echo planar imaging distortions, and correction for eddy currents. Following this, DIPY software (19) was used to implement a bicompartmental model (4) that integrated ECF and tissue compartments, thereby generating maps of FW. Finally, NAWM FW was computed using the NAWM mask to depict the ECF content within the NAWM.
Statistical analyses
The statistical analysis was conducted using SPSS 20.0 software (IBM Corp., Armonk, NY, USA). Qualitative data are as expressed as counts and frequencies, while quantitative data are presented as the mean and standard deviation (SD) or as the median and interquartile range (IQR). The relationship between DMV score, NAWM FW, and GM_N was examined via Spearman correlation analysis. General linear model analysis was performed to identify the factors influencing GM_N. Subsequently, mediation analysis was conducted using PROCESS (created by Preacher and Hayes) Model 4, with 95% CIs and 5,000 bootstrap samples. In this analysis, GM_N was the primary outcome, with NAWM FW serving as the mediator and DMV score as the predictor (Figure 5). Statistical significance was determined at a threshold of P<0.05.
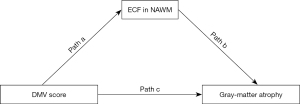
Results
The study enrolled 123 patients diagnosed with CSVD (Figure 1), whose comprehensive baseline information is detailed in Table 1. The mean volume of WMH was 11.97±11.36 mL. The median DMV score was 3 (IQR, 1–7), while for NAWM FW and GM_N, the median was 0.23 (IQR, 0.22–0.24) and 0.27 (IQR, 0.26–0.29), respectively.
Interreader agreement in evaluation of DMV score
The intraclass correlation coefficient (ICC) for interreader reliability of the DMV score on SWI was 0.92, indicating excellent agreement between the readers.
Relationship between DMV score and FW in NAWM
In the Spearman correlation analysis, a positive correlation was observed between DMV score and FW in NAWM (r=0.603; P<0.001; Figure 6A). General linear model analysis indicated an independent association between DMV score and FW in NAWM in model 1 (β=0.656; 95% CI: 0.521–0.790; P<0.001; Table 2). In model 2, after adjustments were made for age, gender, and vascular risk factors, the relationship between DMV score and FW in NAWM remained significant. This association persisted in model 3, which further controlled for normalized WMH volume and CSVD burden.
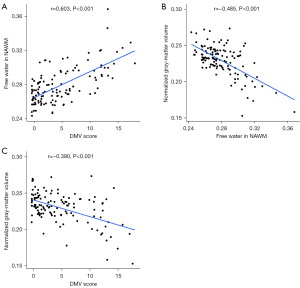
Table 2
Variable | Model 1 | Model 2 | Model 3 | |||||
---|---|---|---|---|---|---|---|---|
β (95% CI) | P value | β (95% CI) | P value | β (95% CI) | P value | |||
Male | 0.140 (0.012, 0.267) | 0.032 | 0.129 (0.004, 0.255) | 0.043 | ||||
Age | 0.489 (0.353, 0.625) | <0.001 | 0.402 (0.255, 0.549) | <0.001 | ||||
Hypertension | 0.020 (−0.099, 0.139) | 0.739 | 0.006 (−0.112, 0.124) | 0.925 | ||||
Diabetes mellitus | 0.033 (−0.078, 0.144) | 0.555 | 0.051 (−0.058, 0.160) | 0.353 | ||||
Current smoking | 0.035 (−0.094, 0.163) | 0.592 | 0.054 (−0.073, 0.180) | 0.404 | ||||
Hyperlipidemia | −0.053 (−0.164, 0.058) | 0.349 | −0.051 (−0.160, 0.059) | 0.360 | ||||
DMV score | 0.656 (0.521, 0.790) | <0.001 | 0.389 (0.266, 0.512) | <0.001 | 0.336 (0.210, 0.462) | <0.001 | ||
Normalized WMH volume | 0.068 (−0.077, 0.213) | 0.353 | ||||||
CSVD burden | 0.141 (−0.019, 0.301) | 0.084 |
Model 1: FW in NAWM served as the primary outcome, DMV score as the predictor. Model 2: Control for age, gender, and vascular risk factors. Model 3: Control for age, gender, vascular risk factors, normalized WMH volume, and CSVD burden. DMV, deep medullary vein; FW, free water; NAWM, normal-appearing white matter; CI, confidence interval; WMH, white matter hyperintensity; CSVD, cerebral small vessel disease.
Relationship between FW in NAWM and GM_N
FW in NAWM exhibited a negative correlation with GM_N according to Spearman correlation analysis (r=−0.485; P<0.001; Figure 6B). General linear model analysis revealed an independent association between FW in NAWM and GM_N in model 1 (β=−0.630; 95% CI: −0.769 to −0.491; P<0.001) (Table 3). In model 2, after adjustments were made for age, gender, and vascular risk factors, this relationship remained significant. Additionally, in model 3, which controlled for age, gender, vascular risk factors, normalized WMH volume, and CSVD burden, the association continued to be significant.
Table 3
Variable | Model 1 | Model 2 | Model 3 | |||||
---|---|---|---|---|---|---|---|---|
β (95% CI) | P value | β (95% CI) | P value | β (95% CI) | P value | |||
Male | −0.053 (−0.224, 0.117) | 0.538 | −0.055 (−0.226, 0.117) | 0.531 | ||||
Age | 0.015 (−0.199, 0.228) | 0.893 | 0.041 (−0.182, 0.263) | 0.718 | ||||
Hypertension | 0.133 (−0.023, 0.290) | 0.094 | 0.137 (−0.022, 0.297) | 0.091 | ||||
Diabetes mellitus | −0.042 (−0.187, 0.103) | 0.567 | −0.053 (−0.200, 0.095) | 0.481 | ||||
Current smoking | −0.030 (−0.199, 0.138) | 0.722 | −0.041 (−0.213, 0.130) | 0.634 | ||||
Hyperlipidemia | −0.041 (−0.188, 0.105) | 0.576 | −0.039 (−0.187, 0.109) | 0.605 | ||||
FW in NAWM | −0.630 (−0.769, −0.491) | <0.001 | −0.656 (−0.864, −0.448) | <0.001 | −0.619 (−0.842, −0.395) | <0.001 | ||
Normalized WMH | −0.046 (−0.242, 0.149) | 0.640 | ||||||
CSVD burden | −0.049 (−0.268, 0.169) | −0.268 |
Model 1: GM_N served as the primary outcome, FW in NAWM as the predictor. Model 2: Control for age, gender, and vascular risk factors. Model 3: Control for age, gender, vascular risk factors, normalized WMH volume, and CSVD burden. FW, free water; NAWM, normal-appearing white matter; GM_N, normalized gray-matter volume; CI, confidence interval; WMH, white-matter hyperintensity; CSVD, cerebral small vessel disease.
Relationship between DMV score and GM_N
Spearman correlation analysis indicated a negative correlation between DMV score and GM_N (r=−0.390; P<0.001; Figure 6C). General linear model analysis demonstrated an independent association between DMV score and GM_N in model 1 (β=−0.502; 95% CI: −0.657 to −0.348; P<0.001; Table 4). In model 2, after adjustments were made for age, gender, and vascular risk, the association between DMV score and GM_N remained significant. Furthermore, in model 3, in which normalized WMH volume and CSVD burden were also accounted for, the association remained significant.
Table 4
Variable | Model 1 | Model 2 | Model 3 | |||||
---|---|---|---|---|---|---|---|---|
β (95% CI) | P value | β (95% CI) | P value | β (95% CI) | P value | |||
Male | −0.142 (−0.322, 0.037) | 0.119 | −0.134 (−0.314, 0.045) | 0.141 | ||||
Age | −0.241 (−0.432, −0.050) | 0.014 | −0.167 (−0.377, 0.044) | 0.119 | ||||
Hypertension | 0.121 (−0.046, 0.288) | 0.155 | 0.132 (−0.037, 0.301) | 0.126 | ||||
Diabetes mellitus | −0.073 (−0.228, 0.083) | 0.356 | −0.088 (−0.245, 0.068) | 0.264 | ||||
Current smoking | −0.050 (−0.230, 0.130) | 0.583 | −0.067 (−0.249, 0.115) | 0.466 | ||||
Hyperlipidemia | −0.005 (−0.162, 0.151) | 0.946 | −0.006 (−0.163, 0.151) | 0.938 | ||||
DMV score | −0.502 (−0.657, −0.348) | <0.001 | −0.384 (−0.557, −0.211) | <0.001 | −0.339 (−0.519, −0.158) | <0.001 | ||
Normalized WMH | −0.068 (−0.276, 0.139) | 0.516 | ||||||
CSVD burden | −0.110 (−0.340, 0.120) | 0.345 |
Model 1: GM_N served as the primary outcome, DMV score as the predictor. Model 2: Control for age, gender, vascular risk factors. Model 3: Control for age, gender, vascular risk factors, normalized WMH volume, and CSVD burden. DMV, deep medullary vein; GM_N, normalized gray-matter volume; CI, confidence interval; WMH, white matter hyperintensity; CSVD, cerebral small vessel disease.
Mediation analyses of DMV score, FW in NAWM, and GM_N
In model 1, GM_N served as the primary outcome, with NAWM FW acting as the mediator and DMV score as the predictor. Mediation analysis indicated a notable indirect effect of FW in NAWM on the association between DMV score and GM_N (β=−0.346; 95% CI: −0.534 to −0.187; P<0.001; Table 5). After adjustments were made for potential confounding factors such as age, gender, and vascular risk factors, the indirect effect remained significant (P<0.001). This mediating effect was also observed when age, gender, vascular risk factors, normalized WMH volume, and CSVD burden were controlled for (P<0.001).
Table 5
Model | Path a | Path b | Path c-direct effect | Path ab-indirect effect |
---|---|---|---|---|
Model 1 | 0.656 (0.521, 0.791)* | −0.528 (−0.710, −0.345)* | −0.156 (−0.339, 0.026) | −0.346 (−0.534, −0.187)* |
Model 2 | 0.389 (0.266, 0.512)* | −0.545 (−0.782, −0.307)* | −0.172 (−0.357, 0.013) | −0.212 (−0.357, −0.098)* |
Model 3 | 0.336 (0.210, 0.462)* | −0.524 (−0.771, −0.277)* | −0.163 (−0.351, 0.026) | −0.176 (−0.329, −0.076)* |
Data are presented as β (95% CI). Model 1: GM_N served as the primary outcome, with NAWM FW acting as the mediator and DMV score as the predictor. Model 2: Control for age, gender, and vascular risk factors. Model 3: Control for age, gender, vascular risk factors, normalized WMH volume, and CSVD burden. *, P<0.05. DMV, deep medullary vein; FW, free water; NAWM, normal-appearing white matter; GM_N, normalized gray-matter volume; CI, confidence interval; WMH, white matter hyperintensity; CSVD, cerebral small vessel disease.
Discussion
This study identified a correlation between DMV score and GM_N in patients with CSVD that was mediated by FW in the NAWM region. Furthermore, this association remained independent of gender, age, vascular risk factors, WMH volume, and CSVD burden. In essence, more pronounced DMV disruption corresponded to higher ECF content in NAWM and smaller gray-matter volume. Thus, we posit a relationship between DMV disruption, NAWM region FW, and gray-matter loss in patients with CSVD.
Several studies have demonstrated a link between reduced DMV visibility and CSVD (12,20,21). The underlying mechanisms include the following: (I) as indicated in postmortem studies, collagen deposition in DMV vessel walls causes luminal narrowing, which is a key pathological basis for CSVD occurrence and development (9,22). (II) Patients with CSVD experience changes in hypoperfusion and hypometabolism, resulting in relatively low oxygen extraction, leading to increased DMV score or reduced visibility (23). Furthermore, DMV scoring has shown high reproducibility with interrater reliability consistent across studies (15,24), including our study, supporting its reliability as a CSVD metric. Regardless of the mechanism, both phenomena can increase ECF in affected areas. Several studies have also confirmed that increased ECF in brain white matter is associated with CSVD presence and severity, as well as cognitive impairment (6,11,25).
Increased ECF in brain white matter can hinder the clearance of brain metabolites, leading to the accumulation of toxic substances. This can directly damage brain nerve fiber bundles and induce neuroinflammatory reactions, exacerbating ECF accumulation in a vicious cycle. Neuroinflammatory reactions also damage nerve fiber bundles, impacting both myelin and axons (26,27).
As a consequence, this damage to nerve fiber bundles can further lead to gray-matter loss through various mechanisms. First, it can increase oxidative stress, triggering inflammatory reactions and cell apoptosis, resulting in gradual gray-matter atrophy (28,29). Second, glial cells in white matter play a crucial role in supporting and protecting neurons (30). When nerve fiber bundles are damaged, glial cells are also affected and are unable to provide sufficient support and protection, thus accelerating neuronal gray-matter atrophy. Finally, demyelination hinders the absorption and utilization of nutrients by neurons, leading to malnutrition-induced neuronal gray-matter atrophy. In summary, disruption of the DMV can lead to edema, which results in increased ECF. This accumulation of toxic substances may trigger neuroinflammation in NAWM. As a consequence, the microenvironment surrounding nerve fiber bundles changes, leading to a cascade of reactions, including increased oxidative stress and damage to glial cells. This, in turn, results in inflammatory responses and nutritional deficits in neuronal cells.
Some studies suggest that WMH volume is one of the factors mediating GM loss (31,32). Our study revealed an independent correlation between DMV score and gray-matter volume after adjustments were made for WMH volume, while WMH volume was not correlated. Considering recent research on cerebral microcirculation and microstructure in CSVD, we propose that WMH may be considered one of the common outcomes of various factors, such as DMV disruption, blood-brain barrier disruption, and neuroinflammatory reactions, which lead to increased ECF in brain white matter. In our view, WMH represents one of the common outcomes resulting from DMV disruption through increased ECF.
Additionally, the median DMV was relatively low in our study. We believe this is likely due to the fact that most of the patients with CSVD we selected had a relatively mild condition. As CSVD severity increases, the DMV score tends to be higher. A lower DMV score with increased visibility on SWI may also be one of the reasons for the high interrater agreement.
This study involved a few limitations which should be addressed. First, we employed a single-center design with a sample size. Second, given the cross-sectional design of this study, the observed associations could not establish causality between DMV score, FW, and GM_N. Longitudinal studies are needed to clarify the temporal sequence of these changes and confirm whether DMV disruption leads to increased ECF and subsequent gray-matter loss. Third, the DMV score is based on visual observation and semiquantitative analysis. Future studies using automated segmentation software for quantitative research may yield more reliable results.
Conclusions
We discovered a correlation between DMV score and GM_N in patients with CSVD, which was mediated by FW in the NAWM region. This observation may indicate a venous contribution to the pathogenesis of gray-matter atrophy in CSVD.
Acknowledgments
None.
Footnote
Reporting Checklist: The authors have completed the STROBE reporting checklist. Available at https://qims.amegroups.com/article/view/10.21037/qims-24-957/rc
Funding: This study was supported by
Conflicts of Interest: All authors have completed the ICMJE uniform disclosure form (available at https://qims.amegroups.com/article/view/10.21037/qims-24-957/coif). The authors have no conflicts of interest to declare.
Ethical Statement: The authors are accountable for all aspects of the work in ensuring that questions related to the accuracy or integrity of any part of the work are appropriately investigated and resolved. This study was conducted in accordance with the Declaration of Helsinki (as revised in 2013) and was approved by Tongde Hospital of Zhejiang Province (No. 2022-42-JY). Informed consent was obtained from all individual participants.
Open Access Statement: This is an Open Access article distributed in accordance with the Creative Commons Attribution-NonCommercial-NoDerivs 4.0 International License (CC BY-NC-ND 4.0), which permits the non-commercial replication and distribution of the article with the strict proviso that no changes or edits are made and the original work is properly cited (including links to both the formal publication through the relevant DOI and the license). See: https://creativecommons.org/licenses/by-nc-nd/4.0/.
References
- Duering M, Biessels GJ, Brodtmann A, Chen C, Cordonnier C, de Leeuw FE, et al. Neuroimaging standards for research into small vessel disease-advances since 2013. Lancet Neurol 2023;22:602-18. [Crossref] [PubMed]
- Rizvi B, Narkhede A, Last BS, Budge M, Tosto G, Manly JJ, Schupf N, Mayeux R, Brickman AM. The effect of white matter hyperintensities on cognition is mediated by cortical atrophy. Neurobiol Aging 2018;64:25-32. [Crossref] [PubMed]
- Berger M, Pirpamer L, Hofer E, Ropele S, Duering M, Gesierich B, Pasternak O, Enzinger C, Schmidt R, Koini M. Free water diffusion MRI and executive function with a speed component in healthy aging. Neuroimage 2022;257:119303. [Crossref] [PubMed]
- Pasternak O, Sochen N, Gur Y, Intrator N, Assaf Y. Free water elimination and mapping from diffusion MRI. Magn Reson Med 2009;62:717-30. [Crossref] [PubMed]
- Duering M, Finsterwalder S, Baykara E, Tuladhar AM, Gesierich B, Konieczny MJ, Malik R, Franzmeier N, Ewers M, Jouvent E, Biessels GJ, Schmidt R, de Leeuw FE, Pasternak O, Dichgans M. Free water determines diffusion alterations and clinical status in cerebral small vessel disease. Alzheimers Dement 2018;14:764-74. [Crossref] [PubMed]
- Man S, Chen S, Xu Z, Zhang H, Cao Z. Increased Extracellular Water in Normal-Appearing White Matter in Patients with Cerebral Small Vessel Disease. J Integr Neurosci 2024;23:46. [Crossref] [PubMed]
- Zhang R, Huang P, Jiaerken Y, Wang S, Hong H, Luo X, Xu X, Yu X, Li K, Zeng Q, Wu X, Lou M, Zhang M. Venous disruption affects white matter integrity through increased interstitial fluid in cerebral small vessel disease. J Cereb Blood Flow Metab 2021;41:157-65. [Crossref] [PubMed]
- Li H, Lan Y, Ju R, Zang P. Deep medullary veins as an important imaging indicator of poor prognosis in acute ischemic stroke: a retrospective cohort survey. Quant Imaging Med Surg 2023;13:5141-52. [Crossref] [PubMed]
- Fulop GA, Tarantini S, Yabluchanskiy A, Molnar A, Prodan CI, Kiss T, Csipo T, Lipecz A, Balasubramanian P, Farkas E, Toth P, Sorond F, Csiszar A, Ungvari Z. Role of age-related alterations of the cerebral venous circulation in the pathogenesis of vascular cognitive impairment. Am J Physiol Heart Circ Physiol 2019;316:H1124-40. [Crossref] [PubMed]
- Yin X, Han Y, Cao X, Zeng Y, Tang Y, Ding D, Zhang J. Association of deep medullary veins with the neuroimaging burden of cerebral small vessel disease. Quant Imaging Med Surg 2023;13:27-36. [Crossref] [PubMed]
- Huang P, Zhang R, Jiaerken Y, Wang S, Hong H, Yu W, Lian C, Li K, Zeng Q, Luo X, Yu X, Wu X, Xu X, Zhang M. White Matter Free Water is a Composite Marker of Cerebral Small Vessel Degeneration. Transl Stroke Res 2022;13:56-64. [Crossref] [PubMed]
- Liu ZY, Zhai FF, Ao DH, Han F, Li ML, Zhou L, Ni J, Yao M, Zhang SY, Cui LY, Jin ZY, Zhu YC. Deep medullary veins are associated with widespread brain structural abnormalities. J Cereb Blood Flow Metab 2022;42:997-1006. [Crossref] [PubMed]
- Mao F, Xu Z, Shao M, Xiang X, Zhou X. Deep medullary veins score is associated with atrophy in patients with cerebral small vessel disease. Front Neurol 2024;15:1417805. [Crossref] [PubMed]
- Lee C, Pennington MA, Kenney CM 3rd. MR evaluation of developmental venous anomalies: medullary venous anatomy of venous angiomas. AJNR Am J Neuroradiol 1996;17:61-70. [PubMed]
- Zhang R, Zhou Y, Yan S, Zhong G, Liu C, Jiaerken Y, Song R, Yu X, Zhang M, Lou M. A Brain Region-Based Deep Medullary Veins Visual Score on Susceptibility Weighted Imaging. Front Aging Neurosci 2017;9:269. [Crossref] [PubMed]
- Maller JJ, Anderson RJ, Thomson RH, Daskalakis ZJ, Rosenfeld JV, Fitzgerald PB. Occipital bending in schizophrenia. Aust N Z J Psychiatry 2017;51:32-41. [Crossref] [PubMed]
- Griffanti L, Zamboni G, Khan A, Li L, Bonifacio G, Sundaresan V, Schulz UG, Kuker W, Battaglini M, Rothwell PM, Jenkinson M. BIANCA (Brain Intensity AbNormality Classification Algorithm): A new tool for automated segmentation of white matter hyperintensities. Neuroimage 2016;141:191-205. [Crossref] [PubMed]
- Tournier JD, Smith R, Raffelt D, Tabbara R, Dhollander T, Pietsch M, Christiaens D, Jeurissen B, Yeh CH, Connelly A. MRtrix3: A fast, flexible and open software framework for medical image processing and visualisation. Neuroimage 2019;202:116137. [Crossref] [PubMed]
- Garyfallidis E, Brett M, Amirbekian B, Rokem A, van der Walt S, Descoteaux M, Nimmo-Smith I. Dipy Contributors. Dipy, a library for the analysis of diffusion MRI data. Front Neuroinform 2014;8:8. [Crossref] [PubMed]
- Chen X, Wei L, Wang J, Shan Y, Cai W, Men X, Liu S, Kang Z, Lu Z, Mok VCT, Wu A. Decreased visible deep medullary veins is a novel imaging marker for cerebral small vessel disease. Neurol Sci 2020;41:1497-506. [Crossref] [PubMed]
- Xu Z, Li F, Xing D, Song H, Chen J, Duan Y, Yang B. A Novel Imaging Biomarker for Cerebral Small Vessel Disease Associated With Cognitive Impairment: The Deep-Medullary-Veins Score. Front Aging Neurosci 2021;13:720481. [Crossref] [PubMed]
- Lahna D, Schwartz DL, Woltjer R, Black SE, Roese N, Dodge H, Boespflug EL, Keith J, Gao F, Ramirez J, Silbert LC. Venous Collagenosis as Pathogenesis of White Matter Hyperintensity. Ann Neurol 2022;92:992-1000. [Crossref] [PubMed]
- Kang P, Ying C, Chen Y, Ford AL, An H, Lee JM. Oxygen Metabolic Stress and White Matter Injury in Patients With Cerebral Small Vessel Disease. Stroke 2022;53:1570-9. [Crossref] [PubMed]
- Zhang K, Zhou Y, Zhang W, Li Q, Sun J, Lou M. MRI-visible perivascular spaces in basal ganglia but not centrum semiovale or hippocampus were related to deep medullary veins changes. J Cereb Blood Flow Metab 2022;42:136-44. [Crossref] [PubMed]
- Lan H, Lei X, Xu Z, Chen S, Gong W, Cai Y. New insights in addressing cerebral small vessel disease: Associated with extracellular fluid in white matter. Front Neurosci 2022;16:1042824. [Crossref] [PubMed]
- Pintér P, Alpár A. The Role of Extracellular Matrix in Human Neurodegenerative Diseases. Int J Mol Sci 2022;23:11085. [Crossref] [PubMed]
- Yu X, Yin X, Hong H, Wang S, Jiaerken Y, Zhang F, Pasternak O, Zhang R, Yang L, Lou M, Zhang M, Huang P. Increased extracellular fluid is associated with white matter fiber degeneration in CADASIL: in vivo evidence from diffusion magnetic resonance imaging. Fluids Barriers CNS 2021;18:29. [Crossref] [PubMed]
- Correale J, Ysrraelit MC. Multiple Sclerosis and Aging: The Dynamics of Demyelination and Remyelination. ASN Neuro 2022;14:17590914221118502. [Crossref] [PubMed]
- Gilgun-Sherki Y, Melamed E, Offen D. Oxidative stress induced-neurodegenerative diseases: the need for antioxidants that penetrate the blood brain barrier. Neuropharmacology 2001;40:959-75. [Crossref] [PubMed]
- Nave KA. Myelination and support of axonal integrity by glia. Nature 2010;468:244-52. [Crossref] [PubMed]
- Wang Y, Yang Y, Wang T, Nie S, Yin H, Liu J. Correlation between White Matter Hyperintensities Related Gray Matter Volume and Cognition in Cerebral Small Vessel Disease. J Stroke Cerebrovasc Dis 2020;29:105275. [Crossref] [PubMed]
- Mayer C, Frey BM, Schlemm E, Petersen M, Engelke K, Hanning U, Jagodzinski A, Borof K, Fiehler J, Gerloff C, Thomalla G, Cheng B. Linking cortical atrophy to white matter hyperintensities of presumed vascular origin. J Cereb Blood Flow Metab 2021;41:1682-91. [Crossref] [PubMed]