Exploration of optimal thresholds for predicting the invasive nature of stage T1 lung adenocarcinoma using artificial intelligence-based 3D solid component volume segmentation
Introduction
Lung adenocarcinoma is the most prevalent subtype of lung cancer, with its early stages categorized into four distinct categories: atypical adenomatous hyperplasia (AAH), adenocarcinoma in situ (AIS), minimally invasive adenocarcinoma (MIA), and invasive adenocarcinoma (IAC). The volume of the solid component is a crucial quantitative parameter widely used for assessing the pathological grading of lung adenocarcinoma. Additionally, the volume doubling time is a commonly used indicator for distinguishing the benign and malignant nature of pulmonary nodules (1,2). However, the current clinical methods for measuring the volume of the solid component and assessing the doubling time are typically based on the measurement of the diameter of the solid component, assuming the singular existence of the solid component and a uniform density distribution of the pulmonary nodule, which limits the accuracy and reproducibility of the measurements.
Computed tomography (CT) threshold segmentation is a method for evaluating the components within a lesion by setting a CT attenuation threshold, offering a more comprehensive assessment of pulmonary nodules and reducing related errors to a certain extent. However, the effectiveness of this method in quantitatively assessing the volume of the solid component of pulmonary nodules depends on the selection of the threshold. Currently, there is no recognized objective standard for predicting the optimal threshold for the invasive grade of lung adenocarcinoma. This study aims to explore the optimal threshold for predicting the invasive grade of T1 lung adenocarcinoma based on artificial intelligence (AI)-assisted threshold segmentation in three-dimensional solid component volume (3D SCV) prediction. We present this article in accordance with the STROBE reporting checklist (available at https://qims.amegroups.com/article/view/10.21037/qims-24-1627/rc).
Methods
Study subjects
In a comprehensive retrospective case–control analysis of patients with pathologically confirmed stage T1 lung adenocarcinoma, a cohort was systematically assembled. The study period spanned from November 2017 to November 2023 at Zhengzhou People’s Hospital and from May 2012 to September 2018 at Huadong Hospital of Fudan University. Consecutive enrollment was conducted, resulting in the inclusion of 820 patients (936 nodules) and 236 patients (243 nodules) from the two centers. The study was conducted in accordance with the Declaration of Helsinki (as revised in 2013). The study was approved by the Institutional Review Boards of The Fifth Clinical Medical College of Henan University of Chinese Medicine (Zhengzhou People’s Hospital) (No. 2024011139) and Huadong Hospital of Fudan University (No. 2019K134), and the requirement for individual consent was waived because it was a retrospective experiment and did not involve patients’ privacy. The imaging data for all included cases were completely collected within 1 month prior to surgery. Chest CT imaging was primarily performed using one of the four CT systems: Somatom Definition, NeuViz 16Classic, GE Discovery CT750 HD, and GE Revolution CT Power. The images of nodules were acquired by multirow (64 rows) spiral CT (at least 16 rows) with a slice thickness of less than or equal to 1.5 mm. Prior to surgery, the patients had not received any form of neoadjuvant therapy. The collected data encompassed the patients’ gender, age, distribution of lung lobes, and thoracic thin-slice CT images (DICOM format, with a slice thickness not exceeding 1.5 mm).
Image post-processing and threshold selection
The collected thin-slice DICOM images were compressed and then imported into the AI workstation of Shukun Technology for quantitative analysis. Through automatic segmentation, identification, and quantification, the 3D SCV of the nodules under different CT thresholds were calculated (unit: cubic millimeters). A total of seven CT thresholds were set in this study: −550, −450, −350, −250, −150, −50, and 0 HU. Accordingly, we obtained seven sets of data, labeled as V−550, V−450, V−350, V−250, V−150, V−50, and V0.
Grouping
Based on the postoperative pathological results, patients diagnosed with IAC were categorized into the invasive group; those diagnosed with AAH, AIS, and MIA were categorized into the non-invasive group. Further subgroup analysis was performed based on the diameter of the pulmonary nodules, dividing the patients into three subgroups: T1a (diameter ≤10 mm), T1b (10 mm < diameter ≤20 mm), and T1c (20 mm < diameter ≤30 mm). The detailed flowchart of the study is shown in Figure 1, and the changes in the solid component of ground-glass nodules in chest CT under different threshold settings are displayed in Figures 2,3.
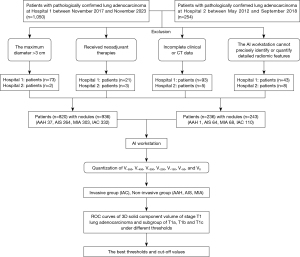
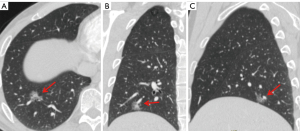
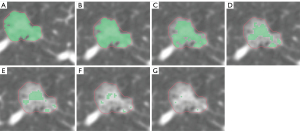
Statistical analysis
Statistical analysis in this study was conducted using SPSS version 23.0. Continuous variables were presented as mean ± standard deviation. Inter-group comparisons for continuous variables were performed using independent samples t-tests. Categorical variables were represented by frequency counts and percentages, with inter-group comparisons made using chi-squared tests. A P value of less than 0.05 was considered to indicate statistical significance. The 3D SCV at different thresholds were used to plot the receiver operating characteristic (ROC) curves, and the predictive performance of these curves, represented by the area under the curve (AUC), was compared to determine the optimal threshold. The cut-off point with the best predictive efficacy at the optimal threshold was also calculated. Furthermore, the nodules were grouped according to their diameter, and statistical analysis was performed on each subgroup.
Results
General characteristics
A total of 1,179 pulmonary nodules were included in this study, with 737 in the non-invasive group, consisting of 38 atypical adenomatous hyperplasias, 328 adenocarcinomas in situ, and 371 minimally invasive adenocarcinomas; the invasive group comprised 442 nodules. The average age of the patients in both groups was 56.67±11.51 years, with statistically significant differences in average age, sex and the maximum diameter of the nodules between the two groups. The solid component volumes obtained at seven different CT thresholds showed significant statistical differences between the two groups (P<0.05), indicating that the solid component volume based on threshold segmentation can stably predict the invasive degree of T1 lung adenocarcinoma. Detailed data are presented in Table 1.
Table 1
Variables | Total (n=1,179) | Non-invasive group (n=737) | Invasive group (n=442) | Statistic | P |
---|---|---|---|---|---|
Age, years | 56.67±11.51 | 53.87±11.83 | 61.36±9.25 | t=−12.10 | <0.001 |
Maximum 3D diameter, mm | 13.22±6.28 | 12.55±5.95 | 14.35±6.63 | t=−4.70 | <0.001 |
V−550, mm3 | 1,101.52±2,685.96 | 239.50±467.69 | 2,538.87±3,948.86 | t=−12.19 | <0.001 |
V−450, mm3 | 938.90±2,599.50 | 143.11±314.50 | 2,265.81±3,881.02 | t=−11.48 | <0.001 |
V−350, mm3 | 816.74±2,513.37 | 81.22±209.79 | 2,043.16±3,793.28 | t=−10.86 | <0.001 |
V−250, mm3 | 710.94±2,408.95 | 51.19±169.73 | 1,811.02±3,675.95 | t=−10.06 | <0.001 |
V−150, mm3 | 604.17±2,272.45 | 29.87±129.57 | 1,561.76±3,506.56 | t=−9.18 | <0.001 |
V−50, mm3 | 482.89±2,053.08 | 16.72±96.18 | 1,260.20±3,205.51 | t=−8.15 | <0.001 |
V0, mm3 | 390.97±1,823.56 | 11.27±70.87 | 1,024.10±2,869.08 | t=−7.42 | <0.001 |
Sex | χ2=7.22 | 0.007 | |||
Male | 392 (33.25) | 224 (30.39) | 168 (38.01) | ||
Female | 787 (66.75) | 513 (69.61) | 274 (61.99) | ||
Lobe | χ2=2.95 | 0.566 | |||
RUL | 400 (33.93) | 246 (33.38) | 154 (34.84) | ||
RML | 83 (7.04) | 54 (7.33) | 29 (6.56) | ||
RLL | 227 (19.25) | 138 (18.72) | 89 (20.14) | ||
LUL | 290 (24.60) | 192 (26.05) | 98 (22.17) | ||
LLL | 179 (15.18) | 107 (14.52) | 72 (16.29) |
Data are presented as mean ± SD or n (%). t: t-test; χ2: Chi-square test. Vx, when the CT threshold is set at x HU, the three-dimensional solid component volume of pulmonary nodules. CT, computed tomography; 3D, three-dimensional; RUL, right upper lobe; RML, right middle lobe; RLL, right lower lobe; LUL, left upper lobe; LLL, left lower lobe; SD, standard deviation.
An analysis of ROC curves
This segment elaborates on the application of AI threshold segmentation to determine the volume of the solid component, which aids in the prediction of invasiveness in T1 stage lung adenocarcinoma. The construction of ROC curves (illustrated in Figure 4) indicated that the threshold setting at −350 HU yielded the best predictive performance. The AUC, along with its 95% confidence interval (CI), was measured to be 0.930 (0.915–0.945). The predictive model was characterized by a cut-off value of 106.5 mm3, a sensitivity of 88.462%, a specificity of 83.853%, an accuracy of 85.581%, and a Youden’s index of 0.72315.
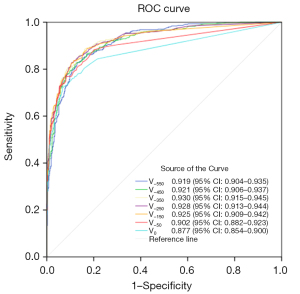
Subgroup analysis
In this study, lung nodules were categorized into three subgroups based on their 3D maximum diameter: the T1a subgroup (507 nodules in total, with 353 in the non-invasive group and 154 in the invasive group), the T1b subgroup (495 nodules in total, with 292 in the non-invasive group and 203 in the invasive group), and the T1c subgroup (177 nodules in total, with 92 in the non-invasive group and 85 in the invasive group). ROC curve analysis revealed that for the T1a subgroup, the optimal predictive performance was achieved at a threshold of −450 HU, with an AUC of 0.926, a cut-off value of 204.5 mm3, a sensitivity of 85.269%, a specificity of 87.013%, an accuracy of 85.799%, and a Youden’s index of 0.72282. For the T1b subgroup, the best threshold was identified at −250 HU, yielding an AUC of 0.922, a cut-off value of 106 mm3, a sensitivity of 89.726%, a specificity of 82.266%, an accuracy of 86.667%, and a Youden’s index of 0.71992. As for the T1c subgroup, the optimal threshold was −350 HU, with an AUC of 0.961, a cut-off value of 472 mm3, a sensitivity of 95.652%, a specificity of 83.529%, an accuracy of 89.831%, and a Youden’s index of 0.79182. The corresponding ROC curves are presented in Figures 5-7, respectively.
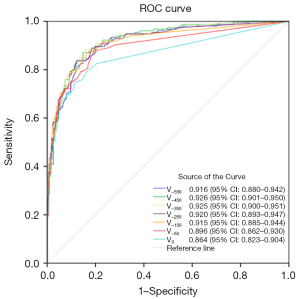
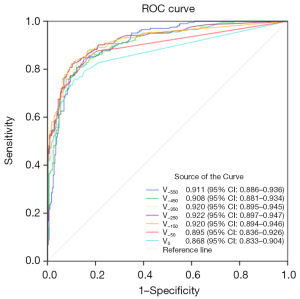
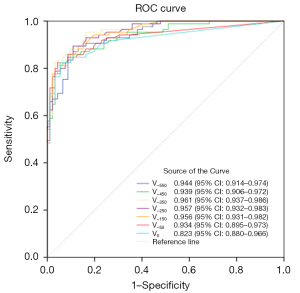
Discussion
The assessment of lung adenocarcinoma’s invasive degree is crucial for clinical diagnosis, with an accuracy comparable to that of intraoperative frozen pathology (3). Such assessments are instrumental in clinical decision-making, treatment planning, and prognostic evaluation. A variety of methods are currently used for predicting the invasive degree of lung adenocarcinoma, including imaging features, quantitative analysis, molecular biological markers, functional imaging techniques, clinical characteristics, volume doubling time, and radiomics (4-9). Among these methods, the calculation of the solid component is particularly critical, as it is often considered a sign of invasive lesions [although in some cases it may represent alveolar collapse (8)] and is significantly correlated with the aggressiveness of the tumor (10-15). The Fleischner Society recommends using mediastinal windows to observe the solid components within nodules (16), while the American College of Radiology (ACR) recommends using lung windows for observation. Existing methods for measuring the solid component include the maximum diameter method, average diameter method, density distribution analysis, manual delineation, threshold segmentation, and computer-aided diagnosis. The maximum diameter method, due to its simplicity, is the most commonly used in clinical practice, but its repeatability is less than ideal due to manual measurement errors and subjectivity. In contrast, threshold segmentation, which automatically segments the solid component using the grayscale threshold of CT images, for example, by setting a CT threshold of −350 HU and considering all areas above this threshold as solid components, appears to be more accurate and repeatable than the simple diameter method, but there is currently no standardized threshold setting.
In the clinical diagnosis of lung cancer, the analysis of CT images of subsolid nodules is an essential method for assessing the invasive degree of lung adenocarcinoma. Zheng et al. (17) retrospectively analyzed the CT images of 102 subsolid nodules and applied the CT threshold segmentation technique to measure the volume of the solid components, further calculating the volume ratio of the solid component to the overall nodule. The study found that when the threshold was set to −250 HU, the diagnostic value for identifying the solid component was the highest (AUC of 0.982), and a threshold of −300 HU also had good diagnostic efficacy (AUC of 0.977). At the thresholds of −250 and −300 HU, there was no significant difference between the median volume of the solid component and the reference standard, indicating that the CT threshold segmentation method can accurately identify and quantify the solid components of subsolid pulmonary nodules, with thresholds set at −250 or −300 HU.
Kawaguchi et al. (18) believe that a threshold of −300 HU has the highest diagnostic value in identifying the solid components within the lesions. Lee et al. (19) retrospectively analyzed the chest thin-section plain CT images of 25 patients with pathologically confirmed MIA, defining the solid component as areas with higher CT values at 50 HU intervals within the range of −600 to −100 HU. The study pointed out that at a threshold of −400 HU, the deviation between the maximum diameter of the solid part of subsolid nodules and the size of the invasive lesion in the pathological specimen was the smallest, thus −400 HU can be considered the optimal threshold for determining that the solid component of subsolid nodules is an invasive lesion.
Liu et al. (20) analyzed the CT images of 326 pathologically confirmed part-solid nodules, setting thresholds at 50 HU intervals from −400 to 50 HU, and found that a threshold of −250 HU in low-dose CT (LDCT) for measuring the volume of the solid component has certain value for predicting its invasive degree and plays an important role in the risk stratification and management of part-solid nodules in lung cancer screening.
Based on the above research, we choose −550 to 0 HU as the interval range for quantifying the volume of pulmonary nodules, which can also be better compared with it. In this study, we identified the optimal threshold for predicting the invasive degree of T1 stage lung adenocarcinoma as −350 HU, with an AUC and 95% CI of 0.930 (0.915–0.945) and a cut-off value of 106.5 mm3. We can consider that in part-solid pulmonary nodules, the components with a CT value greater than −350 HU have a certain degree of invasiveness, so as to better evaluate the invasiveness of early lung adenocarcinoma and facilitate clinical decision-making. Furthermore, the invasive grade of lung adenocarcinoma correlates with the diameter of the pulmonary nodule (21-24). Subgroup analysis based on the diameter of nodules revealed that the optimal thresholds for T1a, T1b, and T1c groups were −450, −250, and −350 HU, respectively. As the diameter of the pulmonary nodule increases, the optimal threshold also shows a noticeable variation. This variation may be related to the fact that nodules in the T1a group are less susceptible to interference from nearby blood vessels in the threshold segmentation method, whereas an increase in the diameter of the pulmonary nodule may lead to blood vessels affecting the identification of the solid component, thereby influencing the threshold assessment. This phenomenon requires further exploration in future studies.
Study limitation
There are certain limitations to this study. Firstly, as a retrospective analysis, there may be biases in case selection. Secondly, differences in CT equipment and scanning parameters across various centers may impact the study results. Additionally, this study did not further analyze the distinction between solid components as alveolar collapse or invasive foci, nor the connection between these solid components and pathological subtypes. An in-depth discussion on the relationship between threshold values and nodule diameter has also not been conducted, and these aspects require further refinement in future research.
Conclusions
In summary, the volume of the solid component measured by the AI-based threshold segmentation method has been proven to be a stable predictive factor for the invasive grade of T1 stage lung adenocarcinoma, demonstrating good predictive efficacy. In the analysis of our case series, the optimal threshold was determined to be −350 HU, with a corresponding cut-off value of 106.5 mm3. Through subgroup analysis, we further identified that the optimal thresholds for the T1a, T1b, and T1c subgroups were −450, −250, and −350 HU, respectively. Therefore, we recommend the following thresholds and cut-off values for predicting the invasiveness of lung adenocarcinoma: for pulmonary nodules with a maximum diameter ≤10 mm, use a threshold of −450 HU and a solid component volume of 204.5 mm3; for nodules with 10 mm < maximum diameter ≤20 mm, use a threshold of −250 HU and a solid component volume of 106 mm3; and for nodules with 20 mm < maximum diameter ≤30 mm, use a threshold of −350 HU and a solid component volume of 472 mm3.
Acknowledgments
Funding: None.
Footnote
Reporting Checklist: The authors have completed the STROBE reporting checklist. Available at https://qims.amegroups.com/article/view/10.21037/qims-24-1627/rc
Conflicts of Interest: All authors have completed the ICMJE uniform disclosure form (available at https://qims.amegroups.com/article/view/10.21037/qims-24-1627/coif). L.Z. is an employee of Shukun (Beijing) Technology Co. The other authors have no conflicts of interest to declare.
Ethical Statement: The authors are accountable for all aspects of the work in ensuring that questions related to the accuracy or integrity of any part of the work are appropriately investigated and resolved. The study was conducted in accordance with the Declaration of Helsinki (as revised in 2013). The study was approved by the Institutional Review Boards of The Fifth Clinical Medical College of Henan University of Chinese Medicine (Zhengzhou People’s Hospital) (No. 2024011139) and Huadong Hospital of Fudan University (No. 2019K134), and the requirement for individual consent was waived because it was a retrospective experiment and did not involve patients’ privacy.
Open Access Statement: This is an Open Access article distributed in accordance with the Creative Commons Attribution-NonCommercial-NoDerivs 4.0 International License (CC BY-NC-ND 4.0), which permits the non-commercial replication and distribution of the article with the strict proviso that no changes or edits are made and the original work is properly cited (including links to both the formal publication through the relevant DOI and the license). See: https://creativecommons.org/licenses/by-nc-nd/4.0/.
References
- Hammer MM, Gupta S, Byrne SC. Volume Doubling Times of Benign and Malignant Nodules in Lung Cancer Screening. Curr Probl Diagn Radiol 2023;52:515-8. [Crossref] [PubMed]
- Ocaña-Tienda B, Eroles-Simó A, Pérez-Beteta J, Arana E, Pérez-García VM. Growth dynamics of lung nodules: implications for classification in lung cancer screening. Cancer Imaging 2024;24:113. [Crossref] [PubMed]
- Wang H, Chen A, Wang K, Yang H, Wen W, Ren Q, Chen L, Xu X, Zhu Q. CT imaging features of lung ground-glass nodule patients with upgraded intraoperative frozen pathology. Discov Oncol 2024;15:29. [Crossref] [PubMed]
- Li C, Jin Y, Deng Q, Yang Y, Duan R, Zhong J, Pan A, Gao M, Xu Z. Development and validation of a nomogram based on CT texture analysis for discriminating minimally invasive adenocarcinoma from glandular precursor lesions in sub centimeter pulmonary ground glass nodules. Oncol Lett 2024;27:26. [Crossref] [PubMed]
- Zhu H, Zhang L, Huang Z, Chen J, Sun L, Chen Y, Huang G, Chen Q, Yu H. Lung adenocarcinoma associated with cystic airspaces: Predictive value of CT features in assessing pathologic invasiveness. Eur J Radiol 2023;165:110947. [Crossref] [PubMed]
- Qiu ZB, Zhang C, Chu XP, Cai FY, Yang XN, Wu YL, Zhong WZ. Quantifying invasiveness of clinical stage IA lung adenocarcinoma with computed tomography texture features. J Thorac Cardiovasc Surg 2022;163:805-815.e3. [Crossref] [PubMed]
- Wu YJ, Liu YC, Liao CY, Tang EK, Wu FZ. A comparative study to evaluate CT-based semantic and radiomic features in preoperative diagnosis of invasive pulmonary adenocarcinomas manifesting as subsolid nodules. Sci Rep 2021;11:66. [Crossref] [PubMed]
- Ambrosi F, Lissenberg-Witte B, Comans E, Sprengers R, Dickhoff C, Bahce I, Radonic T, Thunnissen E. Tumor Atelectasis Gives Rise to a Solid Appearance in Pulmonary Adenocarcinomas on High-Resolution Computed Tomography. JTO Clin Res Rep 2020;1:100018. [Crossref] [PubMed]
- Larici AR, Farchione A, Franchi P, Ciliberto M, Cicchetti G, Calandriello L, Del Ciello A, Bonomo L. Lung nodules: size still matters. Eur Respir Rev 2017;26:170025. [Crossref] [PubMed]
- Li WJ, Chu ZG, Li D, Jing WW, Shi QL, Lv FJ. Accuracy of solid portion size measured on multiplanar volume rendering images for assessing invasiveness in lung adenocarcinoma manifesting as subsolid nodules. Quant Imaging Med Surg 2024;14:1971-84. [Crossref] [PubMed]
- Mizuno T, Terada Y, Katsumata S, Konno H, Nagata T, Isaka M, Ohde Y. Diagnostic sensitivity of solid volume measurement for pathological invasion in non-solid lung adenocarcinoma. J Thorac Dis 2023;15:2916-25. [Crossref] [PubMed]
- Li M, Zhu L, Lv Y, Shen L, Han Y, Ye B. Thin-slice computed tomography enables to classify pulmonary subsolid nodules into pre-invasive lesion/minimally invasive adenocarcinoma and invasive adenocarcinoma: a retrospective study. Sci Rep 2023;13:6999. [Crossref] [PubMed]
- Kato T, Iwano S, Hanamatsu Y, Nakaguro M, Emoto R, Okado S, Sato K, Noritake O, Nakanishi K, Kadomatsu Y, Ueno H, Ozeki N, Nakamura S, Fukumoto K, Takeuchi T, Karube K, Matsui S, Chen-Yoshikawa TF. Prognostic impact of highly solid component in early-stage solid lung adenocarcinoma. Quant Imaging Med Surg 2023;13:5641-52. [Crossref] [PubMed]
- Hayashi H, Ashizawa K, Ogihara Y, Nishida A, Matsumoto K, Yamasaki N, Nagayasu T, Fukuda M, Honda S, Uetani M. Comparison between solid component size on thin-section CT and pathologic lymph node metastasis and local invasion in T1 lung adenocarcinoma. Jpn J Radiol 2017;35:109-15. [Crossref] [PubMed]
- Lee KH, Goo JM, Park SJ, Wi JY, Chung DH, Go H, Park HS, Park CM, Lee SM. Correlation between the size of the solid component on thin-section CT and the invasive component on pathology in small lung adenocarcinomas manifesting as ground-glass nodules. J Thorac Oncol 2014;9:74-82. [Crossref] [PubMed]
- MacMahon H, Naidich DP, Goo JM, Lee KS, Leung ANC, Mayo JR, Mehta AC, Ohno Y, Powell CA, Prokop M, Rubin GD, Schaefer-Prokop CM, Travis WD, Van Schil PE, Bankier AA. Guidelines for Management of Incidental Pulmonary Nodules Detected on CT Images: From the Fleischner Society 2017. Radiology 2017;284:228-43. [Crossref] [PubMed]
- Zheng W, Wang Q, Wang Y, Guo F, Wang X, Yu T. Threshold Segmentation of Pulmonary Subsolid Nodules on CT Images: Detection and Quantification of the Solid Component. Zhongguo Fei Ai Za Zhi 2017;20:341-5. [Crossref] [PubMed]
- Kawaguchi Y, Nakao M, Omura K, Iwamoto N, Ozawa H, Kondo Y, Ichinose J, Matsuura Y, Okumura S, Mun M. The utility of three-dimensional computed tomography for prediction of tumor invasiveness in clinical stage IA lung adenocarcinoma. J Thorac Dis 2020;12:7218-26. [Crossref] [PubMed]
- Lee S, Lee DH, Lee JH, Lee S, Han K, Park CH, Kim TH. Semi-Quantitative Analysis for Determining the Optimal Threshold Value on CT to Measure the Solid Portion of Pulmonary Subsolid Nodules. Taehan Yongsang Uihakhoe Chi 2021;82:670-81. [Crossref] [PubMed]
- Liu J, Xie C, Li Y, Xu H, He C, Qing H, Zhou P. The solid component within part-solid nodules: 3-dimensional quantification, correlation with the malignant grade of nonmucinous pulmonary adenocarcinomas, and comparisons with 2-dimentional measures and semantic features in low-dose computed tomography. Cancer Imaging 2023;23:65. [Crossref] [PubMed]
- Yang Y, Xu J, Wang W, Ma M, Huang Q, Zhou C, Zhao J, Duan Y, Luo J, Jiang J, Ye L. A nomogram based on the quantitative and qualitative features of CT imaging for the prediction of the invasiveness of ground glass nodules in lung adenocarcinoma. BMC Cancer 2024;24:438. [Crossref] [PubMed]
- Liu J, Yang X, Li Y, Xu H, He C, Zhou P, Qing H. Predicting the Invasiveness of Pulmonary Adenocarcinomas in Pure Ground-Glass Nodules Using the Nodule Diameter: A Systematic Review, Meta-Analysis, and Validation in an Independent Cohort. Diagnostics (Basel) 2024;14:147. [Crossref] [PubMed]
- Li X, Zhang W, Yu Y, Zhang G, Zhou L, Wu Z, Liu B. CT features and quantitative analysis of subsolid nodule lung adenocarcinoma for pathological classification prediction. BMC Cancer 2020;20:60. [Crossref] [PubMed]
- Han L, Zhang P, Wang Y, Gao Z, Wang H, Li X, Ye Z. CT quantitative parameters to predict the invasiveness of lung pure ground-glass nodules (pGGNs). Clin Radiol 2018;73:504.e1-7. [Crossref] [PubMed]