A machine learning model incorporating 18F-prostate-specific membrane antigen-1007 positron emission tomography/computed tomography and multiparametric magnetic resonance imaging for predicting prostate-specific antigen persistence in patients with prostate cancer after radical prostatectomy
Introduction
Prostate cancer (PCa) is the second most prevalent solid cancer among males worldwide (1) and one of the leading causes of cancer-related deaths (2). Radical prostatectomy (RP) is currently the primary treatment for patients with PCa, and its indications have become broader as the PCa population continues to evolve, from treating men with low-risk disease to treating men with high-risk, occult metastatic, and definitive metastatic disease (3). However, approximately 10–30% of patients with PCa develop prostate-specific antigen (PSA) persistence after RP (4,5). PSA persistence is strongly associated with residual prostate tumor lesions after surgery, increases the risk of poor prognosis, and is correlated with higher cancer-specific mortality (CSM) (6-8). In the era of precision medicine, the use of clinical and imaging characteristics of patients with PCa allows for the assessment of their postoperative PSA levels and facilitates early detection, which is crucial for personalized treatment and improved prognosis.
Multiparametric magnetic resonance imaging (mpMRI) is one of the primary methods used in the preoperative evaluation of patients with PCa (9). MRI, a noninvasive imaging technique, provides detailed anatomical information about the morphology and structure of prostate tissue (10), which can help to assess the volume and location of lesions, tumor margins, and other features that may be relevant to the short- and long-term prognosis of patients with PCa. Prostate-specific membrane antigen (PSMA) is a protein present in prostate tissue and is overexpressed in PCa cells. PSMA positron emission tomography/computed tomography (PET/CT) is one of the principal noninvasive methods for preoperative evaluation, providing semiquantitative information on the metabolic activity of lesions in patients with PCa. Recent research has investigated the predictive value of mpMRI and PSMA PET/CT in the diagnosis, staging, and prognosis of patients with PCa (11,12). Several retrospective studies have demonstrated the effectiveness of prostate-specific antigen density (PSAD) and the Prostate Imaging-Reporting and Data System (PI-RADS) score from mpMRI in predicting PSA persistence (13,14). Although several studies have assessed the prognostic value of PSMA PET/CT in patients with PCa (11,15,16), the potential benefits of combining PSMA PET/CT with mpMRI parameters in predicting PSA persistence remain unclear. PSMA PET/CT provides vital metabolic and functional insights, whereas mpMRI delivers detailed anatomical and tissue characterization, which can enhance the functional information obtained from PET/CT. We propose that the integration of these two imaging modalities may yield a more comprehensive evaluation of tumor biology, thereby improving the accuracy of prognostic predictions.
In this study, our objective was to evaluate the value of mpMRI and PSMA PET/CT for predicting PSA persistence in patients with PCa after RP and to develop and validate a novel preoperative risk stratification tool based on clinical data, mpMRI, and PSMA PET/CT parameters to predict the probability of PSA persistence. This predictive model may help facilitate timely intervention and optimize personalized patient care. We present this article in accordance with the TRIPOD reporting checklist (available at https://qims.amegroups.com/article/view/10.21037/qims-24-1149/rc).
Methods
Patient population
We conducted a retrospective study of 3487 patients who underwent PSMA PET/CT at the First Affiliated Hospital of Wenzhou Medical University between April 2019 and June 2022. The inclusion criteria were as follows: (I) radical PCa surgery, (II) pathology suggestive of PCa, and (III) both PSMA PET/CT and multiparametric MRI (mpMRI) conducted prior to RP. Meanwhile, the exclusion criteria were as follows: (I) incomplete clinical records or missing PSMA PET/CT or mpMRI images, (II) adjuvant therapy administered before the imaging examination or surgery, (III) no PSA testing within 2 months prior to surgery, and (IV) administration of RP with mpMRI and PSMA PET/CT for more than 1 month. Based on these inclusion and exclusion criteria, 190 male patients were ultimately eligible (Figure 1). This study was conducted in accordance with the Declaration of Helsinki (as revised in 2013) and was approved by the Ethics Committee of the First Affiliated Hospital of Wenzhou Medical University (approval No. KY2022-R012). The requirement for individual consent was waived due to the retrospective nature of the analysis.
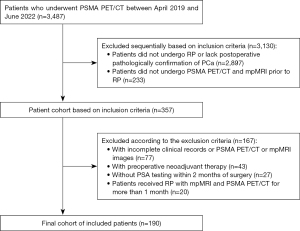
mpMRI and image evaluation
Magnetic resonance scan images were used for analysis. MRI was performed with 3-T MR scanners (Signa HD, GE HealthCare, Chicago, IL, USA; Achieva, Philips Healthcare, Best, The Netherlands). MRI included transverse, sagittal, and coronal T2-weighted imaging and dynamic contrast-enhanced imaging. The dose of Gd-DTPA contrast agent used was 0.2 mmol per kilogram of body weight (BW). The dose was administered intravenously at a rate of 2.0 mL/s with a high-pressure syringe. The detailed scanning parameters are presented in Appendix 1 and Table S1. MR images were scored and reported according to PI-RADS version 2.0 (17). In our study, all patients had a PI-RADS scores greater than 1. To better conduct risk stratification in patients with persistent PSA status, we regrouped the PI-RADS scores into scores of 2–3 and 4–5. The prostate volume (PV) was calculated by measuring the three diameters of the prostate on T2-weighted images. The PV was calculated as follows: PV (cm3) = 0.52 × length (cm) × width (cm) × height (cm). The PSAD was calculated as the total PSA divided by the PV. Abnormal contrast enhancement in or along the seminal vesicles, disappearance of the angle between the base and the seminal vesicles, etc. were defined as seminal vesicle invasion (SVI). Extracapsular extension (EPE) was defined as neurovascular bundle thickening, basalization, augmentation, capsular absence, capsular enhancement, or measurable extracapsular lesion area detected on T2-weighted images (18). All mpMRI findings were evaluated and judged by two experienced radiologists. If their assessments of the mpMRI images were inconsistent, a decision was made through consultation.
18F-PSMA-1007 PET/CT and image evaluation
The radioligand used for PET scanning was 18F-PSMA-1007 (median activity: 282.7 MBq; range: 170.2–366.3 MBq). One hour before the scan, all enrolled patients were injected intravenously with 4.0 MBq/kg of 18F-PSMA-1007 via syringe. Images were acquired with a dedicated PET/CT scanner (Gemini TF 64, Philips Healthcare). A low-dose CT scan was performed from the base of the skull to the midthigh. A low-dose unenhanced CT scan was performed from the skull base to the middle of the thigh under the following parameters: a tube voltage of 140 Kvp, a tube current of 110 mA, a detector collimation of 64×0.625 mm, a pitch of 0.829, a tube rotation speed of 0.5 s, a section thickness of 5 mm, and a reconstruction thickness of 2.5 mm. This was followed by the PET scan that matched the CT section thickness. Images were acquired in 3D mode under the following parameters: field of view, 576 mm; matrix size, 144×144; slice thickness and interval, and 5 mm. The emission scan time per bed position was 1.5 minutes, with a 50% overlap between adjacent bed positions. Moreover, PET images with CT attenuation correction were reconstructed using the time-of-flight algorithm.
All PSMA PET scans were evaluated by one experienced nuclear medicine physician. In the evaluation of PCa lesions, an area with uptake higher than that of the surrounding prostate background activity was considered to be a PCa lesion (19,20). Standardized uptake values (SUVs) were normalized and corrected using BW. Automated criteria outlining the area of the prostate with the most intense uptake determined the maximum SUV (SUVmax) of the primary tumor. For patients with low uptake PCa and patients with multiple foci, the primary tumor was evaluated based on fused PET and CT images. Total lesion PSMA (PSMA-TL) was defined as the sum of metabolic activities in the tumor region and was the product of the mean SUV and the tumor volume. The regions of interest (ROIs) were automatically outlined at thresholds of 40% SUV. For images in which the automatically outlined area did not match the actual image, the PET/CT diagnostician made manual adjustments to determine the ROI based on the visual readings.
Data collection and follow-up
Patient demographics including age, preoperative PSA, mpMRI imaging parameters such as PI-RADS score, PSAD, lesion location [peripheral zones (PZ) and transition zone (TZ)], EPE, SVI, and PSMA PET/CT semiquantitative imaging parameters (SUVmax and PSMA-TL), were collected. The time interval between the preoperative PSA blood test and imaging assessment was required to not exceed 4 weeks.
Preoperative PSA values were defined as the peripheral blood of the patient drawn 1 to 4 weeks prior to RP for the testing of PSA value concentration. According to Mazzone et al., PSA levels typically fall below 0.1 ng/mL within 1 month following RP (14). In our study, PSA persistence was defined as the presence of PSA levels >0.1 ng/mL in the initial postoperative test.
Statistical analysis
Descriptive statistics are used to express patient cohort characteristics. All continuous variables in our data were not normally distributed and are expressed as the median and interquartile range (IQR), while categorical variables are expressed as frequencies and percentages. The Spearman correlation coefficient was calculated to measure the correlation between PSAD, SUVmax, PSMA-TL, and preoperative PSA. We used the Mann-Whitney test for continuous variables to compare the characteristics of PSA persistence and non-PSA persistence. The chi-square test was used to compare the statistical significance of differences in the distribution of categorical data between the two groups.
Variables were selected according to clinical relevance. The associations between PSA persistence and the preoperative predictors were evaluated with univariable logistic regression models. Significant findings from univariate analysis were then incorporated into multivariate logistic regression analysis.
The Youden index was used as a cutoff value for continuous variables. Risk stratification for predicting PSA persistence was established using decision tree analysis, a machine-learning technique widely used in data mining and classification tasks. The independent predictors obtained from the multivariate logistic regression were incorporated into the decision tree analysis. Decision tree analysis identified three different patient risk groups. The predictive accuracy of this risk stratification for PSA persistence after RP in patients with PCa was assessed via the area under the curve (AUC) derived from the receiver operating characteristic (ROC). Segmentation, preprocessing, and machine-learning methods were optimized using repeated five fold cross-validation on the training dataset. Decision curve analysis (DCA) estimated the net benefits of the novel risk stratification model.
All analyses were performed using SPSS version 27.0.1 (IBM Corp., Armonk, NY, USA) and R software version 4.3.3 (The R Project for Statistical Computing; www.r-project.org). The plotting software used was GraphPad Prism 8 (GraphPad Software, Boston, MA, USA). Two-sided P values <0.05 indicated statistically significant differences.
Results
Baseline patient characteristics
The baseline characteristics of the 190 patients are listed in Table 1. The median age and preoperative PSA were 69 (IQR 64–73) years and 10.6 (IQR 6.5–18.2) ng/mL, respectively. Overall, SVI was detected in 31 (16%) patients. The median PSAD was 0.30 (IQR 0.17–0.59) ng/mL2. In addition, 39 (21%) and 151 (79%) patients had maximum PI-RADS scores of 2–3 and 4–5, respectively. The median SUVmax and PSMA-TL were 11.83 (IQR 7.44–20.89) and 41.92 (IQR 21.25–113.83) cm3, respectively.
Table 1
Characteristic | Non-PSA persistence (n=155) | PSA persistence (n=35) | P value |
---|---|---|---|
Age (years) | 67 [64–72] | 71 [66–75] | 0.020 |
PSA (ng/mL) | 15.53 [5.76–14.79] | 29.60 [15.46–41.29] | 0.038 |
PSA density (ng/mL2) | 0.45 [0.15–0.48] | 0.78 [0.37–1.05] | <0.001 |
PI-RADS score | 0.004 | ||
2–3 | 38 [25] | 1 [3] | |
4–5 | 117 [75] | 34 [97] | |
EPE | 0.013 | ||
No | 66 [43] | 7 [20] | |
Yes | 89 [57] | 28 [80] | |
SVI | <0.001 | ||
No | 137 [88] | 22 [63] | |
Yes | 18 [12] | 13 [37] | |
PZ | 0.080 | ||
No | 74 [48] | 11 [31] | |
Yes | 81 [52] | 24 [69] | |
TZ | 0.610 | ||
No | 68 [44] | 17 [49] | |
Yes | 87 [56] | 18 [51] | |
SUVmax | 15.69 [7.06–20.85] | 18.09 [8.33–23.08] | 0.103 |
PSMA-TL (cm3) | 72.35 [18.37–69.77] | 197.74 [71.62–202.81] | <0.001 |
Data are presented as n [%] of median [IQR]. PSA, prostate-specific antigen; PI-RADS, Prostate Imaging Reporting and Data System; EPE, extracapsular extension; SVI, seminal vesicle invasion; PZ, peripheral zones; TZ, transition zone; SUVmax, maximum standardized uptake value; PSMA-TL, total lesion prostate-specific membrane antigen; IQR, interquartile range.
The PZ and TZ on mpMRI and the SUVmax on PSMA PET/CT did not differ significantly between the PSA persistence and non-PSA persistence groups (all P values >0.05; Table 1); in contrast, age, preoperative PSA, PSAD, maximum PI-RADS score, EPE, SVI, and PSMA-TL were significantly different between these groups (all P values <0.05; Table 1).
Correlation of mpMRI semiquantitative parameters and 18F-PSMA-1007 PET/CT with preoperative PSA
Scatter plots for the correlation of preoperative PSA with the semiquantitative parameters of mpMRI and 18F-PSMA-1007 PET/CT suggested a linear trend and positive correlation of preoperative PSA with PSAD, SUVmax, and PSMA-TL (Figure 2). Nonparametric Spearman correlation analysis suggested that the preoperative PSA in patients with PCa was slightly correlated with SUVmax (r=0.41; P<0.001), significantly correlated with PSMA-TL (r=0.58; P<0.001), and strongly correlated with PSAD (r=0.865; P<0.001).
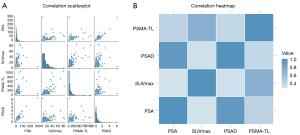
The results of univariate and multivariate logistic regression analyses
Univariate logistic regression analyses indicated that age, PSAD, maximum PI-RADS score, EPE, SVI and PSMA-TL were associated with PSA persistence in postoperative patients with PCa (all P values <0.05; Table 2). Multivariate logistic regression analyses of the above variables indicated that age, PSAD, maximal PI-RADS score, and EPE were not independent predictors of PSA persistence (all P values >0.05; Table 2). In contrast, PSA persistence in patients with PCa after RP could be predicted by SVI [95% confidence interval (CI): 1.017–6.871] and PSMA-TL (95% CI: 1.000–1.005) (all P values <0.05). The AUCs of SVI and PSMA-TL were 0.63 (95% CI: 0.516–0.739) and 0.80 (95% CI: 0.723–0.877), respectively. The cutoff value of PSMA-TL was 63.38 cm3.
Table 2
Variables | Univariate analysis | Multivariate analysis | |||
---|---|---|---|---|---|
OR (95% CI) | P | OR (95% CI) | P | ||
Age (years) | 1.072 (1.009–1.138) | 0.024 | 1.035 (0.970–1.105) | 0.297 | |
PSA density (ng/mL2) | 1.787 (1.062–3.007) | 0.029 | 1.220 (0.725–2.053) | 0.455 | |
PI-RADS score | |||||
2–3 | Reference | Reference | |||
4–5 | 11.043 (1.462–83.408) | 0.020 | 5.682 (0.685–47.140) | 0.108 | |
EPE | |||||
No | Reference | Reference | |||
Yes | 2.966 (1.221–7.204) | 0.016 | 0.984 (0.342–2.830) | 0.977 | |
SVI | |||||
No | Reference | Reference | |||
Yes | 4.497 (1.935–10.455) | <0.001 | 2.643 (1.017–6.871) | 0.046 | |
PZ | |||||
No | Reference | ||||
Yes | 1.993 (0.914–4.349) | 0.083 | |||
TZ | |||||
No | Reference | ||||
Yes | 0.828 (0.397–1.726) | 0.614 | |||
SUVmax | 1.014 (0.988–1.040) | 0.289 | |||
PSMA-TL (cm3) | 1.004 (1.002–1.007) | <0.001 | 1.003 (1.000–1.005) | 0.037 |
mpMRI, multiparametric magnetic resonance imaging; 18F-PSMA-1007 PET/CT, 18F-prostate-specific membrane antigen-1007 positron emission tomography/computed tomography; PSA, density prostate-specific antigen density; OR, odds ratio; CI, confidence interval; PI-RADS, Prostate Imaging Reporting and Data System; EPE, extracapsular extension; SVI, seminal vesicle invasion; PZ, peripheral zones; TZ, transition zone; SUVmax, maximum standardized uptake value; PSMA-TL, total lesion prostate-specific membrane antigen.
Novel risk stratification
Based on the results of the multivariate logistic regression model and clinical relevance, SVI and PSMA-TL were included as variables in the decision tree model. Decision tree analysis categorized patients into low-, intermediate- and high-risk groups. Regardless of SVI, low-risk was considered a PSMA-TL value measured on PSMA PET/CT below the cutoff value. For patients with a PSMA-TL value greater than 63.38 cm3, those without SVI were considered to be at intermediate risk, whereas those with SVI were considered to be at high risk (Figure 3). We found that AUC of the risk stratification tool for predicting PSA persistence was 0.93 (95% CI: 0.850–0.930) (Figure 4). Additionally, this novel tool demonstrated an AUC of 0.77 during cross-validation, with repeated five fold cross-validation for segmentation and validation being applied on the training dataset. Moreover, the DCA of the novel risk stratification model showed a significant net benefit of the prediction model (Figure 5). Furthermore, in the risk stratification tool, the PSA persistence rates corresponding to the low-risk, intermediate-risk, and high-risk groups were 5%, 36%, and 48%, respectively (Figure 3). Figure 3 shows the proportion of patients in the three novel decision tree-derived risk groups for whom PSA persistence was correctly identified using the known cutoff values.
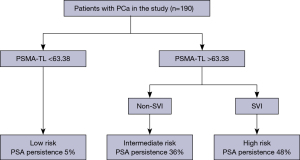
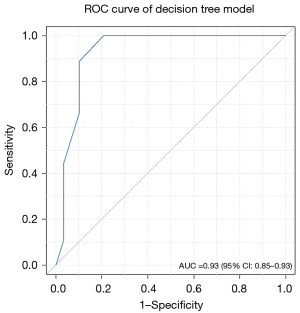
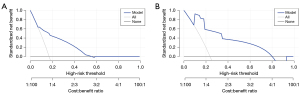
Discussion
Previous studies have shown that mpMRI and PSMA PET/CT each have good performance in predicting PSA persistence after RP (13,21). However, the precise accuracy and predictive efficacy of mpMRI parameters combined with 18F-PSMA-1007 PET/CT semiquantitative parameters for predicting PSA persistence remain uncertain. Therefore, in this study, we established a decision tree model to investigate the joint predictive value of mpMRI and PSMA PET/CT in assessing PSA persistence in a cohort of patients with PCa. By integrating data from both mpMRI and PSMA PET/CT scans, we sought to clarify the potential synergistic effects of these imaging techniques in predicting PSA persistence.
In our study, multivariate logistic regression model revealed that two parameters, SVI on mpMRI and PSMA-TL on PSMA PET/CT, were independent predictors of PSA persistence. PSMA-TL, an imaging biomarker reflecting tumor burden, correlated significantly with the Gleason score and poorer prognosis (16). This could be attributed to PSMA-TL indicating the overall metabolic activity of the tumor, indirectly serving as an indicator for assessing the aggressiveness tumor and the prognosis of patients. Furthermore, a significant relationship was observed among baseline PSMA PET/CT, MRI, clinical parameters, and PSA persistence, thereby further confirming the close association between PSMA PET/CT, mpMRI-related parameters, and serologic findings. PSMA-TL was positively correlated with individual baseline PSA levels, suggesting that PSMA-TL possesses clinical prognostic value. Previous studies have demonstrated that mpMRI is more effective than PSMA PET/CT in detecting SVI (22,23). Building upon this, our research findings indicate that SVI detected on mpMRI serves as a reliable predictor for PSA persistence following RP in patients with PCa. Furthermore, SVI has exhibited promising value for predicting biochemical recurrence in patients undergoing RP for PCa in previous studies (24,25). Our study further confirmed the value of PSMA PET/CT and mpMRI parameters in predicting the short-term prognosis of patients with PCa, and these should be emphasized as useful complementary indicators for these patients, especially those with PCa scheduled for RP.
The AUCs of SVI and PSMA-TL for predicting PSA persistence in patients with PCa receiving RP were 0.63 and 0.80, respectively. It should be mentioned that PSMA-TL demonstrated better predictive value than did SVI (AUC 0.63 vs. 0.80). This may be due to the fact that PSMA-TL may be less affected by surgical intervention, as it primarily reflects the biology of the tumor rather than the anatomic location of the tumor in the body; however, this needs to be confirmed in a study with a larger population. Interestingly, we found that some patients with PCa had lesions with low uptake on 18F-1007-PSMA PET/CT, but PSMA-TL values compensated for this to a certain extent. Paschalis et al. also reported that some patients with PCa have a high degree of inter- or intrafocal heterogeneity and may not express PSMA (26). Meanwhile, PSMA-TL not only involves the degree of tumor uptake but also the metabolic activity of all lesions, thus more accurately reflecting the biological behavior of the tumor. In addition, despite its low AUC, SVI can be combined with other stronger predictors to enhance the predictive power of the overall model. Decision tree models are designed to combine multiple variables for a comprehensive assessment. Therefore, we retained SVI in the decision tree model. We found that PSMA-TL combined with SVI was more capable than either PSMA-TL or SVI alone in predicting PSA persistence after RP (AUC: 0.93 vs. 0.80 and 0.63). This emphasizes the benefit of combined imaging assessments.
We further used decision tree analysis to analyze the PSMA PET/CT and mpMRI parameters and established three-group risk stratification. Risk stratification based on PSMA-TL and SVI could distinguish patients with PCa with different short-term prognoses, providing clinicians the means to conveniently stratify patients for clinical decision-making. Moreover, this new risk stratification tool, with an AUC of 0.77 in the cross-validation analysis, further supports the added value of PSMA PET/CT and mpMRI in patients with PCa. To further evaluate the potential clinical benefits of the prediction model, we conducted DCA, as shown in Figure 5. The novel risk stratification model demonstrated greater net benefits compared to the treat-all or treat-none protocols, emphasizing its clinical utility. Our study showed that only 5% of patients in the low-risk group developed PSA persistence. This finding may imply that patients with PCa who undergo RP have a better prognosis and thus that RP should remain the mainstay of treatment for patients with PCa (3). In contrast, our study found that patients in the high-risk group may be more prone to PSA persistence. This group of patients with PCa may benefit from early intervention; however, further evidence is needed to support this conclusion.
Our study involved certain limitations which should be acknowledged. First, the retrospective nature of our study made it difficult to avoid potential selection bias in determining the patient cohort. Second, there might have been poorly defined borders between the tumor tissue and surrounding fat borders on mpMRI, and mpMRI accuracy could affect the measurement of semiquantitative parameters (27,28). However, our imaging technologists and physicians are from a tertiary hospital and have extensive practical experience in mpMRI and PSMA PET/CT scanning and diagnosis, which could help ensure the accuracy of the image evaluation. Third, our study involved only one center, which may reduce the generalizability of our risk stratification model to other populations. Moreover, although the staging of the patients included in our study met the criteria for RP, variations in staging, such as T stage, may inevitably influence PSA persistence. Therefore, in future research, PSA persistence after RP should be examined with a comprehensive model that integrates imaging, clinical parameters, pathological parameters, and other relevant factors. Finally, although our risk stratification tool showed good calibration, external validation in a larger cohort of patients is needed to further confirm the accuracy of our model.
Conclusions
PSMA PET/CT combined with mpMRI had improved prediction ability for PSA persistence in postoperative patients with PCa. Additionally, a novel risk stratification model was validated, with patients with PSMA-TL levels below the threshold being highly likely not to have PSA persistence, indicating a favorable prognosis. Furthermore, the combined model facilitates both risk stratification and clinical individualization.
Acknowledgments
Funding: None.
Footnote
Reporting Checklist: The authors have completed the TRIPOD reporting checklist. Available at https://qims.amegroups.com/article/view/10.21037/qims-24-1149/rc
Conflicts of Interest: All authors have completed the ICMJE uniform disclosure form (available at https://qims.amegroups.com/article/view/10.21037/qims-24-1149/coif). The authors have no conflicts of interest to declare.
Ethical Statement:
Open Access Statement: This is an Open Access article distributed in accordance with the Creative Commons Attribution-NonCommercial-NoDerivs 4.0 International License (CC BY-NC-ND 4.0), which permits the non-commercial replication and distribution of the article with the strict proviso that no changes or edits are made and the original work is properly cited (including links to both the formal publication through the relevant DOI and the license). See: https://creativecommons.org/licenses/by-nc-nd/4.0/.
References
- Sung H, Ferlay J, Siegel RL, Laversanne M, Soerjomataram I, Jemal A, Bray F. Global Cancer Statistics 2020: GLOBOCAN Estimates of Incidence and Mortality Worldwide for 36 Cancers in 185 Countries. CA Cancer J Clin 2021;71:209-49. [Crossref] [PubMed]
- Adamaki M, Zoumpourlis V. Prostate Cancer Biomarkers: From diagnosis to prognosis and precision-guided therapeutics. Pharmacol Ther 2021;228:107932. [Crossref] [PubMed]
- Costello AJ. Considering the role of radical prostatectomy in 21st century prostate cancer care. Nat Rev Urol 2020;17:177-88. [Crossref] [PubMed]
- Milonas D, Laenen A, Venclovas Z, Jarusevicius L, Devos G, Joniau S. Benefits of early salvage therapy on oncological outcomes in high-risk prostate cancer with persistent PSA after radical prostatectomy. Clin Transl Oncol 2022;24:371-8. [Crossref] [PubMed]
- Wu S, Lin SX, Cornejo KM, Crotty RK, Blute ML, Dahl DM, Wu CL. Clinicopathological and oncological significance of persistent prostate-specific antigen after radical prostatectomy: A systematic review and meta-analysis. Asian J Urol 2023;10:317-28. [Crossref] [PubMed]
- Kimura S, Urabe F, Sasaki H, Kimura T, Miki K, Egawa S. Prognostic Significance of Prostate-Specific Antigen Persistence after Radical Prostatectomy: A Systematic Review and Meta-Analysis. Cancers (Basel) 2021.
- Rogowski P, Trapp C, von Bestenbostel R, Eze C, Ganswindt U, Li M, Unterrainer M, Zacherl MJ, Ilhan H, Beyer L, Kretschmer A, Bartenstein P, Stief C, Belka C, Schmidt-Hegemann NS. Outcome after PSMA-PET/CT-based salvage radiotherapy for nodal recurrence after radical prostatectomy. Eur J Nucl Med Mol Imaging 2022;49:1417-28. [Crossref] [PubMed]
- Shiota M, Takamatsu D, Kimura T, Tashiro K, Matsui Y, Tomida R, et al. Radiotherapy plus androgen deprivation therapy for prostate-specific antigen persistence in lymph node-positive prostate cancer. Cancer Sci 2022;113:2386-96. [Crossref] [PubMed]
- Vilanova JC, Catalá-Sventzetzky V, Hernández-Mancera J. MRI for detection, staging, and follow-up of prostate cancer: Synthesis of the PI-RADS v2.1, MET-RADS, PRECISE, and PI-RR guidelines. Radiologia (Engl Ed) 2023;65:431-46. [Crossref] [PubMed]
- Nikles S, Pezelj I, Tomić M, Knežević M, Vrhovec B, Dumbović L, Pirša M, Kavelj I, Tomašković I. Current role of magnetic resonance imaging in the screening, diagnosis, and treatment of prostate cancer. Acta Clin Croat 2022;61:92-4. [Crossref] [PubMed]
- Pathmanandavel S, Crumbaker M, Nguyen A, Yam AO, Wilson P, Niman R, Ayers M, Sharma S, Eu P, Martin AJ, Stockler MR, Joshua AM, Emmett L. The Prognostic Value of Posttreatment (68)Ga-PSMA-11 PET/CT and (18)F-FDG PET/CT in Metastatic Castration-Resistant Prostate Cancer Treated with (177)Lu-PSMA-617 and NOX66 in a Phase I/II Trial (LuPIN). J Nucl Med 2023;64:69-74. [Crossref] [PubMed]
- Wang Y, Wang W, Yi N, Jiang L, Yin X, Zhou W, Wang L. Detection of intermediate- and high-risk prostate cancer with biparametric magnetic resonance imaging: a systematic review and meta-analysis. Quant Imaging Med Surg 2023;13:2791-806. [Crossref] [PubMed]
- Vives Dilme R, Rivas JG, Fernández Hernández L, De la Parra Sánchez I, Sánchez Del Hoyo R, Galante Romo MI, Redondo González E, Senovilla Pérez JL, Fernández Montarroso L, Moreno Sierra J. Oncological outcomes in robot-assisted radical prostatectomy: the value of PSA density as a preoperative predictive factor. Ther Adv Urol 2024;16:17562872241229250. [Crossref] [PubMed]
- Mazzone E, Gandaglia G, Robesti D, Rajwa P, Gomez Rivas J, Ibáñez L, et al. Which Patients with Prostate Cancer and Lymph Node Uptake at Preoperative Prostate-specific Membrane Antigen Positron Emission Tomography/Computerized Tomography Scan Are at a Higher Risk of Prostate-specific Antigen Persistence After Radical Prostatectomy? Identifying Indicators of Systemic Disease by Integrating Clinical, Magnetic Resonance Imaging, and Functional Imaging Parameters. Eur Urol Oncol 2024;7:231-40. [Crossref] [PubMed]
- Oruç Z, Güzel Y, Ebinç S, Kömek H, Küçüköner M, Kaplan MA, Oruç İ, Urakçi Z, Işikdoğan A. Efficacy of 68Ga-PSMA PET/CT-derived whole-body volumetric parameters in predicting response to second-generation androgen receptor axis-targeted therapy, and the prognosis in metastatic hormone-refractory prostate cancer patients. Nucl Med Commun 2021;42:1336-46. [Crossref] [PubMed]
- Zou Q, Jiao J, Zou MH, Li MZ, Yang T, Xu L, Zhang Y. Semi-automatic evaluation of baseline whole-body tumor burden as an imaging biomarker of (68)Ga-PSMA-11 PET/CT in newly diagnosed prostate cancer. Abdom Radiol (NY) 2020;45:4202-13. [Crossref] [PubMed]
- Weinreb JC, Barentsz JO, Choyke PL, Cornud F, Haider MA, Macura KJ, Margolis D, Schnall MD, Shtern F, Tempany CM, Thoeny HC, Verma S. PI-RADS Prostate Imaging - Reporting and Data System: 2015, Version 2. Eur Urol 2016;69:16-40. [Crossref] [PubMed]
- Mazzone E, Gandaglia G, Ploussard G, Marra G, Valerio M, Campi R, et al. Risk Stratification of Patients Candidate to Radical Prostatectomy Based on Clinical and Multiparametric Magnetic Resonance Imaging Parameters: Development and External Validation of Novel Risk Groups. Eur Urol 2022;81:193-203. [Crossref] [PubMed]
- Krak NC, Boellaard R, Hoekstra OS, Twisk JW, Hoekstra CJ, Lammertsma AA. Effects of ROI definition and reconstruction method on quantitative outcome and applicability in a response monitoring trial. Eur J Nucl Med Mol Imaging 2005;32:294-301. [Crossref] [PubMed]
- Weber WA, Gatsonis CA, Mozley PD, Hanna LG, Shields AF, Aberle DR, Govindan R, Torigian DA, Karp JS, Yu JQ, Subramaniam RM, Halvorsen RA, Siegel BA. ACRIN 6678 Research team; MK-0646-008 Research team. Repeatability of 18F-FDG PET/CT in Advanced Non-Small Cell Lung Cancer: Prospective Assessment in 2 Multicenter Trials. J Nucl Med 2015;56:1137-43. [Crossref] [PubMed]
- Cassin J, Walker PM, Blanc J, Asuncion A, Bardet F, Cormier L, Loffroy R, Cochet A. Role of magnetic resonance imaging for preoperative prediction of early biochemical failure in localized prostate cancer. Quant Imaging Med Surg 2023;13:1440-52. [Crossref] [PubMed]
- Sonni I, Felker ER, Lenis AT, Sisk AE, Bahri S, Allen-Auerbach M, Armstrong WR, Suvannarerg V, Tubtawee T, Grogan T, Elashoff D, Eiber M, Raman SS, Czernin J, Reiter RE, Calais J. Head-to-Head Comparison of (68)Ga-PSMA-11 PET/CT and mpMRI with a Histopathology Gold Standard in the Detection, Intraprostatic Localization, and Determination of Local Extension of Primary Prostate Cancer: Results from a Prospective Single-Center Imaging Trial. J Nucl Med 2022;63:847-54. [Crossref] [PubMed]
- Mookerji N, Pfanner T, Hui A, Huang G, Albers P, Mittal R, et al. Fluorine-18 Prostate-Specific Membrane Antigen-1007 PET/CT vs Multiparametric MRI for Locoregional Staging of Prostate Cancer. JAMA Oncol 2024;10:1097-103. [Crossref] [PubMed]
- Fukunaga A, Maejima A, Shinoda Y, Matsui Y, Komiyama M, Fujimoto H, Miyagi Maeshima A. Prognostic implication of staging of seminal vesicle invasion in patients with prostatic adenocarcinoma after prostatectomy. Int J Urol 2021;28:1039-45. [Crossref] [PubMed]
- Galosi AB, Milanese G, Montesi L, Cimadamore A, Franzese C, Palagonia E, Chiacchio G, Castellani D. The pathway of isolated seminal vesicle invasion has a different impact on biochemical recurrence after radical prostatectomy and pelvic lymphadenectomy. Urol Oncol 2023;41:293.e9-293.e14. [Crossref] [PubMed]
- Paschalis A, Sheehan B, Riisnaes R, Rodrigues DN, Gurel B, Bertan C, et al. Prostate-specific Membrane Antigen Heterogeneity and DNA Repair Defects in Prostate Cancer. Eur Urol 2019;76:469-78. [Crossref] [PubMed]
- Brembilla G, Lavalle S, Parry T, Cosenza M, Russo T, Mazzone E, Pellegrino F, Stabile A, Gandaglia G, Briganti A, Montorsi F, Esposito A, De Cobelli F. Impact of prostate imaging quality (PI-QUAL) score on the detection of clinically significant prostate cancer at biopsy. Eur J Radiol 2023;164:110849. [Crossref] [PubMed]
- Pellegrino F, Tin AL, Martini A, Vertosick EA, Porwal SP, Stabile A, Gandaglia G, Eastham JA, Briganti A, Montorsi F, Vickers AJ. Prostate-specific Antigen Density Cutoff of 0.15 ng/ml/cc to Propose Prostate Biopsies to Patients with Negative Magnetic Resonance Imaging: Efficient Threshold or Legacy of the Past? Eur Urol Focus 2023;9:291-7. [Crossref] [PubMed]