Reproducibility of automatic adipose tissue segmentation using proton density fat fraction images between 1.5 and 3.0 T magnetic resonance
Introduction
The distribution of whole-body fat is an important indicator of metabolic health and disease (1). Accumulation of adipose tissue in the abdomen, particularly visceral fat, can increase the risk of heart disease, type 2 diabetes mellitus, cancer, and other diseases (2). Conversely, gluteal and femoral fat is associated with improved cardiovascular metabolic function (3,4). These conflicting relationships highlight the unique properties of various fat depots. As a result, measuring the distribution of fat throughout the whole-body is vital to predict metabolic profiles (5). Traditional anthropometric indices such as body mass index (BMI), waist circumference, and waist-hip ratio have been commonly used to evaluate the fat accumulation in the body (6). However, these measurements provide little information regarding to the adipose tissue amount or distribution, and therefore lack of specificity in reflecting metabolic disorders and predicting metabolic dysfunction-related diseases. Bioelectrical impedance analysis, hydrodensitometry, and air displacement plethysmography are able to measure the amount of whole-body fat, but these methods cannot assess the distribution of individual fat depots (7,8).
In the last decades, medical imaging techniques, including dual-energy X-ray absorptiometry (DXA), computed tomography (CT), and magnetic resonance imaging (MRI), have become powerful tools to visualize the distribution of adipose tissue and quantify fat deposition in organs such as the liver (9-11). However, DXA and CT are unsuitable for longitudinal studies due to the radiation exposure (12), especially in pediatric and elderly populations. In comparison, MRI is able to separate proton signals from water and fat directly based on the chemical shift-encoded Dixon method (13), and measure the adipose tissue volume quantitatively. In particular, the proton density fat fraction (PDFF) image calculated from six- or more points chemical shift-encoded MRI has been shown to map fat infiltration in organs such as the liver, and volume of adipose tissue with excellent accuracy and reproducibility across different field strengths, vendors, and imaging parameters in many clinical studies (14-16).
Although adipose tissue can be easily identified from fat-only images or fat fraction (FF) images, more efforts need to be made to recognize and classify various types of adipose tissue all over the body. Semi-automatic and automatic methods have been proposed to facilitate the segmentation and quantification of different adipose tissue depots in the abdominal or whole-body images (17). Recently, several studies have reported a cloud-based analysis service provided by AMRA Researcher (AMRA Medical AB, Linköping, Sweden), to measure the adipose tissue distribution and other body composition (18-20) based on a pre-defined multi-atlas dataset built upon a population consisting of 10 healthy adults (19,21) with good reproducibility and repeatability (22). However, further semi-automatic verification and manual correction procedures were necessary to adjust and approve the final segmentation. Besides, the analysis is limited to the adipose tissue distribution in the abdominal region; the information about adipose tissue in other parts was absent.
Deep learning (DL)-based adipose tissue segmentation methods have shown great performance and efficacy without any manual involvement for adipose tissue distribution analysis (23,24). Huber et al. developed an automated segmentation method using a U-net network based on fat-only images, and demonstrated robustness between different imaging parameters and scanners (25). Küstner et al. proposed a three-dimensional (3D) densely connected convolutional neural network for robust segmentation of subcutaneous adipose tissue (SAT) and visceral adipose tissue (VAT) with a transfer learning scheme (26). Haueise et al. assessed the spatial distribution of VAT and SAT in the trunk using a 3.0 T magnetic resonance (MR) scanner, demonstrating the feasibility of DL segmentation in a large population-based cohort (27). Kafali et al. developed automated abdominal SAT/VAT segmentation on longitudinal MRI in adults with overweight/obesity using attention-based competitive dense 3D U-Net and 3D nnU-Net (28), whereas Kway et al. proposed an automated segmentation method for accurate quantification of in neonates and young children using a U-net network (29). However, the performance of these methods might be compromised by the image inhomogeneity resulting from the use of the qualitative T1-weighted images. Comparatively, the PDFF image is free of inhomogeneity problems, and can provide the quantitative fat deposition information simultaneously. Based on PDFF images, some previous studies have achieved the segmentation of abdominal adipose tissue using DL methods (30-32), whereas Wang et al. proposed a DL-based automatic network for whole-body adipose tissue segmentation and distribution quantification (33). By using this network, the SAT and internal adipose tissue (IAT) of the whole-body, covering from neck to knee, was automatically identified accurately and effectively using a 3.0 T MR scanner in a single center. As a result, adipose tissue segmentation and quantification using the DL model has been demonstrated as a reliable method for adipose tissue distribution analysis.
However, for a method to be clinically applicable, the “reproducibility” of the DL-based adipose tissue segmentation model must be evaluated carefully. The “reproducibility” refers to the degree of agreement between measurements taken from the same subject under varying conditions, such as different scanners or field strengths with different radiofrequency (RF) coils, and as a consequence, differences in imaging parameters such as repetition time (TR), echo time (TE) and resolution, as well as the difference in volunteer positioning. These factors would introduce variability into the adipose tissue quantification using an automatic DL-based segmentation method. Performing reproducibility analysis would ensure reliable and consistent results across diverse settings, which allows for data integration over time and across different equipment in multicenter and multi-device cohort studies (22). However, the reproducibility of adipose tissue distribution analysis using the DL-based model has not been formally assessed. Therefore, the aim of this work was to evaluate the reproducibility of the DL-based adipose tissue distribution analysis method across different MR field strengths, 1.5 and 3.0 T, through a prospective, multicenter study, and so establish a reproducible and objective method for adipose tissue quantification. We present this article in accordance with the GRRAS reporting checklist (available at https://qims.amegroups.com/article/view/10.21037/qims-24-1306/rc).
Methods
Volunteers
This study was approved by the Institutional Review Board of Shenzhen Institutes of Advanced Technology, Chinese Academy of Sciences (approval No. IRB-210115-H0551). The study was conducted in accordance with the Declaration of Helsinki (as revised in 2013). A priori prospective power analyses revealed that a total number of 24 participants would be required using a G*power program to ensure sufficient power (1 − β =0.8) at a statistical significance level of P≤0.05 by setting the effect size to 0.6 (34). Therefore, 24 adult Chinese volunteers were recruited to participate in the whole-body MR scans and provided written informed consent. The exclusion criterion was contraindication to MRI.
MRI acquisition
All volunteers underwent image acquisitions at both 1.5 T (uMR660, Shanghai United Imaging Healthcare, Shanghai, China) and 3.0 T (uMR790, Shanghai United Imaging Healthcare) clinical MRI scanners at two separated sites with median interval of 1 week. The volunteers were asked to fast for 4 hours prior to the scans. The anthropometric measures (height, weight, waist circumference, and hip circumference) were recorded before each MR scan. According to the height of the volunteers, seven or eight overlapping axial image stacks were obtained covering the neck-to-knee areas for whole-body scan. A 3D RF spoiled gradient echo (GRE) sequence with six echoes was used for image acquisition. The details of imaging parameters are listed in Table 1, and the coil configuration of each stack is shown in supplemental material Figure S1. Low flip angle (3°) was used to mitigate T1-bias for accurate PDFF quantification. To avoid motion artifacts caused by respiration, volunteers were asked to hold their breath in the end-expiratory period for less than 15 seconds during the chest and upper abdomen scan. A respiration belt was used to monitor the volunteer’s breath-holding condition and the image quality was validated in-line by a radiologist with more than 10 years of experience. If obvious respiratory artifacts were present in abdominal PDFF images, another scan of the imaging stack was repeated immediately. The total scan time for each volunteer was less than 5 minutes.
Table 1
Scan parameters | Site 1 | Site 2 |
---|---|---|
MRI platform | 1.5 T (uMR660) | 3.0 T (uMR790) |
Imaging sequence | 6-echo GRE | 6-echo GRE |
TE1/∆TE (milliseconds) | 1.51/1.51 unipolar | 1.49/1.52 unipolar |
TR (milliseconds) | 11.1 | 11 |
Flip angle (°) | 3 | 3 |
Field of view (cm2) | 400×300 | 400×300 |
Pixel resolution (mm2) | 2.27×2.27 | 2.08×2.08 |
Thickness (mm) | 6.0 | 6.0 |
Slices/slab and acquisition time (seconds) | ||
Neck to pelvic (breath-hold) | 24/12.8 | 24/14.3 |
Pelvic to knee (free breathing) | 24–40/12.8–21.7 | 24–48/14.3–31.0 |
Acceleration factor | Parallel imaging/2.4 | Parallel imaging/2.4 |
Coil | 12 channel body coil ×2 | 12 channel body coil ×2 |
8 channel flexible coil ×1 | 8 channel flexible coil ×1 | |
Bandwidth (Hz)/pixel | 900 | 900 |
MRI, magnetic resonance imaging; GRE, gradient echo; TE1, the first echo time; ∆TE, echo spacing; TR, repetition time.
PDFF calculation
The PDFF images were reconstructed by using the commercial fat-water separation algorithm “FACT” (fat analysis and calculation technique; Shanghai United Imaging Healthcare) procedure based on the multi-echo GRE images. In short, water- and fat-only images were firstly obtained from these multi-echo GRE images using the fat-water separation algorithm with multiple fat peak model, and T2* decay considered and corrected, where a novel region-growing method with multi-resolution self-feeding phasor estimation was adopted to promote robust separation (35), and then the PDFF images were generated from these separated water- and fat-only images.
Automatic adipose tissue distribution analysis
The whole-body mask images were first generated by thresholding on the multi-echo magnitude images. A maximum magnitude projection (MMP) image was firstly calculated by taking the maximum magnitude of the six-echo source images for each pixel. The threshold was then set to the maximum value of the MMP image multiplied by an empirical factor of 0.05. As a result, pixels with a value smaller than the threshold in the MMP image were set as background region and excluded from the subsequent segmentation. The total adipose tissue (TAT) was defined as the regions where FF >30% from the PDFF images. PDFF images were then input to a previously proposed DL model, designed based on the U-Net network, which comprises 23 convolutional layers (including 18 3×3 convolution layers, four 2×2 up convolution layers, and one 1×1 convolution layer) and four 2×2 maximum pooling operations, to generate the SAT and IAT mask images automatically (33). Since the arms were cropped manually and not included in the training procedure, the arms in the segmented mask images were also cropped automatically. The volumes of the whole body (WH), TAT, SAT, and IAT were calculated by multiplying the number of voxels in the corresponding mask images with the pixel resolution and slice thickness, for example, 2.27×2.27×6.0 mm3 for 1.5 T images and 2.08×2.08×6.0 mm3 for 3.0 T images. It should be noted that the low signal regions, such as lung region, intestine, and so on, in whole-body mask imaging was filled when the WH volume was calculated. The volume ratios of TAT/WH, SAT/WH, IAT/WH, SAT/TAT, and IAT/TAT were also compared between the two scanners.
Due to the conflicting relationships of abdominal fat and gluteal and femoral fat with health risks, the whole-body images were further divided into three subparts manually, and the reproducibility of adipose tissue distribution for each subpart was also evaluated. The thorax subpart was defined as the region covering from neck to diaphragm, whereas the abdomen subpart was from the diaphragm to the upper edge of the femoral heads, and the thigh subpart was from the upper edge of the femoral head to the knee. The volume of TAT, SAT, and IAT and the corresponding volume ratio of each subpart were then evaluated and compared between the two scanners. Additionally, the reproducibility analyses of PDFF values for SAT and IAT were also performed across scanners.
Statistical analysis
Pearson correlation coefficient (R) was used to assess the correlation between whole-body adipose tissue distribution measurements using PDFF images obtained by different imaging scanners. The paired t-test was adopted to evaluate the differences in the whole-body adipose tissue distribution measurements. A two-way, mixed-model, single-measure intraclass correlation coefficient (ICC) model was used to assess the absolute agreement in these volume measurements. Bland-Altman plots were used to visualize the reproducibility of PDFF measurements.
Pearson correlation coefficients were classified by using the following definitions: 0–0.19, very weak; 0.2–0.39, weak; 0.40–0.59, moderate; 0.60–0.79, strong; and 0.80–1.0, very strong. ICC strength was classified using the following definitions: 0–0.39, poor; 0.40–0.59, fair; 0.60–0.74, good; and 0.75–1.0, excellent. For all statistical tests, significance was assumed at a level of P<0.05 and 95% confidence intervals (CIs) were calculated. The statistical analyses were performed using the software SPSS 23.0 (IBM Corp., Armonk, NY, USA). The Bland-Altman and linear regression plots were drawn using GraphPad Prism 8.0.2 (GraphPad Software, San Diego, CA, USA).
Results
The anthropometrics of the volunteers are shown in Table 2. The representative PDFF images acquired on both scanners from two volunteers are shown in Figure 1 with the corresponding TAT mask images and SAT mask images segmented by the DL model. There was no significant difference between the 1.5 and 3.0 T images.
Table 2
Anthropometries | Volunteer | |
---|---|---|
Male (n=13) | Female (n=11) | |
Age (years) | 25.00 (22.00, 38.00) | 23.00 (22.00, 35.00) |
Weight (kg) | 71.10 (54.80, 97.00) | 62.60 (44.50, 79.70) |
Height (cm) | 175.00 (161.00, 182.00) | 170.00 (156.00, 177.00) |
BMI (kg/m2) | 23.50 (20.75, 29.94) | 21.60 (17.48, 29.27) |
Waist (cm) | 85.00 (79.00, 107.00) | 78.00 (65.00, 92.00) |
Hip (cm) | 100.00 (90.00, 112.00) | 96.00 (86.00, 113.00) |
WHR | 0.88 (0.76, 0.99) | 0.78 (0.74, 0.83) |
Data are expressed as median (minimum, maximum). BMI, body mass index; WHR, waist-to-hip ratio.
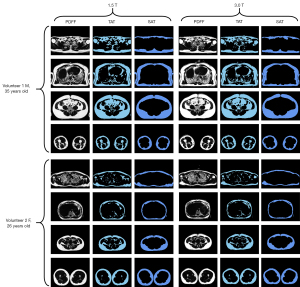
The profiles of the body adiposity along the head-foot axis were calculated and presented in Figure 2 for all 24 volunteers using 3.0 T images. Due to the difference in body size, the axis PDFF images of each volunteer, ranging from 153 to 177 slices, were interpolated into 200 images from neck to knee. Figure 2A shows the mean volumes of WH, TAT, SAT, and IAT, whereas the volume ratios of TAT/WH and SAT/WH are demonstrated in Figure 2C. Figure 2D shows the profiles of SAT/TAT for male and female subjects separately, indicating that females are more inclined to store SAT. A reconstructed coronal PDFF image of one female (BMI =21.9 kg/m2) is shown in Figure 2B. The volumes of adipose tissue for reproducibility analysis were calculated using the original number of slices acquired from MR scanners without interpolation.
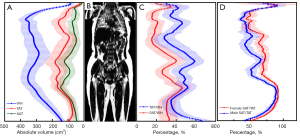
The mean volume of all volunteers for WH, TAT, SAT, and IAT acquired between 1.5 and 3.0 T scanners are listed in Table 3 with values [mean ± standard deviation (SD)] of 47,768.16±8,431.48/47,775.05±8,415.37 cm3 (P=0.95), 23,108.52±6,995.87/23,175.73±6,988.00 cm3 (P=0.40), 13,486.48±4,810.63/13,467.17±4,777.44 cm3 (P=0.74), and 8,964.00±3,699.56/8,982.84±3,681.61 cm3 (P=0.69), respectively. The volume ratio of TAT/WH, SAT/WH, IAT/WH, SAT/TAT, and IAT/SAT are also calculated and listed in Table 3. The ICC and Pearson correlation coefficient of these volumes and volume ratios between the two scanners ranged from 0.995 to 0.999, indicating excellent agreement. The P values of the paired t-test for all comparisons were all larger than 0.05, showing no significant difference.
Table 3
Parameters | 1.5 T | 3.0 T | ICC | R | P value |
---|---|---|---|---|---|
WH volume (cm3) | 47,768.16±8,431.48 | 47,775.05±8,415.37 | 0.998 | 0.998 | 0.95 |
TAT volume (cm3) | 23,108.52±6,995.87 | 23,175.73±6,988.00 | 0.999 | 0.999 | 0.40 |
SAT volume (cm3) | 13,486.48±4,810.63 | 13,467.17±4,777.44 | 0.998 | 0.998 | 0.74 |
IAT volume (cm3) | 8,964.00±3,699.56 | 8,982.84±3,681.61 | 0.998 | 0.998 | 0.69 |
TAT/WH volume ratio | 0.48±0.09 | 0.47±0.09 | 0.996 | 0.996 | 0.42 |
SAT/WH volume ratio | 0.28±0.08 | 0.28±0.08 | 0.997 | 0.997 | 0.72 |
IAT/WH volume ratio | 0.19±0.06 | 0.19±0.06 | 0.998 | 0.998 | 0.74 |
SAT/TAT volume ratio | 0.58±0.08 | 0.58±0.08 | 0.995 | 0.996 | 0.11 |
IAT/TAT volume ratio | 0.37±0.11 | 0.37±0.11 | 0.999 | 0.999 | 0.57 |
Data are expressed as mean ± SD. P<0.05 was considered statistical significance. ICC, intraclass correlation coefficient; R, Pearson correlation coefficient; WH, whole body; TAT, total adipose tissue; SAT, subcutaneous adipose tissue; IAT, internal adipose tissue; SD, standard deviation.
Figure 3 shows the Bland-Altman plots for comparison of the volume of WH, TAT, SAT, and IAT acquired at two sites with the mean bias obtained by subtracting the results from the 3.0 T scanner from those of the 1.5 T scanner for four indexes: −6.89, −67.21, 19.31, and −18.84 cm3, respectively, whereas the intervals defined by the mean difference ±1.96 SD (i.e., 95% limits of agreement) were −1,029.76 to 1,015.98, −812.55 to 678.14, −522.53 to 561.16, and −460.18 to 422.50 cm3, respectively.
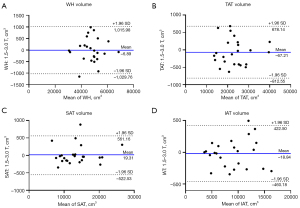
Figure 4 shows the linear regression analysis of the volumes of WH, TAT, SAT, and IAT measured between 1.5 and 3.0 T. The regression showed high correlations in these four indices with R2=0.996, R2=0.997, R2=0.997, and R2=0.993 (P<0.0001) between the two sites. The slopes for four comparisons were almost close to one, with intercept of the regression equitation being 189.60, 127.32, 95.70, and 78.83 cm3, respectively.
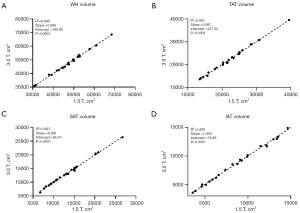
We calculated the mean and SD of PDFF values for SAT and IAT of WH and three subparts, and performed the Bland-Altman analysis to evaluate the reproducibility across scanners. The mean and SD of SAT for 1.5 and 3.0 T MR scanners for WH, thorax, abdomen, and thigh were 94.90%±9.03% and 95.09%±8.38% (P=0.219), 92.13%±13.52% and 92.43%±12.15% (P=0.118), 95.56%±7.07% and 95.48±6.31% (P=0.494), and 94.68%±8.04% and 94.44%±7.91% (P=0.186), respectively. In contrast, these values for IAT were 61.54%±21.68% and 61.19%±22.00% (P=0.136), 53.75%±20.67% and 53.30%±21.31% (P=0.150), 62.84%±20.71% and 62.26%±21.00% (P=0.255), and 68.19%±22.63% and 67.79%±23.11% (P=0.169), respectively. These values are plotted in the supplemental material Figure S2. As shown, the SAT PDFF was more homogeneous with a lower SD than IAT. Figures 5,6 show the Bland-Altman plots for comparison of the PDFF values of SAT and IAT. The results demonstrated good reproducibility of PDFF values, with the mean bias between the 1.5 and 3.0 T scanners for whole-body, thorax, abdomen, and thigh being −0.19%, −0.30%, 0.086%, and 0.24% for SAT, respectively, as well as 0.35%, 0.46%, 0.58%, and 0.40% for IAT, respectively.
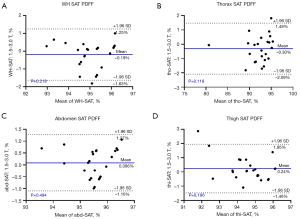
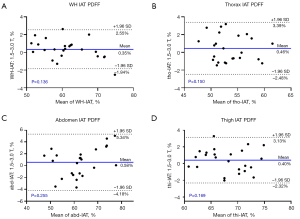
Figure 7 shows the plots of the whole-body tissue adipose volume ratio (TAT/WH, SAT/WH, IAT/WH, SAT/TAT, and IAT/TAT) for all volunteers at 1.5 and 3.0 T. No significant differences were found among these comparisons: TAT/WH with P=0.421, SAT/WH with P=0.721, IAT/WH with P=0.742, SAT/TAT with P=0.107, and IAT/TAT with P=0.565.
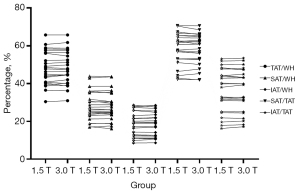
The comparisons of the volumes and volume ratios among the three subparts of thorax, abdomen, and thigh are shown in Table 4. Similar to the results of whole-body, the ICC and R of these indexes between the two scanners were close to 1.0, indicating excellent agreement. However, there existed significant differences in IAT, TAT/WH ratio, and SAT/TAT ratio in the thorax subpart with the values (mean ± SD) of 1,701.34±698.56 and 1,837.35±726.56 cm3 (P<0.05), 0.44±0.10 and 0.44±0.10 (P<0.05), and 0.45±0.07 and 0.43±0.06 (P<0.05) for 1.5 and 3.0 T scanners, respectively, whereas good reproducibility was demonstrated in abdomen and thigh subparts.
Table 4
Parameters | 1.5 T | 3.0 T | ICC | R | P value |
---|---|---|---|---|---|
Thorax (neck to diaphragm) | |||||
WH volume (cm3) | 10,702.08±1,937.36 | 10,663.91±2,023.77 | 0.982 | 0.983 | 0.62 |
TAT volume (cm3) | 4,635.30±1,296.55 | 4,692.89±1,241.29 | 0.986 | 0.987 | 0.20 |
SAT volume (cm3) | 2,085.89±798.63 | 2,070.89±771.47 | 0.992 | 0.993 | 0.46 |
IAT volume (cm3) | 1,701.34±698.56 | 1,837.35±726.56 | 0.990 | 0.991 | <0.05 |
TAT/WH volume ratio | 0.44±0.10 | 0.44±0.10 | 0.987 | 0.987 | <0.05 |
SAT/WH volume ratio | 0.20±0.07 | 0.20±0.07 | 0.997 | 0.997 | 0.94 |
IAT/WH volume ratio | 0.16±0.06 | 0.17±0.06 | 0.991 | 0.991 | <0.05 |
SAT/TAT volume ratio | 0.45±0.07 | 0.43±0.06 | 0.948 | 0.956 | <0.05 |
IAT/TAT volume ratio | 0.38±0.15 | 0.40±0.15 | 0.995 | 0.995 | <0.05 |
Abdomen (diaphragm to caput femoris) | |||||
WH volume (cm3) | 19,871.99±4,752.74 | 19,953.02±4,735.82 | 0.998 | 0.998 | 0.21 |
TAT volume (cm3) | 10,692.39±4,048.15 | 10,693.95±4,096.50 | 0.999 | 0.999 | 0.97 |
SAT volume (cm3) | 5,271.26±2,371.09 | 5,256.39±2,407.12 | 0.998 | 0.998 | 0.62 |
IAT volume (cm3) | 4,499.09±2,113.41 | 4,470.27±2,091.33 | 0.998 | 0.998 | 0.33 |
TAT/WH volume ratio | 0.53±0.09 | 0.52±0.09 | 0.989 | 0.989 | 0.32 |
SAT/WH volume ratio | 0.26±0.07 | 0.26±0.07 | 0.994 | 0.994 | 0.16 |
IAT/WH volume ratio | 0.22±0.07 | 0.22±0.07 | 0.993 | 0.993 | 0.24 |
SAT/TAT volume ratio | 0.49±0.08 | 0.49±0.08 | 0.992 | 0.992 | 0.25 |
IAT/TAT volume ratio | 0.42±0.12 | 0.42±0.12 | 0.996 | 0.996 | 0.55 |
Thigh (caput femoris to knee) | |||||
WH volume (cm3) | 17,126.90±2,784.52 | 17,158.12±2,763.72 | 0.993 | 0.993 | 0.65 |
TAT volume (cm3) | 7,780.83±2,127.00 | 7,788.89±2,073.19 | 0.996 | 0.996 | 0.84 |
SAT volume (cm3) | 6,136.27±1,902.50 | 6,146.44±1,837.79 | 0.996 | 0.997 | 0.76 |
IAT volume (cm3) | 1,294.93±433.88 | 1,276.43±411.96 | 0.987 | 0.989 | 0.19 |
TAT/WH volume ratio | 0.45±0.09 | 0.45±0.09 | 0.996 | 0.997 | 0.84 |
SAT/WH volume ratio | 0.36±0.09 | 0.36±0.09 | 0.997 | 0.997 | 0.77 |
IAT/WH volume ratio | 0.08±0.02 | 0.07±0.02 | 0.985 | 0.989 | 0.09 |
SAT/TAT volume ratio | 0.78±0.10 | 0.79±0.10 | 0.995 | 0.995 | 0.67 |
IAT/TAT volume ratio | 0.17±0.06 | 0.17±0.05 | 0.989 | 0.992 | 0.12 |
Data are expressed as mean ± SD. P<0.05 was considered statistical significance. ICC, intraclass correlation coefficient; R, Pearson correlation coefficient; WH, whole body; TAT, total adipose tissue; SAT, subcutaneous adipose tissue; IAT, internal adipose tissue; SD, standard deviation.
Discussion
Analysis of the whole-body adipose tissue distribution based on MRI has become a viable tool in obesity management. Reproducibility evaluation of the automatic segmentation and quantification based on DL is necessary before this useful tool can be applied in clinical studies. In the present study, datasets of 24 volunteers acquired at both 1.5 and 3.0 T MR scanners were compared, and it is demonstrated that the quantitative adipose tissue distribution features by the automatic method are consistent with good reproducibility between the two scanners. The mean bias for the volumes of WH, TAT, SAT, and IAT were −6.89 cm3 (P=0.95), −67.21 cm3 (P=0.40), 19.31 cm3 (P=0.74), and −18.84 cm3 (P=0.69), respectively. Good reproducibility performances were also found in each subpart, except for the indices of IAT volume, TAT/WH ratio, and SAT/TAT ratio in the thorax due to different susceptibility effects across MR strengths.
Whole-body adipose tissue distribution analysis enables a better understanding of the relationship between adipose tissue distribution and metabolic disease. Previous studies have demonstrated that fat accumulation in VAT is strongly associated with increased metabolic risk (36), whereas adipose tissue in the lower-body depots provides protection against metabolic derangement. For example, some studies have reported that the lower-body adipose tissue exhibited a less inflammatory profile than VAT (37,38). As a result, an accurate characterization of whole-body adipose tissue distribution is highly desirable to properly evaluate individual metabolic disease risk. Comparatively, some previous segmentation methods have focused only on specific parts of the body, such as abdominal and upper-body fat, and muscle distribution (22,27,29,32). In the present study, the whole-body adipose tissue distribution from the neck to knee is studied and segmented into SAT and IAT. The quantitative measurements of the adipose tissue distribution were then evaluated in the WH and three subparts of the thorax, abdomen, and thigh, respectively. As stated in a review article, the lipid turnover was significantly reduced in lower-body adipose tissue with fewer signs of inflammatory insult than upper-body adipose tissue (3). As a result, whole-body adipose tissue distribution analysis provides a visualization tool to study the evolution of lipid storage in different fat compartments of individuals with metabolically healthy obesity and metabolically unhealthy obesity.
Adipose tissue distribution measurements on whole-body and subparts showed good agreement between 1.5 and 3.0 T, except for the IAT volume, TAT/WH ratio, and SAT/TAT ratio in the thorax region. The potential reason may be that the IAT volume in this subpart was quite small, and the quantitative results were vulnerable to the different susceptibility effects due to respiration at 3.0 T (39). As a result, developing advanced acquisition methods or employing ultrashort TE (UTE) imaging would help to minimize the variability in adipose tissue distribution analysis around the lung area across different field strengths.
Multicenter, large population-based cohort studies provide massive databases for the assessment of whole-body adipose tissue distribution using MR images, in which accurate, fast, and reproducible adipose tissue segmentation methods are necessary for whole-body volume quantification of individual fat compartments. Manual segmentation is time-consuming, laborious, and not feasible in large cohort studies, especially for whole-body adipose tissue analysis. In contrast, the DL-based automatic adipose tissue segmentation method would be preferable due to its great efficiency and segmentation accuracy (30,31). For example, using our method, the segmentation time was less than 8 seconds per 100 images with a Dice similarity coefficient (DSC) for SAT of 0.976±0.048 (33). Similar prediction time and DSC were obtained in other recent DL-based studies (27,28). The impact of slice thickness, matrix resolution, and different coil settings on the reproducibility of DL-based methods in whole-body adipose tissue distribution analysis using a 3.0 T scanner was assessed in a previous study (25). However, the reproducibility of the DL-based method in different MR strengths was not reported. Our study demonstrates the feasibility and reproducibility of the proposed DL-based adipose tissue distribution analysis method at 1.5 and 3.0 T with different image resolution and imaging parameters.
In recent studies on adipose tissue segmentation and volume quantification, T1-weighted Dixon/water-suppressed images were commonly used to identify fat compartments due to the good signal contrast among adipose tissue and the surrounding tissue in the separated fat- and water-only images (22,27-29). However, the inhomogeneous sensitivity of the RF-coils would cause intensity variations in the T1-weighted fat- and water-only images, which compromises the performance of the segmentation methods, especially for whole-body images with multiple stacks (18). In our study, PDFF images were used to segment and analyze whole-body adipose tissue distribution, without the need for additional intensity non-uniformity correction, which would increase the accuracy of adipose tissue volume quantification. Besides, PDFF can also be used to quantify fat infiltration in liver, muscle, and other organs in further studies (40). Therefore, PDFF images are a good alternative to the T1-weighted Dixon images in assessing the whole-body adipose tissue distribution.
The PDFF images were calculated using our proposed fat-water separation algorithm, of which the performance was validated using the 2012 ISMRM challenge datasets (35). In the current study, there were no obvious water-fat swaps in all whole-body images. In our current implementation, a threshold of 30% was set to identify the adipose tissue. This threshold was set due to the fact that the PDFF values of bone marrow adipose tissue were commonly larger than 30% (41), whereas the PDFF values of fatty liver were lower than 30% (42). As a result, the bone marrow adipose tissue was considered a part of the TAT. We also investigated the effect of this PDFF threshold on TAT volume quantification of one participant, the result of which is shown in supplemental material Figure S3. Increasing the threshold led to a reduction in the calculated TAT volume. This reduction primarily arose from the exclusion of pixels along the boundaries between adipose tissue and surrounding tissues, influenced by partial volume effects, as well as the pixels of intermuscular fat and intramuscular fat. To further improve the quantification accuracy of adipose tissue, a more elaborate segmentation should be established to recognize more fat depots, such as bone marrow adipose tissue in spine and femur, muscle, liver, and pancreas.
A recent study evaluated the repeatability of the fat and lean tissues distribution analysis throughout the body using a 0.55 T MR scanner (43). In comparison with the commonly used 1.5 and 3.0 T systems, low-field MRI has many advantages, including lower cost, wider bore, better safety profile, and so on, which would promote MR-based whole-body adipose tissue distribution analysis to become a routine clinical screening protocol. Although adequate signal-to-noise-ratio (SNR) for segmentation and visualization were achieved in T1-weigthed Dixon images in the previous study, the effect of low SNR in low-field MRI should be further evaluated in PDFF images with low flip-angles. Besides, direct comparison and reproducibility assessment should also be conducted in both 0.55 T and high-field systems.
Although only young volunteers (ages from 22 to 38 years) were recruited to evaluate the reproducibility of the whole-body adipose tissue distribution using the DL-based segmentation method in the current study, the BMI covered a wide range from 17.48 to 29.94 kg/m2. This automatic method can be also applied to children and older adults, but it is beyond the scope of this study. Another potential limitation of this study is that only two MR scanners (1.5 and 3.0 T MR scanners) from one vendor were included for the reproducibility evaluation. Actually, the PDFF image has been demonstrated as an effective imaging biomarker in quantifying the fat content and distribution, and the linearity, reproducibility, and repeatability of PDFF were well assessed using multicenter, multiplatform, multivendor, and phantom/human experiments (14,44). Taking these findings into consideration, we emphatically evaluated the reproducibility of MR scanners with different field strengths in analyzing adipose tissue distribution in this study. A third limitation is that only 24 volunteers were recruited to evaluate the reproducibility of adipose tissue distribution using DL-based segmentation method across two MR scanners. Although this sample size satisfies the minimum requirements for statistical analysis using a G* power program, it could still impact the reliability and representativeness of the results. Therefore, our next step is to recruit more volunteers to enhance the robustness of our findings. Besides, we focused on the SAT and large IAT in the current study. We will develop new DL networks for more elaborate segmentation and quantification on different kinds of adipose tissues and fat deposition in muscle, liver, and pancreas in our next work. Further studies with larger samples, including children and young and older adults, will be conducted using multicenter datasets from different vendors to evaluate the intra-scanner repeatability and reproducibility, and inter-scanner reproducibility of whole-body composition analysis, including muscle, bone marrow, and adipose tissue.
Conclusions
This work validates that DL-based whole-body adipose tissue distribution analysis using PDFF images shows high reproducibility between 1.5 and 3.0 T, and therefore could be a reliable tool for whole-body adipose tissue distribution quantification.
Acknowledgments
Funding: This research was supported by
Footnote
Reporting Checklist: The authors have completed the GRRAS reporting checklist. Available at https://qims.amegroups.com/article/view/10.21037/qims-24-1306/rc
Conflicts of Interest: All authors have completed the ICMJE uniform disclosure form (available at https://qims.amegroups.com/article/view/10.21037/qims-24-1306/coif). J.G. serves as an unpaid editorial board member of Quantitative Imaging in Medicine and Surgery. The other authors have no conflicts of interest to declare.
Ethical Statement: The authors are accountable for all aspects of the work in ensuring that questions related to the accuracy or integrity of any part of the work are appropriately investigated and resolved. The study was conducted in accordance with the Declaration of Helsinki (as revised in 2013). This study was approved by the Institutional Review Board of Shenzhen Institutes of Advanced Technology, Chinese Academy of Sciences (approval No. IRB-210115-H0551). Written informed consent was provided by each participant before the study enrollment.
Open Access Statement: This is an Open Access article distributed in accordance with the Creative Commons Attribution-NonCommercial-NoDerivs 4.0 International License (CC BY-NC-ND 4.0), which permits the non-commercial replication and distribution of the article with the strict proviso that no changes or edits are made and the original work is properly cited (including links to both the formal publication through the relevant DOI and the license). See: https://creativecommons.org/licenses/by-nc-nd/4.0/.
References
- Sakers A, De Siqueira MK, Seale P, Villanueva CJ. Adipose-tissue plasticity in health and disease. Cell 2022;185:419-46. [Crossref] [PubMed]
- Karlsson T, Rask-Andersen M, Pan G, Höglund J, Wadelius C, Ek WE, Johansson Å. Contribution of genetics to visceral adiposity and its relation to cardiovascular and metabolic disease. Nat Med 2019;25:1390-5. [Crossref] [PubMed]
- Karpe F, Pinnick KE. Biology of upper-body and lower-body adipose tissue--link to whole-body phenotypes. Nat Rev Endocrinol 2015;11:90-100. [Crossref] [PubMed]
- Vogel MAA, Jocken JWE, Sell H, Hoebers N, Essers Y, Rouschop KMA, Cajlakovic M, Blaak EE, Goossens GH. Differences in Upper and Lower Body Adipose Tissue Oxygen Tension Contribute to the Adipose Tissue Phenotype in Humans. J Clin Endocrinol Metab 2018;103:3688-97. [Crossref] [PubMed]
- Stefan N. Causes, consequences, and treatment of metabolically unhealthy fat distribution. Lancet Diabetes Endocrinol 2020;8:616-27. [Crossref] [PubMed]
- Kuriyan R. Body composition techniques. Indian J Med Res 2018;148:648-58. [Crossref] [PubMed]
- Holmes CJ, Racette SB. The Utility of Body Composition Assessment in Nutrition and Clinical Practice: An Overview of Current Methodology. Nutrients 2021;13:2493. [Crossref] [PubMed]
- Lemos T, Gallagher D. Current body composition measurement techniques. Curr Opin Endocrinol Diabetes Obes 2017;24:310-4. [Crossref] [PubMed]
- Messina C, Albano D, Gitto S, Tofanelli L, Bazzocchi A, Ulivieri FM, Guglielmi G, Sconfienza LM. Body composition with dual energy X-ray absorptiometry: from basics to new tools. Quant Imaging Med Surg 2020;10:1687-98. [Crossref] [PubMed]
- Tolonen A, Pakarinen T, Sassi A, Kyttä J, Cancino W, Rinta-Kiikka I, Pertuz S, Arponen O. Methodology, clinical applications, and future directions of body composition analysis using computed tomography (CT) images: A review. Eur J Radiol 2021;145:109943. [Crossref] [PubMed]
- Hemke R, Buckless C, Torriani M. Quantitative Imaging of Body Composition. Semin Musculoskelet Radiol 2020;24:375-85. [Crossref] [PubMed]
- Barone M, Losurdo G, Iannone A, Leandro G, Di Leo A, Trerotoli P. Assessment of body composition: Intrinsic methodological limitations and statistical pitfalls. Nutrition 2022;102:111736. [Crossref] [PubMed]
- Dixon WT. Simple proton spectroscopic imaging. Radiology 1984;153:189-94. [Crossref] [PubMed]
- Hu HH, Yokoo T, Bashir MR, Sirlin CB, Hernando D, Malyarenko D, et al. Linearity and Bias of Proton Density Fat Fraction as a Quantitative Imaging Biomarker: A Multicenter, Multiplatform, Multivendor Phantom Study. Radiology 2021;298:640-51. [Crossref] [PubMed]
- Hernando D, Sharma SD, Aliyari Ghasabeh M, Alvis BD, Arora SS, Hamilton G, Pan L, Shaffer JM, Sofue K, Szeverenyi NM. Multisite, multivendor validation of the accuracy and reproducibility of proton‐density fat‐fraction quantification at 1.5 T and 3T using a fat–water phantom. Magn Reson Med 2017;77:1516-24. [Crossref] [PubMed]
- Bainbridge A, Bray TJP, Sengupta R, Hall-Craggs MA. Practical Approaches to Bone Marrow Fat Fraction Quantification Across Magnetic Resonance Imaging Platforms. J Magn Reson Imaging 2020;52:298-306. [Crossref] [PubMed]
- Hui SCN, Zhang T, Shi L, Wang D, Ip CB, Chu WCW. Automated segmentation of abdominal subcutaneous adipose tissue and visceral adipose tissue in obese adolescent in MRI. Magn Reson Imaging 2018;45:97-104. [Crossref] [PubMed]
- Leinhard OD, Johansson A, Rydell J, Smedby O, Nystrom F, Lundberg P, Borga M Quantitative abdominal fat estimation using MRI. In: 2008 19th International Conference on Pattern Recognition. IEEE; 2008.
- Karlsson A, Rosander J, Romu T, Tallberg J, Grönqvist A, Borga M, Dahlqvist Leinhard O. Automatic and quantitative assessment of regional muscle volume by multi-atlas segmentation using whole-body water-fat MRI. J Magn Reson Imaging 2015;41:1558-69. [Crossref] [PubMed]
- Dalili D, Bazzocchi A, Dalili DE, Guglielmi G, Isaac A. The role of body composition assessment in obesity and eating disorders. Eur J Radiol 2020;131:109227. [Crossref] [PubMed]
- Borga M, Thomas EL, Romu T, Rosander J, Fitzpatrick J, Dahlqvist Leinhard O, Bell JD. Validation of a fast method for quantification of intra-abdominal and subcutaneous adipose tissue for large-scale human studies. NMR Biomed 2015;28:1747-53. [Crossref] [PubMed]
- Borga M, Ahlgren A, Romu T, Widholm P, Dahlqvist Leinhard O, West J. Reproducibility and repeatability of MRI-based body composition analysis. Magn Reson Med 2020;84:3146-56. [Crossref] [PubMed]
- Greco F, Mallio CA. Artificial intelligence and abdominal adipose tissue analysis: a literature review. Quant Imaging Med Surg 2021;11:4461-74. [Crossref] [PubMed]
- Estrada S, Lu R, Conjeti S, Orozco-Ruiz X, Panos-Willuhn J, Breteler MMB, Reuter M. FatSegNet: A fully automated deep learning pipeline for adipose tissue segmentation on abdominal dixon MRI. Magn Reson Med 2020;83:1471-83. [Crossref] [PubMed]
- Huber FA, Chaitanya K, Gross N, Chinnareddy SR, Gross F, Konukoglu E, Guggenberger R. Whole-body Composition Profiling Using a Deep Learning Algorithm: Influence of Different Acquisition Parameters on Algorithm Performance and Robustness. Invest Radiol 2022;57:33-43. [Crossref] [PubMed]
- Küstner T, Hepp T, Fischer M, Schwartz M, Fritsche A, Häring HU, Nikolaou K, Bamberg F, Yang B, Schick F, Gatidis S, Machann J. Fully Automated and Standardized Segmentation of Adipose Tissue Compartments via Deep Learning in 3D Whole-Body MRI of Epidemiologic Cohort Studies. Radiol Artif Intell 2020;2:e200010. [Crossref] [PubMed]
- Haueise T, Schick F, Stefan N, Schlett CL, Weiss JB, Nattenmüller J, et al. Analysis of volume and topography of adipose tissue in the trunk: Results of MRI of 11,141 participants in the German National Cohort. Sci Adv 2023;9:eadd0433. [Crossref] [PubMed]
- Kafali SG, Shih SF, Li X, Kim GHJ, Kelly T, Chowdhury S, Loong S, Moretz J, Barnes SR, Li Z, Wu HH. Automated abdominal adipose tissue segmentation and volume quantification on longitudinal MRI using 3D convolutional neural networks with multi-contrast inputs. MAGMA 2024;37:491-506. [Crossref] [PubMed]
- Kway YM, Thirumurugan K, Tint MT, Michael N, Shek LP, Yap FKP, Tan KH, Godfrey KM, Chong YS, Fortier MV, Marx UC, Eriksson JG, Lee YS, Velan SS, Feng M, Sadananthan SA. Automated Segmentation of Visceral, Deep Subcutaneous, and Superficial Subcutaneous Adipose Tissue Volumes in MRI of Neonates and Young Children. Radiol Artif Intell 2021;3:e200304. [Crossref] [PubMed]
- Langner T, Hedström A, Mörwald K, Weghuber D, Forslund A, Bergsten P, Ahlström H, Kullberg J. Fully convolutional networks for automated segmentation of abdominal adipose tissue depots in multicenter water-fat MRI. Magn Reson Med 2019;81:2736-45. [Crossref] [PubMed]
- Shen N, Li X, Zheng S, Zhang L, Fu Y, Liu X, Li M, Li J, Guo S, Zhang H. Automated and accurate quantification of subcutaneous and visceral adipose tissue from magnetic resonance imaging based on machine learning. Magn Reson Imaging 2019;64:28-36. [Crossref] [PubMed]
- Somasundaram A, Wu M, Reik A, Rupp S, Han J, Naebauer S, Junker D, Patzelt L, Wiechert M, Zhao Y, Rueckert D, Hauner H, Holzapfel C, Karampinos DC. Evaluating Sex-specific Differences in Abdominal Fat Volume and Proton Density Fat Fraction at MRI Using Automated nnU-Net-based Segmentation. Radiol Artif Intell 2024;6:e230471. [Crossref] [PubMed]
- Wang Z, Cheng C, Peng H, Qi Y, Wan Q, Zhou H, Qu S, Liang D, Liu X, Zheng H, Zou C. Automatic segmentation of whole-body adipose tissue from magnetic resonance fat fraction images based on machine learning. MAGMA 2022;35:193-203. [Crossref] [PubMed]
- Faul F, Erdfelder E, Buchner A, Lang AG. Statistical power analyses using G*Power 3.1: tests for correlation and regression analyses. Behav Res Methods 2009;41:1149-60. [Crossref] [PubMed]
- Cheng C, Zou C, Liang C, Liu X, Zheng H. Fat-water separation using a region-growing algorithm with self-feeding phasor estimation. Magn Reson Med 2017;77:2390-401. [Crossref] [PubMed]
- Ibrahim MM. Subcutaneous and visceral adipose tissue: structural and functional differences. Obes Rev 2010;11:11-8. [Crossref] [PubMed]
- Pinnick KE, Nicholson G, Manolopoulos KN, McQuaid SE, Valet P, Frayn KN, Denton N, Min JL, Zondervan KT, Fleckner J, McCarthy MI, Holmes CC, Karpe F. Distinct developmental profile of lower-body adipose tissue defines resistance against obesity-associated metabolic complications. Diabetes 2014;63:3785-97. [Crossref] [PubMed]
- Divoux A, Erdos E, Whytock K, Osborne TF, Smith SR. Transcriptional and DNA Methylation Signatures of Subcutaneous Adipose Tissue and Adipose-Derived Stem Cells in PCOS Women. Cells 2022;11:848. [Crossref] [PubMed]
- Yu J, Xue Y, Song HK. Comparison of lung T2* during free-breathing at 1.5 T and 3.0 T with ultrashort echo time imaging. Magn Reson Med 2011;66:248-54. [Crossref] [PubMed]
- Idilman IS, Yildiz AE, Karaosmanoglu AD, Ozmen MN, Akata D, Karcaaltincaba M. Proton density fat fraction: magnetic resonance imaging applications beyond the liver. Diagn Interv Radiol 2022;28:83-91. [Crossref] [PubMed]
- Schmeel FC, Vomweg T, Träber F, Gerhards A, Enkirch SJ, Faron A, Sprinkart AM, Schmeel LC, Luetkens JA, Thomas D, Kukuk GM. Proton density fat fraction MRI of vertebral bone marrow: Accuracy, repeatability, and reproducibility among readers, field strengths, and imaging platforms. J Magn Reson Imaging 2019;50:1762-72. [Crossref] [PubMed]
- Caussy C, Reeder SB, Sirlin CB, Loomba R. Noninvasive, Quantitative Assessment of Liver Fat by MRI-PDFF as an Endpoint in NASH Trials. Hepatology 2018;68:763-72. [Crossref] [PubMed]
- Nayak KS, Cui SX, Tasdelen B, Yagiz E, Weston S, Zhong X, Ahlgren A. Body composition profiling at 0.55T: Feasibility and precision. Magn Reson Med 2023;90:1114-20. [Crossref] [PubMed]
- Yokoo T, Serai SD, Pirasteh A, Bashir MR, Hamilton G, Hernando D, Hu HH, Hetterich H, Kühn JP, Kukuk GM, Loomba R, Middleton MS, Obuchowski NA, Song JS, Tang A, Wu X, Reeder SB, Sirlin CBRSNA-QIBA PDFF Biomarker Committee. Linearity, Bias, and Precision of Hepatic Proton Density Fat Fraction Measurements by Using MR Imaging: A Meta-Analysis. Radiology 2018;286:486-98. [Crossref] [PubMed]