Dual-layer detector spectral computed tomography quantitative parameters for predicting pathological complete remission after neoadjuvant treatment of breast cancer
Introduction
As a common cancer among women around the world, breast cancer (BC) has attracted widespread attention. On 1 February 2024, according to the latest data released by the Agency for Research on Cancer, the number of new cases of BC in the world reached 2.3 million, ranking second among cancers in the world, and becoming the fourth highest cause of cancer-related deaths with 670,000 deaths (1). In contemporary times, the management of BC involves a comprehensive strategy that includes surgical intervention, chemotherapy, radiation therapy, and systemic treatment (2-4). The aim is to combine and sequence these different treatments according to each patient’s needs and preferences and to de-escalate treatment whenever possible while preserving oncological safety (5). Neoadjuvant therapy (NAT) has become a treatment option for patients with early-stage BC (6,7). NAT has the potential to decrease tumor size through preoperative intervention, thereby converting inoperable BC to operable BC and non-breast-conserving BC to breast-conserving BC. Additionally, it can enhance the likelihood of successful surgical outcomes and provide valuable in vivo drug sensitivity information to guide postoperative treatment (6). NAT is frequently employed in 17–40% of early BC patients (8). The NAT strategy for BC has also continued to develop and evolve. The indication has shifted from being based on stage to comprehensively considering stage and molecular typing (9). The treatment model has been updated from single chemotherapy to chemotherapy combined with anti-human epidermal growth factor receptor 2 (HER2)-targeted therapy, chemotherapy combined with immunotherapy, endocrine therapy, and so on. The present advancement of NAT for BC primarily focuses on HER2-positive BC, triple-negative breast cancer (TNBC), and hormone receptor (HR)-positive BC based on molecular categorization (10). In the case of HER2-positive BC, NAT has evolved from a singular chemotherapy regimen to a combination of chemotherapeutic agents and targeted medications. Notably, the dual targeting regimen of trastuzumab in conjunction with pertuzumab has emerged as the fundamental approach in NAT for HER2-positive BC (11).
Ideally, it could imply an extremely favorable long-term outcome when a pathological complete response (pCR) is achieved after NAT (7,12). Roughly 20% of patients experience pCR following NAT, with variations in rates based on the subtype and stage of BC (13). Registry data shows that 40% of women with HER2-positive BC achieve pCR after NAT, with 23% among triple negative tumors and only 0.3% among luminal A tumors (9,14). Recent studies on dual HER2 blockade and carboplatin regimens in the treatment of triple-negative tumors have shown pCR rates as high as 68% and 80%, respectively, suggesting that pCR rates after NAT may increase further in the next years (13,15).
At present, BC patients who achieve pCR after NAT still have to undergo routine breast surgery without considering the obvious degree of pathological remission. In view of the high pCR rate of some subgroups after NAT, especially when breast-conserving surgery patients receive adjuvant whole breast radiotherapy, it should be questioned whether surgery is a redundant link in the overall management of this part of BC (5). If breast surgery is not performed, however, the standard method of determining response to NAT, being pathological examination of the surgical specimen, is not possible. The main impediments for potential elimination of breast surgery have been the fact that conventional and functional breast imaging techniques are incapable of accurate prediction of residual disease. In addition, a study showed that 10–35% of BCs were insensitive to NAT, and approximately 5% of patients had larger tumors after NAT (16); in these cases, NAT failed to exhibit a therapeutic effect and instead delayed surgical treatment. A recent meta-analysis discovered that patients who received NAT had a 15-year local recurrence rate that was 5.5% higher compared to those who received conventional chemotherapy (21.4% vs. 15.9%) (6). The varied outcomes of NAT, based on histopathological and molecular features, further complicate the accurate prediction of pCR prior to treatment (17), which is important for effective treatment planning. The increasing understanding of the variety of BC has led to a growing need for techniques that can help assess the early response to NAT in individual patients. There is a lack of imaging markers that enable noninvasive pretreatment prediction of pCR. Primary breast tumors and axillary lymph node metastases often respond similarly in pattern and degree of response (18). In this article, we mainly focus on the response in the breast to NAT.
Dual-layer detector spectral computed tomography (DLCT) is a new imaging technology offering functional imaging and molecular imaging. DLCT allows for the utilization of a dual-layer detector to capture both low- and high-energy photons within the same imaging beam. It offers energy spectrum analysis in the original data space, resulting in the generation of multi-parameter images. These images, including monoenergetic images and base material images, serve as analytical tools and quantitative indicators for clinical diagnosis. DLCT is capable of quantitatively measuring the concentration and decomposition, as well as inhibiting or enhancing the ability of materials such as iodine, water, calcium, or uric acid (19).
DLCT can provide 12 categories of energy parameters; this article will introduce a few commonly used parameters for breast diseases. By acquiring attenuation values at two different energy levels, it is possible to generate supplementary datasets, including an iodine no water, color-coded Z-effective images and the spectral Hounsfield unit (HU) curve, alongside conventional computed tomography (CT) images. Its application in the field of breast diseases has begun to receive widespread attention, such as distinguishing tumor lesions, pathological classification, molecular subtypes, analyzing immunohistochemical biomarkers, and determining the stage of lymph nodes (20-25). Thus, we aimed to investigate the value of quantitative multiparametric data, such as iodine concentration (IC), the spectral HU curve, and effective atomic number (Zeff) in predicting pathological complete remission after NAT of BC detected on DLCT. We present this article in accordance with the STARD reporting checklist (available at https://qims.amegroups.com/article/view/10.21037/qims-24-511/rc).
Methods
Patients
The study was conducted in accordance with the Declaration of Helsinki (as revised in 2013). This retrospective study was approved by the Ethics Committee of Shandong Cancer Hospital and Institute (approval No. SDTHEC 2023010022) and the requirement for individual consent for this retrospective analysis was waived. The patients who received NAT and underwent surgical treatment between 2019 and 2023 were consecutively enrolled in our study, and we obtained the imaging data and medical records of these patients. The inclusion criteria were as follows: (I) biopsy-verified primary BC without distant metastasis; (II) breast multiparametric DLCT performed before the biopsy; (III) complete NAT with no prior treatment; and (IV) surgery followed by a pathological examination performed after completion of NAT. The exclusion criteria were as follows: (I) combination treatment with multiple NAT regimens (chemotherapy + targeting + immunity); (II) ductal carcinoma in situ, inflammatory BC; (III) history of other malignant tumors; (IV) lack of some images (e.g., lack of arterial phase imaging); (V) low-quality images or the hardly visible lesion rendering difficulty with measurements (e.g., owing to motion artifacts); (VI) neoadjuvant immunotherapy (small sample size); and (VII) surgery was performed at an outside institution.
NAT and classification criteria
All patients received 6–8 cycles of NAT. Although there were some differences among the different patients, the treatment protocol and timeline followed the National Comprehensive Cancer Network guideline (26). All patients had not received any other treatment before or during treatment with NAT, and patients treated with multiple NAT methods in combination (chemotherapy + immunity + targeting) were excluded. Samples that meet the inclusion criteria were divided into two groups based on the treatment method, namely the neoadjuvant chemotherapy group (NC group) and the neoadjuvant targeted therapy group (NT group).
The indication for NAT in the 2023 Chinese Society of Clinical Oncology guidelines for the diagnosis and treatment of BC is one of the following conditions: (I) large mass (>5 cm); (II) TNBC; (III) HER2-positive BC; (IV) axillary lymph node metastasis; and (V) the tumor is large and difficult to conserve breast, but there is still a willingness to conserve breast. In addition, the guidelines prioritize HER2-positive BC in recommending treatment options that include single- or dual-targeted drugs. Chemotherapy regimens that include anthracyclines and taxanes are preferred for TNBC. For HR-positive BC, anthracyclines combined with taxanes are recommended.
The NAT regimen of the enrolled patients was determined by two clinicians in Shandong Cancer Hospital and Institute. The grouping criteria were as follows: (I) NC group: a simple chemotherapy regimen containing chemotherapeutic drugs such as anthracyclines and taxanes, such as EC-T, AC-T, TAC, and AT; (II) NT group: chemotherapy combined with anti-HER2 targeted (trastuzumab and pertuzumab) therapy, such as TCbH, TCbHP, AC-THP, and THP.
Pathological assessment of response
All patients who received NAT underwent a comprehensive preoperative evaluation before treatment and the clinical staging of BC according to the American Joint Committee on Cancer (AJCC, 8th edition, 2018) (27), and according to the 2013 International Expert Consensus on the Primary Treatment of Early Breast Cancer in St. Gallen (28,29), BC was subdivided into four molecular subtypes, namely luminal A, luminal B, HER2-rich, and TNBC. The patient’s clinical baseline data including menstrual status, estrogen receptor (ER), progesterone receptor (PR), HER2 and Ki-67 status, clinical stage, molecular typing, and so on, were collected.
The conventional histopathological analysis method was employed to assess the response to NAT for pathological evaluation. The Miller-Payne grading system (30) is a common method for assessing the pathological response to NAT in BC. This system evaluates treatment efficacy by categorizing the pathological response into five grades based on the comparison of tumor cell density and morphology between pre-treatment core biopsies and definitive surgical specimens. The Miller-Payne grading system is as follows:
- Grade 1: no change or some minor alteration in individual malignant cells, but no reduction in overall cellularity.
- Grade 2: a minor loss of tumor cells, but overall high cellularity; up to 30% reduction of cellularity.
- Grade 3: between an estimated 30% and 90% reduction in tumor cellularity.
- Grade 4: a marked disappearance of more than 90% of tumor cells such that only small clusters or widely dispersed individual cells remain (almost pCR).
- Grade 5: no invasive malignant cells identifiable in sections from the site of the tumor (pCR).
In this study, Grade 5 cases were classified as pCR. Grade 1–4 cases were classified as non-pCR. The grouping of this study is shown in Table 1.
Table 1
Treatment methods | Arterial phase | Venous phase | |||
---|---|---|---|---|---|
pCR | Non-pCR | pCR | Non-pCR | ||
Neoadjuvant chemotherapy group | 16 | 64 | 10 | 40 | |
Neoadjuvant targeted therapy group | 16 | 24 | 8 | 12 |
pCR, pathological complete response.
Spectral CT image acquisition
All breast CT imaging examinations were performed using DLCT (Philips IQon Spectral CT; Philips, Amsterdam, Netherlands), within 1 week before initiating NAT. The imaging sequence included arterial phase iodine density map, color-coded Z-effective images, and the spectral HU curve, and conventional CT images, including venous phase images in some cases. A set of manual sketching examples in Spectral Diagnostic Suite is presented in Figure 1.
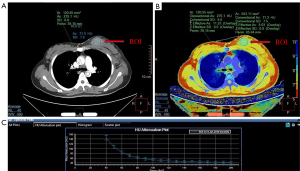
Image data were acquired on a DLCT unit (Philips IQon Spectral CT) in dual layer detector mode through two layers of detectors to simultaneously collect low- and high-energy data in all patients using standard CT protocols. The settings for the scanners were as follows: tube voltage, 100 kVp; reference tube current time product, 260–350 mAs; pitch, 1.375; reformatted section thickness, 5 mm.
Holland Philips IQon Spectral CT machine was used for chest biphasic enhanced scanning. The entire chest was examined from the superior opening of the thorax to the lower border of the costophrenic angle while taking a deep breath, encompassing the breast and armpit area. For contrast-enhanced scanning, an iodinated nonionic contrast media (ioversol, 320 mg/mL iodine; HENGRUI Medicine, Jiangsu, China) was administered through the right or left ulnar vein by a dual-head injector. The dosage was 1.5 mL/kg with a flow rate of 2.5 mL/s, followed by a bolus injection of 30 mL of saline given at the same flow rate. The ulnar vein on the opposite side of the suspected breast lesion was chosen for injection to prevent a beam hardening artifact in the axillary vein. Following the injection, the arterial phase scans began using a bolus-tracking method with a threshold of 250 HU in the descending aorta. The venous phase scanning was delayed by 45 seconds after the arterial phase scanning was completed.
DLCT image analysis
All the images were analyzed by using viewer software on a workstation (Intelli Space Portal version 10.0). DLCT quantitative parameters were measured by two radiologists (W.L., with 10 years of experience in breast and chest diagnostic imaging, and S.G., with 2 years of experience in post-reconstruction imaging, Philips) who were blinded to the immune histochemical results of invasive BC, by placing a circular region of interest (ROI), excluding any area of obviously gross necrosis, calcification, or large vessels. The immunohistochemical results of the puncture were unknown, and consistency analysis was performed by intra-class coefficient correlation (ICC), with the average of the two taken as the final result. The ROI region was selected according to the arterial phase image, and the ROI region was pasted on iodine density map and atomic number image using the copy function to ensure that the size and position of the ROI region are completely consistent. The CT value, IC, and Zeff of the ROI were divided by the value of the aorta in the same layer, respectively, to obtain the normalized CT value (NCT), normalized iodine concentration (NIC), and normalized effective atomic number (NZeff). The slope of the spectral HU curve (λHU) was calculated as follows (23):
Statistical analysis
Statistical analyses were performed using the commercially available statistical software SPSS 22.0 (IBM Corp., Armonk, NY, USA). The ICC was used to evaluate the consistency of the two readers in the measurement of quantitative parameters of DLCT, and an ICC >0.75 indicated good consistency. In addition to age, clinical baseline data were selected based on sample size characteristics for Fisher’s exact Chi-squared test. Age was used to analyze the significance of differences by t-test. The Mann-Whitney U test was used to compare the distribution differences of DLCT quantitative parameters between the pCR group and the non-pCR group. Receiver operating characteristic (ROC) curve analysis was used to evaluate the diagnostic capacity of DLCT quantitative parameters, and the corresponding sensitivity and specificity were obtained. The level of significance was defined as P<0.05. The Mann-Whitney U test was a two-tailed analysis and the ROC curve analysis was a one-tailed analysis.
Results
Clinical baseline characteristics of patients
A total of 120 patients were enrolled in our study; all participants in this study are female. A flowchart of the study population is shown below (Figure 2). The patients’ pathological results were verified by the pathology department of Shandong Cancer Hospital and Institute to meet the enrollment criteria. The pathological type of the patients was invasive cancer, and the clinical tumor node metastasis (cTNM) stages were all I–III. Detailed patient statistics are summarized in Table 2. The results of Chi-squared test showed that the efficacy of NAT was not correlated with clinical factors such as menstrual status, ER, PR, HER2, cTNM stage, and molecular typing (P>0.05). However, the results of Chi-squared test showed that the efficacy of NC was correlated with Ki-67 (P=0.028).
Table 2
Clinical characteristics | Neoadjuvant chemotherapy | Neoadjuvant targeted therapy | |||||
---|---|---|---|---|---|---|---|
pCR | Non-pCR | P value | pCR | Non-pCR | P value | ||
Age (years) | 50.1±10.7 | 47.5±10.7 | 0.153 | 49.3±11.7 | 48.9.1±10.6 | 0.223 | |
Menstrual status | 0.833 | 0.833 | |||||
Non-menopausal | 9 (56.3) | 37 (57.8) | 8 (50.0) | 11 (45.8) | |||
Menopausal | 7 (43.7) | 27 (42.2) | 8 (50.0) | 13 (54.2) | |||
ER | 0.573 | 0.272 | |||||
(+) | 1 (6.3) | 6 (9.4) | 2 (12.5) | 7 (29.2) | |||
(−) | 15 (93.7) | 58 (90.6) | 14 (87.5) | 17 (70.8) | |||
PR | 0.655 | 0.261 | |||||
(+) | 1 (6.3) | 5 (7.8) | 3 (18.8) | 8 (33.3) | |||
(−) | 15 (93.7) | 59 (92.2) | 13 (81.2) | 16 (66.7) | |||
HER2 | 0.833 | 0.212 | |||||
(+) | 0 | 2 (3.1) | 15 (93.7) | 19 (79.2) | |||
(−) | 16 (100.0) | 62 (96.9) | 1 (6.3) | 5 (20.8) | |||
Ki-67 | 0.028 | 0.165 | |||||
High expression (>20) | 14 (87.5) | 48 (75.0) | 12 (75.0) | 20 (83.3) | |||
Low expression (≤20) | 2 (12.5) | 16 (25.0) | 4 (25.0) | 4 (16.7) | |||
Clinical TNM stage | 0.642 | 0.146 | |||||
I/II | 11 (68.8) | 40 (62.5) | 9 (56.2) | 6 (25.0) | |||
III | 5 (31.2) | 24 (37.5) | 7 (43.8) | 18 (75.0) | |||
Molecular typing | 0.629 | 0.629 | |||||
Luminal A | 0 | 3 (4.7) | 0 | 1 (4.2) | |||
Luminal B | 1 (6.3) | 1 (1.6) | 2 (1.3) | 6 (25.0) | |||
HER2-rich | 0 | 2 (3.1) | 14 (8.7) | 17 (70.8) | |||
Triple negative | 15 (93.7) | 58 (90.6) | 0 | 0 |
Data are presented as mean ± standard deviation or n (%). (+) = positive, (−) = negative. pCR, pathological complete response; ER, estrogen receptor; PR, progesterone receptor; HER2, human epidermal growth factor receptor 2; TNM, tumor node metastasis.
Comparison of DLCT quantitative parameters of pathological pCR and non-pCR in BC patients after NAT
The ICC value measured by the two physicians for arterial phase DLCT quantitative parameters was 0.942 (range, 0.801 to 0.979), indicating that the quantitative parameters measured by the two doctors have strong consistency. In the NC group, the pCR group tended to display higher arterial phase λHU, IC, Zeff, and NIC compared with the non-pCR group, and the difference was statistically significant (P<0.05). In the NT group, the non-pCR group tended to display higher arterial phase CT value, IC, Zeff, and NIC compared with the pCR group (P<0.05), and the difference was statistically significant (Table 3).
Table 3
Parameters | Neoadjuvant chemotherapy | Neoadjuvant targeted therapy | |||||
---|---|---|---|---|---|---|---|
pCR | Non-pCR | P value | pCR | Non-pCR | P value | ||
Arterial phase λHu (HU/keV) | 2.35±0.387 | 1.37±0.107 | 0.018 | 1.725±0.336 | 2.199±0.137 | 0.057 | |
Arterial phase CT value (HU) | 64.148±19.117 | 54.264±11.382 | 0.496 | 53.150±3.909 | 65.889±2.013 | 0.010 | |
Arterial phase NCT (HU) | 0.260±0.035 | 0.214±0.012 | 0.517 | 0.199±0.016 | 0.243±0.011 | 0.057 | |
Arterial phase IC (mg/mL) | 1.346±0.256 | 0.732±0.065 | 0.010 | 0.805±0.112 | 1.173±0.091 | 0.020 | |
Arterial phase NIC | 0.178±0.042 | 0.089±0.009 | 0.018 | 0.085±0.132 | 0.122±0.009 | 0.047 | |
Arterial phase Zeff | 8.056±0.106 | 7.700±0.035 | 0.003 | 7.757±0.065 | 7.944±0.039 | 0.025 | |
Arterial phase NZeff | 0.765±0.023 | 0.712±0.017 | 0.088 | 0.711±0.010 | 0.725±0.007 | 0.343 |
Data are presented as mean ± standard deviation. pCR, pathological complete response; λHu, the slope of the spectral HU curve; HU, Hounsfield unit; CT, computed tomography; NCT, normalized CT value; IC, iodine concentration; NIC, normalized iodine concentration; Zeff, effective atomic number; NZeff, normalized effective atomic number.
Analysis of diagnostic efficiency of DLCT quantitative parameters
The quantitative parameters with statistically significant differences between pCR and non-pCR in BC patients after NAT were included in the ROC curve (Figure 3 and Table 4). In the NC group, the results showed that the area under the curve (AUC) of λHU, IC, Zeff, and NIC were 0.768, 0.791, 0.834, and 0.770, respectively, and the difference was statistically significant (P<0.05). Taking the arterial phase Zeff of 7.97 as the diagnostic threshold, it had the highest diagnostic efficiency in differential diagnosis of pCR versus non-pCR (AUC of 0.834, sensitivity of 62.50%, specificity of 90.63%). In the NT group, the results showed that the AUC of CT value, IC, Zeff, and NIC were 0.844, 0.813, 0.802, and 0.766, respectively, and the difference was statistically significant (P<0.05). Taking the arterial phase CT value of 57.3 as the diagnostic threshold, it had the highest performance in differential diagnosis of pCR versus non-pCR (AUC of 0.844, sensitivity of 75.00%, specificity of 91.67%).
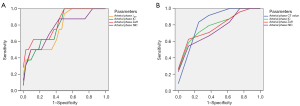
Table 4
Parameters | AUC (95% CI) | Threshold of parameter | Sensitivity (%) | Specificity (%) | P value |
---|---|---|---|---|---|
Neoadjuvant chemotherapy pCR vs. non-pCR | |||||
Arterial phase λHu (HU/keV) | 0.768 (0.5914–0.9438) | 1.27 | 100.00 | 50.00 | 0.021 |
Arterial phase IC (mg/mL) | 0.791 (0.6314–0.9506) | 0.66 | 100.00 | 50.00 | 0.012 |
Arterial phase Zeff | 0.834 (0.6803–0.9877) | 7.97 | 62.50 | 90.63 | 0.004 |
Arterial phase NIC | 0.770 (0.5747–0.9644) | 0.15 | 50.00 | 96.88 | 0.020 |
Neoadjuvant targeted therapy pCR vs. non-pCR | |||||
Arterial phase CT value (HU) | 0.844 (0.6403–1.0472) | 57.3 | 75.00 | 91.67 | 0.011 |
Arterial phase IC (mg/mL) | 0.813 (0.6248–1.0002) | 1.09 | 87.50 | 66.67 | 0.021 |
Arterial phase Zeff | 0.802 (0.6097–0.9944) | 7.92 | 100.00 | 58.33 | 0.025 |
Arterial phase NIC | 0.766 (0.5567–0.9746) | 0.13 | 100.00 | 50.00 | 0.049 |
pCR, pathological complete response; λHu, the slope of the spectral HU curve; HU, Hounsfield unit; IC, iodine concentration; Zeff, effective atomic number; NIC, normalized iodine concentration; CT, computed tomography; AUC, area under the curve; CI, confidence interval.
Statistical analysis of DLCT venous phase quantitative parameters
Our study included 120 patients, among whom 70 included venous phase images as well as arterial phase images. The ICC value of the quantitative parameters measured by the two physicians for venous phase DLCT was 0.878 (range, 0.791 to 0.970), indicating that the quantitative data measured by the two physicians had strong consistency. We obtained the quantitative parameters of DLCT venous phase according to the same method, and statistical analysis was performed using the Mann-Whitney U test. The results showed that there was no significant difference (P>0.05) in DLCT venous phase quantitative parameters between pCR and non-pCR (Table 5).
Table 5
Parameters | Neoadjuvant chemotherapy | Neoadjuvant targeted therapy | |||||
---|---|---|---|---|---|---|---|
pCR | Non-pCR | P value | pCR | Non-pCR | P value | ||
Venous phase λHu (HU/keV) | 3.206±0.698 | 2.611±0.788 | 0.148 | 2.960±0.934 | 3.593±0.931 | 0.352 | |
Venous phase CT value (HU) | 71.712±8.157 | 72.061±14.649 | 0.921 | 74.825±13.532 | 88.838±15.070 | 0.067 | |
Venous phase NCT (HU) | 0.518±0.098 | 0.521±0.120 | 0.921 | 0.510±0.136 | 0.628±0.097 | 0.171 | |
Venous phase IC (mg/mL) | 1.512±0.314 | 1.320±0.370 | 0.336 | 1.508±0.443 | 1.807±0.449 | 0.476 | |
Venous phase NIC | 0.388±0.087 | 0.328±0.104 | 0.216 | 0.373±0.139 | 0.433±0.094 | 0.610 | |
Venous phase Zeff | 8.134±0.158 | 8.037±0.203 | 0.303 | 8.138±0.216 | 8.275±0.216 | 0.476 | |
Venous phase NZeff | 0.890±0.021 | 0.871±0.032 | 0.272 | 0.880±0.036 | 0.892±0.020 | 0.762 |
Data are presented as mean ± standard deviation. pCR, pathological complete response; λHu, the slope of the spectral HU curve; HU, Hounsfield unit; CT, computed tomography; NCT, normalized CT value; IC, iodine concentration; NIC, normalized iodine concentration; Zeff, effective atomic number; NZeff, normalized effective atomic number.
Discussion
Currently, there is still no standard method to predict responses to NAT, and which diagnostic tools can accurately confirm or rule out residual disease in the breast after NAT remains to be determined. At present, there are a variety of imaging methods available to predict the feasibility of NAT for BC, but they all have some limitations. Multiple small prospective and retrospective trials have produced varying but generally mediocre results in terms of the diagnostic accuracy of imaging in determining residual disease after NAT (31,32). Diagnostic accuracy might also depend on tumor biology (33). Dynamic contrast-enhanced magnetic resonance imaging (DCE MRI) of the breast offers the highest diagnostic accuracy in primary tumor therapy response assessment among the currently established methods (physical examination, mammography, and ultrasound) (31). Based on DCE MRI feature analysis, combined with intratumoral and peri-tumor dynamic enhanced magnetic resonance imaging texture features can accurately predict the pCR, with a reported AUC value of 0.74 after NAT (34). In a combined analysis of six studies, the positive predictive value (ability to correctly predict the presence of residual disease at final pathologic examination) was high at 93%. The negative predictive value (the ability to correctly predict disease at the final pathological examination) was only 65%, which reduced the overall diagnostic accuracy to 84% (35). In addition, changes in pharmacologic kinetic model parameters have been investigated in BC patients undergoing NC. Two published systematic reviews suggest that the volume transfer constant (Ktrans) is a promising parameter for early identification of treatment response (36,37). Although clinical DCE MRI is routinely performed in accordance with the standards of the American Society of Radiology, the use of DCE MRI for pharmacokinetic analysis is still in the research stage (31).
Despite these promising data, DCE MRI is currently not reliable enough to allow patients to avoid surgical resection after a complete imaging response (31); limitations remain in detecting small tumor foci scattered after NAT (34,38). It may overestimate or underestimate the residual lesions, and its accuracy is also closely related to the morphology, histology, atrophy pattern, and molecular subtypes of the tumor (39,40). In diffuse cell loss, tumors degenerate in a more uneven manner, leaving multiple scattered foci of single tumor cells after chemotherapy, often with little or no change in overall tumor size (41).
Positron emission tomography has a sensitivity of 84.0% and a specificity of 66.0% in predicting pCR after NAT. It can be used to identify non-remission lesions and replace non-cross-resistant NAT regimens in the early stage of NAT (42). Formation of tumor fibrosis occurs after NAT, residual intraductal carcinoma, and tumor remaining after the disappearance of invasive cancer components. The change in density leads to inaccurate evaluation of residual tumors by mammography and ultrasound (43).
Minimally invasive image-guided biopsy has demonstrated potential in detecting residual disease in the breast following NAT (44). Nonetheless, conflicting concerns emerge: for instance, the sensitivity and specificity of image-guided biopsy may be influenced by factors such as molecular subtypes, tumor heterogeneity, and the dimensions of both initial and residual imaging abnormalities, which may present challenges in terms of ease and accuracy of sampling (45).
Future clinical trials may explore the incorporation of biomarkers alongside image-guided biopsies to predict pCR without necessitating surgical intervention, as this approach has the potential to enhance diagnostic precision. Early research has shown that the alpha- and gamma-adaptin binding protein monotherapy biomarker can predict pCR in small samples with an accuracy of 78–100% (46). Other studies have shown that higher levels of tumor-infiltrating lymphocytes and anti-HER2 CD4+ T-helper type 1 are associated with higher polymerase chain reaction ratios (47,48). However, these biomarkers have not yet been clinically confirmed.
Considering there is still no standard method to predict responses to NAT, this study aimed to evaluate the value of quantitative parameters of DLCT for pretreatment prediction of pCR to NAT for BC.
The recognized imaging method for NAT evaluation is DCE MRI; however, it still has some shortcomings and many contraindications. CT examination is convenient, fast, affordable, and can be “one-stop” scanning. It cannot only observe breast lesions, but also understand lymph node and lung metastases. As a new technology for CT examination, DLCT is increasingly being used and has been shown to be useful in tumor imaging across multiple organ systems (49-51). Based on the concept that biomedical images contain information that may reflect underlying pathophysiology, and that their relationships could be revealed through quantitative image analyses, quantitative parameters of DLCT turn medical images into minable data to improve diagnostic, prognostic, and predictive accuracy, thereby bridging the gap between medical imaging and personalized medicine. Iodine density maps provide quantitative information about tissue vascularity and perfusion. Virtual monoenergetic images at lower keV allow enhancement of iodine and could lead to a better delineation of breast lesions that contain iodine, thus increasing their visibility and detectability (49,52). Some studies have found that quantitative parameters of dual energy CT may allow estimating the benign or malignant nature of breast masses, such as NIC, λHU, and NZeff (21,25,53). Using color-coded Zeff images is more efficient than comparing HU values on traditional CT scans for differentiating between various tissues based on their effective atomic number (49,52). Wang et al. (54) found that venous phase NIC, as well as arterial and venous phase NZeff, can distinguish between the luminal A and non-luminal A subtypes. Some researchers have shown that it can be used to distinguish between positive benign focal findings that do not require further clarification and suspicious or positive malignant focal findings, thus helping to avoid unnecessary follow-up examinations (21,53,54). Dual energy CT can predict the pathological classification, molecular subtype, and immunohistochemical classification of BC (24,25,54,55). To the best of our knowledge, our study is the first to predict breast pCR of NAT using quantitative parameters of DLCT. No invasive procedure was needed in our study. Therefore, we evaluated the value of DLCT quantitative parameters in predicting pCR before NAT for BC. Our research shows that DLCT can provide useful value in predicting the efficacy of NAT in BC patients.
We explored the potential reasons for the significant differences in arterial phase DLCT parameters between pCR and non-pCR groups. The DLCT iodine uptake value is used to calculate the actual IC of the contrast agent in the enhanced image, which more accurately reflects the blood supply and enhancement characteristics of the tissue than the CT value. The iodine map is the distribution map that the DLCT reflects the iodine uptake (49,56). In this study, there were differences in IC and NIC between the pCR and non-pCR groups. This may have been due to the different number of new blood vessels in the lesion and the inflammatory reaction caused by tumor cell degeneration and necrosis leading to changes in vascular permeability, resulting in different IC in the lesion. The arterial and venous phase energy spectrum curves of BC patients showed a downward trend. As the keV level rises to about 70 keV, the energy spectrum curves tend to be parallel, so the slope of the 40–70 keV range was selected for analysis in this study. The richer the blood vessels in the tumor tissue, the more the iodine uptake, and the more obvious the attenuation of the energy spectrum curve. Therefore, the λHU can indirectly reflect the tumor by reflecting the blood supply. As an important parameter of DLCT, the principle of Zeff is to add the information of material composition to each pixel and present it in the form of color quantization. In this study, the difference of Zeff value between pCR and non-pCR groups reflects the difference of material composition between the two groups.
In this study, our results showed that there is a correlation between DLCT arterial phase quantitative parameters and BC pCR of NAT, which can provide a reference value for the clinical prediction of breast pCR of NAT. In addition, we obtained a total of seven sets of arterial phase data. Each group only screened out four parameters with statistically significant differences (P<0.05). After pairwise combination of other parameters (P>0.05), we further explored the potential value of DLCT quantitative parameters. Among the arterial phase parameters of DLCT in the NC group, a good diagnostic efficiency can also be achieved by combining arterial phase CT with NCT and NZeff. The AUC was 0.762 (sensitivity of 50.00%, specificity of 96.88%, P=0.23). But in the group NT, the diagnostic effect of the combination of parameters with P>0.05 was poor. This study has several limitations. Firstly, the number of cases was limited, the data of pCR and non-pCR distribution was unbalanced. Clinically, the number of BC patients who received a preoperative DLCT scan and completed NAT was relatively small. pCR of NAT was encountered less frequently, which is consistent with clinical observation. Nonetheless, the ROC curves exhibited sensitivity to imbalanced datasets, which may have impacted the interpretations drawn from the findings. Therefore, it is recommended that additional research involving larger sample sizes be conducted to corroborate the results of the current study through the application of thresholding techniques. Secondly, we focused only on two therapeutic methods in NAT, because the number of cases for other treatments was too small and there are no further studies. The quantity of data obtained from the venous phase images in these cases was limited, which could have led to significant errors in data analysis. Therefore, it is important to further explore the potential of quantitative parameters in the venous phase DLCT. Additional research utilizing larger sample sizes and a broader range of NATs for BC is necessary.
- Predicting response to radiotherapy and chemotherapy:
- Current limitation: currently, the application of DLCT in assessing the efficacy of radiotherapy and chemotherapy for cancer is limited. This is primarily because DLCT technology is still in its developmental phase and its full potential in this area has not been fully explored.
- Future research: future research should focus on developing and validating DLCT-based biomarkers that can predict the response of tumors to radiotherapy and chemotherapy. This could involve using DLCT to measure changes in tumor blood flow, perfusion, and metabolic activity, which are known to be affected by these treatments. By identifying such biomarkers, clinicians could potentially tailor treatment plans to individual patients, improving outcomes and reducing unnecessary toxicity.
- Combining DLCT imaging with radiomics:
- Current potential: in the era of personalized medicine, radiomics—the extraction of quantitative features from medical images—holds great promise for enhancing various aspects of BC management, including diagnosis, prognosis, prediction, monitoring, image-guided interventions, and evaluation of treatment response.
- Integration with DLCT: by combining DLCT imaging with radiomics, researchers can potentially unlock new insights into BC biology and behavior. DLCT provides additional spectral information that can be used to differentiate tissues based on their iodine content and other material properties. This spectral information, combined with radiomic features, could lead to more accurate and personalized assessments of BC.
- Future research: future research should explore the integration of DLCT and radiomics in BC management. This could involve developing and validating radiomic models that incorporate DLCT-derived features to improve diagnostic accuracy, predict prognosis, and monitor treatment response. Additionally, studies should investigate the potential of these models to guide personalized treatment decisions, such as selecting the most appropriate therapy based on an individual patient’s tumor characteristics.
Conclusions
The present preliminary study suggested a possibility that DLCT provided a potential tool to predict pCR to NAT in BC. The early prediction of the efficacy of NAT offers the opportunity to modify preoperative treatments or aids in determining surgical options.
Acknowledgments
Funding: This work was supported by
Footnote
Reporting Checklist: The authors have completed the STARD reporting checklist. Available at https://qims.amegroups.com/article/view/10.21037/qims-24-511/rc
Conflicts of Interest: All authors have completed the ICMJE uniform disclosure form (available at https://qims.amegroups.com/article/view/10.21037/qims-24-511/coif). The authors have no conflicts of interest to declare.
Ethical Statement: The authors are accountable for all aspects of the work in ensuring that questions related to the accuracy or integrity of any part of the work are appropriately investigated and resolved. The study was conducted in accordance with the Declaration of Helsinki (as revised in 2013). The study was approved by ethics committee of Shandong Cancer Hospital and Institute (approval No. SDTHEC 2023010022) and individual consent for this retrospective analysis was waived.
Open Access Statement: This is an Open Access article distributed in accordance with the Creative Commons Attribution-NonCommercial-NoDerivs 4.0 International License (CC BY-NC-ND 4.0), which permits the non-commercial replication and distribution of the article with the strict proviso that no changes or edits are made and the original work is properly cited (including links to both the formal publication through the relevant DOI and the license). See: https://creativecommons.org/licenses/by-nc-nd/4.0/.
References
- Bray F, Laversanne M, Sung H, Ferlay J, Siegel RL, Soerjomataram I, Jemal A. Global cancer statistics 2022: GLOBOCAN estimates of incidence and mortality worldwide for 36 cancers in 185 countries. CA Cancer J Clin 2024;74:229-63. [Crossref] [PubMed]
- Biganzoli L, Marotti L, Hart CD, Cataliotti L, Cutuli B, Kühn T, Mansel RE, Ponti A, Poortmans P, Regitnig P, van der Hage JA, Wengström Y, Rosselli Del Turco M. Quality indicators in breast cancer care: An update from the EUSOMA working group. Eur J Cancer 2017;86:59-81. [Crossref] [PubMed]
- Telli ML, Gradishar WJ, Ward JH. NCCN Guidelines Updates: Breast Cancer. J Natl Compr Canc Netw 2019;17:552-5. [Crossref] [PubMed]
- Rubio IT, Sobrido C. Neoadjuvant approach in patients with early breast cancer: patient assessment, staging, and planning. Breast 2022;62:S17-24. [Crossref] [PubMed]
- Heil J, Kuerer HM, Pfob A, Rauch G, Sinn HP, Golatta M, Liefers GJ, Vrancken Peeters MJ. Eliminating the breast cancer surgery paradigm after neoadjuvant systemic therapy: current evidence and future challenges. Ann Oncol 2020;31:61-71. [Crossref] [PubMed]
- Long-term outcomes for neoadjuvant versus adjuvant chemotherapy in early breast cancer: meta-analysis of individual patient data from ten randomised trials. Lancet Oncol 2018;19:27-39. [Crossref] [PubMed]
- Broglio KR, Quintana M, Foster M, Olinger M, McGlothlin A, Berry SM, Boileau JF, Brezden-Masley C, Chia S, Dent S, Gelmon K, Paterson A, Rayson D, Berry DA. Association of Pathologic Complete Response to Neoadjuvant Therapy in HER2-Positive Breast Cancer With Long-Term Outcomes: A Meta-Analysis. JAMA Oncol 2016;2:751-60. [Crossref] [PubMed]
- Murphy BL, Day CN, Hoskin TL, Habermann EB, Boughey JC. Neoadjuvant Chemotherapy Use in Breast Cancer is Greatest in Excellent Responders: Triple-Negative and HER2+ Subtypes. Ann Surg Oncol 2018;25:2241-8. [Crossref] [PubMed]
- Haque W, Verma V, Hatch S, Suzanne Klimberg V, Brian Butler E, Teh BS. Response rates and pathologic complete response by breast cancer molecular subtype following neoadjuvant chemotherapy. Breast Cancer Res Treat 2018;170:559-67. [Crossref] [PubMed]
- Swain SM, Miles D, Kim SB, Im YH, Im SA, Semiglazov V, Ciruelos E, Schneeweiss A, Loi S, Monturus E, Clark E, Knott A, Restuccia E, Benyunes MC, Cortés J. CLEOPATRA study group. Pertuzumab, trastuzumab, and docetaxel for HER2-positive metastatic breast cancer (CLEOPATRA): end-of-study results from a double-blind, randomised, placebo-controlled, phase 3 study. Lancet Oncol 2020;21:519-30. [Crossref] [PubMed]
- Prat A, Pineda E, Adamo B, Galván P, Fernández A, Gaba L, Díez M, Viladot M, Arance A, Muñoz M. Clinical implications of the intrinsic molecular subtypes of breast cancer. Breast 2015;24:S26-35. [Crossref] [PubMed]
- Cortazar P, Zhang L, Untch M, Mehta K, Costantino JP, Wolmark N, et al. Pathological complete response and long-term clinical benefit in breast cancer: the CTNeoBC pooled analysis. Lancet 2014;384:164-72. [Crossref] [PubMed]
- van Ramshorst MS, van der Voort A, van Werkhoven ED, Mandjes IA, Kemper I, Dezentjé VO, Oving IM, Honkoop AH, Tick LW, van de Wouw AJ, Mandigers CM, van Warmerdam LJ, Wesseling J, Vrancken Peeters MT, Linn SC, Sonke GSDutch Breast Cancer Research Group (BOOG). Neoadjuvant chemotherapy with or without anthracyclines in the presence of dual HER2 blockade for HER2-positive breast cancer (TRAIN-2): a multicentre, open-label, randomised, phase 3 trial. Lancet Oncol 2018;19:1630-40. [Crossref] [PubMed]
- Houssami N, Macaskill P, von Minckwitz G, Marinovich ML, Mamounas E. Meta-analysis of the association of breast cancer subtype and pathologic complete response to neoadjuvant chemotherapy. Eur J Cancer 2012;48:3342-54. [Crossref] [PubMed]
- Santonja A, Albanell J, Chacon JI, Lluch A, Sanchez-Muñoz A, Rojo F, et al. Triple-negative breast cancer subtypes and pathologic complete-response rate to neoadjuvant chemotherapy: Results from the GEICAM/2006-2003 study. J Clin Oncol 2014;32:1024. [Crossref] [PubMed]
- Rastogi P, Anderson SJ, Bear HD, Geyer CE, Kahlenberg MS, Robidoux A, Margolese RG, Hoehn JL, Vogel VG, Dakhil SR, Tamkus D, King KM, Pajon ER, Wright MJ, Robert J, Paik S, Mamounas EP, Wolmark N. Preoperative chemotherapy: updates of National Surgical Adjuvant Breast and Bowel Project Protocols B-18 and B-27. J Clin Oncol 2008;26:778-85. [Crossref] [PubMed]
- von Minckwitz G, Untch M, Blohmer JU, Costa SD, Eidtmann H, Fasching PA, Gerber B, Eiermann W, Hilfrich J, Huober J, Jackisch C, Kaufmann M, Konecny GE, Denkert C, Nekljudova V, Mehta K, Loibl S. Definition and impact of pathologic complete response on prognosis after neoadjuvant chemotherapy in various intrinsic breast cancer subtypes. J Clin Oncol 2012;30:1796-804. [Crossref] [PubMed]
- Samiei S, van Nijnatten TJA, de Munck L, Keymeulen KBMI, Simons JM, Kooreman LFS, Siesling S, Lobbes MBI, Smidt ML. Correlation Between Pathologic Complete Response in the Breast and Absence of Axillary Lymph Node Metastases After Neoadjuvant Systemic Therapy. Ann Surg 2020;271:574-80. [Crossref] [PubMed]
- Johnson TR, Krauss B, Sedlmair M, Grasruck M, Bruder H, Morhard D, Fink C, Weckbach S, Lenhard M, Schmidt B, Flohr T, Reiser MF, Becker CR. Material differentiation by dual energy CT: initial experience. Eur Radiol 2007;17:1510-7. [Crossref] [PubMed]
- Okamura Y, Yoshizawa N, Yamaguchi M, Kashiwakura I. Application of Dual-Energy Computed Tomography for Breast Cancer Diagnosis. Int J Med Phys Clin Eng Radiat Oncol 2016;5:288-97.
- Lan X, Wang X, Qi J, Chen H, Zeng X, Shi J, Liu D, Shen H, Zhang J. Application of machine learning with multiparametric dual-energy computed tomography of the breast to differentiate between benign and malignant lesions. Quant Imaging Med Surg 2022;12:810-22. [Crossref] [PubMed]
- Cheng YG, Sun ZQ, Zhang HX, Mao GQ. An application study of low-dose computed tomography perfusion imaging (LDCTPI) in breast cancer and breast fibroadenoma. J Xray Sci Technol 2018;26:681-90. [Crossref] [PubMed]
- Zhang X, Zheng C, Yang Z, Cheng Z, Deng H, Chen M, Duan X, Mao J, Shen J. Axillary Sentinel Lymph Nodes in Breast Cancer: Quantitative Evaluation at Dual-Energy CT. Radiology 2018;289:337-46. [Crossref] [PubMed]
- Wang P, Tang Z, Xiao Z, Wu L, Hong R, Duan F, Wang Y, Zhan Y. Dual-energy CT in predicting Ki-67 expression in laryngeal squamous cell carcinoma. Eur J Radiol 2021;140:109774. [Crossref] [PubMed]
- Wang X, Liu D, Zeng X, Jiang S, Li L, Yu T, Zhang J. Dual-energy CT quantitative parameters for evaluating Immunohistochemical biomarkers of invasive breast cancer. Cancer Imaging 2021;21:4. [Crossref] [PubMed]
- Gradishar WJ, Anderson BO, Balassanian R, Blair SL, Burstein HJ, Cyr A, et al. Breast Cancer, Version 4.2017, NCCN Clinical Practice Guidelines in Oncology. J Natl Compr Canc Netw 2018;16:310-20. [Crossref] [PubMed]
- Giuliano AE, Edge SB, Hortobagyi GN. Eighth Edition of the AJCC Cancer Staging Manual: Breast Cancer. Ann Surg Oncol 2018;25:1783-5.
- Goldhirsch A, Winer EP, Coates AS, Gelber RD, Piccart-Gebhart M, Thürlimann B, Senn HJ. Panel members. Personalizing the treatment of women with early breast cancer: highlights of the St Gallen International Expert Consensus on the Primary Therapy of Early Breast Cancer 2013. Ann Oncol 2013;24:2206-23. [Crossref] [PubMed]
- Ignatiadis M, Buyse M, Sotiriou C. St Gallen International Expert Consensus on the primary therapy of early breast cancer: an invaluable tool for physicians and scientists. Ann Oncol 2015;26:1519-20. [Crossref] [PubMed]
- Ogston KN, Miller ID, Payne S, Hutcheon AW, Sarkar TK, Smith I, Schofield A, Heys SD. A new histological grading system to assess response of breast cancers to primary chemotherapy: prognostic significance and survival. Breast 2003;12:320-7. [Crossref] [PubMed]
- Fowler AM, Mankoff DA, Joe BN. Imaging Neoadjuvant Therapy Response in Breast Cancer. Radiology 2017;285:358-75. [Crossref] [PubMed]
- Li H, Yao L, Jin P, Hu L, Li X, Guo T, Yang K. MRI and PET/CT for evaluation of the pathological response to neoadjuvant chemotherapy in breast cancer: A systematic review and meta-analysis. Breast 2018;40:106-15. [Crossref] [PubMed]
- Schaefgen B, Mati M, Sinn HP, Golatta M, Stieber A, Rauch G, Hennigs A, Richter H, Domschke C, Schuetz F, Sohn C, Schneeweiss A, Heil J. Can Routine Imaging After Neoadjuvant Chemotherapy in Breast Cancer Predict Pathologic Complete Response? Ann Surg Oncol 2016;23:789-95. [Crossref] [PubMed]
- Morrow M, Waters J, Morris E. MRI for breast cancer screening, diagnosis, and treatment. Lancet 2011;378:1804-11. [Crossref] [PubMed]
- Croshaw R, Shapiro-Wright H, Svensson E, Erb K, Julian T. Accuracy of clinical examination, digital mammogram, ultrasound, and MRI in determining postneoadjuvant pathologic tumor response in operable breast cancer patients. Ann Surg Oncol 2011;18:3160-3. [Crossref] [PubMed]
- Prevos R, Smidt ML, Tjan-Heijnen VC, van Goethem M, Beets-Tan RG, Wildberger JE, Lobbes MB. Pre-treatment differences and early response monitoring of neoadjuvant chemotherapy in breast cancer patients using magnetic resonance imaging: a systematic review. Eur Radiol 2012;22:2607-16. [Crossref] [PubMed]
- Marinovich ML, Sardanelli F, Ciatto S, Mamounas E, Brennan M, Macaskill P, Irwig L, von Minckwitz G, Houssami N. Early prediction of pathologic response to neoadjuvant therapy in breast cancer: systematic review of the accuracy of MRI. Breast 2012;21:669-77. [Crossref] [PubMed]
- Turnbull LW. Dynamic contrast-enhanced MRI in the diagnosis and management of breast cancer. NMR Biomed 2009;22:28-39. [Crossref] [PubMed]
- Lobbes MB, Prevos R, Smidt M, Tjan-Heijnen VC, van Goethem M, Schipper R, Beets-Tan RG, Wildberger JE. The role of magnetic resonance imaging in assessing residual disease and pathologic complete response in breast cancer patients receiving neoadjuvant chemotherapy: a systematic review. Insights Imaging 2013;4:163-75. [Crossref] [PubMed]
- Mukhtar RA, Yau C, Rosen M, Tandon VJ, Hylton N, Esserman LJ. Clinically meaningful tumor reduction rates vary by prechemotherapy MRI phenotype and tumor subtype in the I-SPY 1 TRIAL (CALGB 150007/150012; ACRIN 6657). Ann Surg Oncol 2013;20:3823-30. [Crossref] [PubMed]
- Loibl S, Volz C, Mau C, Blohmer JU, Costa SD, Eidtmann H, et al. Response and prognosis after neoadjuvant chemotherapy in 1,051 patients with infiltrating lobular breast carcinoma. Breast Cancer Res Treat 2014;144:153-62. [Crossref] [PubMed]
- Wang Y, Zhang C, Liu J, Huang G. Is 18F-FDG PET accurate to predict neoadjuvant therapy response in breast cancer? A meta-analysis. Breast Cancer Res Treat 2012;131:357-69. [Crossref] [PubMed]
- Zhuang X, Chen C, Liu Z, Zhang L, Zhou X, Cheng M, Ji F, Zhu T, Lei C, Zhang J, Jiang J, Tian J, Wang K. Multiparametric MRI-based radiomics analysis for the prediction of breast tumor regression patterns after neoadjuvant chemotherapy. Transl Oncol 2020;13:100831. [Crossref] [PubMed]
- Kuerer HM, Vrancken Peeters MTFD, Rea DW, Basik M, De Los Santos J, Heil J. Nonoperative Management for Invasive Breast Cancer After Neoadjuvant Systemic Therapy: Conceptual Basis and Fundamental International Feasibility Clinical Trials. Ann Surg Oncol 2017;24:2855-62. [Crossref] [PubMed]
- Yates LR. Intratumoral heterogeneity and subclonal diversification of early breast cancer. Breast 2017;34:S36-42. [Crossref] [PubMed]
- Bownes RJ, Turnbull AK, Martinez-Perez C, Cameron DA, Sims AH, Oikonomidou O. On-treatment biomarkers can improve prediction of response to neoadjuvant chemotherapy in breast cancer. Breast Cancer Res 2019;21:73. [Crossref] [PubMed]
- Denkert C, von Minckwitz G, Darb-Esfahani S, Lederer B, Heppner BI, Weber KE, et al. Tumour-infiltrating lymphocytes and prognosis in different subtypes of breast cancer: a pooled analysis of 3771 patients treated with neoadjuvant therapy. Lancet Oncol 2018;19:40-50. [Crossref] [PubMed]
- Datta J, Berk E, Xu S, Fitzpatrick E, Rosemblit C, Lowenfeld L, Goodman N, Lewis DA, Zhang PJ, Fisher C, Roses RE, DeMichele A, Czerniecki BJ. Anti-HER2 CD4(+) T-helper type 1 response is a novel immune correlate to pathologic response following neoadjuvant therapy in HER2-positive breast cancer. Breast Cancer Res 2015;17:71. [Crossref] [PubMed]
- Lennartz S, Le Blanc M, Zopfs D, Große Hokamp N, Abdullayev N, Laukamp KR, Haneder S, Borggrefe J, Maintz D, Persigehl T. Dual-Energy CT-derived Iodine Maps: Use in Assessing Pleural Carcinomatosis. Radiology 2019;290:796-804. [Crossref] [PubMed]
- De Cecco CN, Darnell A, Rengo M, Muscogiuri G, Bellini D, Ayuso C, Laghi A. Dual-energy CT: oncologic applications. AJR Am J Roentgenol 2012;199:S98-S105. [Crossref] [PubMed]
- Chae EJ, Song JW, Seo JB, Krauss B, Jang YM, Song KS. Clinical utility of dual-energy CT in the evaluation of solitary pulmonary nodules: initial experience. Radiology 2008;249:671-81. [Crossref] [PubMed]
- Zopfs D, Große Hokamp N, Reimer R, Bratke G, Maintz D, Bruns C, Mallmann C, Persigehl T, Haneder S, Lennartz S. Value of spectral detector CT for pretherapeutic, locoregional assessment of esophageal cancer. Eur J Radiol 2021;134:109423. [Crossref] [PubMed]
- Demirler Şimşir B, Krug KB, Burke C, Hellmich M, Maintz D, Coche E. Possibility to discriminate benign from malignant breast lesions detected on dual-layer spectral CT-evaluation. Eur J Radiol 2021;142:109832. [Crossref] [PubMed]
- Wang X, Liu D, Zeng X, Jiang S, Li L, Yu T, Zhang J. Dual-energy CT quantitative parameters for the differentiation of benign from malignant lesions and the prediction of histopathological and molecular subtypes in breast cancer. Quant Imaging Med Surg 2021;11:1946-57. [Crossref] [PubMed]
- CHEN L. FU J, CHENG Y, LAN Y. The value of dual-layer detector spectral CT in evaluation of immunohistochemical expression in breast cancer patients. Chinese Journal of Radiology 2021;1270-6.
- Deniffel D, Sauter A, Dangelmaier J, Fingerle A, Rummeny EJ, Pfeiffer D. Differentiating intrapulmonary metastases from different primary tumors via quantitative dual-energy CT based iodine concentration and conventional CT attenuation. Eur J Radiol 2019;111:6-13. [Crossref] [PubMed]