Reproducibility of proton density fat fraction assessment of thigh muscle in a multi-site, multi-vendor cohort study at 10 years after anterior cruciate ligament reconstruction
Introduction
Fat-water-based magnetic resonance imaging (MRI) has been used for proton density fat fraction (PDFF) quantification in many tissues, including muscle. Fat-water-based MRI enables PDFF quantification by obtaining MR signal measurements at multiple echo times (TE) and then processing those measurements based on known differences in hydrogen resonant frequencies in water and fat molecules (1). Intramuscular PDFF is being developed as a non-invasive biomarker for muscle composition that may serve a role in patient assessment and clinical trials. Fat accumulation in skeletal muscle occurs in a variety of neural and muscle disorders as well as in atrophy that occurs postoperatively and with muscular disuse and aging. As an example, a higher preoperative estimated fat signal fraction has been associated with a higher likelihood of tendon retear following rotator cuff repair (2). Such assessments may be extended to therapies for other musculoskeletal diseases (e.g., muscular dystrophy, sarcopenia, arthritis). While the reported reproducibility of PDFF in phantoms and in liver has been encouraging (3,4), reproducibility has been observed to degrade substantially when considering cross-vendor comparisons with a mix of processing methods (5). The combination of processing method, vendor, and acquisition protocol has also been observed to substantively affect quantification (6). Encouragingly, recent multi-site and multi-vendor reports demonstrate that reproducible muscle PDFF can be obtained when considering data acquisition, commercial fat-water separation algorithms, T2* compensation, and fat image post-processing (7,8). These observations motivate the investigation of acquisition and processing approaches for reproducible muscle PDFF quantification.
There is an incompletely understood association between knee osteoarthritis (OA) severity and muscle composition and function. Degraded thigh muscle function has been associated with knee OA (9). This impaired muscle function may result in, or be due in part to, fat accumulation in thigh muscle that is part of OA disease progression. A study of Osteoarthritis Initiative data has identified independent associations of thigh muscle size and muscle/fat ratio with knee degeneration (10). In a meta-analysis, both thigh inter- and intra-muscular fat were found to be elevated in patients with knee OA compared to patients without knee OA (11). Furthermore, quadriceps intramuscular fat has been associated with symptomatic and structural severity of knee OA, whereas quadriceps cross-sectional area (size) was not (12). Since fat accumulation in lower limb muscles may be a part of the overall lean muscle mass reduction associated with knee OA (13), reproducible measurement of intramuscular fat broadly applicable across multiple imaging sites is important for longitudinal and cross-sectional studies of OA.
Evaluation of thigh muscle fat accumulation may serve as an indicator of muscle degeneration that can follow joint trauma or surgical interventions, with the associated higher risk of OA. Patients who have had anterior cruciate ligament (ACL) tears have an elevated risk of knee post-traumatic OA (PTOA) even following surgical treatment by anterior cruciate ligament reconstruction (ACLR) (14). Thigh muscle weakness, atrophy, and impaired neuromuscular functions following ACLR have been documented and are suggested as potential risk factors for PTOA development after ACLR (15-21). Early evaluation of thigh muscle fat accumulation may support clinical management of patients to mitigate PTOA onset or progression.
In this study, quantitative PDFF obtained by 6-point Dixon MRI was evaluated in a multi-vendor, multi-site context. Acquisition and quantitative processing techniques applicable across vendors were optimized in phantoms. A novel image post-processing method was developed to mitigate image scaling related errors observed in the Dixon data from some scanners. After developing the scaling correction method in a small subset of data, reproducibility was assessed in phantoms and traveling control subjects across vendors and imaging sites along with intra-site repeatability in phantoms and control subjects. The applicability of the method, and its sensitivity to bilateral PDFF changes, was explored by measuring thigh muscle PDFF in patients approximately 10 years after ACLR and comparing muscle PDFF between the operated and non-operated limbs, testing the hypothesis that patients who had ACL injuries treated by ACLR would have asymmetrically elevated intramuscular fat for some muscle groups. We present this article in accordance with the GRRAS reporting checklist (available at https://qims.amegroups.com/article/view/10.21037/qims-24-287/rc).
Methods
Study sites and MRI hardware
Five clinical MRI systems at three different sites were involved in this study, termed “Site 1”, “Site 2”, and “Site 3”. Site 1 used a 3T MAGNETOM SkyraFit scanner (Siemens Healthcare, Erlangen, Germany) and a 3T Ingenia scanner (Philips Healthcare, Best, Netherlands). Site 2 used a 3T Achieva scanner (Philips Healthcare). Site 3 used a 3T Achieva scanner and a 3T Ingenia Elition X scanner (Philips Healthcare). All scanners employed a multi-channel anterior radiofrequency (RF) coil or an anterior flex RF coil and combined with a multi-channel, in-bed, posterior RF coil (Table 1).
Table 1
Scanner label | Site 1 scanner A | Site 1 scanner B* | Site 2 scanner C | Site 3 scanner D | Site 3 scanner E |
---|---|---|---|---|---|
Scanner model | 3T Siemens MAGNETOM SkyraFit | 3T Philips Ingenia | 3T Philips Achieva | 3T Philips Achieva | 3T Philips Ingenia Elition X |
Receive coils (maximum channel count) | Body 18 (18 channel) | Anterior (16 channel) | Anterior (16 channel) | Anterior (16 channel) | Anterior (16 channel) |
Spine 32 (32 channel) | Posterior (12 channel) | Posterior (12 channel) | Posterior (12 channel) | Posterior (12 channel) |
*, Site 1, scanner B was used only for phantom inter-site/same-vendor or intra-site/inter-vendor experiments. All in vivo scanning at Site 1 used scanner A. MRI, magnetic resonance imaging.
In vitro: phantoms
Water-fat phantoms were constructed in-house as described in a previous report (22). The phantom includes three 50 mL conical tubes containing a mixture of peanut oil, agar, and gadolinium-diethylenetriaminepentacetate (DTPA) contrast agent with targeted volume fat fraction values of 5%, 10%, and 15% [spanning the range of previously reported intramuscular fat in OA (12)] with the noted fat fraction percentage referring to the relative volume of peanut oil in the mixture (Figure 1). Note that the phantom also has three non-fat-containing reference tubes designed for other imaging evaluations of relaxometry, which were not analyzed in this study but did serve as 0% PDFF references for assessment of the proposed scaling correction (see section MRI data processing and Appendix 1). Each phantom included two external markers (vitamin E capsules) that were used to support consistent phantom positioning. After an initial evaluation at Site 1 on scanners A and B, three replicates of the phantom were distributed, one to each site.
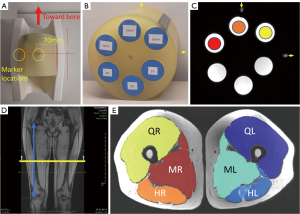
In vivo: healthy volunteers and patients
To assess clinical scan-rescan repeatability and inter-site reproducibility of intramuscular PDFF measurements, healthy (asymptomatic) control subjects over 18 years of age were recruited. The study was conducted in accordance with the Declaration of Helsinki (as revised in 2013) with approval by the Institutional Review Boards (IRBs) of Cleveland Clinic, Vanderbilt University Medical Center, and Ohio State University Medical Center. The IRB number for this protocol is 19-573 (MOON10YR MRI) and the associated Federal Wide Assurance number is FWA00005367. Informed consent was taken from all individual participants. Subjects with clinically diagnosed arthritis, musculoskeletal disease, neuromuscular disease, previous lower limb injuries, contraindications to MRI including metallic implants, or claustrophobia were excluded.
To assess sensitivity of the PDFF measure in a clinically relevant population, both thighs (operated and non-operated sides) of patients at 10 years or more after ACLR were scanned under approved IRB protocols with written informed consent. Patients were recruited from the Multicenter Orthopaedic Outcomes Network (MOON) cohort, and patients were scanned at one of the three sites as part of a multi-site, multi-vendor MRI cohort study.
MRI data acquisition
The MRI PDFF quantification protocols at all three sites included a high-resolution T1-weighted turbo spin echo (T1w-TSE) scan and a monopolar gradient interleaved 6-point Dixon scan using the corresponding vendor product sequences on the scanners with parameters summarized in Table 2. Phantom and human subject protocols were identical except the T1w-TSE was not performed for phantoms. Protocol parameters for the 6-point Dixon acquisition were first optimized at Site 1 and then harmonized between sites, including gradient polarity, repetition time (TR), TE values, flip angle, voxel size, and readout bandwidth, in order to minimize potential inter-site differences in PDFF quantification. TE values were selected to provide optimal in-phase and opposed-phase images for fat-water separation as previously reported (23). The monopolar gradient interleaved 6-point Dixon scan was comprised of two separate 3-point Dixon scans with TE spacing (ΔTE) of 2.46 ms, one 3-point Dixon scan with first TE of 1.23 ms and the other 3-point Dixon scan with first TE of 2.46 ms. The monopolar gradient method was selected to avoid gradient polarity-related errors that may affect the resultant magnitude and phase images. To evaluate the effect of acquisition method, a bipolar gradient 6-point Dixon scan was performed on scanner A. The bipolar gradient scan used the same vendor product sequence and parameters as the monopolar acquisition, except for gradient polarity, and was performed in a single 6-point acquisition with duration 1:39 (1 minute, 39 seconds).
Table 2
Sequence | Parameter | Siemens (Site 1) | Philips (Sites 1–3) |
---|---|---|---|
T1w-TSE | TR (ms) | 795 | 607 |
TE (ms) | 10 | 10 | |
Echo train length | 2 | 2 | |
Bandwidth (Hz) | 199 | 218.5 | |
Matrix size | 512×400×28 | 432×432×28 | |
Field of view (mm2) | 400×312.4 | 312×432.5 | |
Slice thickness (mm) | 5 | 5 | |
Scan time (min:s) | 1:07 | 2:10 | |
Parallel imaging [acceleration factor] | GRAPPA [2] | SENSE [2] | |
6-point Dixon | TR (ms) | 16.37 | 16.37 |
TE (first echo) (ms) | 1.23 | 1.23 | |
ΔTE (ms) | 1.23 | 1.23 | |
Flip angle (deg) | 3 | 3 | |
Bandwidth (Hz) | 1,500 | 1,479.5 | |
Matrix size | 256×192×28 | 256×256×28 | |
Field of view (mm2) | 400×300 | 400×400 | |
Slice thickness (mm) | 5 | 5 | |
Scan time (min:s) | 3:18 | 2:55 | |
Parallel imaging [acceleration factor] | Caipirinha [2] | SENSE [1] |
Bipolar gradient acquisition (Siemens) used the same parameters but with scan time of 1:39. TE, echo time; TR, repetition time; MRI, magnetic resonance imaging; T1w, T1-weighted; TSE, turbo spin echo.
At all sites, the phantom was positioned with tubes oriented in the same way (caps toward scanner bore) with external markers positioned in the same orientation (Figure 1A-1C). Phantoms were scanned at two positions similar to lower limb locations in order to assess side-to-side changes that could occur due to system imperfections: 70 mm left of isocenter and 70 mm right of isocenter.
The protocol for human subjects included bilateral thigh scans using the T1w-TSE and 6-point Dixon sequences. Scans were acquired in the supine, feet-first position. For all scans, the axial T1w-TSE and Dixon scans were acquired at the midpoint between the knee joint line and the superior margin of the greater trochanter (Figure 1D), as determined from a coronal localizer scan. For control subjects, rescans were acquired in the same orientation at the same targeted anatomic location after repositioning the subject.
MRI data processing
PDFF maps were computed from 6-Point Dixon magnitude and/or phase images using three computational approaches: a vendor-independent software package [“FattyRiot” (24)] with complex image processing, the same vendor-independent software package with magnitude-only image processing, and a commercially available, vendor-specific hybrid multi-step adaptive fitting algorithm on scanner A (25). The FattyRiot software incorporates T2* correction, off-resonance map calculation, and a multi-peak fat model (26) as part of the fat-water separation computation; graph-cut and whole-image based optimization approaches are employed in conjunction with a 3×3 pixel “voting kernel” to adaptively select the fat-water separation with locally smallest residuals (24). Considering both acquisition and computational approaches, a total of five methods were evaluated: (I) monopolar acquisition with vendor-independent magnitude processing (termed as ‘monopolar vendor-independent magnitude’); (II) monopolar vendor-independent complex; (III) bipolar vendor-independent magnitude; (IV) bipolar vendor-independent complex; and (V) bipolar vendor-specific.
Phantom regions of interest (ROIs) were automatically defined by thresholding the signal in the center four slices of the first echo magnitude image. Mean PDFF percentage values were calculated within the defined ROI of each phantom tube.
Muscle PDFF for subjects were analyzed for the hamstrings, quadriceps, and medial (medial compartment + sartorius) muscle groups of each thigh (Figure 1E). The muscle groups were defined on T1w-TSE images by fully manual segmentation, or an in-house developed automated segmentation (27) with manual correction, for the four center slices. The T1w-TSE images were registered to the 3rd echo of the 6-point Dixon data (TE =3.69 ms) using an affine transformation and Mattes mutual information similarity metric as implemented in advanced normalization tools (ANTs) (28,29). The 3rd echo was used as it exhibited high contrast between muscle tissue and the surrounding subcutaneous fat as well as strong edges due to the opposing phase of water and fat signal. Average PDFF for each muscle group was recorded.
A scaling correction was developed to mitigate errors observed on some scanner platforms, where the two monopolar 3-point Dixon scans were observed to have different global scaling factors that could degrade the PDFF reproducibility. The correction re-scales the even echoes [2, 4, 6] to have the same scaling as the odd echoes [1, 3, 5] prior to performing PDFF quantification. The signal model used for this correction assumes that a voxel’s signal, S, is non-negative and can be described by an exponential decay with characteristic T2* with signal deviations from the pure exponential due to fat-water phase differences,
where M0 is the initial magnetization, TEi is the echo time at the i-th echo, f is the amount of fat, ai is the signal deviation from the exponential due to fat at the i-th echo, and k is the scaling factor of the even echoes as relative to the odd echoes. Correction of the original data is then performed by determining the value of k and then dividing the even echo signal measurements by this scaling factor, leaving the original signal’s contributions of T2* decay and fat content intact,
Determination of k is carried out using a multi-voxel, regularized, parameter estimation procedure using voxels selected with presumed low levels of fat (e.g., 15% PDFF or less) selected in lean muscle. Details of the method and implementation are included in Appendix 1.
MRI experiments
Evaluation of acquisition and processing methods was performed using phantom data to determine the optimal quantification technique with respect to repeatability. Data for these analyses were acquired on Scanner A for six scans over the course of seven months using one of the three phantom replicates (phantom 1). Repeatability of PDFF measurements was assessed at the left and right positions independently (N=6) as well as when pooling data from both positions (N=12). Data were acquired between March 21, 2020 and August 29, 2023.
Validation of the correction method was performed in phantoms and in a limited number of subjects that had an agarose gel tube placed in the field of view when the Dixon data were acquired. In phantoms, the re-scaling constant k derived from the mixed “fat and water” (FW) correction described previously was compared to a “water only” (WO) correction (see Appendix 1). Most subject scans in this work did not have additional agarose gel tubes, motivating the use of the FW correction following validation experiments. Phantom and traveling control subjects were analyzed to assess inter-site PDFF reproducibility with and without the FW scaling correction.
The most repeatable technique on scanner A was then evaluated for intra-site repeatability, inter-site reproducibility, and inter-vendor. Each site used one of the three phantom replicates (phantom 1 at Site 1, phantom 2 at Site 2, phantom 3 at Site 3) scanned six times for scanners A, C, D, and E over the course of seven months. Prior to the distribution of the phantoms, one set of left (N=1) and right (N=1) position scans for all three phantoms were obtained on Scanner A and Scanner B for intra-site, inter-vendor PDFF reproducibility assessments. After phantom distribution to sites, repeatability was evaluated for the left (N=15), right (N=15), and combined position (N=30) measurements. Intra-site, same vendor reproducibility was assessed at Site 3 for scanners A and B using phantom 3. Inter-site, same vendor reproducibility was assessed for Sites 1 and 2 (phantom 2), and Sites 1 and 3 (phantom 3). Overall reproducibility was assessed by comparing PDFF measurements from scanners A, C, D, and E.
Subjects were recruited and scanned at all sites (scanners A, C, D, and E) using the same acquisition and processing method as the phantom reproducibility assessments. Twenty-seven subjects were enrolled in total, including four local controls at Site 1, eight local controls at Site 2, ten local controls at Site 3, and five traveling controls that were scanned at all three sites. Data were acquired between December 10, 2020 and October 3, 2022. To assess in vivo repeatability of PDFF measurements, the 22 local control and 5 traveling control subjects underwent scan-rescan acquisitions at each site, with the exceptions of only 4 traveling subjects being scanned at Site 2 and only 3 traveling subjects receiving rescans at Site 3 due to logistical constraints.
A total of 135 ACLR patients (59 male, 76 female; 34 from Site 1, 23 from Site 2, 78 from Site 3) were scanned between June 22, 2020 and May 23, 2023. PDFF of the thigh muscle was compared between operated and non-operated limbs to assess potential differences.
Statistical analysis
Repeatability was assessed using the standard deviation (SD) of PDFF measurements, calculated first by treating left and right scan positions as separate categories of measurements, and second by treating left and right scan positions as repeated measures under the same category. Reproducibility assessment also used the SD of PDFF measurements. As such, SD served as an unscaled metric of PDFF measurement precision. The 2-way, absolute intraclass correlation coefficient (ICC) (30) was used as a scaled metric to characterize overall repeatability of the phantom tube measurements for each of left, right, and combined positions. SD and ICC were also used to assess PDFF variability across the phantoms on the same scanner, as phantom variability could be an uncharacterized confounder to inter-site analyses if not separately evaluated. All ICC values are reported on a scale from 0 to 1.
Differences in mean PDFF between left and right scan positions were evaluated using paired, two-tailed t-tests. Unpaired, two-tailed t-tests were used to assess differences in mean PDFF between scanners, where left and right position scans were treated as independent measurements. For the intra-site, inter-vendor comparison, PDFF measurements from left and right position scans for the three phantoms were used as independent measures and paired t-tests were used to assess differences between the scanners. Holm-Bonferroni correction for multiple comparisons was applied for each set of comparisons. All comparisons used a significance threshold of P=0.05 after correction.
In vivo repeatability in controls was assessed by the within-subject standard deviation (wSD) (31) at scan-rescan for each muscle group. Reproducibility was assessed by the wSD across scanners for each muscle group.
In ACLR patients, comparisons of PDFF in corresponding thigh muscle groups between the operated and non-operated limbs used a paired, two-tailed t-test. To account for multiple comparisons, adjusted P values were computed using the Holm-Bonferroni method.
Results
Phantom experiments
The phantom was scanned in six sessions on Scanner A over the course of 7 months (6 for each scan position). Scaling variations between even and odd interleaved 3-echo monopolar scans were not observed in these data and thus the correction was not applied. All quantitative methods, except for the bipolar vendor-independent complex method, provided uniform fat fractions across the phantom tubes (Figure 2). Since there was substantial artifact for the bipolar vendor-independent complex method, this technique was omitted from subsequent analyses. ICC indicated strong repeatability (ICC ≥0.94) among the remaining four methods (Table 3), however, only the monopolar vendor-independent magnitude method provided mean PDFF estimation between left and right positions that were not statistically different (Figure 3). At a fixed position, the PDFF SD was the lowest for the bipolar vendor-specific method, but when evaluating both positions, the monopolar vendor-independent magnitude method has a lower PDFF SD, indicating the best PDFF repeatability in this consideration. The methods other than monopolar vendor-independent magnitude had higher SDs for the combined left and right positions than each position independently, suggesting PDFF variation was position dependent (Table 3, see also Table S1). Patterns of bias for the methods showed overall under-estimation from the monopolar vendor-independent magnitude method that was comparable to the vendor-specific method (using the vendor’s commercial single-voxel spectroscopy-based PDFF quantification as a reference, see Figure S1). Based on these findings, subsequent analyses used only the monopolar vendor-independent magnitude method. No fat-water swaps were observed in this study with this harmonized data acquisition and processing framework.
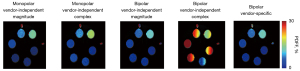
Table 3
Metric | Reference fat fraction | Monopolar vendor-independent magnitude (N=6) | Monopolar vendor-independent complex (N=6) | Bipolar vendor-independent magnitude (N=6) | Bipolar vendor-specific (N=6) | |||||||||||
---|---|---|---|---|---|---|---|---|---|---|---|---|---|---|---|---|
L | R | L & R | L | R | L & R | L | R | L & R | L | R | L & R | |||||
PDFF, SD, % | 5% | 0.7 | 0.3 | 0.5 | 0.7 | 0.2 | 1.7 | 0.7 | 0.3 | 0.8 | 0.1 | 0.3 | 0.7 | |||
10% | 0.3 | 0.2 | 0.2 | 0.4 | 0.2 | 0.5 | 0.2 | 0.1 | 0.6 | 0.2 | 0.2 | 0.6 | ||||
15% | 0.5 | 0.3 | 0.4 | 0.5 | 0.3 | 1.1 | 0.6 | 0.5 | 0.6 | 0.2 | 0.1 | 0.4 | ||||
ICC | All | 0.98 | 1.00 | 0.99 | 0.99 | 1.00 | 0.94 | 0.99 | 0.99 | 0.99 | 1.00 | 1.00 | 1.00 |
PDFF, proton density fat fraction; L, left; R, right; ICC, intraclass correlation coefficient; SD, standard deviation.
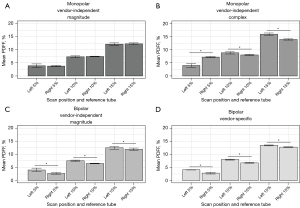
The WO and FW corrections in phantom are shown for a representative case in Figure 4. When applied to 30 phantom scans (5 scan sessions/scanner × 2 scan positions/session × 3 scanners), the scaling value, k, obtained from the FW correction was comparable to the WO-derived value (k=0.96±0.05 for WO vs. k=0.96±0.05 for FW with mean absolute error 0.006), with less error than when the WO method was applied in the 5% FF tube (k=1.00±0.05 with mean absolute error 0.034). Reproducibility, as assessed by PDFF SD in phantoms, wSD in traveling control subjects, and ICC in both, improved when employing the scaling correction (Table 4, see also Table S2). In an example ACLR patient with an added agarose gel tube (no fat), the FW correction was observed to bring the PDFF measurement of the tube closer to 0% PDFF, while also showing an effect on muscle (Figure 5). Scaling correction was employed for affected scanners in the following analyses.
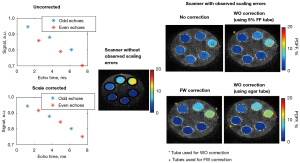
Table 4
Metric | Reference fat fraction | Phantom (without correction) | Phantom (water only correction) | Phantom (fat and water correction) |
---|---|---|---|---|
PDFF, SD, % | 5% | 1.4 | 0.5 | 0.6 |
10% | 1.7 | 0.6 | 0.7 | |
15% | 2.7 | 1.2 | 1.2 | |
ICC | All | 0.80 | 0.98 | 0.98 |
PDFF, proton density fat fraction; SD, standard deviation; ICC, intraclass correlation coefficient.
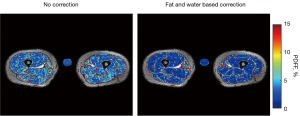
Intra-site repeatability as described by SD and ICC ranged between 0.2–0.9% PDFF and 0.98–1.00, respectively (Table 5, see also Table S3). SD for the combined left and right positions was comparable to or lower than each position independently, suggesting position-dependent PDFF variation was negligible relative to fixed-position repeatability. One dataset from Scanner D was omitted due to improper positioning that precluded PDFF quantification.
Table 5
Metric | Reference fat fraction | Site 1, scanner A (N=15) | Site 2, scanner C (N=15) | Site 3, scanner D (N=14) | Site 3, scanner E (N=15) | |||||||||||
---|---|---|---|---|---|---|---|---|---|---|---|---|---|---|---|---|
L | R | L & R | L | R | L & R | L | R | L & R | L | R | L & R | |||||
PDFF, SD, % | 5% | 0.9 | 0.6 | 0.8 | 0.2 | 0.3 | 0.3 | 0.3 | 0.3 | 0.3 | 0.3 | 0.2 | 0.3 | |||
10% | 0.5 | 0.2 | 0.4 | 0.3 | 0.3 | 0.3 | 0.2 | 0.2 | 0.2 | 0.4 | 0.3 | 0.4 | ||||
15% | 0.4 | 0.4 | 0.4 | 0.3 | 0.3 | 0.3 | 0.3 | 0.2 | 0.2 | 0.7 | 0.3 | 0.5 | ||||
ICC | All | 0.98 | 0.99 | 0.98 | 1.00 | 1.00 | 0.99 | 1.00 | 1.00 | 0.99 | 0.99 | 1.00 | 0.99 |
PDFF, proton density fat fraction; ICC, intraclass correlation coefficient; SD, standard deviation; L, left; R, right.
Reproducibility across scanners at Site 3 showed comparable mean PDFF values (Figure 6A). Within Site 1, mean PDFF across scanners showed statistically significant differences of 0.6% and 1.0% PDFF (inter-vendor, same site), which was smaller than significant differences observed between Sites 1 and 2, 1.0–1.7% PDFF (same vendor, different site comparison) (Figure 6B,6C). However, comparison of mean values for Sites 1 and 3 showed differences of 0.3–0.5% PDFF that did not reach statistical significance (same vendor, different site comparison) (Figure 6D). Cross-phantom PDFF variability was also evaluated to confirm low variation in phantom values (Table S4).
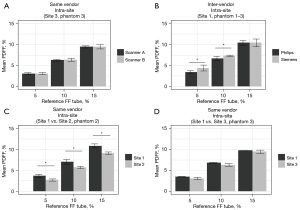
Intra-site, inter-vendor evaluation showed high reproducibility at Site 1 with SD between 0.5–0.7% PDFF and ICC of 0.96 (Table 6). For inter-site, same vendor assessment, SD ranged from 0.5–1.0% PDFF (ICC =0.99–1.00). Overall inter-site, inter-vendor reproducibility had SD between 0.8–1.7% PDFF and ICC =0.97.
Table 6
Metric | Reference fat fraction | Intra-site (Site 1), inter-vendor (N=6) |
Inter-site (Sites 1, 2), same vendor (N=2) | Inter-site (Sites 1, 3), same vendor (N=2) | Inter-site (Sites 1, 2, 3), inter-vendor (N=3) |
---|---|---|---|---|---|
PDFF, SD, % | 5% | 0.7 | 0.5 | 0.5 | 0.7 |
10% | 0.5 | 0.7 | 0.5 | 0.8 | |
15% | 0.5 | 0.8 | 1.0 | 1.7 | |
ICC | All | 0.96 | 1.00 | 0.99 | 0.97 |
PDFF, proton density fat fraction; SD, standard deviation; ICC, intraclass correlation coefficient.
In vivo experiments
Representative segmented PDFF maps are overlaid on co-registered T1w-TSE images in Figure 7 for scan-rescan experiments from each site. Reproducibility in traveling control subjects, as assessed by wSD and ICC, improved when employing the scaling correction (Table 7, see also Table S5). The intra-site PDFF wSD ranged between 0.2–0.8% PDFF with ICC between 0.95–0.98 (Table 8, see also Table S6). In an example ACLR patient with an added agarose gel tube (no fat), the FW correction was observed to bring the PDFF measurement of the tube closer to 0% PDFF, while also showing an effect on muscle. Inter-site reproducibility from traveling control subjects scanned across the three sites (four scanners) had higher wSD (0.7–1.2%), and lower ICC (0.92), as compared to same-scanner repeatability.
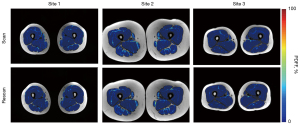
Table 7
Metric | Muscle group | Traveling control (without correction) | Traveling control (fat and water correction) |
---|---|---|---|
PDFF, wSD (%) | HL | 1.6 | 1.4 |
HR | 1.8 | 1.0 | |
QL | 1.5 | 0.9 | |
QR | 1.6 | 0.8 | |
ML | 1.6 | 0.7 | |
MR | 1.6 | 0.6 | |
ICC | All | 0.70 | 0.92 |
HL, hamstrings left; HR, hamstrings right; QL, quadriceps left; QR, quadriceps right; ML, medial left; MR, medial right; PDFF, proton density fat fraction; ICC, intraclass correlation coefficient; wSD, within-subject standard deviation.
Table 8
Metric | Muscle Group | Site 1 (N=8) | Site 2 (N=11) | Site 3 (N=16) | Inter-Site (N=5) |
---|---|---|---|---|---|
PDFF, wSD (%) | HL | 0.7 | 0.4 | 0.6 | 1.2 |
HR | 0.3 | 0.3 | 0.5 | 1.0 | |
QL | 0.8 | 0.2 | 0.4 | 0.9 | |
QR | 0.4 | 0.2 | 0.3 | 0.7 | |
ML | 0.7 | 0.4 | 0.8 | 0.9 | |
MR | 0.2 | 0.3 | 0.6 | 0.8 | |
ICC | All | 0.96 | 0.98 | 0.95 | 0.92 |
wSD, within-subject standard deviation; PDFF, proton density fat fraction; ICC, intraclass correlation coefficient; HL, hamstrings left; HR, hamstrings right; QL, quadriceps left; QR, quadriceps right; ML, medial left; MR, medial right.
A total of 135 ACLR patients were evaluated with a mean age of 33.7±5.2 years and body mass index (BMI) of 25.7±4.8 kg/m2. Representative segmented PDFF maps are overlaid on co-registered T1w-TSE images in Figure 8 for patients from each site. Visually evident fat accumulation was present in the operated limbs of some, but not all, ACLR patients (Figure 8). Comparisons of operated and non-operated thigh muscles indicated higher PDFF in operated side hamstrings as compared to the contralateral hamstrings (6.2%±3.5% vs. 5.7%±2.8%, P=0.006) (Figure 9). Medial and quadriceps muscle groups did not have statistically significant differences (medial: 5.7%±2.5% vs. 5.5%±2.3%, P=0.133) (quadriceps: 4.0%±2.0% vs. 4.0%±2.1%, P=0.961).
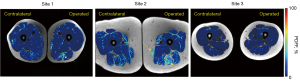
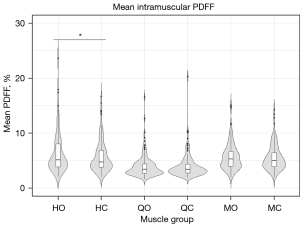
Discussion
In this multi-vendor, multi-site study, we developed an acquisition and processing framework for reproducible multi-site, multi-vendor intramuscular fat fraction quantification by 6-point Dixon MRI and demonstrated its utility for assessment of thigh muscle fat accumulation in an ACLR patient cohort. Monopolar gradient acquisition with vendor-independent, magnitude-based processing yielded the most reproducible PDFF measurements overall in phantom experiments. With the harmonized MRI protocols in this study, reproducibility as assessed by SD in phantoms ranged between 0.5–1.7% PDFF for all inter-scanner evaluations. Same scanner repeatability in healthy controls as assessed by wSD was between 0.2–0.8% PDFF for all sites and muscle groups. Reproducibility in traveling controls as assessed by wSD was between 0.7–1.2% across muscle groups. When applied to ACLR patients, intramuscular PDFF was elevated in the hamstrings of the operated limbs.
The reliability of PDFF quantification in the phantom experiments was highly dependent on the acquisition and processing methods. We observed the best repeatability at a fixed scan position with the bipolar gradient vendor-specific method, however, PDFF varied significantly between left and right scan positions for bipolar gradient acquisition independent of post-processing algorithm. In contrast, PDFF measurements using the monopolar acquisition with vendor-independent magnitude-based processing did not vary significantly with phantom position. We suspect the observed position-dependent differences in the bipolar gradient methods may be due to position-related field inhomogeneity, which may have exacerbated errors due to other system imperfections in bipolar acquisition and with complex processing. Effects of gradient polarity on PDFF have been described previously and have been attributed to system imperfections that include echo misalignments in k-space, concomitant gradient fields, constant phase offset between time-interleaved echo trains, and asymmetric amplitude modulation in readouts due to receiver frequency response characteristics (32,33). With respect to magnitude processing versus complex processing, our observation of more precise PDFF quantification is consistent with a previous comparison of similar 6-echo chemical shift-based MRI methods (34). However, we observed consistent underestimation on the order of 1–6% PDFF when compared to the nominal fat fraction of the phantom tubes [5–15% FF (22)], a relative underestimation of approximately 20–30% that has been similarly reported with magnitude-based fitting [e.g., Fig. 3 of (35)] and may be due to echo sampling effects. Complex-based methods have the advantage of better noise performance and lower sensitivity to fat signal modeling errors compared to magnitude-based methods with the tradeoff of being more prone to previously described phase errors (32,36-38). Concomitant gradients alone can lead to PDFF errors of up to 10% with complex image-based processing, but can be reduced to as low as 1% with correction (36). In addition to phase errors, other confounding factors that need to be considered to minimize bias on PDFF estimates include the presence of multiple peaks in the fat spectrum, presence of susceptibility-induced fat resonance shifts, T1 effects, and temperature effects (3,39-43). Magnitude image processing is also impacted by susceptibility-induced fat resonance shifts that can impact PDFF estimates particularly in skeletal muscle (44). When such confounding effects are accounted for via corrections, complex image processing may be preferable to magnitude image processing due to the additional information contained within phase data. Recent reports have shown that 2-point Dixon water-fat reconstructions may be corrected using post-processing to enable reproducible multi-site, multi-vendor muscle fat assessment (7,8), which may also be applicable to 6-point Dixon data. Despite limitations of various acquisition and processing methods, our observations suggest that, with the acquisition protocols and processing methods used in this study, monopolar gradient acquisitions with magnitude-based processing may be preferable to bipolar gradient acquisition or complex-based processing in order to obtain position-independent, reproducible intramuscular PDFF quantification.
The scaling correction method was observed to improve reproducibility by mitigating observed scaling differences between the two interleaved 3-point Dixon acquisitions on affected scanners. It should be noted that while the scans used product versions of 3-point Dixon sequences, the combination employed in this work used separate scans in a way that is not typically supported on the vendor platform. Despite efforts being taken to minimize calibrations or other system changes between the two scans, the resulting images still exhibited the observed scaling difference, necessitating such correction. The correction method’s single regularization parameter was tuned and confirmed by using one phantom dataset from each scanner and one traveling control subject’s dataset from each scanner, and reproducibility was assessed on the remaining held-out datasets. We observed that the effect of the correction was similar when including the tuning datasets (see Tables S2,S5). Note that this correction assumes that voxels with low levels of fat can be selected, which may not be true in severely degraded muscle seen in some medical conditions or in all anatomic locations. Beyond the specific application of this study, the approach taken for this scaling correction may be applicable to other problems where the underlying signal model is known but there is a scaling parameter that needs to also be estimated and used for a correction. MRI researchers, particularly in multi-site study contexts, do not always have access to research sequences or detailed scanner settings and may benefit from this image post-processing approach.
Intra-site repeatability in terms of PDFF SD was <1% and reproducibility was 2% across scanners, sites, and vendors. Interestingly, differences between scanners from different vendors were not observed to be as large as differences between some scanners of the same vendor. Specifically, the difference in phantom PDFF between two vendors at Site 1 was not as substantial as that observed for the same vendor between Sites 1 and 3. Furthermore, the same scanner platform at Site 1 when compared with Site 2 did not show statistically significant differences. Taken together, these observations suggest that scanners of a common vendor may not necessarily have the same reproducibility profiles, even if acquisition protocols and processing are harmonized.
The repeatability metrics from this study are within or lower than the range of PDFF SD reported by Schneider et al. (5), SD of 1.3–4.1% PDFF, for comparable phantom reference fat fraction tubes of 0–16% fat. Overall inter-vendor, inter-site reproducibility in the present study for both phantom and in vivo data had SD and wSD of 0.7–1.7% PDFF, which is also notably less than the 7% reported previously, potentially due in part to their use of the vendor-specific (commercial) acquisition parameters and processing methods of three vendors. Standardized acquisition and processing may therefore improve reproducibility. In a report by Hu et al. (6), variation of acquisition method was shown to affect the linearity and bias of PDFF measurements as compared to reference values in phantom with the use of vendor-specific processing. Whereas the systems in the present study exhibited relatively good inter-vendor reproducibility under a standardized protocol, PDFF measurements in the previous report showed differing bias and linearity characteristics, suggesting that vendor-specific processing may degrade inter-vendor reproducibility as compared to centralized, vendor-independent processing (6).
In the ACLR patients, PDFF was elevated in the hamstrings muscle group of the operated limb as compared to the contralateral limb. The mean difference in PDFF observed between operated ACLR and contralateral muscle groups was relatively small, on the order of 0.5% PDFF, emphasizing the need for precise, reproducible intramuscular PDFF quantification. Fat accumulation in the thighs of ACLR operated limbs may be due to a combination of effects. Significant fat accumulation in the hamstrings was observed within the semitendinosus muscle consistent with the previously reported muscle atrophy of semitendinosus muscle at 9–11 years post-ACL reconstruction (45). We observed no significant elevation in intramuscular fat fraction for the quadriceps muscles of ACLR limbs vs. non-operated limbs (4.0%±2.0% vs. 4.0%±2.1%, P=0.961), which is notably different than reports of knee OA where quadriceps fat is increased (12). The lack of apparent quadriceps fat accumulation post-ACLR may indicate a different disease process or an earlier time point in PTOA development as compared to other forms or more advanced knee OA.
We note limitations of the study. This study focused on reproducibility and did not emphasize the accuracy or bias of various acquisition and processing methods. Patterns of PDFF bias from MRI vendors’ commercial processing methods have been reported, showing that accuracy varies depending on both the vendor and the target object’s fat content (5). Additionally, magnitude-based processing has been reported to under-estimate PDFF as compared to MR spectroscopy while complex-based processing over-estimated PDFF (46), but both approaches exhibited strong agreement with MR spectroscopy. These observations emphasize that caution should be taken to avoid mixing of different PDFF quantification methods as well as when assessing the reproducibility and bias needs of a given application. It should also be noted that this study was limited to 3T platforms from two vendors. Another limitation is the lack of inter-site reproducibility assessment in patients with muscle-fat replacement or other abnormal fat accumulation. A higher degree of fat accumulation may coincide with different tissue properties as compared to controls, which may exhibit different reproducibility characteristics. Scan-rescan of patients and “traveling patient” scans could address this limitation. Also, the choice of fat MR spectrum model may influence results. The Dixon MRI processing that was used had been previously reported for skeletal muscle evaluation and assumed a fat spectrum model derived from liver (47), however, as muscular fat differs from liver fat, a dedicated intramuscular fat-specific spectrum model (48) may improve reproducibility and/or sensitivity to fat accumulation. Lastly, this work only evaluated the mean intramuscular PDFF acquired in four mid-thigh slices. Other statistical measures of fat fraction (49), as well as textural features (50), and full 3D volumetric measurements (51) have been reported for evaluation of proximal lower limb muscle quality, and these measures may contain additional insight into fat accumulation following ACLR.
Conclusions
We investigated the repeatability, reproducibility, and an example application of 6-point Dixon MRI for intramuscular fat quantification in a multi-site, multi-vendor context. Using a vendor-independent magnitude-based processing pipeline and monopolar acquisition, repeatability in phantoms and in vivo was characterized by SD less than 1% PDFF, and overall inter-site, inter-vendor reproducibility had SD less than 2% PDFF. Using a multi-vendor, multi-site cohort of patients after ACLR, we observed elevated PDFF in the hamstrings of the operated limb as compared to the contralateral, non-operated limb. Evaluation of thigh intramuscular fat by 6-point Dixon MRI may provide insight into disease progression and is feasible in a properly designed multi-vendor, multi-site study.
Acknowledgments
The authors would like to recognize Mark George (Vanderbilt University Medical Center) for his assistance with making the phantoms, Charlotte Lo (Cleveland Clinic) for her assistance with data analysis, and Kihwan Kim (Cleveland Clinic) for his assistance with interpretation of results.
Funding: This work was funded in part by the following sources:
Footnote
Reporting Checklist: The authors have completed the GRRAS reporting checklist. Available at https://qims.amegroups.com/article/view/10.21037/qims-24-287/rc
Conflicts of Interest: All authors have completed the ICMJE uniform disclosure form (available at https://qims.amegroups.com/article/view/10.21037/qims-24-287/coif). B.L.E. serves as an unpaid editorial board member of Quantitative Imaging in Medicine and Surgery. B.L.E. received support from NIH/NIAMS T32AR007505 and NIH/NIA K25AG070321. He is an employee of Clario as of August 2024; C.S.W. reports grant support from NIH/NIAMS 075422. C.S.W. reports unpaid leadership role(s) and/or board membership for the Society of Academic Bone Radiologists and Osteoarthritis and Cartilage. W.Z. reports salary support from NIH/NIAMS T32AR007505. K.D.H. reports grant support from NIH/NIAMS R01AR075422. B.M.D. received remuneration for professional efforts on this project, in the form of salary support between 7/2019 and 2/2021. L.J.H. reports the affiliated institution (Vanderbilt University) received subcontract funds from Cleveland Clinic for an NIH grant awarded for work related to this manuscript. N.A.O. provided statistical consulting to RSNA and FNIH to study the reproducibility of PDFF and other imaging biomarkers through contracts between RSNA/FNIH and the Cleveland Clinic. She has received support for meetings/travel from RSNA. N.A.O. serves on the DSMB for ECOG-ACRIN which oversees studies of imaging biomarkers and is on the NCI CISC which reviews a variety of imaging biomarker proposals. X.Z. was a Siemens employee while the study was ongoing. X.Z. received salary, stock, travel reimbursement and other incentives from Siemens because of his Siemens employment, which were not associated with this study. X.Z. is currently an employee of the University of California, Los Angeles and receives research funding from Siemens Medical Solutions USA, Inc. which is not associated with this study. K.L. is an employee of Siemens Medical Solutions, Inc. H.F. was an employee of Philips Healthcare. D.C.K. reports grant support from Philips Healthcare. M.H.J. has received grant support from Pacira Therapeutics, Arthritis Foundation, and the National Institutes of Health; consulting fees from the Journal of Bone and Joint Surgery for online curriculum development, Regeneron for serving on the scientific advisory board, and Grunenthal for serving on the scientific advisory board. K.P.S. reports grant support from NIH/NIAMS AR075422, NIH/NIAMS AR074131 and NIH/NIAMS AR053684; royalties from Oberd; consulting fees from NFL and Novopedics, Inc.; travel support from AOSSM; board or leadership roles in Novopedics, Inc. and AOSSM; and research-related material support from DJO Orthopaedics, and other financial or non-financial interest with Novopedics, Inc. X.L. reports research grant and support for meetings/travel from NIH/NIAMS AR075422 and the Arthritis Foundation. The other authors have no conflicts of interest to declare.
Ethical Statement: The authors are accountable for all aspects of the work in ensuring that questions related to the accuracy or integrity of any part of the work are appropriately investigated and resolved. The study was conducted in accordance with the Declaration of Helsinki (as revised in 2013) with approval by the Institutional Review Boards (IRBs) of Cleveland Clinic, Vanderbilt University Medical Center, and Ohio State University Medical Center. The IRB number for this protocol is 19-573 (MOON10YR MRI) and the associated Federal Wide Assurance number is FWA00005367. Informed consent was taken from all individual participants.
Open Access Statement: This is an Open Access article distributed in accordance with the Creative Commons Attribution-NonCommercial-NoDerivs 4.0 International License (CC BY-NC-ND 4.0), which permits the non-commercial replication and distribution of the article with the strict proviso that no changes or edits are made and the original work is properly cited (including links to both the formal publication through the relevant DOI and the license). See: https://creativecommons.org/licenses/by-nc-nd/4.0/.
References
- Hu HH, Börnert P, Hernando D, Kellman P, Ma J, Reeder S, Sirlin C. ISMRM workshop on fat-water separation: insights, applications and progress in MRI. Magn Reson Med 2012;68:378-88. [Crossref] [PubMed]
- Nozaki T, Tasaki A, Horiuchi S, Ochi J, Starkey J, Hara T, Saida Y, Yoshioka H. Predicting Retear after Repair of Full-Thickness Rotator Cuff Tear: Two-Point Dixon MR Imaging Quantification of Fatty Muscle Degeneration-Initial Experience with 1-year Follow-up. Radiology 2016;280:500-9. [Crossref] [PubMed]
- Hernando D, Sharma SD, Aliyari Ghasabeh M, Alvis BD, Arora SS, Hamilton G, Pan L, Shaffer JM, Sofue K, Szeverenyi NM, Welch EB, Yuan Q, Bashir MR, Kamel IR, Rice MJ, Sirlin CB, Yokoo T, Reeder SB. Multisite, multivendor validation of the accuracy and reproducibility of proton-density fat-fraction quantification at 1.5T and 3T using a fat-water phantom. Magn Reson Med 2017;77:1516-24. [Crossref] [PubMed]
- Yokoo T, Serai SD, Pirasteh A, Bashir MR, Hamilton G, Hernando D, Hu HH, Hetterich H, Kühn JP, Kukuk GM, Loomba R, Middleton MS, Obuchowski NA, Song JS, Tang A, Wu X, Reeder SB, Sirlin CBRSNA-QIBA PDFF Biomarker Committee. Linearity, Bias, and Precision of Hepatic Proton Density Fat Fraction Measurements by Using MR Imaging: A Meta-Analysis. Radiology 2018;286:486-98. [Crossref] [PubMed]
- Schneider E, Remer EM, Obuchowski NA, McKenzie CA, Ding X, Navaneethan SD. Long-term inter-platform reproducibility, bias, and linearity of commercial PDFF MRI methods for fat quantification: a multi-center, multi-vendor phantom study. Eur Radiol 2021;31:7566-74. [Crossref] [PubMed]
- Hu HH, Yokoo T, Bashir MR, Sirlin CB, Hernando D, Malyarenko D, et al. Linearity and Bias of Proton Density Fat Fraction as a Quantitative Imaging Biomarker: A Multicenter, Multiplatform, Multivendor Phantom Study. Radiology 2021;298:640-51. [Crossref] [PubMed]
- Borga M, Ahlgren A, Romu T, Widholm P, Dahlqvist Leinhard O, West J. Reproducibility and repeatability of MRI-based body composition analysis. Magn Reson Med 2020;84:3146-56. [Crossref] [PubMed]
- Widholm P, Ahlgren A, Karlsson M, Romu T, Tawil R, Wagner KR, Statland JM, Wang LH, Shieh PB, van Engelen BGM, Cadavid D, Ronco L, Odueyungbo AO, Jiang JG, Mellion ML, Dahlqvist Leinhard O. Quantitative muscle analysis in facioscapulohumeral muscular dystrophy using whole-body fat-referenced MRI: Protocol development, multicenter feasibility, and repeatability. Muscle Nerve 2022;66:183-92. [Crossref] [PubMed]
- Krishnasamy P, Hall M, Robbins SR. The role of skeletal muscle in the pathophysiology and management of knee osteoarthritis. Rheumatology (Oxford) 2018;57:iv124. [Crossref] [PubMed]
- Jungmann PM, Baum T, Nevitt MC, Nardo L, Gersing AS, Lane NE, McCulloch CE, Rummeny EJ, Link TM. Degeneration in ACL Injured Knees with and without Reconstruction in Relation to Muscle Size and Fat Content-Data from the Osteoarthritis Initiative. PLoS One 2016;11:e0166865. [Crossref] [PubMed]
- Pedroso MG, de Almeida AC, Aily JB, de Noronha M, Mattiello SM. Fatty infiltration in the thigh muscles in knee osteoarthritis: a systematic review and meta-analysis. Rheumatol Int 2019;39:627-35. [Crossref] [PubMed]
- Kumar D, Karampinos DC, MacLeod TD, Lin W, Nardo L, Li X, Link TM, Majumdar S, Souza RB. Quadriceps intramuscular fat fraction rather than muscle size is associated with knee osteoarthritis. Osteoarthritis Cartilage 2014;22:226-34. [Crossref] [PubMed]
- Lee SY, Ro HJ, Chung SG, Kang SH, Seo KM, Kim DK. Low Skeletal Muscle Mass in the Lower Limbs Is Independently Associated to Knee Osteoarthritis. PLoS One 2016;11:e0166385. [Crossref] [PubMed]
- Wang LJ, Zeng N, Yan ZP, Li JT, Ni GX. Post-traumatic osteoarthritis following ACL injury. Arthritis Res Ther 2020;22:57. [Crossref] [PubMed]
- Baugher WH, Warren RF, Marshall JL, Joseph A. Quadriceps atrophy in the anterior cruciate insufficient knee. Am J Sports Med 1984;12:192-5. [Crossref] [PubMed]
- Keays SL, Newcombe PA, Bullock-Saxton JE, Bullock MI, Keays AC. Factors involved in the development of osteoarthritis after anterior cruciate ligament surgery. Am J Sports Med 2010;38:455-63. [Crossref] [PubMed]
- Øiestad BE, Holm I, Gunderson R, Myklebust G, Risberg MA. Quadriceps muscle weakness after anterior cruciate ligament reconstruction: a risk factor for knee osteoarthritis? Arthritis Care Res (Hoboken) 2010;62:1706-14. [Crossref] [PubMed]
- Tourville TW, Jarrell KM, Naud S, Slauterbeck JR, Johnson RJ, Beynnon BD. Relationship between isokinetic strength and tibiofemoral joint space width changes after anterior cruciate ligament reconstruction. Am J Sports Med 2014;42:302-11. [Crossref] [PubMed]
- Lautamies R, Harilainen A, Kettunen J, Sandelin J, Kujala UM. Isokinetic quadriceps and hamstring muscle strength and knee function 5 years after anterior cruciate ligament reconstruction: comparison between bone-patellar tendon-bone and hamstring tendon autografts. Knee Surg Sports Traumatol Arthrosc 2008;16:1009-16. [Crossref] [PubMed]
- Pamukoff DN, Montgomery MM, Choe KH, Moffit TJ, Garcia SA, Vakula MN. Bilateral Alterations in Running Mechanics and Quadriceps Function Following Unilateral Anterior Cruciate Ligament Reconstruction. J Orthop Sports Phys Ther 2018;48:960-7. [Crossref] [PubMed]
- Norte GE, Hertel J, Saliba SA, Diduch DR, Hart JM. Quadriceps Neuromuscular Function in Patients With Anterior Cruciate Ligament Reconstruction With or Without Knee Osteoarthritis: A Cross-Sectional Study. J Athl Train 2018;53:475-85.
- Bush EC, Gifford A, Coolbaugh CL, Towse TF, Damon BM, Welch EB. Fat-Water Phantoms for Magnetic Resonance Imaging Validation: A Flexible and Scalable Protocol. J Vis Exp 2018;57704. [Crossref] [PubMed]
- Grimm A, Meyer H, Nickel MD, Nittka M, Raithel E, Chaudry O, Friedberger A, Uder M, Kemmler W, Quick HH, Engelke K. Evaluation of 2-point, 3-point, and 6-point Dixon magnetic resonance imaging with flexible echo timing for muscle fat quantification. Eur J Radiol 2018;103:57-64. [Crossref] [PubMed]
- Smith DS, Berglund J, Kullberg J, Ahlstrm H, Welch E. Optimization of Fat-Water Separation Algorithm Selection and Options Using Image-Based Metrics with Validation by ISMRM Fat-Water Challenge Datasets. In: Proc 21st Annu Meet ISMRM. 2013:2413.
- Zhong X, Nickel MD, Kannengiesser SA, Dale BM, Kiefer B, Bashir MR. Liver fat quantification using a multi-step adaptive fitting approach with multi-echo GRE imaging. Magn Reson Med 2014;72:1353-65. [Crossref] [PubMed]
- Hamilton G, Yokoo T, Bydder M, Cruite I, Schroeder ME, Sirlin CB, Middleton MS. In vivo characterization of the liver fat 1H MR spectrum. NMR Biomed 2011;24:784-90. [Crossref] [PubMed]
- Gaj S, Eck BL, Xie D, Lartey R, Lo C, Zaylor W, Yang M, Nakamura K, Winalski CS, Spindler KP, Li X. Deep learning-based automatic pipeline for quantitative assessment of thigh muscle morphology and fatty infiltration. Magn Reson Med 2023;89:2441-55. [Crossref] [PubMed]
- Avants BB, Tustison N, Song G. Advanced normalization tools (ANTS). Insight J 2009;2:1-35.
- Avants BB, Tustison NJ, Song G, Cook PA, Klein A, Gee JC. A reproducible evaluation of ANTs similarity metric performance in brain image registration. Neuroimage 2011;54:2033-44. [Crossref] [PubMed]
- Weir JP. Quantifying test-retest reliability using the intraclass correlation coefficient and the SEM. J Strength Cond Res 2005;19:231-40. [Crossref] [PubMed]
- Whisenant JG, Ayers GD, Loveless ME, Barnes SL, Colvin DC, Yankeelov TE. Assessing reproducibility of diffusion-weighted magnetic resonance imaging studies in a murine model of HER2+ breast cancer. Magn Reson Imaging 2014;32:245-9. [Crossref] [PubMed]
- Ruschke S, Eggers H, Kooijman H, Diefenbach MN, Baum T, Haase A, Rummeny EJ, Hu HH, Karampinos DC. Correction of phase errors in quantitative water-fat imaging using a monopolar time-interleaved multi-echo gradient echo sequence. Magn Reson Med 2017;78:984-96. [Crossref] [PubMed]
- Yu H, Shimakawa A, McKenzie CA, Lu W, Reeder SB, Hinks RS, Brittain JH. Phase and amplitude correction for multi-echo water-fat separation with bipolar acquisitions. J Magn Reson Imaging 2010;31:1264-71. [Crossref] [PubMed]
- Tyagi A, Yeganeh O, Levin Y, Hooker JC, Hamilton GC, Wolfson T, Gamst A, Zand AK, Heba E, Loomba R, Schwimmer J, Middleton MS, Sirlin CB. Intra- and inter-examination repeatability of magnetic resonance spectroscopy, magnitude-based MRI, and complex-based MRI for estimation of hepatic proton density fat fraction in overweight and obese children and adults. Abdom Imaging 2015;40:3070-7. [Crossref] [PubMed]
- Hernando D, Hines CD, Yu H, Reeder SB. Addressing phase errors in fat-water imaging using a mixed magnitude/complex fitting method. Magn Reson Med 2012;67:638-44. [Crossref] [PubMed]
- Colgan TJ, Hernando D, Sharma SD, Reeder SB. The effects of concomitant gradients on chemical shift encoded MRI. Magn Reson Med 2017;78:730-8. [Crossref] [PubMed]
- Wang X, Hernando D, Reeder SB. Sensitivity of chemical shift-encoded fat quantification to calibration of fat MR spectrum. Magn Reson Med 2016;75:845-51. [Crossref] [PubMed]
- Hernando D, Liang ZP, Kellman P. Chemical shift-based water/fat separation: a comparison of signal models. Magn Reson Med 2010;64:811-22. [Crossref] [PubMed]
- Karampinos DC, Yu H, Shimakawa A, Link TM, Majumdar S T. 1-corrected fat quantification using chemical shift-based water/fat separation: application to skeletal muscle. Magn Reson Med 2011;66:1312-26. [Crossref] [PubMed]
- Karampinos DC, Melkus G, Baum T, Bauer JS, Rummeny EJ, Krug R. Bone marrow fat quantification in the presence of trabecular bone: initial comparison between water-fat imaging and single-voxel MRS. Magn Reson Med 2014;71:1158-65. [Crossref] [PubMed]
- Yu H, Shimakawa A, McKenzie CA, Brodsky E, Brittain JH, Reeder SB. Multiecho water-fat separation and simultaneous R2* estimation with multifrequency fat spectrum modeling. Magn Reson Med 2008;60:1122-34. [Crossref] [PubMed]
- Bydder M, Yokoo T, Hamilton G, Middleton MS, Chavez AD, Schwimmer JB, Lavine JE, Sirlin CB. Relaxation effects in the quantification of fat using gradient echo imaging. Magn Reson Imaging 2008;26:347-59. [Crossref] [PubMed]
- Liu CY, McKenzie CA, Yu H, Brittain JH, Reeder SB. Fat quantification with IDEAL gradient echo imaging: correction of bias from T(1) and noise. Magn Reson Med 2007;58:354-64. [Crossref] [PubMed]
- Karampinos DC, Yu H, Shimakawa A, Link TM, Majumdar S. Chemical shift-based water/fat separation in the presence of susceptibility-induced fat resonance shift. Magn Reson Med 2012;68:1495-505. [Crossref] [PubMed]
- Snow BJ, Wilcox JJ, Burks RT, Greis PE. Evaluation of muscle size and fatty infiltration with MRI nine to eleven years following hamstring harvest for ACL reconstruction. J Bone Joint Surg Am 2012;94:1274-82. [Crossref] [PubMed]
- Haufe WM, Wolfson T, Hooker CA, Hooker JC, Covarrubias Y, Schlein AN, Hamilton G, Middleton MS, Angeles JE, Hernando D, Reeder SB, Schwimmer JB, Sirlin CB. Accuracy of PDFF estimation by magnitude-based and complex-based MRI in children with MR spectroscopy as a reference. J Magn Reson Imaging 2017;46:1641-7. [Crossref] [PubMed]
- Damon BM, Li K, Dortch RD, Welch EB, Park JH, Buck AK, Towse TF, Does MD, Gochberg DF, Bryant ND. Quantitative Magnetic Resonance Imaging of Skeletal Muscle Disease. J Vis Exp 2016;52352. [Crossref] [PubMed]
- Krššák M, Lindeboom L, Schrauwen-Hinderling V, Szczepaniak LS, Derave W, Lundbom J, Befroy D, Schick F, Machann J, Kreis R, Boesch C. Proton magnetic resonance spectroscopy in skeletal muscle: Experts' consensus recommendations. NMR Biomed 2021;34:e4266. [Crossref] [PubMed]
- Reyngoudt H, Baudin P, Araujo E, Carlier P, Marty B. IMAGING: EP.338 Fat fraction histogram metrics assessed by quantitative MRI demonstrate differences between neuromuscular diseases. Neuromuscular Disorders 2021;31:S152-3.
- Dieckmeyer M, Inhuber S, Schläger S, Weidlich D, Mookiah MRK, Subburaj K, Burian E, Sollmann N, Kirschke JS, Karampinos DC, Baum T. Association of Thigh Muscle Strength with Texture Features Based on Proton Density Fat Fraction Maps Derived from Chemical Shift Encoding-Based Water-Fat MRI. Diagnostics (Basel) 2021;11:302. [Crossref] [PubMed]
- Huysmans L, De Wel B, Claeys KG, Maes F. Automated MRI quantification of volumetric per-muscle fat fractions in the proximal leg of patients with muscular dystrophies. Front Neurol 2023;14:1200727. [Crossref] [PubMed]