Enhancement of the nontumor component in newly diagnosed glioblastoma as a more accurate predictor of local recurrence location: a multicenter study
Introduction
The high recurrence rate and poor response to the treatment of glioblastoma (GBM) have been attributed to its heterogeneity, which has thus garnered considerable research attention (1). Even with total tumor resection and combined radiochemotherapy, recurrence of GMB still occurs. The accurate prediction of the spatial location of recurrent cancer may be a productive area of cancer research.
The spatial and temporal heterogeneity of GBM can be clearly mapped by the different habitats generated by multisequence magnetic resonance imaging (MRI) (2-8), as this can be used to perform survival risk stratification without incorporating the relationship between spatiotemporal heterogeneity of GBM and the local recurrence site. A recent study on the local recurrence of high-grade glioma indicated that the spatial pattern of local recurrence is related to patient prognosis and that the progression-free survival and overall survival of patients with recurrence within the surgical cavity are longer than those associated with recurrence outside the surgical cavity (9). However, the study only stratified local recurrence and did not accurately predict the spatial recurrence site. Another study on brain metastasis after stereotactic radiosurgery achieved successful prediction of the recurrence site (10); this was possible due to the observation that brain metastasis after stereotactic radiosurgery is less associated with brain tissue and lesion deformation as compared to GBM surgery (11). In addition, radiomics (12,13), machine learning (14,15), and deep learning (16,17) can also identify the spatiotemporal heterogeneity of GBM and better predict survival. However, none of the studies employing these modalities attempted to predict the spatial location of local recurrence (SLLR) in GBM.
The different degrees of enhancement in GBM include both the solid component and necrotic component of the tumor; however, the means to distinguishing between these different components and their value in predicting the site of GBM recurrence has not been extensively examined. Radiation-induced temporal lobe necrosis (TLN) of nasopharyngeal carcinoma (NPC) shares similar MRI and pathological findings with GBM except for the tumor cell (18,19). Therefore, in this current study, we used the semisupervised clustering of seeded k-means clustering algorithm to label radiation-induced TLN of NPC as nontumor components and performed mixed clustering with multisequence MRI imaging data of preoperative GBM. We sought to determine whether the clustering results and category features obtained with this method could distinguish between the nontumor component from the tumor cell based on solid enhancement of GBM, and we further aimed to analyze the relationship between the spatial sites of the two-component types and the SLLR. We present this article in accordance with the STROBE reporting checklist (available at https://qims.amegroups.com/article/view/10.21037/qims-24-1319/rc).
Methods
The retrospective study was conducted in accordance with the Declaration of Helsinki (as revised in 2013) and was approved by institutional ethics boards of Tianjin First Central Hospital (No. 2021N118KY), Tianjin Huanhu Hospital (No. 2023-103), and Hunan Cancer Hospital (No. KY2024533). The requirement for individual consent was waived due to the retrospective nature of the analysis.
Participants
A total of 728 patients in four independent cohorts were included for the retrospective analysis (Figure S1): data set 1 was used as a reference for the image information of the nontumor components to introduce category labels (20), while data set 2 was used as the preoperative imaging feature information for GBM. We combined T1-weighted images (T1WIs) enhancement, T2-weighted images (T2WIs), and apparent diffusion coefficient (ADC) sequences and used the seeded k-means clustering algorithm to establish a semisupervised clustering model to obtain clustering results and category features. Data sets 3 and 4 were used for out-of-set validation of the above model and to further predict the SLLR based on the clustering results. The overall study design is shown in Figure 1.
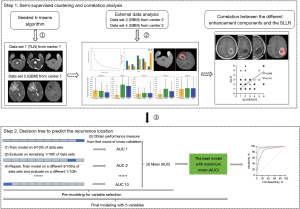
For data set 1, 109 patients with radiation-induced TLN from NPC were enrolled from March 2018 to August 2020 and were collected as a reference for the nontumor portion enhancement of GBM. The inclusion criteria were as follows: (I) histopathology confirmed of NPC; (II) patients with NPC who received radiotherapy for the first time and completed all radiotherapy as planned; (III) radiographic evidence supporting the diagnosis of radiation-induced TLN; and (IV) age >18 years. Meanwhile, the exclusion criteria were as follows: (I) evidence of tumor recurrence or metastases; (II) cerebral vascular events or inflammatory and neurodegenerative diseases; (III) loss to follow-up, (IV) poor imaging quality and incomplete images; and (V) registration error during data preprocessing.
For data sets 2, 3, and 4, a total of 619 patients with GBM were enrolled from November 2016 to December 2023. The inclusion criteria were as follows: (I) pathological diagnostic criteria meeting the 2021 World Health Organization fifth edition classification of glioma (21); (II) measurable volume of the enhanced region ≥1 cm3 and no history of preoperative treatment; (III) enhanced region on the postcontrast T1WI from total resection (in patients with partial resection, the residual lesion is the primary cause of disease progression, which can affect the prediction of local recurrence site); (IV) regular follow-up; and (V) pathology or radiographic evidence supporting the diagnosis of local recurrence. Meanwhile, the exclusion criteria were as follows: (I) partial resection and biopsy; (II) pseudoprogression or radiation necrosis; (III) distant recurrence and cerebrospinal fluid dissemination (as the aim of the study was to predict the SLLR of GBM); (IV) cases in which the recurrence filled the surgical cavity (as this precluded determination of the initial recurrence location); (V) assessment criteria with which recurrence could not be assessed; (VI) poor imaging quality and incomplete images; (VII) loss to follow-up; and (VIII) registration error during data preprocessing.
Imaging protocol
The GBM and radiation-induced TLN images were acquired on 3-T magnetic resonance (MR) scanners, including the Ingenia CX (Philips Healthcare, Best, the Netherlands) and the MAGENTOM Trio and Skyra (Siemens Healthineers, Erlangen, Germany). The sequences included T2WI, diffusion-weighted imaging (DWI), and postcontrast T1WI. The detailed parameters are shown in Table S1. Functional sequences were used to assess the recurrence after GBM treatment and included perfusion-weighted imaging (PWI) and magnetic resonance spectroscopy (MRS). PWI included arterial spin labeling (ASL), dynamic susceptibility contrast (DSC), and dynamic contrast enhancement (DCE). The parameters of functional sequences are provided in Appendix 1.
Imaging analysis
Criteria for radiation-induced TLN (data set 1)
All patients with NPC received radiotherapy. An enhanced MRI was performed when head symptoms occurred. The criteria (22) for patients with radiation-induced TLN were as follows: (I) history of radiotherapy for NPC; (II) availability of plain and contrast MRI data, including (i) white matter (WM) lesions (finger-like lesions of increased signal intensity in T2WI) located in the temporal lobe; (ii) contrast-enhanced lesions (lesions with or without necrosis in contrast-enhanced T1WI with heterogeneous signal abnormalities in T2 images); (iii) cysts (round or oval-shaped well-defined lesions of very high signal intensity in T2 images with thin or imperceptible walls); and (iv) follow-up data showing no progression of the TLN; and (III) no brain metastasis, intracranial tumors, brain abscesses, or other intracranial diseases.
Assessment of preoperative GBM (data sets 2, 3, and 4)
The imaging characteristics of all patients with newly diagnosed GBM were evaluated, including tumor location, involved corpus callosum, involved subventricular zone (SVZ), and cortical contact. Tumor location included bilateral frontal, parietal, occipital, temporal, and cerebellar sites. Involvement of the corpus callosum was defined as edema rather than enhanced tumor involvement in the corpus callosum. Involvement of the SVZ was defined as enhanced tumor contact with the lateral ventricular edge. Cortical contact was defined as enhanced tumor involvement in the cerebral cortex.
Assessment for the recurrence of GBM (data sets 3 and 4)
All enrolled patients underwent maximal safe resection (enhanced total tumor resection) via the intraoperative navigation system. Postoperative radiotherapy combined with temozolomide-based chemotherapy and sequential temozolomide-based chemotherapy were performed. If O6-methylguanine-DNA methyltransferase (MGMT) methylation was negative on molecular pathology, platinum-based sensitizers were added. All patients underwent MRI enhancement within 3 days after surgery, 3 days before radiotherapy, and 2–4 weeks after radiotherapy, and follow-up MRI enhancement was performed every 2–4 months. All patients were followed up until local recurrence or death.
Local recurrence included recurrence at the margins in the resection cavity or surgical area and recurrence within 2 cm from the operative cavity (9). All cases of recurrence were confirmed by the following: (I) pathology (gold standard); (II) Response Assessment in Modified Neuro-Oncology (RANO) criteria (23), continuous follow-up with enhanced MRI; and (III) appearance of a new enhancing lesion appeared and no subsequent follow-up MRI, with assessment of functional MRI (PWI and MRS). The specific manifestation is enhanced lesions showed hyperperfusion, increased choline (Cho) level, decreased N-acetyl aspartate (NAA) level, and a Cho:NAA ratio >2.5 were considered indicative of tumor progression and as confirmation of recurrence.
The SLLR was assessed as follows: (I) according to the enhanced MRI within 3 days after surgery, the surgical area was evaluated. (II) During follow-up enhanced MRI, the changes in the surgical area and the formation of the resection cavity were observed. (III) When a newly enhanced lesion appeared and recurrence confirmed, the MRI of the recurrence site and the previous follow-up MRI were compared to evaluate the positional relationship between the local recurrence and surgical cavity, including six spatial locations of the anterior, posterior, superior, inferior, WM side, and gray matter (GM) side. No recurrence on the GM side was observed, and the remaining five spatial locations of local recurrence are shown in Figure S2.
Clustering analysis
Clustering analysis was performed using T2WI, ADC, and T1WI-enhanced images. Data preprocessing, including format conversion, skull-stripping, registration, delineation region of interest (ROI), resampling, and normalization, is detailed in Appendix 1.
A semisupervised clustering analysis model was built to obtain the cluster results and category features via the seeded k-means clustering algorithm in scikit-learn (version 1.0.2) in Python 3.9 (Python Software Foundation, Wilmington, DE, USA) (Figure 2). First, pixels of the three sequences from data sets 1 and 2 were extracted according to the ROI on T1WI-enhanced images, with the number of pixels denoted as n. The three-channel data from two data sets were merged to obtain an n×3 pixel matrix, with the first column being T1WI enhancement, the second column being T2WI, and the third column being ADC, which were then used as input data for the seeded k-means clustering algorithm. Second, the number of clusters was determined according to the elbow curve. Next, the seeded k-means supervised clustering algorithm required independent prior labels, and so any radiation-induced TLN was labeled. Finally, after the modeling, the T1WI enhancement, T2WI, and ADC gray-value ranges were counted, and the saved model was displayed and visualized on the T1WI enhancement sequence for subsequent analysis. On the visualized images, the spatial location of tumor component enhancement (SLTE) and the spatial location of nontumor component enhancement (SLNTE) of GBM were evaluated and recorded according to the labeled method of the SLLR, including six spatial locations: the anterior, posterior, superior, inferior, WM side, and GM side.
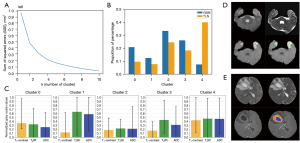
Assessment of the relationship among SLLR, SLTE, and SLNTE
Since the SLLR, SLTE, and SLNTE may include multiple spatial locations, their relationship was divided into the following three aspects (Figure 3): (I) only SLNTE correlating with SLLR; (II) only SLTE correlating with SLLR; and (III) both SLTE and SLNTE correlating with SLLR.
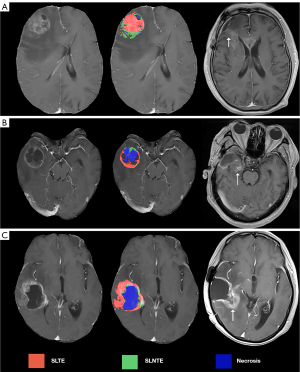
We denoted the SLLR corresponding to the SLNTE as SLLR_1 and that corresponding to the SLTE as SLLR_2. When the SLLR spanned two or more locations, such as the anterior and WM side, if the SLNTE was in the anterior and GM side and if the SLTE was in the WM side and posterior, these would, be labeled as SLLR_1 (anterior) and SLLR_2 (WM side), respectively.
Statistical analysis
The SPSS 26.0 (IBM Corp., Armonk, NY, USA) and GraphPad Prism 8.0 (GraphPad Software, San Diego, CA, USA) were used to analyze all data. The significance level was set at P<0.05 for all analyses. The SLLR, SLTE, and SLNTE were assessed by two radiologists, one with 7 years of experience and with 11 years, with the former performing the second assessment after 3 months. The intra- and intraobserver agreement for the radiologists was evaluated using the intraclass correlation coefficient (ICC) and the 95% confidence interval (CI). The ICC model used two-way random effects, single measurement, and absolute agreement.
The Chi-squared test was used to determine whether there were differences in the relationship between the SLLR and SLTE and between the SLLR and SLNTE. Spearman rank correlation was used to analyze the correlation between the SLLR and SLNTE or SLTE.
To further determine the ability of the SLNTE and SLTE to predict the SLLR, 10-fold cross-validation of the decision tree prediction model was performed using the classification regression tree (CRT) algorithm. The predictive performance of the decision tree model was evaluated by calculating the area under the curve (AUC), sensitivity, and specificity.
Results
Patient characteristics
The clinical and imaging data of 728 patients from three tertiary centers were retrospectively analyzed from November 2016 to December 2023. A total of 281 patients who met the inclusion and exclusion criteria were included in the final analysis, including four data sets. Additionally, 90 patients with the radiation-induced TLN (data set 1) and 110 pretreatment patients with GBM (data set 2) from center 1 (Hunan Cancer Hospital) were used to build a clustering analysis model for distinguishing between tumor and nontumor components in the enhanced region of GBM. Patients with GBM and local recurrence, including 67 participants for data set 3 from center 2 (Tianjin Huanhu Hospital) and 14 for data set from center 3 (Tianjin First Central Hospital), were used to analyze the relationship among the SLTE, SLNTE, and SLLR and to predict the SLLR. The clinical and imaging characteristics of the patients with GBM and radiation-induced TLN are listed in Tables S2,S3, respectively.
The results of the clustering analysis model
Data from patients with radiation-induced TLN (data set 1) and GBM (data set 2) were used to build the clustering analysis model, and we selected five clusters based on the sum of squared errors of the elbow method (Figure 2A). The clusters 0, 1, 2, 3, and 4 corresponded to red, blue, yellow, orange, and green colors. When modeling, we used radiation-induced TLN as a prior label and labeled it as cluster 4 (green), with the MRI manifestations being marked enhancement and higher T2WI and ADC values. The green zone represented the SLNTE. From Figure 2B-2E, we can observe that cluster 0, in red, is the tumor enhancement area, and the characteristic MRI features included noticeable enhancement and low T2WI and ADC values. The red zone represented SLTE. In Figure 2, cluster 1 is the necrotic area, in blue and was characterized by a lack of enhancement on MRI and high T2WI, and ADC values; meanwhile, clusters 2 (yellow) and 3 (orange) are undefined areas, with the MRI features being mild enhancement and slightly lower or higher T2WI and ADC values. According to our observation, the distribution locations were relatively fixed, such as the enhancement-edema junction area or the enhancement-necrosis junction area.
Inter- and intraobserver agreement for the spatial location assessment among radiologists
For data sets 3 and 4, the SLLR of recurrent GBM was evaluated, both the ICC values for the interobserver agreement (ICC_inter) and intraobserver agreement (ICC_intra) for SLLR were excellent (ICC_inter >0.80 and ICC_intra >0.90). Based on the clustering analysis model, we assessed the SLNTE and SLTE of GBM and their consistency, and both the inter- and intraobserver agreement for the radiologists was also excellent (ICC_inter >0.80 and ICC_intra >0.90). These results are summarized in Table S4.
The relationship between the SLLR and SLNTE or SLTE
For data sets 3 and 4, the labeled results of the SLLR, SLNTE, and SLTE are shown in Table S5. The chi-square test showed that for data set 3, the SLNTE had a stronger spatial relationship with SLLR than did SLTE (χ2=4.77; P=0.029). However, SLNTE did not have a stronger spatial relationship with SLLR than did SLTE for data set 4 (Fisher test, P=0.07) (Table 1). Although statistical significance was not reached in data set 4, a larger sample size might have resulted in significant differences.
Table 1
Relationship type | Only SLNTE | Only SLTE | Both | χ2 | P value |
---|---|---|---|---|---|
Data set 3 | 19 | 13 | 35 | 4.77 | 0.029†,* |
Data set 4 | 7 | 4 | 3 | NA | 0.07‡ |
Data are presented as number, unless otherwise specified. Since the SLLR, SLTE, and SLNTE may include multiple spatial locations, their relationship was divided into the following three aspects: (I) only SLNTE correlated with the SLLR; (II) only SLTE correlated with the SLLR; and (III) both SLTE and SLNTE correlated with the SLLR. †, corrected P value; ‡, Fisher exact test; *, statistical significance. SLLR, spatial location of local recurrence; SLNTE, spatial location of nontumor component enhancement; SLTE, spatial location of tumor component enhancement.
As shown in Figure 4, Spearman rank correlation indicated significant correlations between the SLLR and SLNTE for data set 3 (rSLNTE=0.70; 95% CI: 0.54–0.80; P<0.001) and data set 4 (rSLNTE=0.59; 95% CI: 0.07–0.86; P=0.03). Although there was a correlation between the SLLR and SLTE for data set 3 (rSLTE=0.34; 95% CI: 0.10–0.54; P=0.005), there was no correlation for data set 4 (rSLTE=0.20; 95% CI: −0.39 to 0.67; P=0.50). Therefore, the SLNTE had a stronger correlation with SLLR than did SLTE.
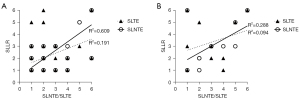
Construction of the decision tree prediction model
To further confirm the relationship between the SLLR and the SLNTE and SLTE, we constructed two decision tree models to predict the SLLR. The final decision tree parameters had a maximum depth of five layers; the minimum number of cases of the parent and child nodes was set to 6 and 3, respectively; and the test level of split and combined nodes was α=0.05. The SLLR was used as the dependent variable, and the independent variables included age, sex, time to recurrence, involved SVZ, cortical contact, and the SLNTE and SLTE. The importance of the independent variables in data set 3 is shown in Figure 5A, with SLNTE and SLTE being the most important variables. The dendrograms based on the SLNTE and SLTE are presented in Figure 5B,5C, respectively. The accuracy of the decision tree models based on the SLNTE and SLTE for predicting the SLLR was 81% and 72%, respectively (Table 2 and Table S6).
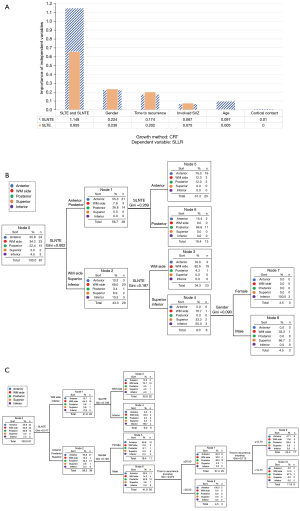
Table 2
Truth spatial location | Prediction | Correct percentage (%) | ||||
---|---|---|---|---|---|---|
Anterior | WM side | Posterior | Superior | Inferior | ||
Anterior | 19 | 3 | 2 | 0 | 0 | 79 |
WM side | 3 | 19 | 0 | 1 | 0 | 83 |
Posterior | 3 | 1 | 11 | 0 | 0 | 73 |
Superior | 0 | 0 | 0 | 2 | 0 | 100 |
Inferior | 0 | 0 | 0 | 0 | 3 | 100 |
Data are presented as number, unless otherwise specified. The accuracy of the decision tree model based on the SLNTE in predicting the SLLR was 81% for data set 3. SLLR_1, the SLLR corresponding to the SLNTE. SLNTE, spatial location of nontumor component enhancement; SLLR, spatial location of local recurrence; WM, white matter.
For data set 4, we used the same approach to build two decision tree models based on the SLNTE and SLTE to predict the SLLR (Figure S3A-S3C). The accuracy of the decision tree models in predicting the SLLR was 79% and 50%, respectively (Table 3 and Table S7).
Table 3
Truth spatial location | Prediction | Correct percentage (%) | ||||
---|---|---|---|---|---|---|
Anterior | WM side | Posterior | Superior | Inferior | ||
Anterior | 0 | 1 | 0 | 0 | 1 | 0 |
WM side | 0 | 5 | 0 | 0 | 0 | 100 |
Posterior | 0 | 0 | 4 | 0 | 0 | 100 |
Superior | 0 | 0 | 1 | 0 | 0 | 0 |
Inferior | 0 | 0 | 0 | 0 | 2 | 100 |
Data are presented as number, unless otherwise specified. The accuracy of the decision tree model based on the SLNTE in predicting the SLLR was 79% for data set 4. SLLR_1, the SLLR corresponding to the SLNTE. SLNTE, spatial location of nontumor component enhancement; SLLR, spatial location of local recurrence; WM, white matter.
The predictive efficacy of the decision tree models
In both data set 3 and data set 4, the decision tree models based on the SLNTE had a better prediction for the SLLR than did the SLTE (Tables S8,S9), and the AUC values based on the SLNTE were over 0.80 and 0.75, respectively.
Discussion
This study provides two key contributions in the research on GBM recurrence. First, we used radiation-induced TLN of NPC as a reference to distinguish the nontumor component from the tumor cell based on solid enhancement of GBM using semisupervised clustering analysis. Second, we found that the nontumor-enhanced portion of GBM could more accurately predict the SLLR.
In the examination of the relationship between the nontumor and tumor components of GBM and tumor invasion, it is necessary to separate these two components. Although numerous studies have demonstrated differences between pseudoprogression and radiation necrosis after GBM treatment and true progression based on various technical approaches (24-29), these lesions are complexes containing both inflammatory and tumor components. We selected radiation-induced TLN of NPC as a reference to build a clustering analysis model for the following reasons: (I) the lesion is located in the intracranial and completely unrelated to GBM; (II) the tissue composition is purer than pseudoprogression and radiation necrosis after GBM treatment (18,19); (III) we had a relatively large number of cases; and (IV) no previous study had compared TLN after NPC radiotherapy with GBM. The clustering analysis model we used could distinguish the nontumor component from the tumor cell based on solid enhancement of GBM.
Clustering methods can classify images based on the information of pixel points, but currently, in the application of machine learning algorithms to medical image segmentation, mainly unsupervised learning methods are used, such as k-means, density-based spatial clustering of applications with noise (DBSCAN), balanced iterative reducing and clustering using hierarchies (BIRCH), and ordering points to identify the clustering structure (OPTICS), among others. Among them, k-means is applicable to data of spherical clusters, which is the predominant form of GBM. Additionally, other algorithms have higher computational complexity and require a significant amount of computing resources and storage space, while k-means involves relatively lower requirements. Due to the high randomness of unsupervised clustering (30), incorporating prior knowledge can improve the accuracy and objectivity of clustering. The seeded k-means clustering algorithm (20) introduces the seeds set for independent prior labels and then uses the expectation maximization algorithm for class division. The distance calculation uses the Euclidean distance calculation formula. Compared with the k-means clustering algorithm and traditional unsupervised k-means algorithms, seeded k-means clustering has a superior performance (31).
Numerous studies at the cellular level have confirmed that the presence of nontumor components such as neutrophils, M2 type macrophages, CD4+ and CD8+ lymphocytes, and extracellular matrix contributes to regulating tumor growth and invasion in GBM (32-36). Moreover, age, location of the tumor, degree of tumor enhancement, ADC value, and TERT mutation status have been associated with tumor-associated macrophage infiltration (CD68+ and CD163+) at the imaging level (37). Therefore, we examined the relationship between the SLLR and the nontumor component of GBM from the imaging level. We found that the nontumor portion enhancement of GBM was more correlated with the SLLR compared to tumor components and could more accurately predict it, suggesting that the marginal area adjacent to the nontumor portion enhancement of GBM may be a high-risk spatial site for local recurrence. However, these results require one-to-one evidence of imaging and pathology or spatial transcriptomics. In addition, prediction of the SLLR based on the nontumor component was not possible with only a small number of datapoints in this study. Indeed, the clustering results did not show obvious nontumor components in 11.9% and 21.4% of the data in data set 3 (eight patients) and data set 4 (three patients), and we classified them as the only tumor part correlated with SLLR. The data in which there was little nontumor component need be confirmed by pathology.
Decision tree is a fully matured form of supervised machine learning. In the classification problem, the decision tree represents the process of classifying instances based on features (38). In our decision tree model, SLTE and SLNTE were the most important imaging features for predicting SLLR, which may suggest that SLLR is associated with the location of tumor and nontumor components and not with features of tumor invasion, such as SVZ involvement and cortical contact. Therefore, the location of the tumor and nontumor components warrant increased attention, especially SLNTE.
Due to its higher proliferation and migration ability, the tumor periphery of GBM is a critical region that significantly influences the tumor’s growth, invasion, and response to therapy (39,40). Li et al. found that for patients with GBM, resecting ≥53.21% of the abnormal T2WI or fluid-attenuated inversion recovery (FLAIR) area significantly prolonged patient survival (41). Although the importance of peritumoral tissue has been recognized, there is currently no precise method for defining the boundary of peritumoral invasive tumor tissue, except for invasive pathological approaches. Recently, a method of rapidly identifying the molecular boundary of tumors by acquiring multiple samples from the tumor cavity during surgery may achieve supertotal resection (42). However, the main issue with this is the blindness of tumor cavity sampling. In our included cases, we found that after the complete resection of the T1WI-enhanced lesions, the recurrence sites around the operative cavity were localized and heterogeneous. Therefore, the expanded resection should be performed on one side (in a certain direction) rather than the entire tumor periphery, which requires noninvasive preoperative imaging guidance.
The clinical significance of this study is that it provides an imaging reference for the extended resection of GBM based on the heterogeneity of the SLLR. Numerous studies have shown that the degree of tumor resection is a key indicator of glioma prognosis (43,44), and a greater resection of tumor tissue can provide a survival benefit to patients. However, a blind extension of resection may increase the risk of neurological dysfunction (45,46).
Our study involved several limitations which should be discussed. One major caveat of our study is that the tumor and nontumor components were distinguished based on T2WI, ADC, and enhanced T1WI images via clustering analysis. Further analysis using pathology and spatial transcriptomics is needed to clarify the pathological or molecular basis of the relationship between the nontumor components and the SLLR. We did not perform pathological or spatial transcriptomic analysis due to a lack of availability of pathological tissue in this retrospective study, but this will be undertaken in a future prospective study. Another concern is that the sample sizes of data sets 3 and 4 were insufficiently large. The main reason is the total resection of the T1WI-enhanced lesion is often difficult to achieve due to the location of GBM and the surgeon’s experience. But, based on the aim of this study, this more stringent inclusion criteria is necessary. Another issue was that data were derived from multiple centers, and the scanners and protocols were different. However, certain measures were undertaken to mediate this effect, including the use of quality images, identical preprocessing and standardization, and a consensus on ROI delineation and image evaluation between radiologists. Finally, the assessments of the SLLR, SLNTE, and SLTE were subjective. In order to be more objective, we considered using parameters such as the coordinate system or angle for quantitative evaluation, but the analysis process was complicated and unsuitable for clinical practice.
Conclusions
We developed a method for identifying the SLNTE and SLTE of GBM by combining GBM and radiation induced TLN for NPC through a retrospective analysis of three tertiary medical centers. The SLNTE of GBM showed a significant correlation with the SLLR and could predict it more accurately than could SLTE. Therefore, for the newly diagnosed GBM, the marginal zone adjacent to the nontumor component enhancement may be at higher risk of local recurrence, which may provide a more precise image basis for treatment options such as supertotal resection. However, our findings require further validation in larger cohorts, ideally from multiple centers.
Acknowledgments
The authors thank all the participants in the study.
Funding: This work was supported by
Footnote
Reporting Checklist: The authors have completed the STROBE reporting checklist. Available at https://qims.amegroups.com/article/view/10.21037/qims-24-1319/rc
Conflicts of Interest: All authors have completed the ICMJE uniform disclosure form (available at https://qims.amegroups.com/article/view/10.21037/qims-24-1319/coif). The authors have no conflicts of interest to declare.
Ethical Statement: The authors are accountable for all aspects of the work in ensuring that questions related to the accuracy or integrity of any part of the work are appropriately investigated and resolved. This retrospective study was conducted in accordance with the Declaration of Helsinki (as revised in 2013) and was approved by institutional ethics boards of Tianjin First Central Hospital (No. 2021N118KY), Tianjin Huanhu Hospital (No. 2023-103), and Hunan Cancer Hospital (No. KY2024533). The requirement for individual consent was waived due to the retrospective nature of the analysis.
Open Access Statement: This is an Open Access article distributed in accordance with the Creative Commons Attribution-NonCommercial-NoDerivs 4.0 International License (CC BY-NC-ND 4.0), which permits the non-commercial replication and distribution of the article with the strict proviso that no changes or edits are made and the original work is properly cited (including links to both the formal publication through the relevant DOI and the license). See: https://creativecommons.org/licenses/by-nc-nd/4.0/.
References
- Horbinski C, Nabors LB, Portnow J, Baehring J, Bhatia A, Bloch O, et al. NCCN Guidelines® Insights: Central Nervous System Cancers, Version 2.2022. J Natl Compr Canc Netw 2023;21:12-20. [Crossref] [PubMed]
- Cui Y, Tha KK, Terasaka S, Yamaguchi S, Wang J, Kudo K, Xing L, Shirato H, Li R. Prognostic Imaging Biomarkers in Glioblastoma: Development and Independent Validation on the Basis of Multiregion and Quantitative Analysis of MR Images. Radiology 2016;278:546-53. [Crossref] [PubMed]
- Juan-Albarracín J, Fuster-Garcia E, Pérez-Girbés A, Aparici-Robles F, Alberich-Bayarri Á, Revert-Ventura A, Martí-Bonmatí L, García-Gómez JM. Glioblastoma: Vascular Habitats Detected at Preoperative Dynamic Susceptibility-weighted Contrast-enhanced Perfusion MR Imaging Predict Survival. Radiology 2018;287:944-54. [Crossref] [PubMed]
- John F, Bosnyák E, Robinette NL, Amit-Yousif AJ, Barger GR, Shah KD, Michelhaugh SK, Klinger NV, Mittal S, Juhász C. Multimodal imaging-defined subregions in newly diagnosed glioblastoma: impact on overall survival. Neuro Oncol 2019;21:264-73. [Crossref] [PubMed]
- Beig N, Bera K, Prasanna P, Antunes J, Correa R, Singh S, Saeed Bamashmos A, Ismail M, Braman N, Verma R, Hill VB, Statsevych V, Ahluwalia MS, Varadan V, Madabhushi A, Tiwari P. Radiogenomic-Based Survival Risk Stratification of Tumor Habitat on Gd-T1w MRI Is Associated with Biological Processes in Glioblastoma. Clin Cancer Res 2020;26:1866-76. [Crossref] [PubMed]
- Park JE, Kim HS, Kim N, Park SY, Kim YH, Kim JH. Spatiotemporal Heterogeneity in Multiparametric Physiologic MRI Is Associated with Patient Outcomes in IDH-Wildtype Glioblastoma. Clin Cancer Res 2021;27:237-45. [Crossref] [PubMed]
- Yang Y, Han Y, Zhao S, Xiao G, Guo L, Zhang X, Cui G. Spatial heterogeneity of edema region uncovers survival-relevant habitat of Glioblastoma. Eur J Radiol 2022;154:110423. [Crossref] [PubMed]
- Li G, Li L, Li Y, Qian Z, Wu F, He Y, Jiang H, Li R, Wang D, Zhai Y, Wang Z, Jiang T, Zhang J, Zhang W. An MRI radiomics approach to predict survival and tumour-infiltrating macrophages in gliomas. Brain 2022;145:1151-61. [Crossref] [PubMed]
- Wang H, Zeng L, Wu H, Tian J, Xie H, Zhang L, Ran Q, Zhong P, Chen L, Yi L, Wang S. Preoperative vascular heterogeneity based on dynamic susceptibility contrast MRI in predicting spatial pattern of locally recurrent high-grade gliomas. Eur Radiol 2024;34:1982-93. [Crossref] [PubMed]
- Lee DH, Park JE, Kim N, Park SY, Kim YH, Cho YH, Kim JH, Kim HS. Tumor Habitat Analysis Using Longitudinal Physiological MRI to Predict Tumor Recurrence After Stereotactic Radiosurgery for Brain Metastasis. Korean J Radiol 2023;24:235-46. [Crossref] [PubMed]
- Xiao Y, Eikenes L, Reinertsen I, Rivaz H. Nonlinear deformation of tractography in ultrasound-guided low-grade gliomas resection. Int J Comput Assist Radiol Surg 2018;13:457-67. [Crossref] [PubMed]
- Verma R, Hill VB, Statsevych V, Bera K, Correa R, Leo P, Ahluwalia M, Madabhushi A, Tiwari P. Stable and Discriminatory Radiomic Features from the Tumor and Its Habitat Associated with Progression-Free Survival in Glioblastoma: A Multi-Institutional Study. AJNR Am J Neuroradiol 2022;43:1115-23. [Crossref] [PubMed]
- Du P, Wu X, Liu X, Chen J, Chen L, Cao A, Geng D. The application of decision tree model based on clinicopathological risk factors and pre-operative MRI radiomics for predicting short-term recurrence of glioblastoma after total resection: a retrospective cohort study. Am J Cancer Res 2023;13:3449-62.
- Suter Y, Knecht U, Alão M, Valenzuela W, Hewer E, Schucht P, Wiest R, Reyes M. Radiomics for glioblastoma survival analysis in pre-operative MRI: exploring feature robustness, class boundaries, and machine learning techniques. Cancer Imaging 2020;20:55. [Crossref] [PubMed]
- Fan L, Sowmya A, Meijering E, Song Y. Cancer Survival Prediction From Whole Slide Images With Self-Supervised Learning and Slide Consistency. IEEE Trans Med Imaging 2023;42:1401-12. [Crossref] [PubMed]
- Wan Y, Rahmat R, Price SJ. Deep learning for glioblastoma segmentation using preoperative magnetic resonance imaging identifies volumetric features associated with survival. Acta Neurochir (Wien) 2020;162:3067-80. [Crossref] [PubMed]
- Yan J, Sun Q, Tan X, Liang C, Bai H, Duan W, et al. Image-based deep learning identifies glioblastoma risk groups with genomic and transcriptomic heterogeneity: a multi-center study. Eur Radiol 2023;33:904-14. [Crossref] [PubMed]
- Tang Y, Rong X, Hu W, Li G, Yang X, Yang J, Xu P, Luo J. Effect of edaravone on radiation-induced brain necrosis in patients with nasopharyngeal carcinoma after radiotherapy: a randomized controlled trial. J Neurooncol 2014;120:441-7. [Crossref] [PubMed]
- Ali FS, Arevalo O, Zorofchian S, Patrizz A, Riascos R, Tandon N, Blanco A, Ballester LY, Esquenazi Y. Cerebral Radiation Necrosis: Incidence, Pathogenesis, Diagnostic Challenges, and Future Opportunities. Curr Oncol Rep 2019;21:66. [Crossref] [PubMed]
- Basu S, Banerjee A, Mooney RJ. Semi-supervised clustering by seeding. In: Proceedings of the 19th International Conference on Machine Learning (ICML-2022). Sydney: Morgan Kaufmann Publishers. 2002:19-26.
- Louis DN, Perry A, Wesseling P, Brat DJ, Cree IA, Figarella-Branger D, Hawkins C, Ng HK, Pfister SM, Reifenberger G, Soffietti R, von Deimling A, Ellison DW. The 2021 WHO Classification of Tumors of the Central Nervous System: a summary. Neuro Oncol 2021;23:1231-51. [Crossref] [PubMed]
- Zhang B, Lian Z, Zhong L, Zhang X, Dong Y, Chen Q, Zhang L, Mo X, Huang W, Yang W, Zhang S. Machine-learning based MRI radiomics models for early detection of radiation-induced brain injury in nasopharyngeal carcinoma. BMC Cancer 2020;20:502. [Crossref] [PubMed]
- Ellingson BM, Wen PY, Cloughesy TF. Modified Criteria for Radiographic Response Assessment in Glioblastoma Clinical Trials. Neurotherapeutics 2017;14:307-20. [Crossref] [PubMed]
- Kim JY, Park JE, Jo Y, Shim WH, Nam SJ, Kim JH, Yoo RE, Choi SH, Kim HS. Incorporating diffusion- and perfusion-weighted MRI into a radiomics model improves diagnostic performance for pseudoprogression in glioblastoma patients. Neuro Oncol 2019;21:404-14. [Crossref] [PubMed]
- Muscas G, van Niftrik CHB, Sebök M, Della Puppa A, Seystahl K, Andratschke N, Brown M, Weller M, Regli L, Piccirelli M, Fierstra J. Distinct Cerebrovascular Reactivity Patterns for Brain Radiation Necrosis. Cancers (Basel) 2021.
- Li Y, Ma Y, Wu Z, Xie R, Zeng F, Cai H, Lui S, Song B, Chen L, Wu M. Advanced Imaging Techniques for Differentiating Pseudoprogression and Tumor Recurrence After Immunotherapy for Glioblastoma. Front Immunol 2021;12:790674. [Crossref] [PubMed]
- Chawla S, Bukhari S, Afridi OM, Wang S, Yadav SK, Akbari H, Verma G, Nath K, Haris M, Bagley S, Davatzikos C, Loevner LA, Mohan S. Metabolic and physiologic magnetic resonance imaging in distinguishing true progression from pseudoprogression in patients with glioblastoma. NMR Biomed 2022;35:e4719. [Crossref] [PubMed]
- Taylor C, Ekert JO, Sefcikova V, Fersht N, Samandouras G. Discriminators of pseudoprogression and true progression in high-grade gliomas: A systematic review and meta-analysis. Sci Rep 2022;12:13258. [Crossref] [PubMed]
- Young JS, Al-Adli N, Scotford K, Cha S, Berger MS. Pseudoprogression versus true progression in glioblastoma: what neurosurgeons need to know. J Neurosurg 2023;139:748-59. [Crossref] [PubMed]
- Xie H, Zhang L, Lim CP, Yu Y, Liu C, Liu H, Walters J. Improving K-means clustering with enhanced Firefly Algorithms. Applied Soft Computing 2019;84:105763.
- Bair E. Semi-supervised clustering methods. Wiley Interdiscip Rev Comput Stat 2013;5:349-61. [Crossref] [PubMed]
- Massara M, Persico P, Bonavita O, Mollica Poeta V, Locati M, Simonelli M, Bonecchi R. Neutrophils in Gliomas. Front Immunol 2017;8:1349. [Crossref] [PubMed]
- Liang J, Piao Y, Holmes L, Fuller GN, Henry V, Tiao N, de Groot JF. Neutrophils promote the malignant glioma phenotype through S100A4. Clin Cancer Res 2014;20:187-98. [Crossref] [PubMed]
- Tamma R, Ingravallo G, Annese T, d'Amati A, Lorusso L, Ribatti D. Tumor Microenvironment and Microvascular Density in Human Glioblastoma. Cells 2022;12:11. [Crossref] [PubMed]
- Moffet JJD, Fatunla OE, Freytag L, Kriel J, Jones JJ, Roberts-Thomson SJ, Pavenko A, Scoville DK, Zhang L, Liang Y, Morokoff AP, Whittle JR, Freytag S, Best SA. Spatial architecture of high-grade glioma reveals tumor heterogeneity within distinct domains. Neurooncol Adv 2023;5:vdad142. [Crossref] [PubMed]
- Mathur R, Wang Q, Schupp PG, Nikolic A, Hilz S, Hong C, et al. Glioblastoma evolution and heterogeneity from a 3D whole-tumor perspective. Cell 2024;187:446-463.e16. [Crossref] [PubMed]
- Zhou Q, Xue C, Man J, Zhang P, Ke X, Zhao J, Zhang B, Zhou J. Correlation of tumor-associated macrophage infiltration in glioblastoma with magnetic resonance imaging characteristics: a retrospective cross-sectional study. Quant Imaging Med Surg 2023;13:5958-73. [Crossref] [PubMed]
- Chen T, Shang C, Su P, Keravnou-Papailiou E, Zhao Y, Antoniou G, Shen Q. A Decision Tree-Initialised Neuro-fuzzy Approach for Clinical Decision Support. Artif Intell Med 2021;111:101986. [Crossref] [PubMed]
- Venkataramani V, Yang Y, Schubert MC, Reyhan E, Tetzlaff SK, Wißmann N, et al. Glioblastoma hijacks neuronal mechanisms for brain invasion. Cell 2022;185:2899-2917.e31. [Crossref] [PubMed]
- Streibel Y, Breckwoldt MO, Hunger J, Pan C, Fischer M, Turco V, et al. Tumor biomechanics as a novel imaging biomarker to assess response to immunotherapy in a murine glioma model. Sci Rep 2024;14:15613. [Crossref] [PubMed]
- Li YM, Suki D, Hess K, Sawaya R. The influence of maximum safe resection of glioblastoma on survival in 1229 patients: Can we do better than gross-total resection? J Neurosurg 2016;124:977-88. [Crossref] [PubMed]
- Li J, Han Z, Ma C, Chi H, Jia D, Zhang K, Feng Z, Han B, Qi M, Li G, Li X, Xue H. Intraoperative rapid molecular diagnosis aids glioma subtyping and guides precise surgical resection. Ann Clin Transl Neurol 2024;11:2176-87. [Crossref] [PubMed]
- Zinn PO, Colen RR, Kasper EM, Burkhardt JK. Extent of resection and radiotherapy in GBM: A 1973 to 2007 surveillance, epidemiology and end results analysis of 21,783 patients. Int J Oncol 2013;42:929-34. [Crossref] [PubMed]
- Karschnia P, Vogelbaum MA, van den Bent M, Cahill DP, Bello L, Narita Y, Berger MS, Weller M, Tonn JC. Evidence-based recommendations on categories for extent of resection in diffuse glioma. Eur J Cancer 2021;149:23-33. [Crossref] [PubMed]
- Sanai N, Berger MS. Intraoperative stimulation techniques for functional pathway preservation and glioma resection. Neurosurg Focus 2010;28:E1. [Crossref] [PubMed]
- Wolbers JG. Novel strategies in glioblastoma surgery aim at safe, supra-maximum resection in conjunction with local therapies. Chin J Cancer 2014;33:8-15. [Crossref] [PubMed]