Quantitative plaque characterization, pericoronary fat attenuation index, and fractional flow reserve: a novel method for differentiating between stable and unstable angina pectoris in a case-control study
Introduction
Ruptured plaque accounts for approximately 70% of all coronary thrombotic events and cardiovascular deaths (1). The 2021 American Heart Association/American College of Cardiology/American Society of Echocardiography/American College of Chest Physicians/Society for Academic Emergency Medicine/Society of Cardiovascular Computed Tomography/Society for Cardiovascular Magnetic Resonance Guideline for the Evaluation and Diagnosis of Chest Pain states that coronary computed tomography angiography (CCTA) can visualize the coronary arteries to help diagnose the extent of coronary artery disease (CAD), plaque composition, and high-risk plaques (2). However, CCTA only provides visual imaging information (3,4), which is insufficient to differentiate noncalcified plaque subtypes (5) or show inflammatory cell infiltration or neovascularization within the plaque.
Recent studies have demonstrated that the pericoronary fat attenuation index (FAI) captures coronary inflammation and is associated with the culprit lesions in major adverse cardiac events (MACEs) (6-8). Additionally, the risk of hemodynamically significant CAD increases with higher epicardial fat volume (9). Fractional flow reserve with computed tomography (FFR-CT) is a promising non-invasive coronary physiology index that correlates well with angiographic FFR and has been used by the 2022 Coronary Artery Disease Reporting and Data System 2.0 for the assessment of myocardial ischemia (10).
Therefore, we hypothesized that the combined assessment of quantitative plaque characterization, FAI, and FFR-CT could provide valuable diagnostic information to differentiate between stable and unstable angina. This study utilized a fully automated one-stop analysis of quantitative plaque features, FAI, and FFR-CT using a coronary artificial intelligence (AI)-assisted diagnostic system, which may provide reference to clinicians for risk-stratifying patients and taking intervention measures. We present this article in accordance with the STROBE reporting checklist (available at https://qims.amegroups.com/article/view/10.21037/qims-24-1031/rc).
Methods
Patients
This retrospective study was conducted in accordance with the Declaration of Helsinki (as revised in 2013). The study was approved by the Ethics Committee of Tongji Medical College of Huazhong University of Science (No. 2020-S336), which waived the requirement for informed consent. Consecutive patients who were first diagnosed with angina pectoris between January 2015 and August 2023 at Tongji Hospital and underwent CCTA were included in this study. Patients’ clinical data were collected from electronic medical records during their first visit. The inclusion criteria were age ≥18 years, heart rate of 60–70 beats per minute, absence of arrhythmia, and availability of complete CCTA examination data. The exclusion criteria were as follows: (I) patients with poor CCTA image quality that could not be used for diagnosis; (II) history of infarction; percutaneous coronary intervention; coronary artery bypass grafting; (III) coronary artery malformation or myocardial bridge; and (IV) history of statin administration. Figure 1 illustrates the inclusion process.
CCTA
A Canon 320-row CT scanner (Aquilion Vision, Canon Medical Systems, Otawara, Japan) was used in prospective cardiac-gated scanning mode with a scanning range of 1 cm below the tracheal bifurcation to the apex. The scanning parameters were: pre-volume gated scan, 100 kV, 0.5×320 mm, intelligent mA control, and an exposure range of 70–80%. Iterative reconstruction was used for image post-processing.
Quantitative plaque characterization, FAI, and FFR-CT analysis
Quantitative plaque characteristics, FAI, and FFR-CT were automatically analyzed using a coronary AI-assisted diagnostic system (Shukun Technology Co., Ltd., Beijing, China) with a deep learning function, and a radiologist with vast operational experience corrected poorly analyzed data. The algorithms for pre-trained Convolutional Neural Network fully automated deep learning for the coronary AI-assisted diagnostic system are as follows (11-13). First, the extraction of blood vessels was applied using the improved three-dimensional U-Net architecture combined with the Growth Iterative Prediction Network and the bottle-neck model, and the visualization of multiplanar recombinants was carried out. Subsequently, the vessels were named, the centerline was extracted, and the vessel lumen was segmented. The local feature vectors of the points on the vessel path were integrated again. Additionally, the sequence information provided by the vessels was used to globalize the whole vessel tree and reconstruct the cross-sectional image perpendicular to the vessel centerline to obtain accurate blood flow features (including FFR-CT values), quantitative plaque features, and FAI. Plaque characteristics, FAI, and FFR-CT were collected from the following three main branches: the left anterior descending (LAD), left circumflex (LCX), and right coronary arteries (RCAs). Plaque characteristics included the degree of luminal stenosis in the proximal-middle portions, the luminal area of the narrowest point, total plaque volume and length, and volume and percentage of components within the total plaque [lipid <30 Hounsfield units (HU), fibrolipid (30–129 HU), fibrous (130–350 HU), and calcification (>350 HU)]. FAI was the pericoronary adipose tissue CT attenuation value, which equaled the coronary artery diameter, approximately 10–50 mm long from the opening. FFR-CT is generally performed at 2 cm distal to the last stenosis site. Two evaluators (T.Z. and D.L.) calculated and independently assessed all vessels’ plaque characteristics, FAI, and FFR-CT. After an interval of 1 month from the end of measurements in the last patient, a second measurement was performed on all patients to calculate intra- and interobserver agreements (14).
Determination of vessels in offenders with unstable angina pectoris
Two experienced interventional cardiologists categorized angina pectoris into stable and unstable angina pectoris based on electrocardiograms, cardiac biomarkers, and clinical symptoms (15). To ensure impartiality and objectivity, these two cardiologists were completely independent of this study and were not influenced by any factors related to the study. Stable angina was defined if there was no change in the duration, frequency, or intensity of chest pain, whereas new progressive, severe, or resting angina was defined as unstable angina (16). Then, without knowledge about the CCTA results, they identified the culprit and non-culprit lesions based on invasive coronary angiography lesion morphology and stenosis severity, echocardiographic wall motion abnormalities, electrocardiogram findings, and revascularization (17). Of the seven patients with culprit lesions, a total of 14 vessels did not reach initial consensus; eight vessels in the last seven patients were identified as having culprit lesions after discussion and reaching consensus, whereas the remaining six vessels exhibited stable lesions.
Statistical analysis
Statistical analyses were performed using IBM SPSS Statistics for Windows, version 26.0 (IBM Corporation, Armonk, NY, USA) and MedCalc (version 20.0, Ostend, Belgium). Count data were compared using the Chi-squared test and expressed as frequencies (percentages). Measurement data were compared using the Mann-Whitney U or independent sample t-test based on normal distribution after Shapiro-Wilk test analysis and expressed as the median (interquartile range) or mean ± standard deviation. Propensity score matching (PSM) was performed using a multivariate logistic regression model based on sex, smoking and alcohol consumption history, high blood pressure, diabetes mellitus, and hyperlipidemia. Pairs of 168:168 patients were derived using 1:1 greedy nearest-neighbor matching with a propensity score of 0.05. Receiver operating characteristic (ROC) curves were used to analyze each plaque characteristic, FAI, FFR-CT, and their combined model (pericoronary FAI, FFR-CT, stenosis degree, lumen area at the narrowest point, total plaque length, and percentage of intraplaque lipids, fibrolipids, fiber, calcium components) to differentiate the diagnostic efficacy between stable and unstable angina. The steps for generating the ROC curve of the joint model were as follows. First, the indicators for joint were used as independent variables. The presence of unstable angina pectoris was used as the dependent variable for multifactor binary logistic regression analysis; a logistic regression model was fitted to compute the predictive probability value. Subsequently, this predicted probability value was used as a parameter of the joint model for ROC curve-plotting. The sensitivity, specificity, and area under the ROC curve (AUC) were calculated. We determined the cutoff value based on the maximum Youden’s index. FFR-CT was the reference value of 0.8 for invasive FFR, and an FFR-CT ≤0.8 was considered a positive value (18). The degree of stenosis was alternative: used as the cutoff at 50%, and ≥50% was considered a positive value. The Delong test was used to compare the differences in the AUC. The risk factors for unstable angina were analyzed using binary logistic analysis. First, univariate binary logistic regression analysis was performed. Second, indicators with P<0.1 from the univariate analysis were fitted in the multifactorial regression model for analysis. After the ROC curve analysis, all indicators were treated as dichotomous variables according to the best critical values before inclusion in the multivariate model. Intra- and intergroup variability between the two assessors was tested using the intra-class correlation coefficient (ICC) (ICC >0.80 was considered good agreement). Statistical significance was set at P<0.05.
Results
Baseline characteristics of patients
Overall, 417 patients were enrolled in this study; 215 and 202 had stable and unstable angina, respectively. The group with unstable angina had a significantly younger age of onset (P=0.043), a higher proportion of men, and a history of smoking and diabetes mellitus (P=0.017, 0.027, and 0.023, respectively) compared with the group with stable angina. We performed PSM to avoid selectivity bias in baseline information. After PSM, no significant differences were observed between the 168 pairs of patients with stable and unstable angina regarding sex, smoking status, history of diabetes mellitus, or other baseline data (all P>0.05) (Table 1).
Table 1
Characteristics | Before propensity score matching | After propensity score matching | |||||
---|---|---|---|---|---|---|---|
Stable angina | Unstable angina | P | Stable angina | Unstable angina | P | ||
Male | 127 (59.1) | 142 (70.3) | 0.017 | 114 (67.9) | 110 (65.5) | 0.643 | |
Age (years) | 67.8±10.9 | 65.6±11.4 | 0.043 | 67.4±11.2 | 66.0±11.6 | 0.265 | |
Body mass index (kg/m2) | 24.4±3.3 | 26.6±23.8 | 0.242 | 24.4±3.4 | 26.3±25.4 | 0.376 | |
Low-density lipoprotein (mmol/L) | 2.6 (1.8, 3.1) | 2.8 (1.7, 3.4) | 0.670 | 2.5 (1.8, 3.1) | 2.7 (1.7, 3.4) | 0.840 | |
Triglycerides (mmol/L) | 1.8 (0.9, 2.5) | 1.5 (1, 2.1) | 0.998 | 1.9 (1.2, 2.7) | 1.3 (1, 2) | 0.565 | |
High-density lipoprotein (mmol/L) | 1 (0.8, 1.2) | 1.1 (0.8, 1.2) | 0.050 | 1 (0.8, 1.2) | 1 (0.9, 1.2) | 0.143 | |
Myoglobin (pg/mL) | 38.4 (32.2, 48.6) | 41 (28.7, 54.9) | 0.976 | 39.2 (32.1, 54.4) | 41.9 (28.7, 55.3) | 0.957 | |
Heart-type creatine kinase isoenzyme (pg/mL) | 0.9 (0.7, 1.5) | 0.9 (0.6, 1.2) | 0.501 | 1 (0.7, 1.5) | 1 (0.6, 1.2) | 0.738 | |
Glucose (mmol/L) | 5.8 (5.4, 7.4) | 5.6 (4.9, 8.2) | 0.448 | 6.1 (5.4, 7.6) | 5.3 (4.9, 7.5) | 0.553 | |
Total cholesterol (mmol/L) | 4.1 (3.2, 4.8) | 4.6 (3.1, 5.4) | 0.255 | 4 (3.1, 4.8) | 4.5 (3, 4.9) | 0.350 | |
History of smoking | 62 (28.8) | 79 (39.1) | 0.027 | 60 (35.7) | 59 (35.1) | 0.909 | |
History of alcohol consumption | 39 (18.1) | 47 (23.3) | 0.196 | 36 (21.5) | 35 (20.8) | 0.894 | |
Hypertension | 98 (45.6) | 109 (54.0) | 0.087 | 78 (46.4) | 86 (51.2) | 0.383 | |
Diabetes | 45 (20.9) | 62 (30.7) | 0.023 | 43 (25.6) | 44 (26.2) | 0.901 | |
Hyperlipidemia | 37 (17.2) | 38 (18.8) | 0.67 | 29 (17.2) | 28 (16.7) | 0.884 |
Data are n (%), mean ± standard deviation or median (interquartile range) as appropriate.
Quantitative plaque characterization, pericoronary FAI, and FFR-CT analysis
The 168 patients with stable angina had 354 diseased vessels (149 LADs, 87 LCXs, and 118 RCAs); the 168 patients with unstable angina had 174 culprit vessels (94 LADs, 27 LCXs, and 53 RCAs). In addition, in patients with unstable angina, there were 195 non-culprit lesion vessel strips (47 LADs; 71 LCXs; and 77 RCAs).
The patients with unstable angina pectoris had a significantly higher pericoronary FAI, greater volume of fibrous, lipid, and fibrolipid and percentage of lipid and fibrolipid components within the total plaque, more severe stenosis, and longer plaque lengths (all P<0.001), and significantly lower FFR-CT, calcification percentage, and lumen area at the most stenotic point (all P<0.05) than did those with stable angina pectoris (Table 2).
Table 2
Characteristics | Stable angina | Unstable angina | P |
---|---|---|---|
FAI (HU) | −85 (−91, −79.8) | −83 (−88, −77) | <0.001 |
FFR-CT | 0.9 (0.8, 0.9) | 0.8 (0.7, 0.9) | 0.003 |
Ductal area at the narrowest point (mm2) | 4.1 (2.6, 6.5) | 3.5 (1.7, 5.9) | 0.003 |
Degree of stenosis (%) | 50 (20, 71) | 60 (26, 82.5) | <0.001 |
Total plaques | |||
Plaque length (mm) | 19.5 (7.8, 38.8) | 26.6 (11.2, 44.8) | 0.015 |
Plaque volume (mm3) | 59.9 (18.3, 162) | 91 (32.1, 177.5) | 0.053 |
Lipid percentage (%) | 1.4 (0, 5.9) | 3.4 (0.9, 7.9) | <0.001 |
Fibrolipid percentage (%) | 10.8 (0.7, 32.6) | 27.2 (7.7, 46) | <0.001 |
Fibre percentage (%) | 18.3 (8.8, 31.2) | 20.7 (12.4, 30.6) | 0.147 |
Calcification percentage (%) | 65.3 (18.3, 87.1) | 43.4 (4.6, 74.9) | <0.001 |
Lipid volume (mm3) | 1.2 (0, 4.8) | 2.6 (0.5, 8) | <0.001 |
Fibrolipid volume (mm3) | 7.5 (0.3, 22.6) | 17.7 (6.4, 39.7) | <0.001 |
Fiber volume (mm3) | 11.4 (2.6, 25.6) | 16.2 (5.1, 30.9) | 0.009 |
Calcification Volume (mm3) | 25 (3.2, 96.9) | 21.5 (1.4, 99) | 0.449 |
Data are median (interquartile range). FAI, fat attenuation index; FFR-CT, fractional flow reserve with computed tomography; HU, Hounsfield units.
Diagnostic efficacy analysis for identifying stable versus unstable angina pectoris
The AUC values of pericoronary FAI, with an optimal threshold of −82 HU, fibrolipid volume within the total plaque required to differentiate stable angina from unstable angina, and the combined model were 0.602, 0.631, and 0.698, respectively (all P<0.001) (Table 3). The AUC value of the combined model was significantly higher than that of the diagnostic model using a single indicator (all P<0.001) (Figure 2).
Table 3
Variables | Cutoff value | Sensitivity (%) | Specificity (%) | AUC (95% CI) | P |
---|---|---|---|---|---|
FAI | >−82 HU | 46.55 | 67.51 | 0.602 (0.559–0.644) | <0.001 |
FFR-CT | – | 48.85 | 67.23 | 0.58 (0.537–0.623) | <0.001 |
Degree stenosis | – | 68.97 | 47.46 | 0.582 (0.539–0.625) | <0.001 |
Area of the narrowest canal | ≤2.62 mm2 | 39.66 | 75.42 | 0.579 (0.536–0.622) | 0.003 |
Total plaque volume | >88.7 mm3 | 51.15 | 60.73 | 0.552 (0.508–0.595) | 0.049 |
Total plaque length | >23.1 mm3 | 55.17 | 57.91 | 0.565 (0.522–0.608) | 0.014 |
Calcification percentage | ≤69.7% | 71.26 | 47.18 | 0.6 (0.557–0.642) | <0.001 |
Fiber volume | >10.2 mm3 | 66.67 | 47.74 | 0.57 (0.527–0.613) | 0.008 |
Fibrolipid volume | >6.5 mm3 | 75.29 | 48.87 | 0.631 (0.588–0.672) | <0.001 |
Fibrolipid percentage | >6.7% | 77.59 | 42.94 | 0.621 (0.578–0.663) | <0.001 |
Lipid volume | >4.4 mm3 | 44.25 | 74.01 | 0.609 (0.566–0.651) | <0.001 |
Lipid percentage | >1.2% | 71.84 | 48.87 | 0.605 (0.562–0.647) | <0.001 |
Combined model | – | 72.99 | 60.73 | 0.698 (0.657–0.737) | <0.001 |
FFR-CT ≤0.8 and stenosis ≥50% were considered positive. The combined model comprised the percentages of lipid, fibrolipid, fiber, and calcium components within the total plaque, total plaque length, degree of stenosis, lumen area at the narrowest point, FFR-CT, and pericoronary FAI. ROC, receiver operating characteristic; FAI, fat attenuation index; FFR-CT, fractional flow reserve with computed tomography; HU, Hounsfield units; AUC, area under the curve; CI, confidence interval.
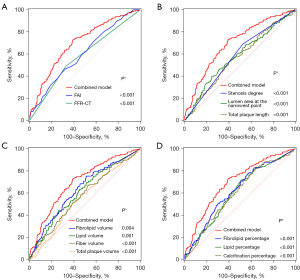
Analysis of risk factors for unstable angina
After excluding FFR-CT confounders, stenosis degree, total plaque length, volume of lipid components within the total plaque, area of the narrowest diameter; and number of plaques, the pericoronary FAI >−82 HU and percentage of lipid components within the total plaque >1.2% were identified as independent risk factors for unstable angina pectoris (odds ratios: 1.798 and 1.865, P=0.003 and 0.009, respectively) (Figure 3).
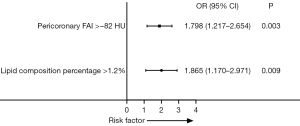
Intra- and inter-observer reproducibility analysis
Quantitative coronary characterization, pericoronary FAI, and FFR-CT measurements showed good intraobserver (ICC: 0.899–0.931) and interobserver (ICC: 0.887–0.918) reproducibility (Table 4).
Table 4
Observer | FAI | FFR-CT | |||||
---|---|---|---|---|---|---|---|
ICC value | 95% CI | P | ICC value | 95% CI | P | ||
A† and A‡ | 0.931 | 0.923–0.938 | <0.001 | 0.909 | 0.899–0.918 | <0.001 | |
A† and B† | 0.899 | 0.888–0.909 | <0.001 | 0.914 | 0.905–0.923 | <0.001 | |
A† and B‡ | 0.900 | 0.889–0.910 | <0.001 | 0.895 | 0.883–0.905 | <0.001 | |
A‡ and B† | 0.912 | 0.902–0.921 | <0.001 | 0.917 | 0.907–0.925 | <0.001 | |
A‡ and B‡ | 0.914 | 0.905–0.923 | <0.001 | 0.887 | 0.874–0.898 | <0.001 | |
B† and B‡ | 0.910 | 0.900–0.919 | <0.001 | 0.918 | 0.909–0.926 | <0.001 |
A, Tingting Zhu (T.Z.); B, Defu Li (D.L.). † and ‡ represent the first and second survey after an interval of 1 month, respectively. FAI, fat attenuation index; FFR-CT, fractional flow reserve with computed tomography; ICC, intra-class correlation coefficient; CI, confidence interval.
Discussion
This study presents three substantial findings. First, quantitative plaque characteristics, pericoronary FAI, and FFR-CT differed significantly between stable and unstable angina. Second, the combined model utilizing quantitative plaque characteristics, FAI, and FFR-CT effectively differentiates between stable and unstable angina. Furthermore, pericoronary FAI and total intraplaque lipid percentage >−82 HU and >1.2%, respectively, were identified as independent risk factors for unstable angina.
CCTA has emerged as an indispensable non-invasive diagnostic tool for managing cardiovascular diseases. The latest guidelines highlight that CCTA not only visualizes coronary arteries but also aids in diagnosing the severity of CAD, plaque composition, and characteristics of high-risk plaques (2,15). Regarding the ischemic cascade, the application of CCTA is pivotal in facilitating the early detection of signs of myocardial blood-flow restriction, which is crucial for preventing further myocardial damage and enabling the formulation of timely treatment strategies.
Previous preliminary studies on quantitative plaque characteristics have shown a strong correlation between these characteristics and MACE, particularly low-density plaque volume (19). However, this study only considered the traditional definitions of low-density and calcified plaque volume without further analysis of the lipid, fibrolipid, fiber, or calcification components within each plaque.
Our deep-learning-based AI-assisted diagnostic system can quickly and accurately determine the nature, volume, and length of plaques, specifically assessing the volume and percentage of lipids, fibrolipids, fibers, and calcification components within the plaques. In our study, the volume and percentage of lipid and fibrolipid components within the total plaque were significantly higher in patients with unstable angina than in those with stable angina, while the percentage of calcified components was significantly lower. Dong et al., using intravascular ultrasound, similarly observed that patients with unstable angina had larger necrotic cores and a lower percentage of calcified volume than those with stable angina (20). A portion of the lipid component may represent a low-density plaque with a necrotic core, whereas a portion of the fibrolipid component can indicate a thin fibrous cap on the surface of the lipid core. Additionally, a portion of the calcification may represent punctate calcification when the volume of calcification is sufficiently small. In this study, variations in the lipid, fibrolipid, and calcification components within plaques may indicate that plaques in the coronary arteries of patients with unstable angina are more unstable and prone to rupture than those in individuals with stable angina.
In contrast to our study, Li et al. found no significant difference in calcification volume between culprit and non-culprit lesions (21). Furthermore. Yan et al. discovered that patients with FFR ≤0.8 had significantly larger calcification volumes than did those with FFR >0.8 (22). These differences in calcification volume may be attributed to multiple factors. First, the analysis software differed across studies; we used a deep learning coronary AI-based assisted diagnosis system, whereas the other studies did not. Second, our sample size was relatively large, which may have enhanced the study’s credibility. Third, the study populations focused on patients with stable and unstable angina, while previous studies included patients with acute coronary syndrome (ACS) and those with angina or angina-like CAD. Moreover, the quantitative classification of plaques varied; we employed AI techniques to quantitatively assess plaque composition, whereas other studies classified plaques conventionally based on low density, fibers, and calcifications.
In our study, a lipid percentage ≥1.2% of the total plaque volume was identified as a risk factors for unstable angina. The extent of the lipid core, thickness and structure of the fibrous cap are critical indicators of plaque vulnerability (23). Plaque formation progresses through different stages of development. As the fibrous atherosclerotic plaque advances, vascular endothelial growth factors produced by macrophages, hypoxia, and oxidative stress continue to contribute to neovascularization. These neovessels, which lack smooth muscle-cell layers and gap junctions, are prone to leakage, leading to plaque hemorrhage, necrotic-core enlargement, and, ultimately, plaque rupture (24). The percentage of lipid content represents, to some extent, the proportion of the lipid necrotic core, and a higher significantly predicts a greater risk of rupture. In a 2003 report defining vulnerable plaques (high-risk plaques) and risk-assessment strategies, one of the main criteria for high-risk plaques was the presence of a lipid core comprising >40% of the total plaque volume (25). Lipid-rich inflammatory plaques are frequently observed in asymptomatic patients (25). Therefore, establishing methods to quantify lipid content percentage within the plaque to predict the risk of plaque rupture should be explored in further investigations.
Vascular inflammation is a major driver of plaque rupture events, and pericoronary FAI is used to assess coronary inflammation, corresponds to vascular inflammation (6,26). Pericoronary FAI progressively increases attenuation values across the following three stages: no disease, stable CAD, and acute myocardial infarction (27). This trend was also observed in our study, where patients with unstable angina had significantly greater pericoronary FAI than did those with stable angina. Thus, pericoronary FAI serves as another risk factor for unstable angina, in addition to total lipid content percentage. Moreover, pericoronary FAI is also a relevant risk factor for offender lesions (vessels) (21,28). Therefore, pericoronary FAI provides a solid basis for the clinical implementation of preventive strategies.
In our study, the AUC values for FFR-CT, pericoronary FAI, and total fibrolipid volume for differentiating between stable and unstable angina ranged from 0.58 to 0.631, indicating fair diagnostic results. Although the pathophysiological mechanisms may differ between stable and unstable angina, the differences in plaque characteristics between the two conditions may be narrower than the distinctions between regular patients and those with myocardial infarction or other MACE. Plaque rupture is not always associated with an immediate related event and can occur independently; it has been demonstrated pathologically that previously ruptured plaques are frequently found to have healed in patients without clinical heart disease (29). Additionally, the thin fibrous cap and lipid-rich plaque factors associated with plaque rupture in 25–60% of ACS cases may be caused by plaque erosion, calcified nodules, or functional coronary alterations (30).
Currently, various strategies exist for assessing chest pain; however, clinical guidance remains inadequate. While invasive FFR can guide treatment, its invasiveness and high-cost limit routine use (31). Traditional CCTA shows limited diagnostic performance; its anatomical assessment is restricted, and functional assessment (e.g., FFR-CT) is insufficient. Thus, relying on a single technique is insufficient to effectively meet clinical needs. In our study, diagnostic efficacy was significantly improved when full plaque characterization, FAI, and FFR-CT were combined. This approach may provide a new and effective non-invasive diagnostic method for clinically differentiating between stable and unstable angina. It integrates more detailed anatomical, physiological, and functional information on CCTA, and its data can be obtained automatically through a coronary artery-assisted diagnostic system in a simple, fast, and repeatable manner, making it practical in clinical practice. The 2022 CAD-reporting and data system version 2.0 is no longer limited to anatomically relevant plaque properties and stenosis. However, it currently includes FFR-CT and myocardial CT perfusion as assessment indicators (10). Therefore, this combination of tools and indicators may present a new approach to assessing chest pain, aiding in the screening of “vulnerable populations”, and is expected to help optimize chest pain assessment strategies, thereby reducing the risk of MACE in patients.
There are some limitations in this study. First, due to its retrospective nature, there may have been selection bias in patient inclusion. Second, the determination of the components within the plaque may be affected by the spatial resolution of CCTA images and beam artifacts related to calcification plaque imaging. However, our AI technology is particularly well-suited to processing pixel-based information, thereby reducing observer variation, saving analysis time, and enhancing the daily clinical practice of CCTA (32,33). The external validity and broad applicability of the diagnostic model in this study were not fully validated; therefore, larger, multicenter prospective studies are needed to further assess its accuracy and reliability.
Conclusions
In conclusion, patients with stable and unstable angina exhibited different degrees of intraplaque lipids, fibrolipids, fibers, and calcification components, with a greater risk of unstable angina associated with a total intraplaque lipid component percentage >1.2% and pericoronary FAI >−82 HU. Therefore, the multiparameter combination of quantitative plaque characteristics based on the AI-assisted diagnostic system, pericoronary FAI, and FFR-CT may provide a new approach for differentiating between stable and unstable angina.
Acknowledgments
None.
Footnote
Reporting Checklist: The authors have completed the STROBE reporting checklist. Available at https://qims.amegroups.com/article/view/10.21037/qims-24-1031/rc
Funding: The research leading to these results received funding from
Conflicts of Interest: All authors have completed the ICMJE uniform disclosure form (available at https://qims.amegroups.com/article/view/10.21037/qims-24-1031/coif). The authors have no conflicts of interest to declare.
Ethical Statement: The authors are responsible for all aspects of the work, ensuring that any questions regarding the accuracy or integrity of any part are thoroughly investigated and resolved. This retrospective study was conducted in accordance with the Declaration of Helsinki (as revised in 2013). The study was approved by the Ethics Committee of Tongji Medical College of Huazhong University of Science (No. 2020-S336), which waived the requirement for informed consent.
Open Access Statement: This is an Open Access article distributed in accordance with the Creative Commons Attribution-NonCommercial-NoDerivs 4.0 International License (CC BY-NC-ND 4.0), which permits the non-commercial replication and distribution of the article with the strict proviso that no changes or edits are made and the original work is properly cited (including links to both the formal publication through the relevant DOI and the license). See: https://creativecommons.org/licenses/by-nc-nd/4.0/.
References
- Virmani R, Burke AP, Farb A, Kolodgie FD. Pathology of the vulnerable plaque. J Am Coll Cardiol 2006;47:C13-8. [Crossref] [PubMed]
- Gulati M, Levy PD, Mukherjee D, Amsterdam E, Bhatt DL, Birtcher KK, et al. 2021 AHA/ACC/ASE/CHEST/SAEM/SCCT/SCMR Guideline for the Evaluation and Diagnosis of Chest Pain: A Report of the American College of Cardiology/American Heart Association Joint Committee on Clinical Practice Guidelines. Circulation 2021;144:e368-454. [Crossref] [PubMed]
- Marwan M, Taher MA, El Meniawy K, Awadallah H, Pflederer T, Schuhbäck A, Ropers D, Daniel WG, Achenbach S. In vivo CT detection of lipid-rich coronary artery atherosclerotic plaques using quantitative histogram analysis: a head to head comparison with IVUS. Atherosclerosis 2011;215:110-5. [Crossref] [PubMed]
- Feuchtner G, Kerber J, Burghard P, Dichtl W, Friedrich G, Bonaros N, Plank F. The high-risk criteria low-attenuation plaque <60 HU and the napkin-ring sign are the most powerful predictors of MACE: a long-term follow-up study. Eur Heart J Cardiovasc Imaging. 2017;18:772-9. [Crossref] [PubMed]
- Kristensen TS, Kofoed KF, Kühl JT, Nielsen WB, Nielsen MB, Kelbæk H. Prognostic implications of nonobstructive coronary plaques in patients with non-ST-segment elevation myocardial infarction: a multidetector computed tomography study. J Am Coll Cardiol 2011;58:502-9. [Crossref] [PubMed]
- Antonopoulos AS, Sanna F, Sabharwal N, Thomas S, Oikonomou EK, Herdman L, et al. Detecting human coronary inflammation by imaging perivascular fat. Sci Transl Med 2017;9:eaal2658. [Crossref] [PubMed]
- Hedgire S, Baliyan V, Zucker EJ, Bittner DO, Staziaki PV, Takx RAP, Scholtz JE, Meyersohn N, Hoffmann U, Ghoshhajra B. Perivascular Epicardial Fat Stranding at Coronary CT Angiography: A Marker of Acute Plaque Rupture and Spontaneous Coronary Artery Dissection. Radiology 2018;287:808-15. [Crossref] [PubMed]
- Goeller M, Achenbach S, Cadet S, Kwan AC, Commandeur F, Slomka PJ, Gransar H, Albrecht MH, Tamarappoo BK, Berman DS, Marwan M, Dey D. Pericoronary Adipose Tissue Computed Tomography Attenuation and High-Risk Plaque Characteristics in Acute Coronary Syndrome Compared With Stable Coronary Artery Disease. JAMA Cardiol 2018;3:858-63. [Crossref] [PubMed]
- Yu W, Chen Y, Zhang F, Liu B, Wang J, Shao X, Yang X, Shi Y, Wang Y. Association of epicardial adipose tissue volume with increased risk of hemodynamically significant coronary artery disease. Quant Imaging Med Surg 2023;13:2582-93. [Crossref] [PubMed]
- Cury RC, Leipsic J, Abbara S, Achenbach S, Berman D, Bittencourt M, et al. CAD-RADS™ 2.0 - 2022 Coronary Artery Disease-Reporting and Data System: An Expert Consensus Document of the Society of Cardiovascular Computed Tomography (SCCT), the American College of Cardiology (ACC), the American College of Radiology (ACR), and the North America Society of Cardiovascular Imaging (NASCI). J Cardiovasc Comput Tomogr 2022;16:536-57. [Crossref] [PubMed]
- Qin B, Li Z, Zhou H, Liu Y, Wu H, Wang Z. The Predictive Value of the Perivascular Adipose Tissue CT Fat Attenuation Index for Coronary In-stent Restenosis. Front Cardiovasc Med 2022;9:822308. [Crossref] [PubMed]
- Yi Y, Xu C, Guo N, Sun J, Lu X, Yu S, Wang Y, Vembar M, Jin Z, Wang Y. Performance of an Artificial Intelligence-based Application for the Detection of Plaque-based Stenosis on Monoenergetic Coronary CT Angiography: Validation by Invasive Coronary Angiography. Acad Radiol 2022;29:S49-58. [Crossref] [PubMed]
- Fu F, Wei J, Zhang M, Yu F, Xiao Y, Rong D, Shan Y, Li Y, Zhao C, Liao F, Yang Z, Li Y, Chen Y, Wang X, Lu J. Rapid vessel segmentation and reconstruction of head and neck angiograms using 3D convolutional neural network. Nat Commun 2020;11:4829. [Crossref] [PubMed]
- Westra J, Sejr-Hansen M, Koltowski L, Mejía-Rentería H, Tu S, Kochman J, Zhang Y, Liu T, Campo G, Hjort J, Mogensen LJH, Erriquez A, Andersen BK, Eftekhari A, Escaned J, Christiansen EH, Holm NR. Reproducibility of quantitative flow ratio: the QREP study. EuroIntervention 2022;17:1252-9. [Crossref] [PubMed]
- Knuuti J, Wijns W, Saraste A, Capodanno D, Barbato E, Funck-Brentano C, et al. 2019 ESC Guidelines for the diagnosis and management of chronic coronary syndromes. Eur Heart J 2020;41:407-77. [Crossref] [PubMed]
- Hoffmann U, Moselewski F, Nieman K, Jang IK, Ferencik M, Rahman AM, Cury RC, Abbara S, Joneidi-Jafari H, Achenbach S, Brady TJ. Noninvasive assessment of plaque morphology and composition in culprit and stable lesions in acute coronary syndrome and stable lesions in stable angina by multidetector computed tomography. J Am Coll Cardiol 2006;47:1655-62. [Crossref] [PubMed]
- Lin A, Kolossváry M, Cadet S, McElhinney P, Goeller M, Han D, Yuvaraj J, Nerlekar N, Slomka PJ, Marwan M, Nicholls SJ, Achenbach S, Maurovich-Horvat P, Wong DTL, Dey D. Radiomics-Based Precision Phenotyping Identifies Unstable Coronary Plaques From Computed Tomography Angiography. JACC Cardiovasc Imaging 2022;15:859-71. [Crossref] [PubMed]
- Tonino PA, Fearon WF, De Bruyne B, Oldroyd KG, Leesar MA, Ver Lee PN, Maccarthy PA, Van't Veer M, Pijls NH. Angiographic versus functional severity of coronary artery stenoses in the FAME study fractional flow reserve versus angiography in multivessel evaluation. J Am Coll Cardiol 2010;55:2816-21. [Crossref] [PubMed]
- Nadjiri J, Hausleiter J, Jähnichen C, Will A, Hendrich E, Martinoff S, Hadamitzky M. Incremental prognostic value of quantitative plaque assessment in coronary CT angiography during 5 years of follow up. J Cardiovasc Comput Tomogr 2016;10:97-104. [Crossref] [PubMed]
- Dong L, Mintz GS, Witzenbichler B, Metzger DC, Rinaldi MJ, Duffy PL, Weisz G, Stuckey TD, Brodie BR, Yun KH, Xu K, Kirtane AJ, Stone GW, Maehara A. Comparison of plaque characteristics in narrowings with ST-elevation myocardial infarction (STEMI), non-STEMI/unstable angina pectoris and stable coronary artery disease (from the ADAPT-DES IVUS Substudy). Am J Cardiol 2015;115:860-6. [Crossref] [PubMed]
- Li N, Dong X, Zhu C, Shi K, Si N, Shi Z, Pan H, Wang S, Zhao M, Zhang T. Model development and validation of noninvasive parameters based on coronary computed tomography angiography to predict culprit lesions in acute coronary syndromes within 3 years: value of plaque characteristics, hemodynamics and pericoronary adipose tissue. Quant Imaging Med Surg 2023;13:4325-38. [Crossref] [PubMed]
- Yan H, Zhao N, Geng W, Hou Z, Gao Y, Lu B. Pericoronary fat attenuation index and coronary plaque quantified from coronary computed tomography angiography identify ischemia-causing lesions. Int J Cardiol 2022;357:8-13. [Crossref] [PubMed]
- Emfietzoglou M, Mavrogiannis MC, García-García HM, Stamatelopoulos K, Kanakakis I, Papafaklis MI. Current Toolset in Predicting Acute Coronary Thrombotic Events: The "Vulnerable Plaque" in a "Vulnerable Patient" Concept. Life (Basel) 2023;13:696. [Crossref] [PubMed]
- Mura M, Della Schiava N, Long A, Chirico EN, Pialoux V, Millon A. Carotid intraplaque haemorrhage: pathogenesis, histological classification, imaging methods and clinical value. Ann Transl Med 2020;8:1273. [Crossref] [PubMed]
- Naghavi M, Libby P, Falk E, Casscells SW, Litovsky S, Rumberger J, et al. From vulnerable plaque to vulnerable patient: a call for new definitions and risk assessment strategies: Part I. Circulation 2003;108:1664-72. [Crossref] [PubMed]
- Sagris M, Antonopoulos AS, Simantiris S, Oikonomou E, Siasos G, Tsioufis K, Tousoulis D. Pericoronary fat attenuation index-a new imaging biomarker and its diagnostic and prognostic utility: a systematic review and meta-analysis. Eur Heart J Cardiovasc Imaging 2022;23:e526-36. [Crossref] [PubMed]
- Lin A, Nerlekar N, Yuvaraj J, Fernandes K, Jiang C, Nicholls SJ, Dey D, Wong DTL. Pericoronary adipose tissue computed tomography attenuation distinguishes different stages of coronary artery disease: a cross-sectional study. Eur Heart J Cardiovasc Imaging 2021;22:298-306. [Crossref] [PubMed]
- Huang M, Han T, Nie X, Zhu S, Yang D, Mu Y, Zhang Y. Clinical value of perivascular fat attenuation index and computed tomography derived fractional flow reserve in identification of culprit lesion of subsequent acute coronary syndrome. Front Cardiovasc Med 2023;10:1090397. [Crossref] [PubMed]
- Arbab-Zadeh A, Fuster V. From Detecting the Vulnerable Plaque to Managing the Vulnerable Patient: JACC State-of-the-Art Review. J Am Coll Cardiol 2019;74:1582-93. [Crossref] [PubMed]
- Tomaniak M, Katagiri Y, Modolo R, de Silva R, Khamis RY, Bourantas CV, et al. Vulnerable plaques and patients: state-of-the-art. Eur Heart J 2020;41:2997-3004. [Crossref] [PubMed]
- Tavoosi A, Kadoya Y, Chong AY, Small GR, Chow BJW. Utility of FFRCT in Patients with Chest Pain. Curr Atheroscler Rep 2023;25:427-34. [Crossref] [PubMed]
- Dey D, Slomka PJ, Leeson P, Comaniciu D, Shrestha S, Sengupta PP, Marwick TH. Artificial Intelligence in Cardiovascular Imaging: JACC State-of-the-Art Review. J Am Coll Cardiol 2019;73:1317-35. [Crossref] [PubMed]
- Cau R, Flanders A, Mannelli L, Politi C, Faa G, Suri JS, Saba L. Artificial intelligence in computed tomography plaque characterization: A review. Eur J Radiol 2021;140:109767. [Crossref] [PubMed]