Deep learning-based reconstruction: a reliability assessment in preoperative magnetic resonance imaging for primary rectal cancer
Introduction
In recent years, the incidence of colorectal cancer has been steadily increasing, making it the third most common malignancy worldwide after breast and lung cancers, and the second leading cause of cancer-related death, following lung cancer (1-3). Therefore, standardized clinical screening, diagnosis, and treatment are crucial for improving patient survival rates and quality of life.
The current treatment options for rectal cancer include endoscopic local excision and radical surgical resection, combined with neoadjuvant therapy. The effectiveness of these treatments depends on the comprehensiveness and accuracy of the preoperative assessment. In the preoperative evaluation of rectal cancer, transrectal ultrasound, computed tomography, and magnetic resonance imaging (MRI) are among the most widely used imaging techniques. MRI, with its high image quality, provides detailed and comprehensive information, making it an essential tool in the diagnosis and staging of rectal cancer. High-resolution MRI (HR-MRI), particularly T2-weighted imaging (T2WI), is currently the primary method for diagnosis and staging. It can accurately assess tumor location and morphology, perform T and N staging, and evaluate prognosis-related factors such as peritoneal reflection involvement, extramural vascular invasion (EMVI) status, and circumferential resection margin (CRM) status. Thus, MRI is a crucial aspect of the comprehensive management of rectal cancer patients (4-6).
Since the introduction of HR-MRI to evaluate rectal cancer, reports and pathological evidence have shown significant improvements in image quality, which may contribute to the improvement of diagnostic accuracy (4,6-8). However, to obtain high-quality images, longer acquisition times are required, which introduces unavoidable motion artifacts, including those from breathing and intestinal peristalsis. This poses significant challenges, as the demand for high-quality imaging increases (2). Over the past few decades, certain imaging techniques have been applied clinically, resulting in considerable improvements in image quality. However, all MRI undersampling techniques eventually reach their performance limits, as conventional reconstruction (ConR) methods cannot fully recover undersampled data (9,10). Parallel acquisition technology (PAT) and compressed sensing (CS) are two traditional undersampling methods. PAT has a signal-to-noise ratio (SNR) loss proportional to the PAT factor increase, while CS often results in excessive image smoothing. Both PAT and CS inherently suffer from unavoidable SNR loss and residual artifacts, leading to image blurring and unrealistic image texture. However, deep learning (DL) can enhance image quality without additional scanning time. Deep learning reconstruction (DLR) based on MRI aims to reconstruct high-quality images from k-space data using deep neural networks. It is based on well-trained deep convolutional neural networks, which are trained on curated datasets that include over 10,000 images, and over 4 million unique image-augmentation combinations for added robustness (11,12). The advantage of DL lies in its ability to generate more detailed images by learning a large number of model parameters.
Current research on DLR primarily focuses on optimizing model parameters using example data, and then deploying these models in scanners for expected data. Given its breakthrough performance in various complex tasks, particularly in image analysis, where it can extract valuable information from clinical images, DLR has rapidly advanced in radiology. Research has shown that DLR has better image quality and fewer artifacts than ConR (13). However, DLR can introduce instability, such as masking minor pathological findings or distorting structural features (14). Some studies have shown the potential of DLR methods to improve image quality (9,12,15-20), but research and literature on the use of DLR in the preoperative diagnosis of rectal cancer in clinical settings remain relatively scarce.
Therefore, this study aimed to evaluate and compare the effectiveness of the DLR and ConR methods in the preoperative evaluation of primary rectal cancer, focusing on both the objective and subjective image quality, and the diagnostic performance of these methods. We present this article in accordance with the STROBE reporting checklist (available at https://qims.amegroups.com/article/view/10.21037/qims-24-907/rc).
Methods
Data sets
This retrospective study was conducted in accordance with the Declaration of Helsinki (as revised in 2013). The study was approved by the Ethics Committee of Ruijin Hospital, Shanghai Jiao Tong University of Medicine (No. 2016-89), and the requirement of individual consent for this retrospective analysis was waived. The participants comprised patients diagnosed with primary rectal cancer by endoscopic biopsy, who underwent rectal MRI at Ruijin Hospital between March 2022 and October 2022. The magnetic resonance (MR) examination was performed less than 2 weeks before surgery. To be eligible for inclusion in this study, the patients had to meet the following inclusion criteria: have rectal adenocarcinoma confirmed by pathology, have surgical specimens, and have an interval between the biopsy and MR imaging of 3 days or more. Initially, 167 patients who met the inclusion criteria were identified for inclusion in the study. Patients were excluded from the study if they met any of the following exclusion criteria: (I) had received any adjuvant treatment before surgery; (II) had images that were not sufficient to fully show the lesions and draw accurate regions of interest (ROIs) due to motion or sensitivity artifacts; and/or (III) had incomplete DL MRI image data. Ultimately, 61 patients (mean age: 65±12 years; range: 34–90 years) were included in our study, of whom 38 were men and 23 were women (Figure 1). Among the final population, 41 patients were staged as tumour (T)3–4/node (N)1–2M0 in the preoperative radiological reports; however, they still underwent radical surgery based on multi-disciplinary team discussions and patient preferences.
DLR
A commercially available DLR algorithm (AIR Recon DL; GE Healthcare, USA) was used in this study. It is a Food and Drug Administration approved technique that reconstructs the acquired raw k-space data and complex valued data into images using a deep convolutional neural network (11). It was trained on a database of over 4 million image/augmentation combinations consisting of high-resolution, minimal ringing artifacts, high SNR images, and corresponding lower resolution, more ringing, lower SNR images. As a result, it can improve the image SNR and contrast. It has the following three adjustable noise reduction levels: DLR-H: 75% noise reduction; DLR-M: 50% noise reduction; and DLR-L: 25% noise reduction (11).
Imaging protocol
All patients underwent a standard preoperative MRI examination using a 3.0T MR scanner (SIGNA Architect, GE Healthcare, USA) with a 32-channel flexible coil. Before the MR scan, each patient fasted for 4 hours and underwent a 30-mL rectal enema (glycerol enema, Shanghai Xiaofang Pharmaceutical Co., LTD, China) 2 hours beforehand to achieve better contrast between the tumor and the rectal lumen (21). All examinations adhered to the standard protocols of our hospital. The imaging parameters are provided in Table 1. Sagittal T2-weighted (T2W) turbo spin-echo (TES) sequences without fat saturation were acquired, along with oblique axial and coronal images. The orientation of the oblique axial images was perpendicular to the bowel wall at the deepest point of tumor invasion. The oblique axial plane was selected for DLR. Routine T2WI were acquired in 2 minutes and 17 seconds using the Auto-calibrating Reconstruction for Cartesian sampling (ARC, the GE MRI scanner refers to GRAPPA as ARC) acceleration technique with a factor of 2, specific to the GE MRI scanner. The images were reconstructed using the DLR method at three noise reduction levels (DLR-H, DLR-M, and DLR-L) and the ConR method.
Table 1
Parameters | Oblique axial T2WI |
---|---|
TR/TE, ms/ms | 7,559/180 |
Number of slices | 32 |
Slice thickness, mm | 3 |
Spacing, mm | 0.6 |
FOV, mm | 240×240 |
Matrix size | 420×300 |
Voxel size, mm | 0.6×0.8×3.0 |
Number of averages | 2 |
Echo train length | 60 |
Acceleration factor | 2 |
Scan time | 2 min 17 s |
T2WI, T2-weighted imaging; TR/TE, repetition time/echo time; FOV, field of view.
Imaging assessment
The assessment included an objective assessment of image quality, a subjective assessment of quality, and an assessment of diagnostic performance.
Objective image quality assessment
The quantitative evaluation was conducted using a commercial post-processing workstation (AW VolumeShare 7, GE Healthcare). The objective assessment included the measurement of the SNR and contrast-to-noise ratio (CNR). In this study, the SNR was calculated as the ratio of the mean signal intensity in the tumor ROI (Stumor) to the standard deviation (SD) of background noise (SDbackground), as expressed in the following equation:
The CNR was defined as the ratio of the difference in signal intensity between two tissues to the SD of those tissues. In this study, the CNR was calculated as the mean signal intensity difference between tumor (Stumor) and normal tissue (Stissue) divided by the SD of the tumor (SDtumor) and normal tissue (SDtissue). The CNR values between the tumor and five normal tissues were calculated using the following equation:
All ROIs were systematically drawn on the ConR image set, and then copied to the other image sets. The ROIs were delineated using the single-section method, with one ROI outlining the tumor on a single section. A single freehand ROI was defined by drawing a line along the edge of the perceived tumor in the section containing the largest tumor region. The mean signal intensity and its SD were denoted as Stumor and SDtumor, respectively. Similarly, circular or oval ROIs were placed in homogeneous normal tissue located in the same section but away from the tumor area, and the mean signal intensity and SD were recorded as Stissue and SDtissue, respectively. Additionally, a single circular ROI was positioned in a non-signal background, and its SD was recorded as SDbackground. To ensure consistency and rigor in ROI delineation, all ROIs in one patient’s images were copied from the same image. The tissues for which the CNR was computed included the gluteus, subcutaneous fat, femur, pubis, and iliopsoas muscle (Figure 2).
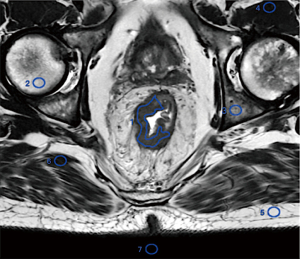
Subjective image quality assessment
All identified markers were removed. The images were anonymized and numbered to conceal both the sequence and patient information by K.W., who was the only individual with access to the clinical information corresponding to each number. The anonymized images were independently evaluated by two radiologists, one with 2 years of experience in rectum imaging, and the other with 7 years of experience. The two radiologists underwent adaptive training for evaluating DLR images one month prior to the image assessment. The images used for this training were sourced from patients not included in the study.
Imaging studies were assessed for the following parameters using a Likert scale ranging from 1 to 4, on which 4 indicated the highest quality. An example is provided in Figure 3. The subjective image quality total score was obtained by summing the following values:
- Image quality: 1= non-diagnostic; 2= poor image quality; 3= good image quality; 4= excellent image quality.
- Diagnostic confidence: 1= non-diagnostic; 2= severely limited confidence, repetition of examination recommended; 3= good confidence; 4= very good confidence.
- Noise levels: 1= a great deal of noise, severely hampering readability; 2= some noise, slightly hampering readability; 3= slight noise, not hampering readability; 4= no noise.
- Artifacts: 1= excessive artifacts distorting images; 2= artifacts hampering readability; 3= some artifacts limiting readability; 4= no artifacts.
- Sharpness of images: 1= severely blurred edges; 2= burred edges hampering readability; 3= slightly blurred edges; 4= no blurring.
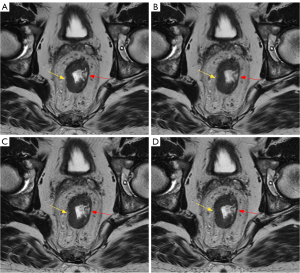
Diagnostic performance assessment
For T staging, according to the criteria of the American Joint Committee on Cancer Staging Eighth Edition of the Rectal Cancer Staging Manual, the two radiologists performed T staging for all cases. Histopathological T staging is the gold standard for T staging. Due to the difficulty in correlating individual lymph node metastasis with MRI imaging, a consistency check for N staging was also conducted. For the other prognostic factors (e.g., CRM and EMVI status), we also assessed consistency due to the lack of a pathological gold standard.
In terms of T staging, carcinoma growing in the submucosa layer was staged as T1; carcinoma extending into but contained in the muscularis propria was staged as T2; carcinoma extending into the subserosa and/or perirectal tissue was staged as T3; and carcinoma invading the surface of visceral peritoneum or other organs/structures was staged as T4. The perilesional anatomical structures were also evaluated to assess image quality.
Lymph nodes were classified as metastatic if they met the following criteria on the T2WI images: had a maximum short-axis diameter >9 mm; had a maximum short-axis diameter of 5–9 mm and met any two of the following morphological criteria: irregular shape, indistinct margins, or inhomogeneous signal; had a maximum short-axis diameter <5 mm, met all three morphological criteria, and had increased signal in diffusion-weighted sequences.
The CRM status of the patients was also assessed. CRM is a surgical concept that refers to the shortest distance between the deepest point of tumor invasion and the boundary of the mesorectal excision. CRM positivity serves as a predictor of local recurrence and poor prognosis. In the MRI evaluation, the status of mesorectal fascia involvement represents CRM status. If the distance between the tumor tissue or affected lymph nodes or involved blood vessels to the mesorectal fascia measured <1 mm, it was considered positive; if it measured between 1–2 mm, it was considered suspicious; and if it measured >2 mm, it was considered negative.
EMVI status refers to the presence of EMVI by the tumor, and is typically observed in T3 and above stages. The scoring criteria for EMVI were as follows:
- 0 points: no penetration of the intestinal wall by the mass, and no visible extramural blood vessels in the corresponding area.
- 1 point: minimal EMVI or penetration of the intestinal wall by the mass, but no visible extramural blood vessels in the corresponding area.
- 2 points: penetration or non-penetration of the intestinal wall by the mass, with visible extramural blood vessels in the corresponding area, but no nodular, streaky, or irregularly shaped tumor densities within the vessel lumen.
- 3 points: nodular or linear extension of the mass into the extramural vessel lumen after penetrating the intestinal wall, with mild dilation of the involved extramural blood vessel diameter.
- 4 points: presence of tumor signal in surrounding blood vessels with enlarged lumens and irregular shapes, sometimes appearing as nodular expansions.
In EMVI status scoring, scores of 2 and below are considered negative while scores of 3 and above are considered positive.
Statistical analysis
Comparisons of subjective image quality analysis scores were conducted using the Friedman test, and the additional P values for all pairwise comparisons were calculated using the Dunn-Bonferroni post-hoc test. This test was used to compare the differences in objective image quality parameters (the SNR and CNR) between the ConR and DLR images. Chi-square tests were used to analyze differences in T and N staging among the four different reconstruction methods. Weighted kappa coefficients were used to assess the subjective image quality parameters, diagnostic performance, and consistency between the preoperative MR and histopathological T staging for inter-observer variability. The Kappa test was used to analyze the consistency of the subjective quality parameters. The interclass correlation coefficient (ICC) test was used to evaluate the between-observer variability of the objective image quality parameters. The ICC and k values were categorized as follows: poor agreement (0.00–0.20), fair agreement (0.21–0.40), moderate agreement (0.41–0.60), good agreement (0.61–0.80), and excellent agreement (0.81–1.00). The statistical analysis was performed using statistical software (SPSS 26.0, SPSS, IBM). A two-sided P value <0.05 was considered statistically significant.
Results
Patient characteristics
A total of 61 patients (38 men and 23 women) were included in the final analysis (Figure 1). The patients had a mean age of 65±12 years (range: 34–90 years). Among them, 29 patients had mid-rectal cancer, 13 had upper rectal cancer, and 19 had lower rectal cancer. In relation to the pathological stage, 6 (9.84%) were classified as pT1, 14 (22.95%) as pT2, 33 (54.10%) as pT3, and 8 (13.11%) as pT4.
Performance of objective assessment
The results consisted of the SNR of the tumor versus the background, and the CNR of each tissue was calculated in relation to the tumor. The objective evaluation results, which are summarized in Table 2, revealed significant differences in the SNR (P<0.001) and CNR (P≤0.040) when comparing the DLR images at various noise reduction levels to the ConR images using a pairwise comparison analysis. Additionally, in terms of the DLR method, significant differences in the SNR and CNR were observed for DLR-H, DLR-M, and DLR-L, of which, DLR-H had the best SNR and CNR (P≤0.001).
Table 2
Parameters | DLR-H | DLR-M | DLR-L | ConR | P value | ||||||
---|---|---|---|---|---|---|---|---|---|---|---|
Overall | Pairwise | ||||||||||
1 vs. 2 | 1 vs. 3 | 1 vs. 4 | 2 vs. 3 | 2 vs. 4 | 3 vs. 4 | ||||||
SNR | 142.172±31.302 | 77.573±17.917 | 59.175±14.113 | 41.557±9.114 | <0.001 | <0.001 | <0.001 | <0.001 | <0.001 | <0.001 | <0.001 |
CNR | |||||||||||
Subcutaneous fat | 9.666±3.435 | 8.445±3.159 | 7.738±2.498 | 7.520±2.406 | <0.001 | <0.001 | <0.001 | 0.033 | <0.001 | <0.001 | 0.04 |
Gluteus | 4.606±1.888 | 3.739±1.483 | 3.429±1.291 | 3.054±1.059 | <0.001 | <0.001 | <0.001 | 0.001 | 0.001 | <0.001 | 0.026 |
Femur | 8.439±3.018 | 6.898±2.624 | 6.127±2.089 | 5.810±2.044 | <0.001 | <0.001 | <0.001 | 0.035 | <0.001 | <0.001 | 0.008 |
Iliopsoas muscle | 4.261±1.655 | 3.502±1.307 | 3.091±1.104 | 2.784±0.893 | <0.001 | <0.001 | <0.001 | 0.005 | <0.001 | <0.001 | 0.016 |
Pubis | 6.546±3.416 | 5.349±3.102 | 4.792±2.530 | 4.463±2.545 | <0.001 | <0.001 | <0.001 | 0.003 | <0.001 | <0.001 | 0.028 |
Data are expressed as mean ± standard deviation. The SNR is calculated between the signal of the tumor and SD of the background, and the CNR of each tissue is calculated in relation to the tumor. The overall comparison’s P value is given using the Friedman test. If the Friedman test revealed a statistically significant P value, additional P values for all pairwise comparisons were provided using the Dunn-Bonferroni post-hoc test. A P value <0.05 was considered statistically significant. SNR, signal-to-noise ratio; CNR, contrast-to-noise ratio; ConR, routine T2-weighted imaging reconstructed with conventional reconstruction; DLR-H, routine T2-weighted imaging reconstructed with the deep learning reconstruction method at the 75% noise reduction level; DLR-M, routine T2-weighted imaging reconstructed with the deep learning reconstruction method at the 50% noise reduction level; DLR-L, routine T2-weighted imaging reconstructed with the deep learning reconstruction method at the 25% noise reduction level; SD, standard deviation.
Performance of subjective assessment
The results of the subjective comparisons of the image quality parameters based on the 4-point rating system are presented in Table 3. Both evaluators agreed that DLR-H and DLR-M provided a significant advantage over ConR in terms of the overall image quality scores (DLR-H and DLR-M vs. ConR, R1: 20 [16, 20]/R2: 20 [15, 20] and 19 [14, 20] vs. 17 [10, 19], all P<0.001). However, there was no significant difference between DLR-L and ConR [Reader 1 (R1), P=0.806; Reader 2 (R2), P>0.999; DLR-L]. In relation to the specific parameters, both evaluators found no difference between the DLR and ConR images in terms of artifacts (R1, overall P=0.195; R2, P=0.099). Additionally, both evaluators observed that the DLR-H and DLR-M images outperformed the ConR images in four other parameters (R1, P≤0.045; R2, P≤0.002), but found no significant difference between the DLR-L and ConR images (R1, P≥0.325; R2, P>0.999).
Table 3
Parameters | DLR-H | DLR-M | DLR-L | ConR | P value | ||||||
---|---|---|---|---|---|---|---|---|---|---|---|
Overall | Pairwise | ||||||||||
1 vs. 2 | 1 vs. 3 | 1 vs. 4 | 2 vs. 3 | 2 vs. 4 | 3 vs. 4 | ||||||
Image quality | |||||||||||
R1 | 4 [3, 4] | 4 [2, 4] | 3 [2, 4] | 3 [2, 4] | <0.001 | >0.999 | <0.001 | <0.001 | <0.001 | <0.001 | 0.325 |
R2 | 4 [3, 4] | 4 [2, 4] | 3 [2, 4] | 3 [2, 4] | <0.001 | >0.999 | <0.001 | <0.001 | <0.001 | <0.001 | >0.999 |
Diagnostic confidence | |||||||||||
R1 | 4 [3, 4] | 4 [3, 4] | 4 [2, 4] | 4 [2, 4] | <0.001 | >0.999 | 0.055 | 0.015 | 0.148 | 0.045 | >0.999 |
R2 | 4 [3, 4] | 4 [3, 4] | 4 [2, 4] | 4 [2, 4] | <0.001 | >0.999 | 0.01 | 0.001 | 0.026 | 0.002 | >0.999 |
Noise | |||||||||||
R1 | 4 [3, 4] | 4 [3, 4] | 3 [2, 4] | 3 [2, 4] | <0.001 | 0.004 | <0.001 | <0.001 | 0.04 | 0.006 | >0.999 |
R2 | 4 [3, 4] | 4 [3, 4] | 3 [2, 4] | 3 [2, 4] | <0.001 | 0.04 | <0.001 | <0.001 | 0.01 | 0.002 | >0.999 |
Artifacts | |||||||||||
R1 | 4 [3, 4] | 4 [3, 4] | 4 [3, 4] | 4 [2, 4] | 0.195 | ||||||
R2 | 4 [3, 4] | 4 [2, 4] | 4 [2, 4] | 4 [2, 4] | 0.099 | ||||||
Sharpness | |||||||||||
R1 | 4 [3, 4] | 4 [3, 4] | 3 [2, 4] | 3 [2, 4] | <0.001 | 0.522 | <0.001 | <0.001 | <0.001 | <0.001 | >0.999 |
R2 | 4 [3, 4] | 4 [3, 4] | 3 [2, 4] | 3 [2, 4] | <0.001 | >0.999 | <0.001 | <0.001 | <0.001 | <0.001 | >0.999 |
Total | |||||||||||
R1 | 20 [16, 20] | 19 [14, 20] | 17 [11, 20] | 17 [10, 19] | <0.001 | 0.075 | <0.001 | <0.001 | <0.001 | <0.001 | 0.806 |
R2 | 20 [15, 20] | 19 [14, 20] | 17 [10, 20] | 17 [10, 19] | <0.001 | 0.448 | <0.001 | <0.001 | <0.001 | <0.001 | >0.999 |
Subjective image quality scores for each parameter are presented as median [minimum, maximum]. The overall comparison’s P value is given using the Friedman test. If the Friedman test revealed a statistically significant P value, additional P values for all pairwise comparisons were provided using the Dunn-Bonferroni post-hoc test. A P value <0.05 was considered statistically significant. R1, Reader 1; R2, Reader 2; DLR-H, routine T2-weighted imaging reconstructed with the deep learning reconstruction method at the 75% noise reduction level; DLR-M, routine T2-weighted imaging reconstructed with the deep learning reconstruction method at the 50% noise reduction level; DLR-L, routine T2-weighted imaging reconstructed with the deep learning reconstruction method at the 25% noise reduction level; ConR, routine T2-weighted imaging reconstructed with conventional reconstruction.
Across the three levels of noise reduction in the DLR method, both evaluators agreed that there was no statistically significant difference in the overall image quality scores between the DLR-H and DLR-M images (R1, P=0.075; R2, P=0.448). However, there was a significant difference between the DLR-L images compared to the DLR-H and DLR-M images (P<0.001). No difference was found in terms of the artifacts among the specific parameters. Significant differences were observed in the image quality, noise, and sharpness between the DLR-L and DLR-H images (all P<0.001), and the DLR-M images (R1, P≤0.040; R2, P≤0.010). In terms of diagnostic confidence, there was no significant difference between the DLR-L and DLR-H images (P=0.055), or the DLR-M images (P=0.148) in terms of R1, but there was a significant difference in terms of R2 (P=0.010, P=0.026). In relation to the comparison between the DLR-H and DLR-M images, significant results were only found in terms of noise (R1, P=0.004; R2, P=0.040).
A consistent evaluation of the evaluators was conducted (Table 4), and the agreement was substantial to perfect (kappa ≥0.711, P<0.001). Notably, the DLR-H images had the highest level of consistency in terms of noise (kappa =1.000, P<0.001).
Table 4
Parameters | DLR-H | DLR-M | DLR-L | ConR | P |
---|---|---|---|---|---|
Image quality | 0.829 | 0.777 | 0.769 | 0.923 | <0.001 |
Diagnostic confidence | 0.794 | 0.784 | 0.755 | 0.765 | <0.001 |
Noise levels | 1.000 | 0.754 | 0.711 | 0.793 | <0.001 |
Artifacts | 0.750 | 0.785 | 0.820 | 0.809 | <0.001 |
Sharpness of images | 0.807 | 0.823 | 0.880 | 0.858 | <0.001 |
Total | 0.921 | 0.916 | 0.874 | 0.947 | <0.001 |
Inter-reader consistency for subjective quality parameters of each series of images was compared using kappa coefficients. Consistency between observers was analyzed by calculating the kappa coefficient, where a kappa value of 0.01–0.20 indicates slight agreement, 0.21–0.40 indicates fair agreement, 0.41–0.60 indicates moderate agreement, 0.61–0.80 indicates substantial agreement, and 0.81–1.00 indicates almost perfect agreement. DLR-H, routine T2-weighted imaging reconstructed with the deep learning reconstruction method at the 75% noise reduction level; DLR-M, routine T2-weighted imaging reconstructed with the deep learning reconstruction method at the 50% noise reduction level; DLR-L, routine T2-weighted imaging reconstructed with the deep learning reconstruction method at the 25% noise reduction level; ConR, routine T2-weighted imaging reconstructed with conventional reconstruction.
Performance of diagnostic performance assessment
The accuracy, sensitivity, and specificity results for each T stage are presented in Table 5. There were varying degrees of difference among the groups of images; however, these differences were not statistically significant (R1, P=0.603; R2, P=0.206). The diagnostic results for T stage exhibited perfect consistency across all groups of images (R1, ICC =0.955, P<0.001; R2, ICC =0.861, P<0.001). The consistency between the two evaluators was substantial to perfect in all groups of images (kappa ≥0.678, P<0.001), while the DLR-H images had the best inter-observer consistency (kappa =0.848, P<0.001).
Table 5
Groups | T1 | T2 | T3 | T4 | Kappa | P | |||||||
---|---|---|---|---|---|---|---|---|---|---|---|---|---|
R1 | R2 | R1 | R2 | R1 | R2 | R1 | R2 | ||||||
DLR-H | 0.848 | <0.001 | |||||||||||
pT1 (n=6) | 4 | 4 | 2 | 2 | 0 | 0 | 0 | 0 | |||||
pT2 (n=14) | 0 | 0 | 11 | 11 | 3 | 3 | 0 | 0 | |||||
pT3 (n=33) | 0 | 0 | 0 | 0 | 27 | 26 | 6 | 7 | |||||
pT4 (n=8) | 0 | 0 | 0 | 0 | 1 | 1 | 7 | 7 | |||||
Accuracy | 0.967 | 0.967 | 0.918 | 0.918 | 0.836 | 0.820 | 0.885 | 0.869 | |||||
Sensitivity | 1.000 | 1.000 | 0.846 | 0.846 | 0.871 | 0.867 | 0.538 | 0.500 | |||||
Specificity | 0.965 | 0.965 | 0.938 | 0.938 | 0.800 | 0.774 | 0.979 | 0.979 | |||||
DLR-M | 0.802 | <0.001 | |||||||||||
pT1 (n=6) | 4 | 6 | 2 | 0 | 0 | 0 | 0 | 0 | |||||
pT2 (n=14) | 0 | 0 | 11 | 11 | 3 | 3 | 0 | 0 | |||||
pT3 (n=33) | 0 | 0 | 0 | 1 | 26 | 25 | 7 | 7 | |||||
pT4 (n=8) | 0 | 0 | 0 | 0 | 1 | 1 | 7 | 7 | |||||
Accuracy | 0.967 | 0.967 | 0.918 | 0.918 | 0.820 | 0.803 | 0.869 | 0.869 | |||||
Sensitivity | 1.000 | 1.000 | 0.846 | 0.846 | 0.867 | 0.862 | 0.500 | 0.500 | |||||
Specificity | 0.965 | 0.965 | 0.938 | 0.938 | 0.774 | 0.750 | 0.979 | 0.979 | |||||
DLR-L | 0.678 | <0.001 | |||||||||||
pT1 (n=6) | 4 | 3 | 2 | 2 | 0 | 1 | 0 | 0 | |||||
pT2 (n=14) | 0 | 0 | 9 | 10 | 5 | 4 | 0 | 0 | |||||
pT3 (n=33) | 0 | 0 | 2 | 3 | 24 | 21 | 7 | 9 | |||||
pT4 (n=8) | 0 | 0 | 0 | 0 | 1 | 2 | 7 | 6 | |||||
Accuracy | 0.967 | 0.967 | 0.852 | 0.918 | 0.754 | 0.689 | 0.869 | 0.820 | |||||
Sensitivity | 1.000 | 1.000 | 0.692 | 0.846 | 0.800 | 0.750 | 0.500 | 0.400 | |||||
Specificity | 0.965 | 0.965 | 0.896 | 0.938 | 0.710 | 0.636 | 0.979 | 0.957 | |||||
ConR | 0.753 | <0.001 | |||||||||||
pT1 (n=6) | 4 | 4 | 2 | 1 | 0 | 1 | 0 | 0 | |||||
pT2 (n=14) | 0 | 0 | 10 | 11 | 4 | 3 | 0 | 0 | |||||
pT3 (n=33) | 0 | 0 | 1 | 2 | 24 | 22 | 8 | 9 | |||||
pT4 (n=8) | 0 | 0 | 0 | 0 | 2 | 2 | 6 | 6 | |||||
Accuracy | 0.967 | 0.967 | 0.885 | 0.918 | 0.754 | 0.721 | 0.836 | 0.820 | |||||
Sensitivity | 1.000 | 1.000 | 0.769 | 0.846 | 0.800 | 0.786 | 0.429 | 0.400 | |||||
Specificity | 0.965 | 0.965 | 0.917 | 0.938 | 0.710 | 0.667 | 0.957 | 0.957 | |||||
Overall | R1, P=0.603; ICC =0.955, P<0.001; R2, P=0.206; ICC =0.861, P<0.001 |
The Kappa values and P values on the right side of the table represent inter-observer consistency for T staging. The P values at the bottom of the table indicate that there is no difference in the overall diagnostic staging results among the four series, with ICC representing consistency. A Kappa/ICC value of 0.01–0.20 indicates slight consistency, 0.21–0.40 indicates fair consistency, 0.41–0.60 indicates moderate consistency, 0.61–0.80 indicates substantial consistency, and 0.81–1.00 indicates perfect consistency. T1, T stage 1; T2, T stage 2; T3, T stage 3; T4, T stage 4; R1, Reader 1; R2, Reader 2; DLR-H, routine T2-weighted imaging reconstructed with the deep learning reconstruction method at the 75% noise reduction level; DLR-M, routine T2-weighted imaging reconstructed with the deep learning reconstruction method at the 50% noise reduction level; DLR-L, routine T2-weighted imaging reconstructed with the deep learning reconstruction method at the 25% noise reduction level; ConR, routine T2-weighted imaging reconstructed with conventional reconstruction.
In terms of specific stages, the accuracy rates were consistent across all sequences for both evaluators for T1 staging (0.967). However, there was a discrepancy in the T2 staging accuracy between the DLR-L (R1 vs. R2: 0.852 vs. 0.918) and ConR (R1 vs. R2: 0.885 vs. 0.918) images. When evaluating the T3 and T4 stages, noticeable differences in accuracy between the evaluators were observed across all groups, except for the T4 stage with the DLR-M images (0.869). However, these differences did not reach the level of statistical significance.
The accuracy, sensitivity, and specificity results for N stage are presented in Table 6. The differences were not found to be statistically significant (R1, P=0.990; R2, P=0.884). Overall, there was substantial to perfect consistency between the images of different groups (R1, ICC =0.798, P<0.001; R2, ICC =0.867, P<0.001). The results indicated perfect inter-rater consistency (kappa ≥0.826, P<0.001).
Table 6
Groups | N0 | N1 | N2 | Kappa | P | |||||
---|---|---|---|---|---|---|---|---|---|---|
R1 | R2 | R1 | R2 | R1 | R2 | |||||
DLR-H | 0.893 | <0.001 | ||||||||
pN0 (n=40) | 37 | 36 | 3 | 3 | 0 | 1 | ||||
pN1 (n=16) | 5 | 6 | 11 | 10 | 0 | 0 | ||||
pN2 (n=5) | 0 | 0 | 2 | 2 | 3 | 3 | ||||
Accuracy | 0.869 | 0.836 | 0.836 | 0.820 | 0.967 | 0.951 | ||||
Sensitivity | 0.881 | 0.857 | 0.688 | 0.667 | 1.000 | 0.750 | ||||
Specificity | 0.842 | 0.789 | 0.889 | 0.870 | 0.966 | 0.965 | ||||
DLR-M | 0.826 | <0.001 | ||||||||
pN0 (n=40) | 35 | 33 | 5 | 6 | 0 | 1 | ||||
pN1 (n=16) | 5 | 6 | 11 | 10 | 0 | 0 | ||||
pN2 (n=5) | 0 | 0 | 2 | 2 | 3 | 3 | ||||
Accuracy | 0.852 | 0.820 | 0.820 | 0.803 | 0.967 | 0.951 | ||||
Sensitivity | 0.878 | 0.854 | 0.647 | 0.625 | 1.000 | 0.750 | ||||
Specificity | 0.800 | 0.750 | 0.886 | 0.867 | 0.966 | 0.965 | ||||
DLR-L | 0.866 | <0.001 | ||||||||
pN0 (n=40) | 35 | 33 | 5 | 6 | 0 | 1 | ||||
pN1 (n=16) | 5 | 6 | 11 | 10 | 0 | 0 | ||||
pN2 (n=5) | 0 | 0 | 2 | 2 | 3 | 3 | ||||
Accuracy | 0.836 | 0.787 | 0.803 | 0.770 | 0.967 | 0.951 | ||||
Sensitivity | 0.875 | 0.846 | 0.611 | 0.556 | 1.000 | 0.750 | ||||
Specificity | 0.762 | 0.682 | 0.884 | 0.860 | 0.966 | 0.965 | ||||
ConR | 0.899 | <0.001 | ||||||||
pN0 (n=40) | 32 | 35 | 6 | 4 | 0 | 1 | ||||
pN1 (n=16) | 5 | 6 | 11 | 10 | 0 | 0 | ||||
pN2 (n=5) | 0 | 0 | 2 | 2 | 3 | 3 | ||||
Accuracy | 0.820 | 0.836 | 0.787 | 0.803 | 0.967 | 0.951 | ||||
Sensitivity | 0.872 | 0.875 | 0.579 | 0.625 | 1.000 | 0.750 | ||||
Specificity | 0.727 | 0.762 | 0.881 | 0.867 | 0.966 | 0.965 | ||||
Overall | R1, P=0.990, ICC =0.798, P<0.001; R2, P=0.884, ICC =0.867, P<0.001 |
The Kappa values and P values on the right side of the table represent inter-observer consistency for T staging. The P values at the bottom of the table indicate that there is no difference in the overall diagnostic staging results among the four series, with ICC representing consistency. A Kappa/ICC value of 0.01–0.20 indicates slight consistency, 0.21–0.40 indicates fair consistency, 0.41–0.60 indicates moderate consistency, 0.61–0.80 indicates substantial consistency, and 0.81–1.00 indicates almost perfect consistency. N0, suspected lymph node stage 0; N1, suspected lymph node stage 1; N2, suspected lymph node stage 2; R1, Reader 1; R2, Reader 2; DLR-H, routine T2-weighted imaging reconstructed with the deep learning reconstruction method at the 75% noise reduction level; DLR-M, routine T2-weighted imaging reconstructed with the deep learning reconstruction method at the 50% noise reduction level; DLR-L, routine T2-weighted imaging reconstructed with the deep learning reconstruction method at the 25% noise reduction level; ConR, routine T2-weighted imaging reconstructed with conventional reconstruction; ICC, Intra-class correlation coefficient.
In relation to the N0 and N1 stages specifically, both evaluators agreed that the DLR-H level exhibited the highest accuracy among the three noise reduction levels (N0, R1 =0.869, R2 =0.836; N1, R1 =0.836, R2 =0.820). In terms of the N2 stage, there was no discrepancy in the accuracy of the evaluators across all sequences (R1 =0.967; R2 =0.951). However, these differences were not statistically significant.
Due to discrepancies in standards between the pathology results and MR evaluations, the assessment of CRM and EMVI was limited to analyzing the inter-observer and inter-sequence consistency (Table 7). The findings revealed substantial to perfect inter-observer consistency for CRM and EMVI (kappa ≥0.700, P<0.001). Moreover, the results revealed substantial to perfect consistency among the four groups for both the evaluators (ICC ≥0.737, <0.001) with no discernible variance in diagnostic outcomes across the four groups of images (P≥0.121).
Table 7
CRM | EMVI | P | |
---|---|---|---|
DLR-H | 0.887 | 0.777 | <0.001 |
DLR-M | 0.864 | 0.718 | <0.001 |
DLR-L | 0.777 | 0.757 | <0.001 |
ConR | 0.810 | 0.704 | <0.001 |
ICC | |||
R1 | 0.737 | 0.900 | <0.001 |
R2 | 0.764 | 0.867 | <0.001 |
Overall P | |||
R1 | 0.572 | 0.121 | |
R2 | 0.724 | 0.144 |
The table first presents the inter-observer consistency for each sequence. The following ICC indicates the consistency between four DWI sequences for each rater. The overall P values for the comparisons were calculated using the Friedman test. If the Friedman test revealed a statistically significant P value, additional P values for all paired comparisons were provided through the Dunn-Bonferroni post-hoc test. A P value <0.05 was considered statistically significant. A Kappa/ICC value of 0.01–0.20 indicates slight consistency, 0.21–0.40 indicates fair consistency, 0.41–0.60 indicates moderate consistency, 0.61–0.80 indicates substantial consistency, and 0.81–1.00 indicates almost perfect consistency. CRM, circumferential resection margin; EMVI, extramural vascular invasion; DLR-H, routine T2-weighted imaging reconstructed with the deep learning reconstruction method at the 75% noise reduction level; DLR-M, routine T2-weighted imaging reconstructed with the deep learning reconstruction method at the 50% noise reduction level; DLR-L, routine T2-weighted imaging reconstructed with the deep learning reconstruction method at the 25% noise reduction level; ConR, routine T2-weighted imaging reconstructed with conventional reconstruction; ICC, intra-class correlation coefficient; R1, Reader 1; R2, Reader 2; DWI, diffusion-weighted imaging.
For the specificity and sensitivity of diagnostic staging, while there were some apparent differences, the results were not statistically significant. Further, in both T and N staging, no statistically significant difference was found in the diagnostic performance at specific stages (Table 8).
Table 8
Stage | R1 | R2 |
---|---|---|
T1 | >0.999 | 0.223 |
T2 | 0.535 | 0.477 |
T3 | 0.557 | 0.260 |
T4 | 0.884 | 0.771 |
T1–2 | 0.604 | 0.833 |
T3–4 | 0.909 | 0.557 |
N0 | 0.891 | 0.884 |
N1 | 0.911 | 0.923 |
N2 | >0.999 | >0.999 |
The values in the table represent P values, indicating the results of comparisons of specific diagnostic staging among the three levels of DLR noise reduction and ConR images. A P value <0.05 was considered statistically significant. R1, Reader 1; R2, Reader 2; DLR, deep learning reconstruction; ConR, routine T2-weighted imaging reconstructed with conventional reconstruction.
Discussion
The effect of the DLR method, at different levels of noise reduction, on image quality and diagnostic effectiveness was analyzed via comparison with the ConR method. The objective evaluation results indicated that images obtained using DLR showed significantly improved image quality without requiring additional scanning time. There were significant differences among the four groups, among which the DLR-H group had the best image quality. Further, the CNR at different anatomical sites highlighted the advantage of DLR. The subjective evaluation results revealed that both evaluators found images produced using DLR-H and DLR-M to have higher image quality than those obtained using ConR, and to have significant benefits in terms of visual image quality, diagnostic confidence, noise reduction, and sharpness. The consistency between the subjective and objective evaluations supports these findings. The diagnostic effectiveness results indicated that the images acquired using DLR had comparable diagnostic efficacy to ConR. While there were differences in T and N staging, DLR was not found to have any statistically significant superior efficacy over ConR. Both the DLR and ConR images demonstrated excellent inter-evaluator and inter-group consistency in assessing CRM and EMVI.
The high level of agreement in the quality assessment indicates that the DLR images provided superior image details, along with significantly improved SNR and CNR results in the objective evaluation. Regardless of whether a subjective or objective analysis was used, DLR-H was found to be significantly better than ConR. In terms of image quality, we found that DLR, particularly DLR-H, produced considerable improvements in image quality and provided a more accurate representation of clear details. This is consistent with the findings of Gassenmaier et al., who applied DL to axial T2W TSE imaging of the prostate, and found that DLR enhanced image quality, lesion detectability, and diagnostic confidence (9). Similarly, Park et al.’s study on orthopedic MRI showed that DLR improved the quality of two-dimensional fast/TSE images (22).
In the assessment of diagnostic efficacy, the DLR images had comparable diagnostic effectiveness to the ConR images, but they did not show superior diagnostic efficacy. It is important to note that there were differences in accuracy between the two evaluators for certain T and N stages, but none of these differences were statistically significant. Considering the varying levels of experience of the evaluators and taking into account both the objective and subjective image quality assessments, it cannot be ruled out that DLR, particularly DLR-H or DLR-M, may enhance diagnostic efficacy, especially for junior radiologists. The present results showed that DLR, particularly DLR-H, improved the diagnostic confidence of junior radiologists. This could potentially lead to improved efficiency in clinical work.
Technical and parameter adjustments aimed at improving image quality often lead to longer scan times. However, Park et al. examined the effect of using shortened acquisition times under DLR on MRI quality and diagnostic performance in prostate cancer patients (22). Their findings suggested that DLR effectively reduced the MRI acquisition time without compromising the image quality or diagnostic performance. Similarly, Almansour et al. and Recht et al. arrived at similar conclusions (10,15). From a different perspective, our research indicated that DLR surpassed ConR in terms of image quality without requiring additional scan time, which aligns with previous findings that DLR has shorter scan times (10,15,22).
Our study had a number of limitations. First, the small sample size, inclusion of heterogeneous participants, and single-center design might limit the generalizability of the results. As recommended by the treatment guidelines, locally advanced rectal cancer patients undergoing neoadjuvant therapy were excluded from this study, which might have contributed to the small sample size. Larger sample size studies are needed for broader evaluation. Second, the accuracy of T staging was assessed using axial T2WI images; however, diffusion-weighted images are usually used for staging in traditional clinical practice. This might have led to discrepancies in diagnostic performance. Third, in comparison to K-space parallel amplitude resonance imaging based on DL, a method for reconstructing missing K-space data using micro U-nets can enhance image quality and achieve an optimal balance between network performance and training sample requirements. Xu et al. developed a small U-net model that uses DL to improve MRI speed and image quality. It reduces noise and artifacts better than traditional methods (23). This approach may represent a promising direction to further improve image quality. Finally, the experimental design did not take rapid scan sequences into account.
In summary, the DLR methods provided higher quality images with excellent diagnostic performance. The imaging sequences in the study used a number of averages of 2, suggesting that DLR could potentially shorten scan times by reducing the number of averages to 1 or even using half-Fourier acquisition, without compromising image quality. Faster scans would also offer substantial clinical benefits, such as reducing the impact of bowel movement, which is of great significance. Future research in this direction is planned.
Conclusions
The use of DLR, particularly DLR-H, in preoperative MRI scanning for primary rectal cancer has the potential to enhance image quality without requiring additional scan time. DLR achieves a new balance between scan time/resolution and the SNR/CNR, while maintaining comparable diagnostic effectiveness or potentially even improving it, thus providing an alternative to ConR. Additionally, DLR shows promise in reducing the scan time.
Acknowledgments
We used a Large Language Model to help us correct grammar errors and improve expression. We did not use the Large Language Model to write the article; it was solely used to correct grammatical errors and help us express our ideas more accurately.
Funding: This work was supported by funding from
Footnote
Reporting Checklist: The authors have completed the STROBE reporting checklist. Available at https://qims.amegroups.com/article/view/10.21037/qims-24-907/rc
Conflicts of Interest: All authors have completed the ICMJE uniform disclosure form (available at https://qims.amegroups.com/article/view/10.21037/qims-24-907/coif). J.D., an employee of GE Healthcare who has a partnership with the hospital, provided technical support for the study. The other authors have no conflicts of interest to declare.
Ethical Statement: The authors are accountable for all aspects of the work in ensuring that questions related to the accuracy or integrity of any part of the work are appropriately investigated and resolved. The study was conducted in accordance with the Declaration of Helsinki (as revised in 2013). The study was approved by the Ethics Committee of Ruijin Hospital, Shanghai Jiao Tong University of Medicine (No. 2016-89), and the requirement of individual consent for this retrospective analysis was waived.
Open Access Statement: This is an Open Access article distributed in accordance with the Creative Commons Attribution-NonCommercial-NoDerivs 4.0 International License (CC BY-NC-ND 4.0), which permits the non-commercial replication and distribution of the article with the strict proviso that no changes or edits are made and the original work is properly cited (including links to both the formal publication through the relevant DOI and the license). See: https://creativecommons.org/licenses/by-nc-nd/4.0/.
References
- Sung H, Ferlay J, Siegel RL, Laversanne M, Soerjomataram I, Jemal A, Bray F. Global Cancer Statistics 2020: GLOBOCAN Estimates of Incidence and Mortality Worldwide for 36 Cancers in 185 Countries. CA Cancer J Clin 2021;71:209-49. [Crossref] [PubMed]
- Yang Y, Wang HY, Chen YK, Chen JJ, Song C, Gu J. Current status of surgical treatment of rectal cancer in China. Chin Med J (Engl) 2020;133:2703-11. [Crossref] [PubMed]
- Crimì F, Lacognata C, Cecchin D, Zucchetta P, Pomerri F. Rectal cancer staging: An up-to-date pictorial review. J Med Imaging Radiat Oncol 2018; Epub ahead of print. [Crossref]
- Bates DDB, Homsi ME, Chang KJ, Lalwani N, Horvat N, Sheedy SP. MRI for Rectal Cancer: Staging, mrCRM, EMVI, Lymph Node Staging and Post-Treatment Response. Clin Colorectal Cancer 2022;21:10-8. [Crossref] [PubMed]
- Ajani JA, D'Amico TA, Bentrem DJ, Chao J, Cooke D, Corvera C, et al. Gastric Cancer, Version 2.2022, NCCN Clinical Practice Guidelines in Oncology. J Natl Compr Canc Netw 2022;20:167-92. [Crossref] [PubMed]
- Horvat N, Carlos Tavares Rocha C, Clemente Oliveira B, Petkovska I, Gollub MJ. MRI of Rectal Cancer: Tumor Staging, Imaging Techniques, and Management. Radiographics 2019;39:367-87. [Crossref] [PubMed]
- Zhang S, Chen F, Ma X, Wang M, Yu G, Shen F, Gao X, Lu J. MRI-based nomogram analysis: recognition of anterior peritoneal reflection and its relationship to rectal cancers. BMC Med Imaging 2021;21:50. [Crossref] [PubMed]
- Curvo-Semedo L. Rectal Cancer: Staging. Magn Reson Imaging Clin N Am 2020;28:105-15. [Crossref] [PubMed]
- Gassenmaier S, Afat S, Nickel D, Mostapha M, Herrmann J, Othman AE. Deep learning-accelerated T2-weighted imaging of the prostate: Reduction of acquisition time and improvement of image quality. Eur J Radiol 2021;137:109600. [Crossref] [PubMed]
- Recht MP, Zbontar J, Sodickson DK, Knoll F, Yakubova N, Sriram A, et al. Using Deep Learning to Accelerate Knee MRI at 3 T: Results of an Interchangeability Study. AJR Am J Roentgenol 2020;215:1421-9. [Crossref] [PubMed]
.Lebel RM Performance characterization of a novel deep learning-based MR image reconstruction pipeline. arXiv:2008.06559.- Hammernik K, Klatzer T, Kobler E, Recht MP, Sodickson DK, Pock T, Knoll F. Learning a variational network for reconstruction of accelerated MRI data. Magn Reson Med 2018;79:3055-71. [Crossref] [PubMed]
- Park EJ, Lee Y, Lee HJ, Son JH, Yi J, Hahn S, Lee J. Impact of deep learning-based reconstruction and anti-peristaltic agent on the image quality and diagnostic performance of magnetic resonance enterography comparing single breath-hold single-shot fast spin echo with and without anti-peristaltic agent. Quant Imaging Med Surg 2024;14:722-35. [Crossref] [PubMed]
- Antun V, Renna F, Poon C, Adcock B, Hansen AC. On instabilities of deep learning in image reconstruction and the potential costs of AI. Proc Natl Acad Sci U S A 2020;117:30088-95. [Crossref] [PubMed]
- Almansour H, Herrmann J, Gassenmaier S, Afat S, Jacoby J, Koerzdoerfer G, Nickel D, Mostapha M, Nadar M, Othman AE. Deep Learning Reconstruction for Accelerated Spine MRI: Prospective Analysis of Interchangeability. Radiology 2023;306:e212922. [Crossref] [PubMed]
- Herrmann J, Koerzdoerfer G, Nickel D, Mostapha M, Nadar M, Gassenmaier S, Kuestner T, Othman AE. Feasibility and Implementation of a Deep Learning MR Reconstruction for TSE Sequences in Musculoskeletal Imaging. Diagnostics (Basel) 2021.
- Gassenmaier S, Afat S, Nickel MD, Mostapha M, Herrmann J, Almansour H, Nikolaou K, Othman AE. Accelerated T2-Weighted TSE Imaging of the Prostate Using Deep Learning Image Reconstruction: A Prospective Comparison with Standard T2-Weighted TSE Imaging. Cancers (Basel) 2021.
- Sreekumari A, Shanbhag D, Yeo D, Foo T, Pilitsis J, Polzin J, Patil U, Coblentz A, Kapadia A, Khinda J, Boutet A, Port J, Hancu I. A Deep Learning-Based Approach to Reduce Rescan and Recall Rates in Clinical MRI Examinations. AJNR Am J Neuroradiol 2019;40:217-23. [Crossref] [PubMed]
- Mazurowski MA, Buda M, Saha A, Bashir MR. Deep learning in radiology: An overview of the concepts and a survey of the state of the art with focus on MRI. J Magn Reson Imaging 2019;49:939-54. [Crossref] [PubMed]
- LeCun Y, Bengio Y, Hinton G. Deep learning. Nature 2015;521:436-44. [Crossref] [PubMed]
- Zhu L, Pan Z, Ma Q, Yang W, Shi H, Fu C, Yan X, Du L, Yan F, Zhang H. Diffusion Kurtosis Imaging Study of Rectal Adenocarcinoma Associated with Histopathologic Prognostic Factors: Preliminary Findings. Radiology 2017;284:66-76. [Crossref] [PubMed]
- Park JC, Park KJ, Park MY, Kim MH, Kim JK. Fast T2-Weighted Imaging With Deep Learning-Based Reconstruction: Evaluation of Image Quality and Diagnostic Performance in Patients Undergoing Radical Prostatectomy. J Magn Reson Imaging 2022;55:1735-44. [Crossref] [PubMed]
- Xu L, Xu J, Zheng Q, Yuan J, Liu J. A miniature U-net for k-space-based parallel magnetic resonance imaging reconstruction with a mixed loss function. Quant Imaging Med Surg 2022;12:4390-401. [Crossref] [PubMed]