Evolutionary patterns and research frontiers of artificial intelligence in age-related macular degeneration: a bibliometric analysis
Introduction
Age-related macular degeneration (AMD) is a progressive degenerative eye disease that primarily affects the macula, leading to severe vision impairment in its advanced stages (1). In 2020, an estimated 196 million people worldwide were affected by AMD (2), with 1.8 million cases resulting in blindness, ranking it as the fourth leading cause of blindness among individuals aged 50 years and above (3). By 2040, the number of AMD patients is projected to reach 288 million globally (2), which will result in more people experiencing a poorer quality of life and impose an escalating burden for the public health sector.
The development of artificial intelligence (AI) in ophthalmology has been rapidly evolving, especially with the advancements of deep learning (DL). DL, a subset of machine learning (ML), has revolutionized AI applications in ophthalmology, with convolutional neural networks (CNNs) demonstrating exceptional capabilities in image processing (4,5). In the early 2010s, researchers began exploring the potential of DL techniques in analyzing ophthalmic images. The publication of pioneering research on DL for diabetic retinopathy (DR) screening in 2016 (6-9) later flourished this area. Studies subsequently demonstrated the efficacy of DL models in detecting a wide range of retinal diseases from medical images (10-12). In this context, AMD has emerged as a particular area of focus. There has been a significant increase in research activity in AI for AMD to address a range of clinical challenges and improve patient outcomes (13,14).
Bibliometrics is a quantitative method used to analyze various aspects of scholarly publications and scientific literature. It involves the application of statistical and mathematical techniques to study patterns of publication, citation, and collaboration within the academic community, aiming to measure the impact, influence, and productivity of research outputs (15). It provides an objective means to identify research trends, emerging topics, and hotspots within a field, especially for areas with large volumes of academic literature (16). This method enables a rapid and efficient evaluation, saving time and resources compared to manual evaluation methods.
Despite the increasing interest and research efforts surrounding AI in AMD, a comprehensive review elucidating the evolutionary patterns and research frontiers in this field remains absent. To address this conspicuous gap, the present study aimed to conduct a thorough bibliometric analysis of AI research in AMD, covering the period from its inception to 2023.
Methods
Data source and literature search strategy
Due to its comprehensive coverage of over 12,000 academic journals and frequent use by researchers, Web of Science was chosen as the primary database for this study. It provides the most comprehensive and reliable bibliometric analysis when compared with other databases such as Scopus, Medline, and PubMed (17). Relevant articles in the Web of Science Core Collection (WoSCC) were searched and exported on 15 April 2024, and the only selected edition was Science Citation Index Expanded (SCI-EXPANDED). After consultation with senior literature search experts, all authors agreed on the search strategy. The keywords focused on AI and AMD, with the detailed searching strategy summarized in Table S1. Table S1 also presents the searching strategies for sub-class analysis.
The timespan of the publication date was set from 1 January 1992 to 31 December 2023. We included only articles, review articles, and meeting abstracts written in English to facilitate further analysis. After removing duplicated publications, two researchers independently screened the titles and abstracts of the retrievals to identify eligible studies. Articles which are not available or relevant were excluded. Any discrepancies were resolved by discussion or consulting a third researcher. Further research was conducted with the complete record and cited references extracted from relevant publications.
Software for bibliometric analysis
In this study, software such as R version 4.3.2 (18), VOSviewer (19), and CiteSpace (20) were used as tools to perform the bibliometric analysis. Bibliometrix R package version 4.1.4 (21) was used to calculate the frequency of collaboration between countries/regions. Based on VOSviewer, we calculated the number of publications, citations, and keyword frequency. Co-occurrence networks of keywords from the scientific literature were constructed and visualized using the software’s embedded clustering algorithm. This tool primarily focused on co-authorship and co-occurrence analysis. If keywords exhibited similar meanings, they were merged using VOSviewer. It was also used to analyze country/region, institution, and author collaborations. In this study, CiteSpace was used to identify keywords and references that gained significant citations during a specified period (22). Our visualization of international collaborations was made possible by the online bibliometrics website (https://bibliometric.com/). Microsoft Excel (23) was used to analyze the exponential growth in the number of publications in this area.
Results
Overview of publication status
This study included 1,721 conventional articles on AI in AMD, as shown in Figure 1. A summary of the annual and cumulative publication counts related to AI in AMD is shown in Figure 2. From 1 publication in 1992 to 484 publications in 2015, the cumulative number has steadily increased. Advancements of DL subsequently led to a rapid increase in publications after 2015, reaching 1,721 in 2023. The relationship between cumulative publications and publication year was evaluated using an exponential growth function, which matched the trend in cumulative publications (R2=0.9912). This strong correlation suggests that research on AI in AMD has experienced significant growth and development.
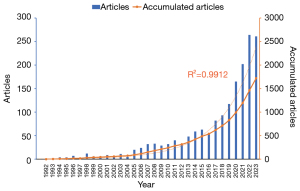
Assessment of the publication counts on a national/regional scale
The countries/regions contributing the most in this field were analyzed using national/regional publication counts. As shown in Figure 3, the USA ranked first with 586 publications, followed by China (n=321), England (n=169), Australia (n=144), Germany (n=125), India (n=121), Japan (n=100), South Korea (n=97), Austria (n=87), and Singapore (n=81). The remaining countries/regions had publications fewer than 80.
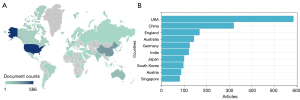
We also visualized the collaborations among countries/regions in Figure S1 as part of our investigation. According to the results, USA leads the way in research of AI in AMD. The most frequent collaborations were between the USA and China (with a frequency of 68) and between the USA and the United Kingdom (UK, with a frequency of 59).
Simultaneously, as shown in Figure S2, we conducted a co-authorship analysis of all publications from the 34 countries/regions with the most publications to investigate the collaboration between countries/regions. The size of the nodes in both the clustering and time-overlapping networks serve as proxies for the number of scholarly publications. The link between the nodes represents the collaboration between countries/regions, and the thickness of the link signals the number of times that the collaboration occurs. The thicker the link between nodes is, the more the collaboration occurs. In the clustering network, each color represents a collaborative cluster. In the time-overlapping network, the color of the nodes delineates the mean publication year for each country/region within the designated research domain. As displayed in Figure S2A, the 34 countries/regions formed 5 clusters. The red cluster, which includes the most countries/regions, has 12 countries/regions. In Figure S2B, the early pioneers in the frontier of AI in AMD were certain European countries such as Australia, France, the Netherlands, and the UK, whereas studies from USA and China in this field are relatively recent and are gradually assuming a dominant role in terms of publication counts.
Assessment of the publication counts on an institutional scale
While investigating the contributions of institutions to the study of AI in AMD, an analysis was conducted on the publication counts from various institutions. The research on AI in AMD encompassed approximately 2,341 institutions globally. As shown in Figure 4, of the top 20 organizations, 7 research institutions are from USA, 3 from the UK, 2 from China, 2 from Singapore, 2 from Australia, 2 from the Netherlands, 1 from Germany, and 1 from Austria. With 64 articles published, University of Melbourne ranked first.
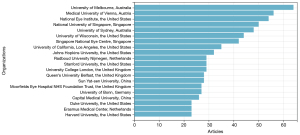
To further investigate collaboration between institutions, we performed a co-authorship analysis of all publications. Figure S3A shows that 51 institutions published at least 15 papers. These 51 institutions formed 8 clusters, with the red cluster being the largest, consisting of 12 institutions, mainly from USA. As is shown in Figure S3B, institutions such as Duke University, University of Sydney, University of Wisconsin, and so on, contributed significantly to the early development of AI in AMD. In contrast, multiple research institutions such as Stanford University, Sun Yat-sen University, and so on, became more involved in research of AI in AMD after 2018.
Analysis of publication quantity and journal impact
The investigation encompassed 1,721 articles from various journals. Table 1 delineates the top 10 journals, ordered by publication quantity, along with their latest 2023 impact factors (IF) (11). Among the top 10 journals, 6 were in the first quartile (Q1) of the Journal Citation Reports (JCR), and 8 out of 10 journal publishers were from USA.
Table 1
Rank | Source | Article | Country | IF | H-index | JCR |
---|---|---|---|---|---|---|
1 | Investigative Ophthalmology & Visual Science | 204 | USA | 4.4 | 218 | Q1 |
2 | Ophthalmology | 87 | USA | 13.7 | 244 | Q1 |
3 | British Journal of Ophthalmology | 53 | USA | 4.1 | 153 | Q1 |
4 | Scientific reports | 52 | UK | 4.6 | 213 | Q2 |
5 | Eye | 46 | UK | 3.9 | 98 | Q1 |
6 | Retina, the Journal of Retinal and Vitreous Diseases | 46 | USA | 3.3 | 120 | Q2 |
7 | Translational Vision Science Technology | 39 | USA | 3 | 21 | Q2 |
8 | American Journal of Ophthalmology | 36 | USA | 4.2 | 186 | Q1 |
9 | PloS One | 35 | USA | 3.7 | 332 | Q2 |
10 | Archives of Ophthalmology | 32 | USA | 8.1 | 196 | Q1 |
IF, impact factor; H-index, Hirsch index; JCR, journal citation reports.
Author impact analysis
A collective of 7,857 authors contributed to research on AI in AMD. According to Table 2, Schmidt-Erfurth emerged as the most prolific author, having published 66 articles, and possessing a notable H-index of 80. Close behind was Bogunovic (62 articles, H-index =29). Notably, Wong had the highest H-index (H-index =167).
Table 2
Rank | Author | Institution | Country | Article | H-index |
---|---|---|---|---|---|
1 | Schmidt-Erfurth, Ursula | Medical University of Vienna | Austria | 66 | 80 |
2 | Bogunovic, Hrvoje | Medizinische Universität Wien | Austria | 62 | 29 |
3 | Wong, Tien Yin | Tsinghua Medicine & Singapore National Eye Center | Singapore | 32 | 167 |
4 | Guymer, Robyn H. | Royal Victorian Eye & Ear Hospital | Austria | 32 | 62 |
5 | Chew, Emily Y. | University of Wisconsin Madison | USA | 31 | 89 |
6 | Reiter, Gregor S. | Medical University of Vienna | Austria | 29 | 11 |
7 | Keane, Pearse A. | NIHR Moorfields Biomedical Research Centre | UK | 26 | 54 |
8 | Keenan, Tiarnan D. L. | NIH National Eye Institute | USA | 24 | 28 |
9 | Gerendas, Bianca S. | Medical University of Vienna | Austria | 22 | 26 |
10 | Seddon, Johanna M. | University of Massachusetts System | USA | 22 | 82 |
H-index, Hirsch index.
The collaborative relationships among researchers are depicted in Figure S4A. The size of each circle corresponds to the number of publications, whereas each color indicates a specific cluster. A total of 54 authors, each with 10 or more articles, were organized into 9 distinct clusters. There were 5 clusters containing at least 5 authors. Only 2 clusters were located outside the more prominent community (i.e., there was no collaboration between these 2 scattered clusters and the larger clusters). The time-overlapping network of clustering outcomes is presented in Figure S4B. It is observed that researchers frequently collaborate with those from the same country/region or institution.
Research hotspot analysis
Most cited publications
The assessment of the most cited articles within a field is feasible by considering the frequency of citations (24). Table S2 provides a compilation of the top 10 most cited publications, all of which have over 300 citations. Out of 10 publications, 6 were related to DL, which all emerged after 2017. A total of 3 articles explored the effects of high-dose vitamins C and E, beta carotene, and zinc supplements on AMD progression and visual acuity, all of which had been published before 2001. The remaining article, published in 2006, discussed the use of computer vision techniques in retinal image processing. The change in the themes of most cited publications reveals how AI in AMD has evolved: initially, logistic regression was used to analyze correlations, and later, DL was used to aid diagnosis and prognostic analysis.
The article with the most citations was a clinical trial revealing that taking a supplement of antioxidants plus zinc can reduce the risk of developing advanced AMD and vision loss (25). Repeated-measures logistic regression was used to analyze the primary outcome. In 2018, Cell published the second most cited publication: ‘Identifying Medical Diagnoses and Treatable Diseases by Image-Based Deep Learning’. A DL framework with transfer learning was developed for screening treatable blinding retinal diseases using optical coherence tomography (OCT) images. The framework achieves performance comparable to human experts in classifying AMD and diabetic macular edema (DME), and provides interpretable diagnoses by highlighting recognized regions. The AI system is generalizable for diagnosing pediatric pneumonia using chest X-ray images.
Analysis of citation bursts
Figure S5 depicts the top 25 references with the strongest citation bursts. A burst in this context refers to a publication receiving a notably higher number of citations than usual, sustaining for a minimum of 2 years. The blue line corresponds to the observation period spanning from 1992 to 2023, whereas the red line denotes the specific burst duration.
The article with the highest citation burst value (citation burst =25.61) was published in 2005, with a bursting range between 2006 and 2010 (26). This genome-wide screen identified a common intronic variant in the complement factor-H gene (CFH) that is strongly associated with AMD. This polymorphism is located in a region of CFH that binds heparin and C-reactive protein. Later, various articles delved deeper into the genetic essence of AMD and how these mutations contribute to AMD. In 2009, a prediction model emerged, using unconditional logistic regression analyses to predict prevalence and incidence of advanced AMD based on genetic, demographic, and environmental variables (27). The years after 2017 comprise the era of DL. Some 4 out of 5 still-bursting articles focus on using DL systems to diagnose AMD or predict its progression (8,13,14,28).
Frequency and clustering analysis of keywords
Out of 5,228 keywords, 70 surpassed the threshold of 30 occurrences and underwent co-occurrence analysis, as shown in Figure 5A,5B. The size of the nodes reflects keyword frequency, and the distance between them signifies relationship strength. As displayed in Figure 5A, by grouping closely related keywords together, the 70 keywords were categorized into 4 clusters. Group 1, the biggest cluster and visually represented in red, focused on the application of ‘DL’ in AMD. Through the utilization of CNNs, which excel in automatic ‘feature extraction’, researchers can create robust algorithms for image analysis tasks such as ‘segmentation’, using mainly ‘OCT’ images. Group 2, represented in green, focused on ‘risk factors’ of AMD. Factors such as ‘smoking’ and ‘cardiovascular disease’ were widely discussed. As research went deeper into the genetic level, keywords such as ‘complement factor-H’ and ‘C-reactive protein’ also emerged. Group 3, represented in blue, focused on the treatment of AMD, mainly focusing on anti-vascular endothelial growth factor (VEGF) drugs such as ‘ranibizumab’ and ‘bevacizumab’. The yellow Group 4 focused on signs during the ‘progression’ of AMD, such as ‘drusen’ and ‘reticular pseudodrusen’. Figure 5B presents the time-overlapping cluster of keywords. Keywords that emerged earlier are depicted in blue, whereas those more recently introduced are displayed in yellow. Early research mainly focused on topics of ‘risk factors’, then about topics of ‘polymorphism’. The most recent studies have concentrated on ‘DL’. This demonstrates that the use of AI in AMD evolved initially from risk-factor analysis, later to genetic analysis, and recently, to DL model development in these 30 years. Figure 5C exhibits the top 20 keywords organized by frequency, with ‘age-related macular degeneration’ standing out as the most frequently utilized keyword, appearing 438 times, followed by ‘risk-factors’ (N=285) and ‘macular degeneration’ (N=257).
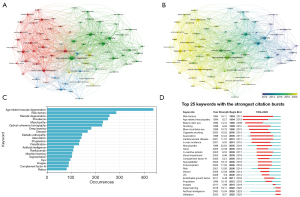
Analysis of keywords bursts
Figure 5D shows the leading 20 keywords experiencing robust citation bursts, lasting a minimum of 1 year. Notably, the term ‘risk factors’ (1994–2017) gained the most prolonged attention. However, keywords such as ‘deep learning’ (2020–2023), ‘artificial intelligence’ (2020–2023), and ‘validation’ (2020–2023) have emerged more recently and are still bursting, indicating the undertaking and upcoming research hotspots.
Sub-class analysis
In the context of the previous findings, which identified three distinct phases in AI research, we conducted a subgroup analysis to delve deeper into the latest trends associated with DL. The subgroup analysis excluded logistic regression to isolate the impact and trajectory of DL research. This approach allows for a clearer picture of how DL is being applied to AMD research and what specific areas are garnering the most attention.
The results of this analysis are presented in Figures 6. Figure 6A shows the publication volumes per year with three sets of keywords. Keywords 1 includes all initially chose keywords, Keywords 2 excludes logistic regression, and Keywords 3 specifically highlights the use of logistic regression within AI research. The graph illustrates a consistent yet gradual increase in the number of publications related to logistic regression. In contrast, publications focusing on DL have seen a rapid surge since 2015. Figure 6C compiles the 20 most frequently cited keywords after the exclusion of logistic regression, with ‘age-related macular degeneration’ standing out as the most frequently utilized keyword, appearing 217 times, followed by ‘deep learning’ (N=183) and ‘optical coherence tomography’ (N=172). These top 70 keywords then underwent co-occurrence analysis, as is shown in Figure 6D. In Figure 6D, by grouping closely related keywords together, the 70 keywords were categorized into 4 clusters. Group 1, the biggest cluster and visually represented in red, focused on the application of ‘machine learning’ in understanding the ‘progression’ and disease ‘prediction’ of AMD. Group 2, represented in green, focused on the ‘diagnosis’ of ophthalmic diseases using ‘image analysis’ and ‘automated detection’. Group 3, represented in blue, concentrates on the application of ‘DL’. The use of ‘OCT’ is highlighted as a crucial imaging tool. The yellow Group 4 focused on the study of ‘retinal structure’, with a particular focus on the identification and analysis of disease-related lesions, such as ‘drusen’, through techniques such as ‘feature extraction’ and ‘segmentation’.
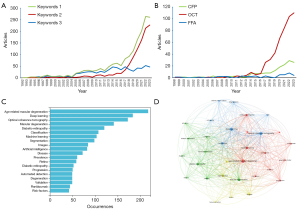
In the context of the keyword clustering that highlighted the significance of OCT in AI research, we conducted a subgroup analysis focusing on imaging modalities. Figure 6B displays the annual publication volumes for OCT, color fundus photography (CFP), and fluorescence fundus angiography (FFA). The data clearly shows a significant concentration of research on OCT, with a substantial portion also focusing on CFP, and a comparatively smaller interest in FFA.
Discussion
The use of AI in AMD is a rapidly evolving field with a large number of studies emerging. In this study, bibliometric methods were employed to investigate the evolution of AI research in AMD from 1992 to 2023. It provides valuable insights into the structure, dynamics, and trends of this field, pinpointing frontier hotspots to promote further research.
The first segment of the findings sought to quantitatively evaluate the productivity and collaborative dynamics within this field across various dimensions, thereby elucidating the development status and the current state of research in the discipline.
The exponential growth in the number of publications on AI in AMD is a clear indication of the rapid development of this field, especially from 2017. This growth is being driven by several factors. Firstly, advancements in DL techniques, particularly CNNs, have revolutionized the field of medical image analysis. These sophisticated algorithms excel at extracting complex patterns and features from medical images, enabling more accurate and reliable diagnosis and prognosis of AMD. The increasing availability of large-scale datasets and computational resources has further facilitated the training and deployment of DL models in AMD research. Secondly, the growing prevalence of AMD and the associated burden on healthcare systems worldwide have underscored the need for innovative and efficient diagnostic and therapeutic approaches. AI-based solutions offer the potential to enhance early detection, monitoring, and treatment planning for AMD, thereby improving patient outcomes and reducing healthcare costs. Moreover, collaborations between multidisciplinary teams, including ophthalmologists, computer scientists, and engineers, have fostered cross-fertilization of ideas and expertise, driving innovation in AI applications for AMD. However, the strong correlation between cumulative publications and publication year suggests that the field of AI research in AMD is still in its early stages of growth. Thus, this is a very exciting time for the field, as there is a great deal of potential for new discoveries and innovations.
By analyzing the number of national/regional publications and the frequency of international collaboration, we can gain insights into the global distribution of research activity and the extent to which researchers are working together across borders. The results of this analysis suggest that the USA is a global leader in AI research in AMD, while China is an emerging force in the field. The USA has the largest number of publications (n=586) and the strongest presence in international collaboration, whereas China has a smaller number of publications (n=321) but is also actively engaged in international collaboration. Moreover, Australia, as well as European countries such as France, the Netherlands, and the UK are the early pioneers in this field. One of the reasons may be the higher rates of AMD in European and North American populations than in those of Asian, Hispanic, or African ancestry (29). However, as populations around the world age, the burden of AMD is expected to increase (29). This will likely lead to increased research activity in countries/regions such as China, which has a large and rapidly aging population. Additionally, the USA and Europe’s leadership can be attributed to the availability of human expertise and access to large datasets. The dense concentration of experts in these regions plays a pivotal role in driving innovation and the development of sophisticated AI models. Furthermore, the wealth of AMD-related data in these regions, due to extensive research and a higher prevalence of AMD, is crucial for training AI systems to accurately diagnose and predict AMD progression. Overall, the field of AI research in AMD will benefit from increased international collaboration because it can help to overcome the challenges of data diversity and the need for large-scale studies.
The investigation into the contributions of institutions to the study of AI in AMD provides another standpoint to view the global research landscape and development trends within this field. Analysis of publication counts from various institutions revealed a diverse distribution of research activity across different countries/regions and organizations. Among the top 20 institutions, the USA still emerged as a dominant contributor, with seven research institutions represented, followed by significant contributions from the UK, China, Singapore, Australia, the Netherlands, Germany, and Austria. The University of Melbourne stood out as the leading institution in terms of publication output, underscoring its prominent role in advancing research on AI in AMD. A deeper analysis of collaboration analysis revealed the formation of eight distinct clusters, with the largest cluster predominantly comprising institutions from the USA. These findings underscore the importance of collaborative networks in driving research progress and innovation in the field of AI in AMD.
The analysis of publication counts and IF in peer-reviewed journals can help to identify target journals for manuscript submissions. The findings revealed that Investigative Ophthalmology & Visual Science leads with the highest publication count, totaling 204 articles, followed by Ophthalmology with 87 articles. Notably, Ophthalmology holds the highest IF at 13.7. Among the top 10 journals by paper count, 60% belong to Q1, whereas the remaining are Q2 journals. USA publishers take the dominant role in this area, accounting for 80% of the top 10 journals. Despite China’s second contributions to AI in AMD, Chinese publishers are notably underrepresented in the top 10 journals. This observation underscores the importance of establishing and cultivating internationally influential journals in China and other Asian countries/regions to enhance the visibility and impact of research outputs from these regions.
The visualization of research collaboration among researchers reveals a structured yet diverse research community. The presence of nine distinct clusters, with 5 being notably larger, underscores the existence of both focused research groups and broader collaborative efforts. The two isolated clusters, lacking integration with the main community, suggest opportunities for enhanced collaboration. These groups could benefit from initiatives that bridge the gap, potentially leading to new insights. The tendency for researchers to collaborate within national/regional or institutional boundaries indicates a reliance on local resources and shared research interests. Although this can foster deep expertise, it also highlights the need for strategies that promote cross-boundary collaboration.
In the second segment of this study, we analyzed the citation patterns and keyword usage of the included literature, respectively performing burst detection analysis. The results provide valuable insights into the evolution of AI research in AMD over time, helping to identify the research hotspots and corresponding influential achievements at different stages, as well as the emerging research frontiers and areas where further research is needed to advance the field.
The comprehensive analysis of keyword frequencies, time-resolved clustering, and burst detection revealed the developmental trajectory of AI in AMD research. Initially, research predominantly revolved around understanding the “risk factors” of AMD. This demonstrates the field’s early emphasis on identifying epidemiological and lifestyle factors, such as “smoking” and “cardiovascular disease”, that could contribute to the incidence and progression of AMD (29). This phase was fundamental in laying the groundwork for subsequent investigation. As the field matured, the research focus expanded to a more detailed exploration of the genetic underpinnings of AMD. Keywords such as “complement factor-H” and “C-reactive protein” began to emerge, signaling a deeper dive into the molecular and genetic aspects of AMD. This phase helps to elucidate the mechanisms of AMD. Later in the mid-2010s, a notable transition toward “DL” research was observed. By leveraging CNNs’ capabilities for automatic “feature extraction”, researchers can develop powerful algorithms for image analysis and computer vision tasks, such as “segmentation” on “OCT”. This has considerably enhanced the diagnostic processes for AMD, potentially leading to more accurate and timely interventions. Moreover, although our current analysis did not detect statistical significance in the keyword “AMD biomarker”, it represents a crucial parameter in AI-based AMD research. The integration of AI in the identification and analysis of AMD biomarkers could revolutionize early diagnosis, enabling more precise stratification of disease risk and personalized treatment planning. For example, Thakoor et al. (30) developed a DL model to detect various stages of AMD. Choroidal neovascularization and geographic atrophy were found to be important biomarkers indicative of AMD risk. This underscores the potential of AI not only in diagnosing AMD but also in identifying key biomarkers that could revolutionize our understanding of the disease’s progression and response to treatment. This will be instrumental in unraveling the complex pathophysiology of AMD and uncovering new therapeutic targets.
In conclusion, in recent years, there has been a consistent shift from focusing on risk factors and genetic polymorphisms to the cutting-edge domain of DL. This demonstrates that AI’s role in AMD has evolved from a supportive tool for risk-factor analysis and genetic insights to a critical component in developing advanced diagnostic and prognostic models.
The assessment of the most cited articles in the field of AI in AMD provides valuable insights into the key achievements and milestones that have shaped the development of this area over time. By analyzing the frequency of citations, researchers can identify seminal works and pivotal contributions that have significantly influenced the trajectory of AI in AMD research. Table S2 presents the top 10 most cited publications in the field, all of which have garnered over 300 citations. Notably, 6 out of 10 publications emerged after 2017 and were predominantly related to DL. This shift towards DL reflects the increasing recognition of its efficacy in aiding diagnosis and prognostic analysis in AMD research. Prior to the emergence of DL techniques, three articles published before 2001 focused on the effects of high-dose vitamins C and E, beta carotene, and zinc supplements on AMD progression and visual acuity. Although these studies may seem peripheral to the contemporary AI discourse, they represent an early form of AI application in the field. They utilized logistic regression to examine the relationship between vitamin supplementation and AMD prognosis. These studies contributed valuable insights into the potential therapeutic strategies for AMD management. The remaining article, published in 2006, explored the application of computer vision techniques in retinal image processing, laying the groundwork for subsequent advancements in AI-driven approaches for analyzing retinal images. The change in the themes of the most cited publications reflects the evolving landscape of AI in AMD. Initially, logistic regression was utilized for analyzing correlations. Later, as the field progressed, DL techniques gained prominence, particularly in aiding diagnosis and prognostic analysis.
The analysis of the top 25 references with the strongest citation bursts provides further insights into the key breakthroughs in the field of AI in AMD. The article with the highest citation burst value, published in 2005, identified a common intronic variant in the CFH gene strongly associated with AMD. This groundbreaking discovery shed light on the genetic underpinnings of AMD and paved the way for subsequent research endeavors into the role of genetic mutations in disease pathogenesis. Following this seminal work, various articles delved deeper into the genetic essence of AMD and elucidated how these mutations contribute to disease development and progression. In 2009, a prediction model emerged, utilizing unconditional logistic regression analyses to predict the prevalence and incidence of advanced AMD based on genetic, demographic, and environmental variables. This predictive model marked a significant advancement in AMD research, offering valuable insights into disease risk stratification and personalized medicine approaches. The years following 2017 witnessed a paradigm shift in AI research, with a predominant focus on DL methodologies. A total of 4 out of 5 still-bursting articles during this period revolved around the use of DL systems to diagnose AMD or predict its prognosis. This underscores the growing recognition of DL as a powerful tool for advancing AMD research and clinical practice, enabling more accurate and efficient disease diagnosis and management. In summary, from seminal discoveries in genetic associations to the emergence of predictive models and the adoption of DL methodologies, each phase represents significant progress in our understanding and management of AMD using AI-driven approaches.
In 2017, four seminal articles emerged and burst, paving the way for later research and still ranking within the 6 most cited publications in terms of AI in AMD. JAMA published an article utilizing CNNs in the automated detection of DR, possible glaucoma, and AMD in diverse populations with diabetes, offering promising implications for improving vision outcomes in diabetic patients (8). JAMA Ophthalmology presented a paper with significant achievements in the automated detection of AMD at an early stage from fundus images using CNNs (13). Lee et al. (28) trained a model utilizing CNNs to categorize OCT images as either normal or AMD. Fang et al. (31) introduced a novel framework, termed CNN-GS, which combines CNNs with graph search methods for automatic segmentation of 9-layer boundaries on retinal OCT images. The CNN-GS framework employs a CNN to extract features and train classifiers for delineating pilot estimates of the 8 layers, followed by a graph search method to refine the boundaries using probability maps generated by the CNN. This innovative approach addresses the challenge of accurate segmentation of retinal layer boundaries in OCT images, improving the efficiency and accuracy of segmentation tasks.
In 2018, Cell published the most-cited article: ‘Identifying Medical Diagnoses and Treatable Diseases by Image-Based Deep Learning’. In this article, a diagnostic tool based on a DL framework was developed, utilizing transfer learning to train a neural network with a fraction of the data required by conventional approaches. The AI system demonstrated performance comparable to that of human experts in classifying AMD and DME using OCT images. A crucial innovation of this research is that it provides a more transparent and interpretable diagnosis by highlighting the regions recognized by the neural network. Moreover, it processes general applicability, also shown to be effective for the diagnosis of pediatric pneumonia using chest X-ray images (9).
In 2020, Nature Medicine published the most-recent high-cited and bursting article: ‘Predicting conversion to wet age-related macular degeneration using deep learning’. In this research, a DL system is developed to predict progression to exudative (exAMD) in the second eye of patients diagnosed with exAMD in one eye. This system combines models based on 3-dimensional (3D) OCT images and corresponding automatic tissue maps, achieving high performance in predicting conversion to exAMD within a clinically actionable 6-month time window. Additionally, automatic tissue segmentation can identify anatomical changes before conversion and high-risk subgroups. The significance of this study is that this AI system enables earlier detection of exAMD and referral for treatment, thus having the potential to improve patient care and lead to better visual outcomes (14).
In conclusion, the trajectory of AI research in AMD can be delineated into distinct stages, each characterized by shifts in research focus, methodologies, and key achievements (Table S3). Initially, in the pre-2010s era, investigations centered on identifying modifiable and non-modifiable “risk factors” associated with AMD, employing logistic regression to develop predictive models. Subsequently, in the 2010s, there was a shift towards unraveling the genetic underpinnings of AMD, facilitated by genome-wide association studies (GWAS) that elucidated key genetic polymorphisms such as the CFH gene. This phase allowed for a deeper understanding of AMD’s molecular mechanisms. In the mid-2010s, a significant transition towards “DL” occurred, revolutionizing AMD research by enabling sophisticated image analysis from OCT and fundus photography. Notable achievements include the creation of AI systems for AMD diagnosis, severity assessment, and progression prediction, leveraging DL technologies such as CNNs and transfer learning.
Our sub-class analysis, designed to explore the recent trends in AI research related to DL for AMD, provided intriguing insights into the research landscape. By excluding logistic regression from our initial keyword set, we aimed to focus solely on the advancements and applications of DL within this domain. The results, as depicted in Figure 6A, reveal a bifurcated trend in publication volumes. On the one hand, there is a steady, albeit slow, increase in publications associated with logistic regression, indicating its enduring relevance in AI research for AMD. On the other hand, a remarkable surge in DL-related publications post-2015 underscores the paradigm shift towards more complex and advanced AI methodologies.
Comparing the results from the keyword analysis with and without logistic regression, we can observe both consistencies and shifts in focus within the AI and AMD research domain. Consistently, ‘age-related macular degeneration’ remains the most utilized keyword in both analyses, reaffirming its central role in research. Initially, the keyword ‘risk-factors’ was second in frequency, which dropped out of the top 3 in the second analysis, suggesting a shift in focus away from traditional risk factor analysis. Instead, ‘deep learning’ and ‘optical coherence tomography’ emerge as the second and third most frequent keywords, respectively, in the dataset excluding ‘logistic regression’. This change underscores a growing emphasis on advanced analytical methods and imaging techniques in AMD research. The co-occurrence analysis in the first dataset clusters keywords into four distinct groups: DL applications in AMD, risk factors of AMD, treatments focusing on anti-VEGF drugs, and signs of AMD progression. In contrast, the second dataset, which excludes ‘logistic regression’, forms clusters around ML for AMD progression and prediction, diagnosis through image analysis and automated detection, DL applications with an emphasis on OCT, and the study of retinal structure and disease-related lesions.
Furthermore, the subgroup analysis focusing on imaging modalities revealed a notable emphasis on OCT within AI research related to AMD. This preference for OCT can be attributed to its high-resolution, cross-sectional imaging capabilities that allow for detailed visualization of retinal layers and pathologies. Moreover, it is a non-invasive imaging modality that has become integral in clinical ophthalmology. Therefore, there is a growing availability of large, well-labeled OCT datasets that facilitate the development and validation of DL models for ophthalmic applications. In contrast, FFA, despite offering detailed vascular images that are crucial for diagnosing vascular eye diseases, receives comparatively less attention. This could be due to the invasive nature of the procedure and the need for expertise in interpreting the dynamic vascular images it produces.
Moving forward, several crucial research directions in AI systems are imperative to advance the field of AMD (Table S4). Early detection of AMD is a primary focus, with AI showing promise in enhancing early identification of conditions such as breast cancer (32). However, the development of predictive models for AMD development is hindered by the scarcity of pre-symptomatic patient data, thus lacking large-scale datasets to facilitate model training. Therefore, an alternative approach is to stratify early-stage AMD patients by risk, identifying those at a higher risk of progressing to the late, sight-threatening stage of the disease. This stratification is crucial for initiating timely clinical actions, including medical interventions and timely monitoring. Pioneering efforts in this area have laid the groundwork (14,33), and further exploration is needed. Additionally, considering the individual variability in response to anti-VEGF treatments and their significant economic burden, predicting treatment outcomes and evaluating therapeutic efficacy is of paramount importance. This remains a notable gap in the literature. Only a few studies have focused on this topic (34-37), and their clinical impact has yet to be fully established. Causal ML stands out as a valuable tool in this context, as it facilitates the estimation of personalized treatment effects, thereby enabling more customized clinical decision-making aligned with each patient’s unique profile (38). Furthermore, the development of interpretable modeling techniques is paramount to unveil the underlying mechanisms of AMD and identify potential therapeutic targets. Although this research approach has not yet been extensively implemented in AMD, it has demonstrated success in other diseases. For instance, explainable AI systems have been employed to capture biological patterns in clinical and molecular data, providing insights into the mechanisms governing remission and flare in rheumatoid arthritis (39). Such an approach could be similarly applied to AMD to enhance our understanding of the disease.
In terms of improvements in AI systems, they need to be validation and refinement on larger and more diverse datasets to enhance generalizability and robustness. Moreover, efforts to enhance the interpretability and reliability of AI models are crucial for their adoption in clinical practice. Lastly, long-term monitoring and evaluation of AI systems in real-world clinical settings are essential to ensure their efficacy, safety, and sustainability in improving patient outcomes. These concerted efforts will pave the way for more effective AMD diagnosis, prognosis, and treatment strategies, ultimately benefiting patients and healthcare providers alike.
Concerning the architecture of the AI systems, previous studies have predominantly employed CNNs for the image-based analysis of AMD. However, large language models (LLMs) are increasingly capturing the attention of researchers. LLMs, renowned for their prowess in natural language processing, are now being explored for a variety of applications in the medical field, including but not limited to, simplifying radiology reports, writing medical records, predicting patient outcomes, and assisting in clinical decision-making (40-42). Currently, in the field of ophthalmology, the applications of LLM mainly include streamlining medical record documentation (43,44), identifying key risk factors (45), developing management recommendations (45,46), answering patient queries (47), and facilitating triage and appointment prioritization (48). For example, Huang et al. (49) compared the diagnostic accuracy and comprehensiveness of responses from GPT-4 with those of glaucoma and retina specialists on ophthalmological questions and real patient case management. They found that the GPT-4 showed comparative proficiency in diagnostic accuracy and completeness, outperforming glaucoma specialists and matching retina specialists. Specifically for AMD, the existing literature is limited to addressing AMD patients’ questions. Recently, Ferro Desideri et al. (50) evaluated the performance of three prominent LLMs—ChatGPT 3.5, Bing AI, and Google Bard—in providing accurate and helpful responses to AMD-related questions. ChatGPT 3.5 consistently offered the most accurate and satisfactory responses, particularly in handling technical inquiries. However, caution is needed regarding the potential for generating incorrect information and the need to protect patient data.
Furthermore, in the domain of image processing, LLMs are making strides. LLMs are built on the Transformer architecture, which allows them to capture global and long-range dependencies among pixels or patches in images without using recurrence or convolutions. This enables more effective image analysis and contributes to a more comprehensive feature extraction (51,52). By integrating the Transformer into a hybrid model, researchers have successfully developed a system that can effectively predict the severity levels of DR from retinal fundus images, achieving state-of-the-art performance in the task (53). Concurrently, LLMs can integrate data from various modalities, such as combining image data with textual information, and increase clinical interactivity by engaging in dialogues with users (52). For instance, researchers have developed an AI system that uses LLMs to generate reports of FFA images and answer patients’ questions about the images (54). The study demonstrates the potential of LLMs to enhance ophthalmic image interpretation and facilitate interactive communication during medical consultations. In addition to direct image processing by LLMs, vision-language models (VLMs) present an alternative approach. VLMs combine the capabilities of LLMs with computer vision to analyze and understand images, and then generate or process text based on the visual information. VLMs have shown strong capabilities in various fields, but their application in ophthalmology has not been extensively studied. Antaki et al. (55) assessed the performance of the Gemini Pro VLM, a generalist model, on expert-level tasks for detecting macular diseases from OCT scans. It turned out that the model had limited capability in detecting pathological features. However, it demonstrated measurable abilities compared against expert for detecting macular hole, pigment epithelial detachment, subretinal hyperreflective material, and subretinal fluid. This study underscores the potential of VLMs in ophthalmology while also highlighting the need for further development and refinement. The limited performance observed in this study could be attributed to the scarcity of highly detailed ophthalmic image-text pairs in the training data of generalist VLMs. As VLMs continue to evolve, the creation of large, ophthalmology-specific benchmarking datasets will be crucial for validating their performance and realizing their potential in the field (44,55). In conclusion, the incorporation of LLMs or VLMs in AI systems for ophthalmologic diseases, including AMD, represents a cutting-edge research direction.
This study has certain limitations. Firstly, it includes only English-language articles from the WoSCC database. Although WoSCC generally contains high-quality studies, the exclusion of non-English articles may affect the comprehensiveness of the results. Secondly, delays in citation recognition may lead to recently published, high-quality studies not receiving appropriate attention, necessitating updates in subsequent research. Thirdly, although bibliometrics employs quantitative scientific methods to analyze research status, the interpretation of research trends and hotspots relies on the subjective understanding of the analysts. However, despite these limitations, this study remains a valuable resource for researchers, providing insights into the development, hotspots, trends, and frontiers of AI in AMD. Additionally, it helps to identify areas requiring further investigation.
Conclusions
In recent years, there has been increasing attention paid to AI, including in areas related to AMD. This study conducted a bibliometric analysis of the publications related to AI in AMD, using the WoSCC database as the data source. The notable rise in yearly publications underscores the escalating significance of this research domain. The study quantitatively assessed productivity and collaborative dynamics at national/regional, institutional, and individual levels, while also identifying the most active journals. In terms of research evolution, AI research in AMD has gone through three stages from 1992 to 2023. Presently, the focal point lies in crafting DL models capable of extracting critical information from OCT images and fundus photography for AMD diagnosis and progression prediction. Future endeavors should concentrate on early detection, disease risk stratification, and treatment efficacy prediction. Interpretable models to uncover the underlying mechanisms of AMD are also in need. The incorporation of LLMs or VLMs in AI systems represents a cutting-edge research direction. Consequently, this study furnishes both novice researchers and policymakers with a comprehensive understanding of the field’s evolution and forefronts.
Acknowledgments
The authors want to thank CiteSpace and VOSviewer for free access by researchers.
Funding: This study was partially sponsored by
Footnote
Conflicts of Interest: All authors have completed the ICMJE uniform disclosure form (available at https://qims.amegroups.com/article/view/10.21037/qims-24-1406/coif). The authors have no conflicts of interest to declare.
Ethical Statement: The authors are accountable for all aspects of the work in ensuring that questions related to the accuracy or integrity of any part of the work are appropriately investigated and resolved.
Open Access Statement: This is an Open Access article distributed in accordance with the Creative Commons Attribution-NonCommercial-NoDerivs 4.0 International License (CC BY-NC-ND 4.0), which permits the non-commercial replication and distribution of the article with the strict proviso that no changes or edits are made and the original work is properly cited (including links to both the formal publication through the relevant DOI and the license). See: https://creativecommons.org/licenses/by-nc-nd/4.0/.
References
- Buonfiglio F, Korb CA, Stoffelns B, Pfeiffer N, Gericke A. Recent Advances in Our Understanding of Age-Related Macular Degeneration: Mitochondrial Dysfunction, Redox Signaling, and the Complement System. Aging Dis 2024; Epub ahead of print. [Crossref]
- Wong WL, Su X, Li X, Cheung CM, Klein R, Cheng CY, Wong TY. Global prevalence of age-related macular degeneration and disease burden projection for 2020 and 2040: a systematic review and meta-analysis. Lancet Glob Health 2014;2:e106-16. [Crossref] [PubMed]
- GBD 2019 Blindness and Vision Impairment Collaborators; Vision Loss Expert Group of the Global Burden of Disease Study. Causes of blindness and vision impairment in 2020 and trends over 30 years, and prevalence of avoidable blindness in relation to VISION 2020: the Right to Sight: an analysis for the Global Burden of Disease Study. Lancet Glob Health 2021;9:e144-e160. Erratum in: Lancet Glob Health 2021;9:e408. [Crossref] [PubMed]
- LeCun Y, Bengio Y, Hinton G. Deep learning. Nature 2015;521:436-44. [Crossref] [PubMed]
- Ting DSW, Pasquale LR, Peng L, Campbell JP, Lee AY, Raman R, Tan GSW, Schmetterer L, Keane PA, Wong TY. Artificial intelligence and deep learning in ophthalmology. Br J Ophthalmol 2019;103:167-75. [Crossref] [PubMed]
- De Fauw J, Ledsam JR, Romera-Paredes B, Nikolov S, Tomasev N, Blackwell S, et al. Clinically applicable deep learning for diagnosis and referral in retinal disease. Nat Med 2018;24:1342-50. [Crossref] [PubMed]
- Gulshan V, Peng L, Coram M, Stumpe MC, Wu D, Narayanaswamy A, Venugopalan S, Widner K, Madams T, Cuadros J, Kim R, Raman R, Nelson PC, Mega JL, Webster DR. Development and Validation of a Deep Learning Algorithm for Detection of Diabetic Retinopathy in Retinal Fundus Photographs. JAMA 2016;316:2402-10. [Crossref] [PubMed]
- Ting DSW, Cheung CY, Lim G, Tan GSW, Quang ND, Gan A, et al. Development and Validation of a Deep Learning System for Diabetic Retinopathy and Related Eye Diseases Using Retinal Images From Multiethnic Populations With Diabetes. JAMA 2017;318:2211-23. [Crossref] [PubMed]
- Kermany DS, Goldbaum M, Cai W, Valentim CCS, Liang H, Baxter SL, et al. Identifying Medical Diagnoses and Treatable Diseases by Image-Based Deep Learning. Cell 2018;172:1122-1131.e9. [Crossref] [PubMed]
- Burlina P, Pacheco KD, Joshi N, Freund DE, Bressler NM. Comparing humans and deep learning performance for grading AMD: A study in using universal deep features and transfer learning for automated AMD analysis. Comput Biol Med 2017;82:80-6. [Crossref] [PubMed]
- Abràmoff MD, Lavin PT, Birch M, Shah N, Folk JC. Pivotal trial of an autonomous AI-based diagnostic system for detection of diabetic retinopathy in primary care offices. NPJ Digit Med 2018;1:39. [Crossref] [PubMed]
- Brown JM, Campbell JP, Beers A, Chang K, Ostmo S, Chan RVP, Dy J, Erdogmus D, Ioannidis S, Kalpathy-Cramer J, Chiang MF. Imaging and Informatics in Retinopathy of Prematurity (i-ROP) Research Consortium. Automated Diagnosis of Plus Disease in Retinopathy of Prematurity Using Deep Convolutional Neural Networks. JAMA Ophthalmol 2018;136:803-10. [Crossref] [PubMed]
- Burlina PM, Joshi N, Pekala M, Pacheco KD, Freund DE, Bressler NM. Automated Grading of Age-Related Macular Degeneration From Color Fundus Images Using Deep Convolutional Neural Networks. JAMA Ophthalmol 2017;135:1170-6. [Crossref] [PubMed]
- Yim J, Chopra R, Spitz T, Winkens J, Obika A, Kelly C, et al. Predicting conversion to wet age-related macular degeneration using deep learning. Nat Med 2020;26:892-9. [Crossref] [PubMed]
- van Raan AFJ. Measurement of Central Aspects of Scientific Research: Performance, Interdisciplinarity, Structure. Measurement: Interdisciplinary Research and Perspectives 2005;3:1-19.
- Hicks D, Wouters P, Waltman L, de Rijcke S, Rafols I. Bibliometrics: The Leiden Manifesto for research metrics. Nature 2015;520:429-31. [Crossref] [PubMed]
- Yeung AWK. Comparison between Scopus, Web of Science, PubMed and publishers for mislabelled review papers. Curr Sci 2019;116:1909-14.
- Ihaka R, Gentleman R R. A Language for Data Analysis and Graphics. J Comput Graph Stat 1996;5:299-314.
- van Eck NJ, Waltman L. Software survey: VOSviewer, a computer program for bibliometric mapping. Scientometrics 2010;84:523-38. [Crossref] [PubMed]
- Chen C. CiteSpace II: Detecting and visualizing emerging trends and transient patterns in scientific literature. J Am Soc Inf Sci Technol 2006;57:359-77.
- Aria M, Cuccurullo C. bibliometrix: An R-tool for comprehensive science mapping analysis. Journal of Informetrics 2017;11:959-75.
- Zhang J, Yang X, Fang J, Cheng QC, Ding HR, Sun YR, Li M, Zhang JL, Zhang L, Zhao H, Sang X, Xu YY, Zhang WG, Lu X. Evolution of associating liver partition and portal vein ligation for staged hepatectomy from 2012 to 2021: A bibliometric analysis. Review Int J Surg 2022;103:106648. [Crossref] [PubMed]
- Jiang S, Liu Y, Zheng H, Zhang L, Zhao H, Sang X, Xu Y, Lu X. Evolutionary patterns and research frontiers in neoadjuvant immunotherapy: a bibliometric analysis. Int J Surg 2023;109:2774-83. [Crossref] [PubMed]
- Xu AH, Sun YX. Research hotspots and effectiveness of repetitive transcranial magnetic stimulation in stroke rehabilitation. Neural Regen Res 2020;15:2089-97. [Crossref] [PubMed]
- A randomized, placebo-controlled, clinical trial of high-dose supplementation with vitamins C and E, beta carotene, and zinc for age-related macular degeneration and vision loss: AREDS report no. 8. Arch Ophthalmol 2001;119:1417-36. [Crossref] [PubMed]
- Klein RJ, Zeiss C, Chew EY, Tsai JY, Sackler RS, Haynes C, Henning AK, SanGiovanni JP, Mane SM, Mayne ST, Bracken MB, Ferris FL, Ott J, Barnstable C, Hoh J. Complement factor H polymorphism in age-related macular degeneration. Science 2005;308:385-9. [Crossref] [PubMed]
- Seddon JM, Reynolds R, Maller J, Fagerness JA, Daly MJ, Rosner B. Prediction model for prevalence and incidence of advanced age-related macular degeneration based on genetic, demographic, and environmental variables. Invest Ophthalmol Vis Sci 2009;50:2044-53. [Crossref] [PubMed]
- Lee CS, Baughman DM, Lee AY. Deep learning is effective for the classification of OCT images of normal versus Age-related Macular Degeneration. Ophthalmol Retina 2017;1:322-7. [Crossref] [PubMed]
- Apte RS. Age-Related Macular Degeneration. N Engl J Med 2021;385:539-47. [Crossref] [PubMed]
- Thakoor KA, Yao J, Bordbar D, Moussa O, Lin W, Sajda P, Chen RWS. A multimodal deep learning system to distinguish late stages of AMD and to compare expert vs. AI ocular biomarkers. Sci Rep 2022;12:2585. [Crossref] [PubMed]
- Fang L, Cunefare D, Wang C, Guymer RH, Li S, Farsiu S. Automatic segmentation of nine retinal layer boundaries in OCT images of non-exudative AMD patients using deep learning and graph search. Biomed Opt Express 2017;8:2732-44. [Crossref] [PubMed]
- Ng AY, Oberije CJG, Ambrózay É, Szabó E, Serfőző O, Karpati E, Fox G, Glocker B, Morris EA, Forrai G, Kecskemethy PD. Prospective implementation of AI-assisted screen reading to improve early detection of breast cancer. Nat Med 2023;29:3044-9. [Crossref] [PubMed]
- Peng Y, Keenan TD, Chen Q, Agrón E, Allot A, Wong WT, Chew EY, Lu Z. Predicting risk of late age-related macular degeneration using deep learning. NPJ Digit Med 2020;3:111. [Crossref] [PubMed]
- Liu Y, Yang J, Zhou Y, Wang W, Zhao J, Yu W, Zhang D, Ding D, Li X, Chen Y. Prediction of OCT images of short-term response to anti-VEGF treatment for neovascular age-related macular degeneration using generative adversarial network. Br J Ophthalmol 2020;104:1735-40. [Crossref] [PubMed]
- Lee H, Kim S, Kim MA, Chung H, Kim HC. Post-treatment prediction of optical coherence tomography using a conditional generative adversarial network in age-related macular degeneration. Retina 2021;41:572-80. [Crossref] [PubMed]
- Zhao X, Zhang X, Lv B, Meng L, Zhang C, Liu Y, Lv C, Xie G, Chen Y. Optical coherence tomography-based short-term effect prediction of anti-vascular endothelial growth factor treatment in neovascular age-related macular degeneration using sensitive structure guided network. Graefes Arch Clin Exp Ophthalmol 2021;259:3261-9. [Crossref] [PubMed]
- Moon S, Lee Y, Hwang J, Kim CG, Kim JW, Yoon WT, Kim JH. Prediction of anti-vascular endothelial growth factor agent-specific treatment outcomes in neovascular age-related macular degeneration using a generative adversarial network. Sci Rep 2023;13:5639. [Crossref] [PubMed]
- Feuerriegel S, Frauen D, Melnychuk V, Schweisthal J, Hess K, Curth A, Bauer S, Kilbertus N, Kohane IS, van der Schaar M. Causal machine learning for predicting treatment outcomes. Nat Med 2024;30:958-68. [Crossref] [PubMed]
- Alivernini S, Cañete JD, Bacardit J, Kurowska-Stolarska M. Using explainable artificial intelligence to predict and forestall flare in rheumatoid arthritis. Nat Med 2024;30:925-6. [Crossref] [PubMed]
- Thirunavukarasu AJ, Ting DSJ, Elangovan K, Gutierrez L, Tan TF, Ting DSW. Large language models in medicine. Nat Med 2023;29:1930-40. [Crossref] [PubMed]
- Shah NH, Entwistle D, Pfeffer MA. Creation and Adoption of Large Language Models in Medicine. JAMA 2023;330:866-9. [Crossref] [PubMed]
- Frosolini A, Catarzi L, Benedetti S, Latini L, Chisci G, Franz L, Gennaro P, Gabriele G. The Role of Large Language Models (LLMs) in Providing Triage for Maxillofacial Trauma Cases: A Preliminary Study. Diagnostics (Basel) 2024;14:839. [Crossref] [PubMed]
- Ong J, Kedia N, Harihar S, Vupparaboina SC, Singh SR, Venkatesh R, Vupparaboina K, Bollepalli SC, Chhablani J. Applying large language model artificial intelligence for retina International Classification of Diseases (ICD) coding. J Med Artif Intell 2023;6:21.
- Jin K, Yuan L, Wu H, Grzybowski A, Ye J. Exploring large language model for next generation of artificial intelligence in ophthalmology. Front Med (Lausanne) 2023;10:1291404. [Crossref] [PubMed]
- Gopalakrishnan N, Joshi A, Chhablani J, Yadav NK, Reddy NG, Rani PK, Pulipaka RS, Shetty R, Sinha S, Prabhu V, Venkatesh R. Recommendations for initial diabetic retinopathy screening of diabetic patients using large language model-based artificial intelligence in real-life case scenarios. Int J Retina Vitreous 2024;10:11. [Crossref] [PubMed]
- Choudhary A, Gopalakrishnan N, Joshi A, Balakrishnan D, Chhablani J, Yadav NK, Reddy NG, Rani PK, Gandhi P, Shetty R, Roy R, Bavaskar S, Prabhu V, Venkatesh R. Recommendations for diabetic macular edema management by retina specialists and large language model-based artificial intelligence platforms. Int J Retina Vitreous 2024;10:22. [Crossref] [PubMed]
- Gilson A, Chen Q, Singer M, Xu H, Adelman RA. Large language models and the retina: a review of current applications and future directions. Journal of Retina Vitreous 2023;32:225-30.
- Betzler BK, Chen H, Cheng CY, Lee CS, Ning G, Song SJ, et al. Large language models and their impact in ophthalmology. Lancet Digit Health 2023;5:e917-24. [Crossref] [PubMed]
- Huang AS, Hirabayashi K, Barna L, Parikh D, Pasquale LR. Assessment of a Large Language Model's Responses to Questions and Cases About Glaucoma and Retina Management. JAMA Ophthalmol 2024;142:371-5. [Crossref] [PubMed]
- Ferro Desideri L, Roth J, Zinkernagel M, Anguita R. Application and accuracy of artificial intelligence-derived large language models in patients with age related macular degeneration Int J Retina Vitreous 2023;9:71. [Crossref] [PubMed]
- Shamshad F, Khan S, Zamir SW, Khan MH, Hayat M, Khan FS, Fu H. Transformers in medical imaging: A survey. Med Image Anal 2023;88:102802. [Crossref] [PubMed]
- Tian D, Jiang S, Zhang L, Lu X, Xu Y. The role of large language models in medical image processing: a narrative review. Quant Imaging Med Surg 2024;14:1108-21. [Crossref] [PubMed]
- Oulhadj M, Riffi J, Khodriss C, Mahraz AM, Yahyaouy A, Abdellaoui M, Andaloussi IB, Tairi H. Diabetic retinopathy prediction based on vision transformer and modified capsule network. Comput Biol Med 2024;175:108523. [Crossref] [PubMed]
- Chen X, Zhang W, Xu P, Zhao Z, Zheng Y, Shi D, He M. FFA-GPT: an automated pipeline for fundus fluorescein angiography interpretation and question-answer. NPJ Digit Med 2024;7:111. [Crossref] [PubMed]
- Antaki F, Chopra R, Keane PA. Vision-Language Models for Feature Detection of Macular Diseases on Optical Coherence Tomography. JAMA Ophthalmol 2024;142:573-6. [Crossref] [PubMed]