Combining pelvic floor ultrasonography with deep learning to diagnose anterior compartment organ prolapse
Introduction
Pelvic organ prolapse (POP), which affects many women in middle age and older (1,2), occurs when one or more pelvic organs protrude out of the vagina via the vaginal fascia, leading to problems such as cystocele, uterine prolapse, and rectocele (3), as well as urinary incontinence, fecal incontinence, perineal pain and swelling, which seriously reduce physical and mental health (4). The anterior compartment is the most common site of prolapse. Anterior compartment prolapse refers to prolapse of the anterior vaginal wall and is inclusive of urethrocele and cystocele (5). Due to the growth in China’s ageing population, the proportion of POP is predicted to increase (6). A substantial proportion (38–76%) of women who experience prolapse require hospitalization, of whom 10–20% require surgery (7). Unfortunately, without adequate recognition and treatment of the multilocular component of the disease process, surgery may not be curative, and the rate of repeat surgery is as high as 30% (8). It is becoming increasingly clear that clinical assessment alone is insufficient to assess pelvic floor function and anatomy, as it describes superficial anatomy rather than true structural abnormalities. Compounding matters, clinical assessment is often influenced by unrecognized confounding factors such as bladder filling and Valsalva motion duration, which can cause the intraoperative findings to be inconsistent with the outpatient diagnosis (9). The ability of the physical examination to accurately detect the underlying pathophysiology varies greatly, and surgeons are beginning to increasingly rely on imaging. Pelvic floor ultrasonography is currently widely used for POP (10-12), which can visualize the anatomical position of pelvic organs and their support systems in real time at relatively low cost and without the need for radiation. Meanwhile, it can also detect minor prolapse that cannot be detected clinically.
Correctly interpreting the ultrasound images requires extensive training and imaging quality can be variable. These disadvantages likely contribute to the fact that many primary medical institutions do not perform pelvic floor ultrasonography. Given the advances in applying deep learning (DL) to image-based diagnosis in various clinical contexts, including ultrasonography (13,14), we wondered whether we could improve the speed and reliability of pelvic floor ultrasonography for diagnosing POP by training neural networks to interpret the ultrasound images, and more hopefully to provide assistance in pelvic floor prolapse screening and diagnosis to primary hospitals. Therefore, we aimed to further promote the popularization of pelvic floor ultrasound, so as to benefit more grassroots people.
In recent years, AlexNet (15), VGGNet (16), and ResNet (17) have been widely used as pre-trained convolutional neural networks (CNNs) that learn from a large number of general images. However, without further training, these CNNs may not correctly identify medical images. Therefore, this study was performed to develop models for the diagnosis of anterior POP based on these CNNs, and evaluate the diagnostic efficacy of the models. We drew our data from the medical records of patients at West China Second University Hospital who were screened for anterior compartment organ prolapse, which involves the urethra, bladder, or anterior vaginal wall. We present this article in accordance with the TRIPOD+AI reporting checklist (available at https://qims.amegroups.com/article/view/10.21037/qims-24-772/rc).
Methods
The study was conducted in accordance with the Declaration of Helsinki (as revised in 2013). This retrospective study was approved by the Medical Ethics Committee of West China Second University Hospital (No. 2023.317), and the requirement for individual consent for this retrospective analysis was waived.
Patients
We retrospectively analyzed medical records for a consecutive series of women who underwent pelvic floor ultrasonography at West China Second University Hospital between March 2019 and September 2021 (n=2,788). To be included in the study, patients had to have complete data available for their gynecological history, conventional ultrasound images, and the ability to perform the Valsalva motion correctly. Patients were excluded if they had middle pelvic or posterior POP (n=78), pelvic floor tumors or pelvic tumors (n=118), history of pelvic surgery or treatment for acute or chronic pelvic inflammatory disease (n=341), infection of the lower urinary tract or reproductive tract in the acute stage (n=267), or congenital malformation of the pelvic floor or any pelvic structures (n=98). Patients were also excluded if they could not perform the Valsalva procedure correctly due to severe prolapse symptoms (n=81). Among them, patients with anterior compartment organ prolapse based on signs and symptoms in accordance with the “pelvic organ prolapse quantitation” method (18) constituted the abnormal group, and those without any POP were allocated to the normal group (Figure 1). According to the above criteria, 59 cases in the abnormal group and 12 cases in the normal group from June to August 2024 in West China Second University Hospital were collected as the new external validation set.
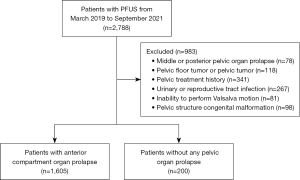
Pelvic floor ultrasonography and dataset of ultrasound images
Two-dimensional static images of the anterior compartment of the pelvic floor while the patient was at rest and under maximal Valsalva motion were obtained from all participants by three physicians who performed pelvic floor ultrasonography in our institution using a color Doppler ultrasound system (Resona OB, Mindray, Shenzhen, China) equipped with a DE10-3U volume probe at a frequency of 4–8 MHz. The maximum Valsalva state was defined as the action of increasing abdominal pressure by holding breath downward after deep inspiration, the movement of pelvic organs to dorsocaudal side or the enlargement of levator hiatus, which lasted ≥6 seconds. During pelvic floor ultrasound examination, the probe was placed in the middle of the perineum of the patient, the indicator point was toward the ventral side of the patient, and the direction of the sound beam was parallel to the sagittal plane of the human body to obtain the standard median sagittal section of the pelvic floor. This section showed the posterior inferior margin of the pubic symphysis, urethra, bladder, vagina, ampulla of rectum, anal canal, and levator ani muscle (Figure 2).
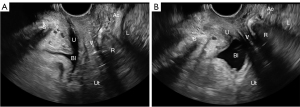
All pictures that showed the standard median sagittal section of the pelvic floor and clearly showed the structure of the pelvic floor were included in the study. Finally, for training neural networks to interpret pelvic floor ultrasound images, we drew on a final dataset of 5,281 abnormal midsagittal images (with 3,423 in the maximal Valsalva motion) and 535 normal midsagittal images (with 360 in the maximal Valsalva motion). We randomly defined 4,652 images (80% of the dataset) as the training set and 1,164 (20% of the dataset) as the test set.
CNNs to interpret pelvic floor ultrasound images
The network parameters needed to be initialized before training. In order to minimize the error between the predicted value and the actual labels, a loss function was defined to measure the difference between the two values. Then, the back propagation algorithm and various optimization algorithms were used to optimize the parameters of the neural network and find the appropriate network parameters, so that the training process of the neural network was faster and more stable.
We trained four CNNs: AlexNet, VGG-16, ResNet-18, and ResNet-50 through a Pytorch DL framework (https://pytorch.org/) using Ubuntu 18.04 (https://ubuntu.com/) running on an Intel Xeon E5-2620 v4 (https://www.intel.com/) and dual-channel Titan Xp GPU (NVIDIA, Santa Clara, CA, USA). For training, image pixel size was adjusted to 224×224, and the following four data enhancement operations were performed to expand the range of training images: random horizontal flip, random rotation, color jitter, and normalization (Figure S1). During internal validation on the test set, images were enhanced only through normalization. During training, the batch size was 32, Adam optimizer (19) was used, epoch count was 100, and the learning rate was initially 0.01, after which it decreased by 20% every 20 epochs.
This study divides the training of each neural network into two ways: random initialization and transfer learning (parameter initialization by loading the pre-trained model) (20), in order to compare the training efficiency of the two methods.
Transfer learning is a technique that uses pre-trained models to suit a new dataset, which can enable neural network models to better learn the generalization features of images, effectively accelerate the training speed, and improve the accuracy (21,22). In this study, transfer learning drew on the ImageNet database [ImageNet (image-net.org)] containing more than 15 million images spanning 20,000 categories. The four neural networks were pre-trained on the ImageNet database, then fine-tuned on pelvic floor ultrasound images (Figure S2).
Evaluation of the CNNs
The identification results were true positive (TP), false positive (FP), true negative (TN), and false negative (FN). The diagnostic performance of each network was assessed in terms of accuracy, precision, recall, and F1-score as described (23,24). These measures were calculated as follows:
Then the receiver operating characteristic (ROC) curves of each network in the training and validation sets were plotted separately. The ROC curve is a graphical image showing the diagnostic ability of a binary classifier when its discrimination threshold changes (25), and the area under the ROC curve (AUC) is a standard indicator in model evaluation.
In addition, the rationality of image classification by neural networks was also assessed using gradient-weighted class activation mapping (26,27). Class activation mapping provides visualization of the most important activation mapping for the target class. It provides a sign that indicates what is the focus of the network.
Comparator sonographers
A group of three sonographers who worked at the ultrasound department of West China Second University Hospital, with more than five years of pelvic ultrasound experience were defined as the comparable group. They were enlisted to evaluate the performance of the training set images, and the average evaluation time of each case was recorded. The evaluation results were summarized, and when there was a discrepancy, a more senior sonographer would evaluate and draw a final conclusion, and then the accuracy of manual evaluation was calculated.
Results
We obtained a dataset of 5,816 pelvic floor ultrasound images from the abnormal group of 1,605 women with anterior compartment organ prolapse (mean age 45.1±12.2 years) and the normal group of 200 women without POP (mean age 38.1±13.4 years), of which 4,652 (average age 40.0±13.1 years) were allocated to the training set and 1,164 (average age 41.0±14.7 years) were allocated to the test set (Figure 1, Table 1).
Table 1
Variables | Abnormal group | Normal group | P value | Training set | Test set | P value |
---|---|---|---|---|---|---|
n | 1,605 | 200 | – | 4,652 | 1,164 | – |
Age (years), mean ± SD | 45.1±12.2 | 38.1±13.4 | <0.01 | 40.0±13.1 | 41.0±14.7 | 0.08 |
Both in the training set and test set, the ResNet-18 network reached maximum accuracy of 92–93% within 20 iterations, and the loss function, which measures the error between the network output and the actual data, decreased to a certain extent and tended to be stable (Figure 3). Similar results were obtained for the other three networks. These results illustrate that the network learned deep features of ultrasound images from women with or without anterior compartment organ prolapse and was able to differentiate them accurately.
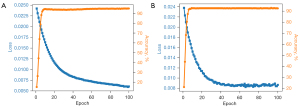
The results of comparison of the four networks, each trained in two ways, showed that the diagnostic speed of AlexNet, VGG-16, ResNet-18, and ResNet-50 for each image was 10.7, 25.4, 13.4, and 21.3 msec, respectively, whereas the average diagnostic speed of human was 15,700 msec. The diagnostic accuracy of these four networks was above 90% (89.51% for human). That is to say, all four greatly accelerated diagnosis of anterior compartment organ prolapse with better accuracy than a human (Table 2). The precision, recall, F1-score, and accuracy all showed that transfer learning led to better performance than random initialization in all four networks. Even without transfer learning, ResNet-18 and ResNet-50 performed better than AlexNet and VGG-16, presumably because they contain a residual connection module, which helps the network fully extract pelvic floor features and accelerates convergence (28). As a result, both of them have excellent performance in anomaly detection on training set, test set, and even external data verification (Table 3).
Table 2
Operator or network | Training method | Precision (%) | Recall (%) | F1-score (%) | Accuracy (%) | Time per image (msec) |
---|---|---|---|---|---|---|
Human | – | – | – | – | 89.51 | 15,700 |
AlexNet | RI | 92.72 | 98.54 | 95.54 | 91.68 | 10.7 |
TL | 93.13 | 98.98 | 95.97 | 92.47 | 10.7 | |
VGG-16 | RI | 92.29 | 99.56 | 95.79 | 92.07 | 25.4 |
TL | 93.99 | 98.25 | 96.07 | 92.73 | 25.4 | |
ResNet-18 | RI | 93.05 | 99.71 | 96.26 | 93.00 | 13.4 |
TL | 94.92 | 98.10 | 96.48 | 93.53 | 13.4 | |
ResNet-50 | RI | 93.86 | 98.25 | 96.01 | 92.60 | 21.3 |
TL | 94.28 | 98.69 | 96.43 | 93.39 | 21.3 |
RI, random initialization; TL, transfer learning.
Table 3
Operator or network | Training method | Precision (%) | Recall (%) | F1-score (%) | Accuracy (%) | Time per image (msec) |
---|---|---|---|---|---|---|
Human | – | – | – | – | 87.53 | 18,700 |
AlexNet | RI | 89.33 | 95.78 | 92.44 | 87.06 | 10.7 |
TL | 87.30 | 99.40 | 92.96 | 87.56 | 10.7 | |
VGG-16 | RI | 90.96 | 96.99 | 93.88 | 89.55 | 25.4 |
TL | 91.01 | 97.59 | 94.19 | 90.05 | 25.4 | |
ResNet-18 | RI | 93.06 | 96.99 | 94.99 | 91.54 | 13.4 |
TL | 94.15 | 96.99 | 95.55 | 92.54 | 13.4 | |
ResNet-50 | RI | 93.14 | 98.19 | 95.60 | 92.54 | 21.3 |
TL | 95.24 | 96.39 | 95.81 | 93.03 | 21.3 |
RI, random initialization; TL, transfer learning.
In both the training sets, all four networks that through transfer learning showed quite high AUCs, all greater than 0.95. In the test set, ResNet-18 and ResNet-50 had larger AUCs than AlexNet and VGG-16, with 0.852 and 0.822, respectively (Figure 4).
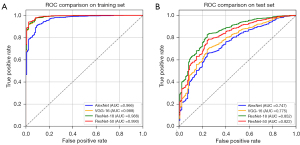
The rationality of image classification was confirmed for ResNet-18 by class activation mapping, which showed that the network paid more attention to clinically relevant regions of ultrasound images (Figure 5).
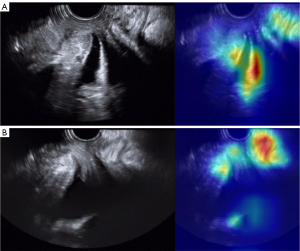
Discussion
Anterior compartment organ prolapse, including anterior vaginal prolapse, urethrocele, and cystocele, is the most common POP and has deleterious effects on women’s health (29). Although clinical examination can be used to evaluate the disease, this remains a relatively subjective measure; it can be affected not only by the skill of the examiner, but also by the ability of the patient to perform the maximum Valsalva motion. Ultrasound is a simple, inexpensive, and harmless diagnostic modality that is easy to implement and is the first choice for most gynecological examinations. Studies have shown that ultrasound has a good diagnostic value in POP (30,31).
DL is a method that extracts hierarchical features from the original input image through its self-learning ability, and finally forms high-level abstract features through iteration, so as to perform classification, which has gained popularity due to its recent success in image segmentation and classification applications (32). In this study, DL and pelvic floor ultrasound were combined to automatically identify and diagnose anterior compartment organ prolapse. The results showed that the current generation of CNNs can rapidly and reliably diagnose anterior compartment organ prolapse, and that transfer learning can improve their diagnostic performance. In fact, the networks provided thousand-fold faster diagnosis with better accuracy than an experienced clinician. There have been reports of DL in magnetic resonance imaging diagnosis of POP (33), but this is the first application of DL to ultrasound diagnosis of anterior compartment organ prolapse.
Our work extends the contexts in which neural networks have been shown to be highly capable at image classification (34,35). Our comparisons suggest that transfer learning can improve diagnostic performance. Neural networks require training on extremely large amounts of data in order to perform accurately without overfitting. Medical image datasets such as the one in the present study are relatively small, so we used data augmentation and transfer learning to help the networks learn to generalize features to a broad range of situations.
This study justifies further exploration of how to apply DL to anterior compartment organ prolapse. However, further research and improvement are still needed. First, this study was a single-center study with a relatively single source of data, and subsequently our findings should be verified and extended in larger, preferably multi-site studies. Second, the models should also be tested for their ability to diagnose situations that are not binary (yes/no) but more nuanced, such as differentiating less and more severe prolapse. Finally, although the models in this study have high accuracy, future work should also train models with datasets that include pelvic ultrasound images from more diverse data for practical clinical use.
Conclusions
Our study suggests that DL enables CNNs to diagnose anterior compartment organ prolapse in women much faster and slightly better than humans. This work may help promote the use of pelvic floor ultrasonography for diagnosing this condition and facilitate the development of pelvic floor ultrasound in primary care. It may also catalyze the application of DL to other types of ultrasound image classification.
Acknowledgments
None.
Footnote
Reporting Checklist: The authors have completed the TRIPOD+AI reporting checklist. Available at https://qims.amegroups.com/article/view/10.21037/qims-24-772/rc
Funding: This work was supported by
Conflicts of Interest: All authors have completed the ICMJE uniform disclosure form (available at https://qims.amegroups.com/article/view/10.21037/qims-24-772/coif). The authors have no conflicts of interest to declare.
Ethical Statement: The authors are accountable for all aspects of the work in ensuring that questions related to the accuracy or integrity of any part of the work are appropriately investigated and resolved. The study was conducted in accordance with the Declaration of Helsinki (as revised in 2013). The study was approved by the Medical Ethics Committee of West China Second University Hospital (No. 2023.317) and the requirement for individual consent for this retrospective analysis was waived.
Open Access Statement: This is an Open Access article distributed in accordance with the Creative Commons Attribution-NonCommercial-NoDerivs 4.0 International License (CC BY-NC-ND 4.0), which permits the non-commercial replication and distribution of the article with the strict proviso that no changes or edits are made and the original work is properly cited (including links to both the formal publication through the relevant DOI and the license). See: https://creativecommons.org/licenses/by-nc-nd/4.0/.
References
- Grinberg K, Sela Y, Nissanholtz-Gannot R. New Insights about Chronic Pelvic Pain Syndrome (CPPS). Int J Environ Res Public Health 2020;17:3005. [Crossref] [PubMed]
- Romeikienė KE, Bartkevičienė D. Pelvic-Floor Dysfunction Prevention in Prepartum and Postpartum Periods. Medicina (Kaunas) 2021;57:387. [Crossref] [PubMed]
- Weintraub AY, Glinter H, Marcus-Braun N. Narrative review of the epidemiology, diagnosis and pathophysiology of pelvic organ prolapse. Int Braz J Urol 2020;46:5-14. [Crossref] [PubMed]
- Araujo CC, Coelho SSA, Martinho N, Tanaka M, Jales RM, Juliato CRT. Clinical and ultrasonographic evaluation of the pelvic floor in primiparous women: a cross-sectional study. Int Urogynecol J 2018;29:1543-9. [Crossref] [PubMed]
- Bahrami S, Khatri G, Sheridan AD, Palmer SL, Lockhart ME, Arif-Tiwari H, Glanc P. Pelvic floor ultrasound: when, why, and how? Abdom Radiol (NY) 2021;46:1395-413. [Crossref] [PubMed]
- Niu K, Zhai Q, Fan W, Li L, Yang W, Ye M, Meng Y. Robotic-Assisted Laparoscopic Sacrocolpopexy for Pelvic Organ Prolapse: A Single Center Experience in China. J Healthc Eng 2022;2022:6201098. [Crossref] [PubMed]
- Wen L, Shek KL, Subramaniam N, Friedman T, Dietz HP. Correlations between Sonographic and Urodynamic Findings after Mid Urethral Sling Surgery. J Urol 2018;199:1571-6. [Crossref] [PubMed]
- Wu S, Zhang X. Advances and Applications of Transperineal Ultrasound Imaging in Female Pelvic Floor Dysfunction. Advanced Ultrasound in Diagnosis and Therapy 2023;7:235-47. [Crossref]
- Dietz HP. Pelvic Floor Ultrasound: A Review. Clin Obstet Gynecol 2017;60:58-81. [Crossref] [PubMed]
- Shek KL, Dietz HP. Pelvic floor ultrasonography: an update. Minerva Ginecol 2013;65:1-20. [PubMed]
- Kozma B, Larson K, Scott L, Cunningham TD, Abuhamad A, Poka R, Takacs P. Association between pelvic organ prolapse types and levator-urethra gap as measured by 3D transperineal ultrasound. J Ultrasound Med 2018;37:2849-54. [Crossref] [PubMed]
- Dietz HP. Ultrasound in the investigation of pelvic floor disorders. Curr Opin Obstet Gynecol 2020;32:431-40. [Crossref] [PubMed]
- Qu E, Zhang X. Advanced Application of Artificial Intelligence for Pelvic Floor Ultrasound in Diagnosis and Treatment. Advanced Ultrasound in Diagnosis and Therapy 2023;7:114-21. [Crossref]
- Li S, Wu H, Tang C, Chen D, Chen Y, Mei L, Yang F, Lv J. Self-supervised Domain Adaptation with Significance-Oriented Masking for Pelvic Organ Prolapse detection. Pattern Recognition Letters 2024;185:94-100. [Crossref]
- Deng J, Dong W, Socher R, Li LJ, Li K, Li F. ImageNet: A large-scale hierarchical image database. Proc of IEEE Computer Vision & Pattern Recognition, Miami, FL, USA; 2009:248-55.
- Simonyan K, Zisserman A. Very Deep Convolutional Networks for Large-Scale Image Recognition. Computer Science. 2014. doi:
10.48550 /arXiv.1409.1556. - He K, Zhang X, Ren S, Sun J. Deep Residual Learning for Image Recognition. IEEE Conference on Computer Vision and Pattern Recognition. 2016 IEEE Conference on Computer Vision and Pattern Recognition (CVPR), Las Vegas, NV, USA; 2016:770-8.
- Bump RC, Mattiasson A, Bø K, Brubaker LP, DeLancey JO, Klarskov P, Shull BL, Smith AR. The standardization of terminology of female pelvic organ prolapse and pelvic floor dysfunction. Am J Obstet Gynecol 1996;175:10-7. [Crossref] [PubMed]
- Kingma DP, Ba JL. Adam: A Method for Stochastic Optimization. 2014. Computer Science. 2014. doi:
10.48550 /arXiv.1412.6980. - Zhuang F, Qi Z, Duan K, Xi D, Zhu Y, Zhu H. A Comprehensive Survey on Transfer Learning. Proceedings of the IEEE 2021;109:43-76. [Crossref]
- Morid MA, Borjali A, Del Fiol G. A scoping review of transfer learning research on medical image analysis using ImageNet. Comput Biol Med 2021;128:104115. [Crossref] [PubMed]
- Hosseinzadeh Taher MR, Haghighi F, Feng R, Gotway MB, Liang J. A Systematic Benchmarking Analysis of Transfer Learning for Medical Image Analysis. Domain Adapt Represent Transf Afford Healthc AI Resour Divers Glob Health (2021) 2021;12968:3-13. [Crossref] [PubMed]
- Kumar Y, Koul A, Singla R, Ijaz MF. Artificial intelligence in disease diagnosis: a systematic literature review, synthesizing framework and future research agenda. J Ambient Intell Humaniz Comput 2023;14:8459-86. [Crossref] [PubMed]
- Wang J, Deng G, Li W, Chen Y, Gao F, Liu H, He Y, Shi G. Deep learning for quality assessment of retinal OCT images. Biomed Opt Express 2019;10:6057-72. [Crossref] [PubMed]
- Liu W, Wang S, Ye Z, Xu P, Xia X, Guo M. Prediction of lung metastases in thyroid cancer using machine learning based on SEER database. Cancer Med 2022;11:2503-15. [Crossref] [PubMed]
- Zhou B, Khosla A, Lapedriza A, Oliva A, Torralba A. Learning Deep Features for Discriminative Localization. 2016 IEEE Conference on Computer Vision and Pattern Recognition (CVPR), Las Vegas, NV, USA; 2016:2921-9.
- Selvaraju RR, Cogswell M, Das A, Vedantam R, Parikh D, Batra D. Grad-CAM: Visual Explanations from Deep Networks via Gradient-Based Localization. Int J Comput Vis 2019;128:336-59. [Crossref]
- Pan X, Xu J, Pan Y, Wen L, Lin W, Bai K, Fu H, Xu Z. AFINet: Attentive Feature Integration Networks for image classification. Neural Netw 2022;155:360-8. [Crossref] [PubMed]
- Li Y, Zhang QY, Sun BF, Ma Y, Zhang Y, Wang M, Ma C, Shi H, Sun Z, Chen J, Yang YG, Zhu L. Single-cell transcriptome profiling of the vaginal wall in women with severe anterior vaginal prolapse. Nat Commun 2021;12:87. [Crossref] [PubMed]
- Nam G, Lee SR, Kim SH, Chae HD. Importance of Translabial Ultrasound for the Diagnosis of Pelvic Organ Prolapse and Its Correlation with the POP-Q Examination: Analysis of 363 Cases. J Clin Med 2021;10:4267. [Crossref] [PubMed]
- Chantarasorn V, Dietz HP. Diagnosis of cystocele type by clinical examination and pelvic floor ultrasound. Ultrasound Obstet Gynecol 2012;39:710-4. [Crossref] [PubMed]
- Akkus Z, Cai J, Boonrod A, Zeinoddini A, Weston AD, Philbrick KA, Erickson BJ. A Survey of Deep-Learning Applications in Ultrasound: Artificial Intelligence-Powered Ultrasound for Improving Clinical Workflow. J Am Coll Radiol 2019;16:1318-28. [Crossref] [PubMed]
- Feng F, Ashton-Miller JA, DeLancey JOL, Luo J. Convolutional neural network-based pelvic floor structure segmentation using magnetic resonance imaging in pelvic organ prolapse. Med Phys 2020;47:4281-93. [Crossref] [PubMed]
- Thwaites D, Moses D, Haworth A, Barton M, Holloway L. Artificial intelligence in medical imaging and radiation oncology: Opportunities and challenges. J Med Imaging Radiat Oncol 2021;65:481-5. [Crossref] [PubMed]
- Krizhevsky A, Sutskever I, Hinton GE. ImageNet Classification with Deep Convolutional Neural Networks Advances in Neural Information Processing Systems, Vol. 25, Curran Associates, Inc., Red Hook, NY; 2012;1097-105.