Combining computed tomography features of left atrial epicardial and pericoronary adipose tissue with the triglyceride-glucose index to predict the recurrence of atrial fibrillation after radiofrequency catheter ablation: a machine learning study
Introduction
Atrial fibrillation (AF), the most common rapid arrhythmia encountered in clinical practice, can lead to severe complications such as ischemic stroke, thromboembolism, and heart failure (HF), and its prevalence has sharply increased over the past 30 years (1). Radiofrequency catheter ablation (RFCA) is currently the most effective method for controlling AF rhythm, but the recurrence rate within 1-year after surgery is high (2). Therefore, identifying predictive indicators for AF recurrence after RFCA has considerable value in clinical practice.
Inflammation plays a crucial role in the occurrence, development, and recurrence of AF (3,4). Insulin resistance (IR), a marker of metabolic disorders and systemic inflammation, is closely associated with cardiovascular disease (CVD) and has been identified as a prognostic predictor independent of type 2 diabetes mellitus (T2DM) status (5). Studies have shown that the triglyceride-glucose (TyG) index is an effective surrogate marker for IR (6,7). Previous research (8,9) has mainly focused on the TyG index and coronary artery disease (CAD), but no study has analyzed the predictive value of the TyG index for post-RFCA recurrence in patients with AF with or without T2DM.
Epicardial adipose tissue (EAT), is an important component of the endocrine system, but excess EAT is associated with an increased risk of hemodynamically significant CAD (10). EAT mediates inflammation-induced myocardial fibrosis, contributing to the occurrence and development of AF. Studies have indicated that the increased inflammatory activity in left atrial EAT (LA-EAT) is positively correlated with AF (11,12); however, the association between LA-EAT and AF recurrence remains controversial. One study reported that patients with higher attenuation of pericardial fat around the left atrium had significantly higher probability of AF recurrence (11), which contradicts the findings of Sang et al. (13). In recent years, an increasing number of studies have suggested that pericoronary adipose tissue (PCAT) is closely related to the development of coronary atherosclerosis and acute cardiovascular events (14,15). A new imaging biomarker, the fat attenuation index (FAI) (16), has been proposed to reflect the inflammatory state of the coronary artery wall. However, little research has been conducted regarding the relationship between PCAT and AF recurrence.
Various clinical models for predicting postoperative AF recurrence have been developed (17-20); however, the performance of these models remains unsatisfactory, and a more precise and robust model is needed. Machine learning is being increasingly integrated into clinical practice and has become an effective method for integrating multiple quantitative variables and thereby improving the accuracy of medical prognosis prediction. In our study, we quantified LA-EAT and PCAT using features derived from preoperative coronary computed tomography angiography (CCTA) and combined them with the TyG index to construct multifactorial machine learning prediction models. The purpose of this research was to identify more reliable noninvasive predictive indicators for the clinical prevention and treatment of AF recurrence and thereby aid in developing precise individualized treatment plans for improved patient outcomes. We present this article in accordance with the TRIPOD+AI reporting checklist (available at https://qims.amegroups.com/article/view/10.21037/qims-24-1393/rc).
Methods
Participants and general data collection
The data of patients with AF who underwent their first successful RFCA and preoperative CCTA examination at The Affiliated Hospital of Xuzhou Medical University from October 2018 to October 2022 were retrospectively collected. The inclusion criteria were as follows: (I) no history of CAD and (II) no medication or electrical cardioversion treatment within 3 months before surgery. Meanwhile, the exclusion criteria were as follows: (I) previous intracardiac electrophysiological examination or RFCA; (II) previous percutaneous coronary intervention (PCI) or cardiac surgery; (III) congenital heart disease, severe cardiomyopathy, heart valve disease, thyroid dysfunction, severe liver, or kidney dysfunction; and (IV) incomplete medical history or poor image quality (Figure 1). Patient data including age, gender, body mass index (BMI), heart rate, and medical history were also collected. The diagnosis of hypertension was in accordance with the 2018 Chinese Guidelines for the Management of Hypertension (21). Patients who had smoked ≥100 cigarettes in their lifetime without quitting were classified as smokers; otherwise, they were classified as nonsmokers (22). On the second day of hospitalization, fasting venous blood samples were collected, and clinical data including blood biochemistry were obtained. The TyG index was calculated using the following equation: TyG index = ln{[fasting triglyceride (TG) (mg/dL) × fasting glucose (mg/dL)]/2} (“ln” represents the natural logarithm function) (23).
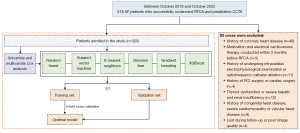
This study was conducted in accordance with the Declaration of Helsinki (as revised in 2013) and was approved by the Affiliated Hospital of Xuzhou Medical University Ethics Committee (No. XYFY2023-KL225-01). The requirement for individual consent was waived due to the retrospective nature of the analysis.
CCTA acquisition
CCTA scans were performed either on a third-generation dual-source CT device (Somatom FORCE; Siemens Healthineers, Erlangen, Germany) with a retrospective electrocardiogram-gating technique or on a 256-row CT device (Revolution CT; GE HealthCare, Chicago, IL, USA) with a prospective electrocardiogram-triggering technique. The scan range was from 1 cm below the tracheal carina to the cardiac diaphragm, and the bolus-tracking technique was used, with the region of interest (ROI) set in the aortic root at the level of the pulmonary artery trunk under a trigger threshold of 100 Hounsfield units (HU) and a delay time of 6 seconds. The iodinated contrast (iopromide, 370 mg iodine/mL; Bayer Pharmaceuticals, Berlin, Germany) contrast agent was injected at a rate of 3–5 mL/s through the right antecubital vein using a single-cylinder high-pressure injector and followed by a 50-mL injection of 0.9% saline at the same rate.
LA-EAT and PCAT measurement
The original CCTA images at 75% of the cardiac diastolic phase were transferred to a Siemens workstation (Syngo.via.VB40) with cardiac postprocessing software. The software automatically outlined the epicardial contours, with manual modifications made as necessary. The CT value range for fat density was defined as −190 to −30 HU. The left ventricular per-mitral annulus EAT, right atrial EAT more than 1 cm outside the right superior pulmonary vein, and EAT below the coronary sinus margin were manually excluded from the EAT. The remaining portion was defined as the LA-EAT, with the system directly calculating the volume and average attenuation of the left atrial epicardial fat. Measurements were obtained from the proximal 40 mm of the three major coronary branches, within a distance equal to the average diameter of the target vessel, to determine the surrounding fat volume and FAI (16). To avoid the influence of the aortic wall, the proximal 10 mm of the right coronary artery (RCA) was excluded, and measurements were obtained from the proximal 10 to 50 mm. The left main stem was not analyzed due to the variability of its length, and measurements were obtained from the proximal 40 mm of the left anterior descending (LAD) artery and left circumflex coronary artery (LCX). During the measurement of the LA-EAT and PCAT, the CT value for fat tissue was consistently defined as –190 to –30 HU. All images were measured and evaluated by two senior cardiovascular radiologists using the same method, with any discrepancies resolved through consultation (Figure 2).
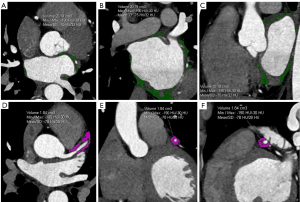
RFCA, outcomes, and follow-up
RCFA was conducted on patients with AF using the CARTO-3 system (Biosense Webster Inc., Irvine, CA, USA) for circumferential pulmonary vein isolation (CPVI). Guided by a circular electrode, both pulmonary veins were isolated. Additional linear ablation was performed if needed. Electrical cardioversion was used if a sinus rhythm was not achieved. The procedure’s endpoint was confirmed by bi-directional block between the left atrium and pulmonary veins.
All patients were followed up for 1 year after RFCA through outpatient visits, hospitalization, or telephone calls, with the recurrence of AF being the follow-up endpoint. Patients were examined at 1, 3, 6, and 12 months post-ablation, and after a 3-month “blanking period”, a 12-lead electrocardiogram or 24-hour Holter monitoring was performed. Recurrence was diagnosed if atrial arrhythmias (AF, flutter, or tachycardia) lasting more than 30 seconds were detected (2). For patients who did not undergo Holter monitoring at our hospital as scheduled, follow-up was conducted via telephone for inquiries concerning the Holter results from other hospitals.
Machine learning model development and evaluation
Six machine learning methods: random forest, support vector machine (SVM), k-nearest neighbor (KNN), gradient boosting, decision tree, and extreme gradient boost (XGBoost), were used to develop prediction models. Patients were randomly assigned to the training and validation sets at a ratio of 8:2 ratio. Fivefold cross-validation was employed to reduce prediction bias (Figure 1). Model discrimination and calibration were evaluated using receiver operating characteristic (ROC) curves, accuracy, sensitivity, F1 score, positive predictive value (PPV), and negative predictive value (NPV).
Feature importance and model interpretability analysis
Except for logistic regression (LR), machine learning models are typically “black box” algorithms. To interpret these models, the best-performing machine learning model was selected based on evaluation results. Shapley additive explanations (SHAP) plots, which use game-theoretic Shapley values to calculate each feature’s contribution to the model output, were used to analyze and explain the results of the machine learning models. Feature importance analysis was conducted on the selected model to determine the impact of features on the prediction, and features were ranked according to their importance. A feature importance plot was used for visualization.
Statistical analysis
The statistically significant indicators identified in the univariate Cox regression were included in the multivariate Cox regression analysis (via forward stepwise selection). Confounding factor adjustments were subsequently made for the selected independent predictors: model 1 adjusted for gender, age, and BMI; model 2, in addition to the factors included in model 1, adjusted for AF type, hypertension, smoking, and TG; and, in addition to the factors included in model 2, model 3 further adjusted for T2DM status, previous stroke/transient ischemic attack (TIA), HF, and high-sensitivity cardiac troponin T (hs-cTnT) level. Based on previous research, we conducted a Spearman correlation analysis on variables that could affect recurrence to analyze their correlations (11,13). Post-hoc subgroup analysis was conducted to evaluate the predictive value of the TyG index for AF recurrence post-RFCA in different patient subgroups. The statistical power for the overall population and each stratified subgroup was calculated using PASS 15.0 Software (NCSS LLC, Kaysville, UT, USA), and the final results were visualized in a forest plot. A P value <0.05 was considered statistically significant.
Statistical analyses were performed using Python 3.11.7 (Python Software Foundation, Wilmington, DE, USA), and R 4.4.0 (The R Foundation for Statistical Computing). Normally distributed continuous variables are expressed as the mean ± standard deviation and were compared using independent t-tests. Nonnormally distributed continuous variables are expressed as the median (with the 25th and 75th percentile) and were compared using the Wilcoxon nonparametric test. Categorical data are expressed as frequencies or percentages (%) and were compared using the χ2 test. A P value <0.05 was considered statistically significant. The areas under curve (AUCs) of the six models were compared using independent t-tests.
Results
Comparison of characteristics
Out of the 418 patients who underwent RFCA for AF, 325 were included in the study. Among them, there were 192 patients with paroxysmal AF (PAF) and 133 patients with persistent AF (PersAF). They were followed up for 12 months, with a median follow-up time of 11.5 months. In the end, 79 cases were confirmed to have recurred, and 246 cases did not recur, resulting in a recurrence rate of 24.3%. Compared with the nonrecurrent patients, patients with recurrent AF had significantly higher TG levels and TyG index but lower serum creatinine levels (all P values <0.05), with no statistical differences for the other clinical characteristics or laboratory indicators (P>0.05) (Table 1). The AF volume of the LA-EAT (LA-EATV), LA-EAT attenuation, and LCX-FAI of the recurrence group were significantly higher than those of the nonrecurrence group (P<0.001). There was no statistically significant difference in the PCT volume, LAD-FAI, or RCA-FAI between the recurrence group and the nonrecurrence group (P>0.05) (Table 1).
Table 1
Variables | Nonrecurrence (n=246) | Recurrence (n=79) | P |
---|---|---|---|
Clinical characteristics | |||
Age (years) | 60.93±9.73 | 59.39±10.31 | 0.230 |
Male | 156 (63.41) | 42 (53.16) | 0.104 |
BMI (kg/m2) | 25.56±3.63 | 25.30±2.98 | 0.555 |
Heart rate (bpm) | 77.18±19.23 | 81.94±20.85 | 0.062 |
Paroxysmal AF | 146 (59.35) | 46 (58.23) | 0.860 |
HTN | 105 (42.68) | 36 (45.57) | 0.652 |
Smoking | 60 (24.39) | 16 (20.25) | 0.450 |
Previous stroke/TIA | 43 (17.48) | 19 (24.05) | 0.196 |
HF | 29 (11.79) | 5 (6.33) | 0.168 |
T2DM | 27 (10.98) | 9 (11.39) | 0.918 |
CHA2DS2-VASc score | 2 [1, 3] | 1 [1, 3] | 0.916 |
HAS-BLED score | 1 [0, 2] | 1 [0, 2] | 0.300 |
Laboratory indicator | |||
TC (mmol/L) | 4.23±0.96 | 4.38±0.97 | 0.219 |
TG (mmol/L) | 1.48±0.74 | 1.71±0.74 | 0.017 |
TyG index | 8.61±0.53 | 8.96±0.49 | <0.001 |
BUN (mmol/L) | 5.80±2.02 | 5.52±1.34 | 0.242 |
Scr (mmol/L) | 68 [59, 75] | 63 [57, 73] | 0.046 |
Uric acid (μmmol/L) | 329.01±92.40 | 313.65±95.61 | 0.203 |
GGT (U/L) | 34.11±27.95 | 35.46±34.12 | 0.725 |
HDL-C (mmol/L) | 1.01 [0.89, 1.24] | 1.07 [0.93, 1.24] | 0.209 |
LDL-C (mmol/L) | 2.49 [1.99, 3.03] | 2.55 [2.15, 3.06] | 0.609 |
hs-cTnT (ng/L) | 9.16 [6.08, 13.37] | 9.32 [5.86, 16.70] | 0.577 |
Imaging characteristics | |||
LA-EATV (cm3) | 21.09 [16.42, 28.79] | 29.08 [23.23, 36.24] | <0.001 |
LA-EAT attenuation (HU) | −81.57±7.35 | −76.76±7.52 | <0.001 |
LAD-V (cm3) | 1.25±0.35 | 1.33±0.36 | 0.067 |
LAD-FAI (HU) | −78.59 [−84.99, −74.43] | −79.00 [−89.11, −74.95] | 0.376 |
LCX-V (cm3) | 1.41±0.37 | 1.33±0.43 | 0.141 |
LCX-FAI (HU) | −84.10±10.52 | −76.80±8.84 | <0.001 |
RCA-V (cm3) | 1.51±0.38 | 1.56±0.44 | 0.326 |
RCA-FAI (HU) | −83.45±10.69 | −84.73±11.54 | 0.366 |
Continuous variables are presented as mean ± standard deviation or as median [interquartile range]; categorical variables are presented as number (%). BMI, body mass index; AF, atrial fibrillation; HTN, hypertension; TIA, transient ischemic attack; HF, heart failure; T2DM, type 2 diabetes mellitus; HAS-BLED, hypertension, abnormal renal/liver function, stroke, bleeding history or predisposition, labile INR, elderly, drugs/alcohol concomitantly; INR, international normalized ratio; CHA2DS2-VASc, congestive heart failure, hypertension, age ≥75 years (doubled), diabetes mellitus, prior stroke or transient ischemic attack (doubled), vascular disease, age 65–74 years, female; TC, total cholesterol; TG, triglyceride; TyG, triglyceride-glucose; BUN, blood urea nitrogen; Scr, serum creatinine; GGT, γ-glutamyltransferase; HDL-C, high-density lipoprotein cholesterol; LDL-C, low-density lipoprotein cholesterol; hs-cTnT, high-sensitivity cardiac troponin T; LA-EATV, volume of the left atrial epicardial adipose tissue; LA-EAT, left atrium epicardial adipose tissue; HU, Hounsfield unit; LAD, left anterior descending artery; LAD-V, adipose volume surrounding the LAD; LAD-FAI, left anterior descending artery fat attenuation index; LCX, left circumflex coronary artery; LCX-V, adipose volume surrounding the LCX; LCX-FAI, left circumflex coronary artery fat attenuation index; RCA, right coronary artery; RCA-V, adipose volume surrounding the RCA; RCA-FAI, right coronary artery fat attenuation index.
AF recurrence feature selection
In the univariate Cox analysis, the TG and hs-cTnT level were identified as the influencing factors for postoperative AF recurrence. However, in the multivariate Cox regression, these two indicators were excluded (P>0.05), indicating that they were not independent predictors (Table 2). The multivariate Cox regression analyses on clinical and imaging parameters identified LA-EATV, LA-EAT attenuation, LCX-FAI, and the TyG index as independent risk factors for AF recurrence after RFCA (Table 2). Further adjustments for various confounding factors confirmed the significance of these four indicators (Table 3). The correlation heatmap of the factors is shown in Figure 3. The correlation coefficients between variables were all lower than 0.8, indicating that no serious collinearity existed. Based on net benefit and threshold probability, the decision curve analysis (Figure 4A) indicated that the combined indicators had good clinical utility for prediction. The calibration curve (Figure 4B) showed that the combined prediction of the four indicators aligned well with the observed actual risk.
Table 2
Variables | Univariate analysis | Multivariate analysis | |||||
---|---|---|---|---|---|---|---|
HR | 95% CI | P | HR | 95% CI | P | ||
Clinical characteristics | |||||||
Age (years) | 0.989 | 0.967–1.011 | 0.303 | – | – | – | |
Male | 0.707 | 0.455–1.101 | 0.125 | – | – | – | |
BMI (kg/m2) | 0.984 | 0.922–1.051 | 0.640 | – | – | – | |
Paroxysmal AF | 0.930 | 0.595–1.455 | 0.752 | – | – | – | |
HTN | 1.110 | 0.713–1.728 | 0.645 | – | – | – | |
T2DM | 1.026 | 0.512–2.054 | 0.943 | – | – | – | |
Previous stroke/TIA | 1.397 | 0.834–2.340 | 0.204 | – | – | – | |
HF | 0.562 | 0.227–1.390 | 0.212 | – | – | – | |
CHA2DS2-VASc score | 1.006 | 0.869–1.164 | 0.936 | – | – | – | |
HAS-BLED score | 1.158 | 0.941–1.425 | 0.166 | – | – | – | |
Laboratory indicators | |||||||
TC (mmol/L) | 1.126 | 0.904–1.402 | 0.289 | – | – | – | |
TG (mmol/L) | 1.375 | 1.059–1.785 | 0.017 | 0.944 | 0.646–1.379 | 0.766 | |
TyG index | 2.603 | 1.784–3.798 | <0.001 | 2.268 | 1.372–3.750 | 0.001 | |
Scr (mmol/L) | 0.987 | 0.972–1.001 | 0.074 | – | – | – | |
HDL-C (mmol/L) | 1.230 | 0.588–2.572 | 0.582 | – | – | – | |
LDL-C (mmol/L) | 1.196 | 0.936–1.528 | 0.152 | – | – | – | |
hs-cTnT (ng/L) | 1.005 | 1.001–1.009 | 0.013 | 1.003 | 0.999–1.008 | 0.115 | |
Imaging characteristics | |||||||
LA-EATV (cm3) | 1.052 | 1.033–1.072 | <0.001 | 1.043 | 1.021–1.066 | <0.001 | |
LA-EAT attenuation (HU) | 1.074 | 1.043–1.106 | <0.001 | 1.042 | 1.007–1.078 | 0.017 | |
LAD-V (cm3) | 1.838 | 0.994–3.398 | 0.052 | – | – | – | |
LAD-FAI (HU) | 0.986 | 0.963–1.010 | 0.242 | – | – | – | |
LCX-V (cm3) | 0.666 | 0.372–1.191 | 0.171 | – | – | – | |
LCX-FAI (HU) | 1.059 | 1.037–1.082 | <0.001 | 1.032 | 1.007–1.058 | 0.012 | |
RCA-V (cm3) | 1.382 | 0.790–2.415 | 0.256 | – | – | – | |
RCA-FAI (HU) | 0.990 | 0.971–1.011 | 0.347 | – | – | – |
AF, atrial fibrillation; HR, hazard ratio; CI, confidence interval; BMI, body mass index; HTN, hypertension; T2DM, type 2 diabetes mellitus; TIA, transient ischemic attack; HF, heart failure; HAS-BLED, hypertension, abnormal renal/liver function, stroke, bleeding history or predisposition, labile INR, elderly, drugs/alcohol concomitantly; INR, international normalized ratio; CHA2DS2-VASc, congestive heart failure, hypertension, age ≥75 years (doubled), diabetes mellitus, prior stroke or transient ischemic attack (doubled), vascular disease, age 65–74 years, female; TC, total cholesterol; TG, triglyceride; TyG, triglyceride-glucose; Scr, serum creatinine; HDL-C, high-density lipoprotein cholesterol; LDL-C, low-density lipoprotein cholesterol; hs-cTnT, high-sensitivity cardiac troponin T; LA-EATV, volume of the left atrial epicardial adipose tissue; LA-EAT, left atrium epicardial adipose tissue; HU, Hounsfield unit; LAD, left anterior descending artery; LAD-V, adipose volume surrounding the LAD; LAD-FAI, left anterior descending artery fat attenuation index; LCX, left circumflex coronary artery; LCX-V, adipose volume surrounding the LCX; LCX-FAI, left circumflex coronary artery fat attenuation index; RCA, right coronary artery; RCA-V, adipose volume surrounding the RCA; RCA-FAI, right coronary artery fat attenuation index.
Table 3
Variable | Model 1 | Model 2 | Model 3 | ||||||||
---|---|---|---|---|---|---|---|---|---|---|---|
HR | 95% CI | P | HR | 95% CI | P | HR | 95% CI | P | |||
TyG index | 2.614 | 1.776–3.847 | <0.001 | 3.337 | 2.086–5.338 | <0.001 | 3.392 | 2.081–5.529 | <0.001 | ||
LA-EATV | 1.061 | 1.040–1.081 | <0.001 | 1.060 | 1.039–1.082 | <0.001 | 1.059 | 1.037–1.082 | <0.001 | ||
LA-EAT attenuation | 1.073 | 1.042–1.106 | <0.001 | 1.079 | 1.047–1.112 | <0.001 | 1.083 | 1.051–1.116 | <0.001 | ||
LCX-FAI | 1.058 | 1.036–1.081 | <0.001 | 1.061 | 1.038–1.085 | <0.001 | 1.064 | 1.040–1.088 | <0.001 |
Model 1: adjusted for gender, age and BMI. Model 2: adjusted for gender, age, BMI, type, HTN, smoking, and TG. Model 3: adjusted for gender, age, BMI, type, HTN, smoking, TG, T2DM, previous stroke/TIA, HF, and hs-cTnT. HR, hazard ratio; CI, confidence interval; TyG, triglyceride-glucose; LA-EATV, volume of the left atrial epicardial adipose tissue; LA-EAT, left atrium epicardial adipose tissue; LCX-FAI, left circumflex coronary artery fat attenuation index; BMI, body mass index; HTN, hypertension; TG, triglyceride; T2DM, type 2 diabetes mellitus; TIA, transient ischemic attack; HF, heart failure; hs-cTnT, high-sensitivity cardiac troponin T.
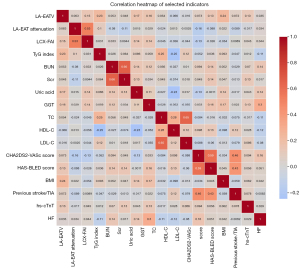
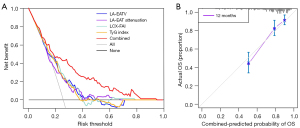
Post-hoc subgroup analysis of the TyG index in predicting endpoint events
Post-hoc subgroup analysis was conducted to evaluate the clinical indicators related to the TyG index for predicting AF recurrence, including age (<65 vs. ≥65 years), type of AF (PersAF vs. PAF), gender (male vs. female), hypertension (yes vs. no), T2DM (yes vs. no), smoking (yes vs. no), TIA (yes vs. no), HF (yes vs. no), and hs-cTnT level [<14 vs. ≥14 ng/L (24)]. After stratification of these indicators, a higher TyG index was found to be significantly associated with an increased risk of AF recurrence after RFCA. Moreover, we observed that the results of the HF subgroup were unreliable due to low statistical power, while the statistical power of the T2DM subgroup was slightly lower (0.692<0.8), and those of the other subgroups were close to 1. Therefore, the predictive efficiency of the TyG index might not have been affected by T2DM status (Pinteraction =0.660) (Figure 5). Additionally, the TyG index effectively predicted AF recurrence in both the patients with PersAF and those with PAF, as shown in Figure 5.
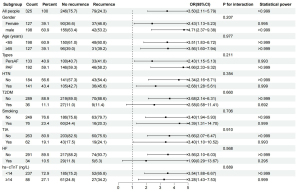
Comparison and explanation of model performance
The detailed metrics of the models are shown in Table 4 and Figure 6. In the training set, the average AUC values were as follows: 0.733 for the random forest model, 0.736 for the SVM model, 0.756 for the KNN model, 0.728 for the gradient boosting model, 0.555 for the decision tree model, and 0.703 for the XGBoost model (Figure 6). The SVM model showed the best predictive efficiency (AUC, 0.793; 95% CI: 0.782–0.805) in this study, and had the highest accuracy (0.804) and PPV (0.710), as shown in Table 4. Additionally, the AUC t-test comparison results for the six models are provided in Table S1, indicating that the SVM model had the best predictive performance. Figure 7 illustrates the explanation of the SVM model. The orders of contributions of each feature for predicting AF recurrence on the entire dataset are shown in Figure 7A,7B, with the TyG index and LA-EATV being the two most important predictive variables. In addition, Figure 7C,7D provide a local model explanation according to the SHAP method, showing how a certain prediction was made for a specific individual by incorporating individualized input data.
Table 4
Model | AUC (95% CI) | Accuracy | F1 score | Sensitivity | PPV | NPV |
---|---|---|---|---|---|---|
Random forest | 0.781 (0.729–0.835) | 0.792 | 0.501 | 0.427 | 0.647 | 0.833 |
SVM | 0.793 (0.782–0.805) | 0.804 | 0.489 | 0.381 | 0.710 | 0.825 |
KNN | 0.702 (0.649–0.747) | 0.750 | 0.379 | 0.329 | 0.472 | 0.806 |
Gradient boosting | 0.759 (0.661–0.837) | 0.762 | 0.425 | 0.363 | 0.545 | 0.814 |
Decision tree | 0.616 (0.549–0.695) | 0.704 | 0.422 | 0.444 | 0.413 | 0.816 |
XGBoost | 0.727 (0.655–0.800) | 0.754 | 0.448 | 0.410 | 0.502 | 0.821 |
RFCA, radiofrequency catheter ablation; AUC, area under the curve; CI, confidence interval; PPV, positive predictive value; NPV, negative predictive value; SVM, support vector machine; KNN, k-nearest neighbor; XGB, extreme gradient boosting.
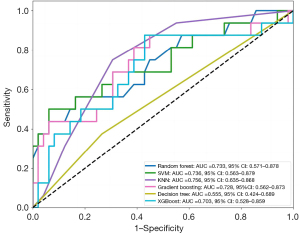
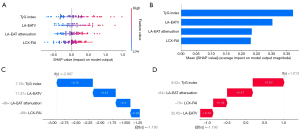
Comparison of machine learning and traditional models
Compared with traditional models from previous studies, the AUC of the SVM model in our study was 0.793, which was higher than the AUCs (0.539–0.780) of the tradition models consisting of the TyG index, LA-EAT, or PCAT. A summary of the related literature is detailed in Table 5.
Table 5
First author/year | Features | AUC |
---|---|---|
Zhao/2020 (17) | CHA2DS2-VASc; SAMe-TT2R2 | 0.612; 0.642 |
Özmen/2023 (20) | C2HEST; CHA2DS2-VASc | 0.769; 0.644 |
Sang/2023 (13) | LA-EAT volume; LA-EAT attenuation | 0.714; 0.615 |
Ma/2023 (19) | LCX-FAI; EATVI | 0.722; 0.630 |
Tang/2022 (18) | TyG index; LAD | 0.737; 0.780 |
CHA2DS2-VASc; APPLE | 0.624; 0.752 | |
Wang/2024 (25) | TyG index; METS-IR | 0.539; 0.603 |
TyG-BMI index; TG/HDL-C ratio | 0.608; 0.524 |
AUC, area under the curve; CHA2DS2-VASc, congestive heart failure, hypertension, age ≥75 years (doubled), diabetes mellitus, prior stroke or transient ischemic attack (doubled), vascular disease, age 65–74 years, female; SAMe-TT2R2, female, age <60 years, a history of at least two comorbidities (hypertension, diabetes mellitus, coronary artery disease or myocardial infarction, peripheral artery disease, congestive heart failure, previous stroke, and pulmonary, hepatic, or renal disease), treatment with drugs interacting with vitamin K antagonist (e.g., amiodarone) (doubled), current/recent tobacco use (within 2 years) (doubled), nonwhite ethnicity (doubled); C2HEST, coronary artery disease/chronic obstructive pulmonary disease, hypertension, age ≥75 years (doubled), systolic heart failure ≥75 years (doubled), thyroid disease (hyperthyroidism); LA-EAT, left atrial epicardial adipose tissue; LCX-FAI, left circumflex coronary artery fat attenuation index; EATV, volume of the epicardial adipose tissue; EATVI, EATV index (EATV/body surface area); TyG, triglyceride-glucose; LAD, left atrial diameter; APPLE, age greater than 65 years, persistent atrial fibrillation, reduced estimated glomerular filtration rate (<60 mL/min/1.73 m2), left atrial diameter >43 mm, and left ventricular ejection fraction <50%; METS-IR, metabolic score for insulin resistance; TyG-BMI, triglyceride-glucose-body mass index; TG/HDL-C, ratio of triglyceride to high-density lipoprotein cholesterol.
Discussion
Although the technology for radiofrequency ablation is continually advancing, the recurrence rate of AF post-ablation remains high (2), and it remains clinically challenging to accurately assess the risk of postoperative recurrence. In our study, the performance of six machine learning models combining the TyG index and CT features in predicting AF recurrence after RFCA were compared, and the results showed that the SVM model had the highest predictive efficiency (AUC, 0.793), accuracy (0.804), and PPV (0.710).
Machine learning algorithms have the ability to deeply mine and analyze large datasets and have been widely applied in disease prognosis. The SVM algorithm is one of the most widely used algorithms in machine learning, suitable for both classification and regression problems. For nonlinear associations, an appropriate kernel function is chosen to map the samples to a high-dimensional space to identify the optimal hyperplane for separating the samples (26). Furthermore, traditional models in previous studies have often relied on single indicators for prediction and had lower predictive efficiency. However, our model quantifies LA-EAT and PCAT based on preoperative CCTA and combines them with the TyG index for predictive evaluation. This approach not only avoids the need for additional examinations but also significantly improves the predictive value.
According to the most recent guidelines (27), AF should not be viewed in isolation due to the various comorbidities associated with its recurrence and progression. Therefore, we adjusted for confounding factors among the significant indicators from the multivariate Cox regression analysis. After adjustments were mode for comorbidities of AF, including T2DM, HF, and previous stroke/TIA, we found that LA-EATV, LA-EAT attenuation, LCX-FAI, and the TyG index remained independent predictors. Additionally, univariate and multivariate Cox regression analyses demonstrated that hs-cTnT level was a factor influencing the recurrence of AF after radiofrequency ablation; however, it was not an independent predictive indicator. Previous studies have extensively demonstrated a close correlation between hs-cTnT levels and the occurrence, progression, and recurrence of AF (28,29). For instance, Nakanishi et al. analyzed 125 patients with AF and found that an elevated serum hs-cTnT level, independent of traditional risk factors, was associated with recurrence after RFCA (30). However, this is inconsistent with the conclusions of our study. The reasons for this finding could potentially be attributed to the larger sample size in our study, the exclusive focus on patients without CAD, and the incorporation of newer imaging markers such as left atrial epicardial and pericoronary fat for analysis, leading to discrepant results.
The results in our study indicated that the TyG index was the primary predictor of AF recurrence. IR is an important risk factor for CVD (31,32). However, the hyperinsulinemic-euglycemic clamp test, a gold-standard test for evaluating IR, is complex, time-consuming, expensive, and highly invasive, limiting its clinical application. Studies have confirmed that the TyG index is a reliable and convenient method for assessing IR (6,7), and it can serve as an indicator for risk stratification and prognosis evaluation of acute coronary syndrome, irrespective of patients’ diabetes status (33,34). IR increases the oxidation of CaMKIIδ, enhances the expression of NCX1, and elevates the phosphorylation levels of phospholamban/RyR-2, resulting in abnormalities of intracellular calcium homeostasis. Additionally, IR stimulates the expression of TGF-β1 in cardiomyocytes and fibroblasts, contributing to atrial structural remodeling through paracrine and autocrine pathways, thereby increasing susceptibility to AF. IR also promotes atrial electrical and structural remodeling by activating the mitogen-activated protein kinase (MAPK) pathway (35,36). An animal experiment has indicated that IR impairs the translocation of glucose transporter 4 and 8 as well as the expression of total protein, providing metabolic substrates for the development of AF (37). Inflammation, IR, and oxidative stress damage are interrelated, further leading to left atrial fibrosis (36) and low-voltage areas in the left atrium (38). A recent retrospective study of nondiabetic patients undergoing cardiac radiofrequency ablation showed that an increased TyG index could increase the risk of late AF recurrence (18), which is consistent with our findings. However, TyG is calculated using fasting plasma glucose (FPG) and TGs, and thus the diabetic status may become a crucial factor affecting the predictive performance of the TyG index. In addition, the predictive efficiency of the TyG index in patients with AF who are diabetic remains unclear. To the best of our knowledge, our study represents the first attempt to explore the predictive independence of the TyG index for AF recurrence in patients with and without T2DM. Our subgroup analysis showed that the TyG index performed well in nondiabetic patients (Pinteraction=0.660; Figure 5). However, it should be noted that the statistical power of the T2DM subgroup was limited, primarily due to the small sample size of the patients with T2DM in this study. Nevertheless, we believe that the TyG index can effectively predict AF recurrence, potentially independent of T2DM status, but naturally, additional research and verification are needed. Similarly, when stratification by AF type was applied, the TyG index had good predictive value for postoperative AF recurrence in both patients with PersAF and those with PAF (Pinteraction =0.21; Figure 5).
In our study, the volume and attenuation of LA-EAT ranked just below the TyG index in feature importance. EAT, as a special type of visceral fat, can lead to atrial remodeling in patients with AF through complex mechanisms such as inflammation, matrix remodeling, fat infiltration, and autonomic ganglia [ganglionated plexi (GP)] (39). As an important component of EAT, LA-EAT encompasses 95% of the five major anatomical GP regions (40). Clinically, RFCA targets generally overlap with LA-EAT locations (39). Therefore, this study focused on LA-EAT rather than overall EAT, and the results indicated that the LA-EATV and attenuation were independent predictors of AF recurrence post-RFCA, consistent with the findings of Tsao et al. and Ciuffo et al. (39,41). For one, a larger LA-EATV suggests a greater degree of infiltration of inflammatory adipokines directly into the left atrium (42), causing myocardial cells to secrete inflammatory mediators that lead to the loss of myocardial cell homogeneity, atrial structural, functional remodeling, and, ultimately, atrial fibrosis. For another, several studies have shown that only LA-EAT expresses genes related to oxidative phosphorylation, muscle contraction, and calcium signaling, which may trigger inflammation, release paracrine factors, alter adipocyte differentiation, and induce lymphocyte migration, leading to increased LA-EAT attenuation (11,40).
In addition to the TyG index and LA-EAT parameters, this study found that LCX-FAI also contributed significantly to predicting post-AF ablation recurrence. However, previous studies mainly used PCAT for research on coronary artery-related diseases, and few studies have analyzed its relationship with AF recurrence after ablation. A recent study reported that the average CT attenuation (HU) of PCAT within 40 mm proximal to all three major coronary arteries (43) could predict AF recurrence after second-generation cryoballoon ablation. Aside from differences in surgical methods, our study primarily measured the PCAT volume and FAI of the three coronary arteries separately and found that only increased LCX-FAI was associated with AF recurrence after ablation. The potential explanations for this finding are as follows: (I) high levels of FAI indicate changes in intracellular lipid accumulation and increased local vascular inflammation (14). The development of AF leads to the release of inflammatory active factors from the LCX near the left atrium, causing dysfunction and cellular remodeling of the surrounding fat, resulting in increased FAI. This further validates our study’s findings that high levels of attenuation in pericardial fat around the left atrium are associated with increased AF recurrence risk. (II) As the LCX supplies the left atrium, changes in LCX-FAI can directly affect the atrial tissue in its supply area. Increased FAI leads to a greater abundance of fat tissue infiltrating myocardial cells, promoting electrical and structural remodeling of atrial tissue, thus increasing the risk of AF recurrence. (III) Although quantitative analysis of PCAT volume and FAI can reflect the mechanisms of AF development from a variety of perspectives (35), CT attenuation shows higher accuracy and sensitivity in reflecting the physiological and pathological changes of fat compared to volume parameters. Our study’s findings are generally consistent with those of Ma et al. (19). However, the study by Ma et al. did not exclude the influence of CAD on PCAT. Our study included 325 patients who were confirmed to be free of CAD through CCTA or coronary angiography, thereby avoiding potential bias in the results.
Previous studies have indicated that the CHA2DS2-VASc [congestive HF, hypertension, age ≥75 years (doubled), diabetes mellitus, prior stroke or TIA (doubled), vascular disease, age 65–74 years, female] score is an independent predictor of AF recurrence after RFCA (17,20). However, in our study, there was no significant difference in this indicator among the recurrence and nonrecurrence groups; therefore, it was not included in the machine learning models. This result may be due to the exclusion of patients with CAD in our study, as vascular disease is a major component of the CHA2DS2-VASc score. Our finding further suggests that the CHA2DS2-VASc score has limited predictive value for AF recurrence in non-CAD populations.
This study also involved certain limitations that should be addressed. First, we employed a retrospective, single-center design that lacked external validation from an independent cohort. Moreover, the sample size and variety of features were relatively small, leading to slightly lower statistical power for certain subgroups in the post-hoc analysis. In addition, the incidence rate of endpoint events was also relatively low (24.3%). Although we attempted to address this issue through fivefold cross-validation, overfitting might have occurred. Second, all imaging parameters were manually measured, which might have introduced errors. Additionally, none of the patients in this study were implanted with implantable loop recorders. Therefore, continuous monitoring of their heart rhythm during the follow-up period was not possible. This potentially led to the overlooking of the asymptomatic recurrence of AF in these patients. Finally, this study primarily evaluated the TyG index of patients at the time of admission, without continuously monitoring their blood glucose levels during the follow-up period. The cumulative TyG index during follow-up may provide a better prediction of AF recurrence. Furthermore, the glycemic control status of patients with T2DM at admission was not recorded, thus preventing an investigation into the relationship between the TyG index and glycemic control.
Conclusions
In contrast to models in previous studies, the machine learning SVM model in our study integrated the TyG index and quantitative CCTA imaging features. This yielded a high and improved prediction accuracy for AF recurrence after RFCA and could provide clear explanations for personalized risk prediction.
Acknowledgments
The authors would like to thank the research staff for their help.
Funding: This study was supported by
Footnote
Reporting Checklist: The authors have completed the TRIPOD+AI reporting checklist. Available at https://qims.amegroups.com/article/view/10.21037/qims-24-1393/rc
Conflicts of Interest: All authors have completed the ICMJE uniform disclosure form (available at https://qims.amegroups.com/article/view/10.21037/qims-24-1393/coif). A.S. is an employee of GE HealthCare China. The other authors have no conflicts of interest to declare.
Ethical Statement: The authors are accountable for all aspects of the work in ensuring that questions related to the accuracy or integrity of any part of the work are appropriately investigated and resolved. This study was conducted in accordance with the Declaration of Helsinki (as revised in 2013) and was approved by the Affiliated Hospital of Xuzhou Medical University Ethics Committee (No. XYFY2023-KL225-01). The requirement for individual consent was waived due to the retrospective nature of the analysis.
Open Access Statement: This is an Open Access article distributed in accordance with the Creative Commons Attribution-NonCommercial-NoDerivs 4.0 International License (CC BY-NC-ND 4.0), which permits the non-commercial replication and distribution of the article with the strict proviso that no changes or edits are made and the original work is properly cited (including links to both the formal publication through the relevant DOI and the license). See: https://creativecommons.org/licenses/by-nc-nd/4.0/.
References
- Arbelo E, Dagres N. The 2020 ESC atrial fibrillation guidelines for atrial fibrillation catheter ablation, CABANA, and EAST. Europace 2022;24:ii3-7. [Crossref] [PubMed]
- Calkins H, Hindricks G, Cappato R, Kim YH, Saad EB, Aguinaga L, et al. 2017 HRS/EHRA/ECAS/APHRS/SOLAECE expert consensus statement on catheter and surgical ablation of atrial fibrillation. Heart Rhythm 2017;14:e275-444. [Crossref] [PubMed]
- Hu YF, Chen YJ, Lin YJ, Chen SA. Inflammation and the pathogenesis of atrial fibrillation. Nat Rev Cardiol 2015;12:230-43. [Crossref] [PubMed]
- Wan P, Yu W, Zhai L, Qian B, Zhang F, Liu B, Wang J, Shao X, Shi Y, Jiang Q, Wang M, Shao S, Wang Y. The relationship between right atrial wall inflammation and poor prognosis of atrial fibrillation based on (18)F-FDG positron emission tomography/computed tomography. Quant Imaging Med Surg 2024;14:1369-82. [Crossref] [PubMed]
- Han S, Wang C, Tong F, Li Y, Li Z, Sun Z, Sun Z. Triglyceride glucose index and its combination with the Get with the Guidelines-Heart Failure score in predicting the prognosis in patients with heart failure. Front Nutr 2022;9:950338. [Crossref] [PubMed]
- Huang R, Wang Z, Chen J, Bao X, Xu N, Guo S, Gu R, Wang W, Wei Z, Wang L. Prognostic value of triglyceride glucose (TyG) index in patients with acute decompensated heart failure. Cardiovasc Diabetol 2022;21:88. [Crossref] [PubMed]
- Tahapary DL, Pratisthita LB, Fitri NA, Marcella C, Wafa S, Kurniawan F, Rizka A, Tarigan TJE, Harbuwono DS, Purnamasari D, Soewondo P. Challenges in the diagnosis of insulin resistance: Focusing on the role of HOMA-IR and Tryglyceride/glucose index. Diabetes Metab Syndr 2022;16:102581. [Crossref] [PubMed]
- Wang M, Mei L, Jin A, Cai X, Jing J, Wang S, Meng X, Li S, Wei T, Wang Y, Pan Y. Association between triglyceride glucose index and atherosclerotic plaques and Burden: findings from a community-based study. Cardiovasc Diabetol 2022;21:204. [Crossref] [PubMed]
- Xie E, Ye Z, Wu Y, Zhao X, Li Y, Shen N, Gao Y, Zheng J. The triglyceride-glucose index predicts 1-year major adverse cardiovascular events in end-stage renal disease patients with coronary artery disease. Cardiovasc Diabetol 2023;22:292. [Crossref] [PubMed]
- Yu W, Chen Y, Zhang F, Liu B, Wang J, Shao X, Yang X, Shi Y, Wang Y. Association of epicardial adipose tissue volume with increased risk of hemodynamically significant coronary artery disease. Quant Imaging Med Surg 2023;13:2582-93. [Crossref] [PubMed]
- El Mahdiui M, Simon J, Smit JM, Kuneman JH, van Rosendael AR, Steyerberg EW, van der Geest RJ, Száraz L, Herczeg S, Szegedi N, Gellér L, Delgado V, Merkely B, Bax JJ, Maurovich-Horvat P. Posterior Left Atrial Adipose Tissue Attenuation Assessed by Computed Tomography and Recurrence of Atrial Fibrillation After Catheter Ablation. Circ Arrhythm Electrophysiol 2021;14:e009135. [Crossref] [PubMed]
- Wang B, Xu YD, Shao S, Zhai LS, Qian B, Zhang FF, Wang JF, Shao XL, Wang YT. Association between inflammation activity of left atrial epicardial adipose tissue measured by (18)F-FDG PET/CT and atrial fibrillation. Zhonghua Xin Xue Guan Bing Za Zhi 2021;49:1213-9. [Crossref] [PubMed]
- Sang C, Hu X, Zhang D, Shao Y, Qiu B, Li C, Li F, Zhang C, Wang Z, Chen M. The predictive value of left atrium epicardial adipose tissue on recurrence after catheter ablation in patients with different types of atrial fibrillation. Int J Cardiol 2023;379:33-9. [Crossref] [PubMed]
- Lin A, Nerlekar N, Yuvaraj J, Fernandes K, Jiang C, Nicholls SJ, Dey D, Wong DTL. Pericoronary adipose tissue computed tomography attenuation distinguishes different stages of coronary artery disease: a cross-sectional study. Eur Heart J Cardiovasc Imaging 2021;22:298-306. [Crossref] [PubMed]
- Yang T, Li G, Wang C, Xu G, Li Q, Yang Y, Zhu L, Chen L, Li X, Yang H. Insulin resistance and coronary inflammation in patients with coronary artery disease: a cross-sectional study. Cardiovasc Diabetol 2024;23:79. [Crossref] [PubMed]
- Goeller M, Achenbach S, Herrmann N, Bittner DO, Kilian T, Dey D, Raaz-Schrauder D, Marwan M. Pericoronary adipose tissue CT attenuation and its association with serum levels of atherosclerosis-relevant inflammatory mediators, coronary calcification and major adverse cardiac events. J Cardiovasc Comput Tomogr 2021;15:449-54. [Crossref] [PubMed]
- Zhao J, Zhou D, Chen M, Zhuo C, Lin Z, Zheng L, Wang Q. CHA2DS2-VASc and SAMe-TT2R2 scores as predictors of recurrence for nonvalvular atrial fibrillation patients on vitamin K antagonists after radiofrequency catheter ablation. J Cardiovasc Med (Hagerstown) 2020;21:200-8. [Crossref] [PubMed]
- Tang Q, Guo XG, Sun Q, Ma J. The pre-ablation triglyceride-glucose index predicts late recurrence of atrial fibrillation after radiofrequency ablation in non-diabetic adults. BMC Cardiovasc Disord 2022;22:219. [Crossref] [PubMed]
- Ma GJ, Guo FQ, Hu J, Liu XW, Chen C, Gao B, Li CY. Association of pericoronary adipose tissue with atrial fibrillation recurrence after ablation based on computed tomographic angiography. Jpn J Radiol 2023;41:955-64. [Crossref] [PubMed]
- Özmen G, Koca F C. (2)HEST and CHA(2)DS(2)-Vasc for predicting recurrence after catheter ablation of paroxysmal atrial fibrillation. Kardiologiia 2023;63:52-60. [Crossref] [PubMed]
- Liu S. 2018 Chinese guidelines for the management of hypertension. Chin J Cardiovasc Med 2019;24:24-56.
- Kehl KL, Zahrieh D, Yang P, Hillman SL, Tan AD, Sands JM, Oxnard GR, Gillaspie EA, Wigle D, Malik S, Stinchcombe TE, Ramalingam SS, Kelly K, Govindan R, Mandrekar SJ, Osarogiagbon RU, Kozono D. Rates of Guideline-Concordant Surgery and Adjuvant Chemotherapy Among Patients With Early-Stage Lung Cancer in the US ALCHEMIST Study (Alliance A151216). JAMA Oncol 2022;8:717-28. [Crossref] [PubMed]
- Thai PV, Tien HA, Van Minh H, Valensi P. Triglyceride glucose index for the detection of asymptomatic coronary artery stenosis in patients with type 2 diabetes. Cardiovasc Diabetol 2020;19:137. [Crossref] [PubMed]
- Giannitsis E, Kurz K, Hallermayer K, Jarausch J, Jaffe AS, Katus HA. Analytical validation of a high-sensitivity cardiac troponin T assay. Clin Chem 2010;56:254-61. [Crossref] [PubMed]
- Wang Z, He H, Xie Y, Li J, Luo F, Sun Z, Zheng S, Yang F, Li X, Chen X, Chen Y, Sun Y. Non-insulin-based insulin resistance indexes in predicting atrial fibrillation recurrence following ablation: a retrospective study. Cardiovasc Diabetol 2024;23:87. [Crossref] [PubMed]
- Lu Y, Chen Q, Zhang H, Huang M, Yao Y, Ming Y, Yan M, Yu Y, Yu L. Machine Learning Models of Postoperative Atrial Fibrillation Prediction After Cardiac Surgery. J Cardiothorac Vasc Anesth 2023;37:360-6. [Crossref] [PubMed]
- Van Gelder IC, Rienstra M, Bunting KV, Casado-Arroyo R, Caso V, Crijns HJGM, et al. 2024 ESC Guidelines for the management of atrial fibrillation developed in collaboration with the European Association for Cardio-Thoracic Surgery (EACTS). Eur Heart J 2024;45:3314-414. [Crossref] [PubMed]
- Anegawa T, Kai H, Adachi H, Hirai Y, Enomoto M, Fukami A, Otsuka M, Kajimoto H, Yasuoka S, Iwamoto Y, Aoki Y, Fukuda K, Imaizumi T. High-sensitive troponin T is associated with atrial fibrillation in a general population. Int J Cardiol 2012;156:98-100. [Crossref] [PubMed]
- Tamura S, Doi A, Matsuo M, Katayama H, Yoshiyama T, Tatsumi H, Izumiya Y, Yoshiyama M. Prognostic value of high-sensitive troponin T for predicting cardiovascular events after atrial fibrillation ablation. J Cardiovasc Electrophysiol 2019;30:1475-82. [Crossref] [PubMed]
- Nakanishi K, Fukuda S, Yamashita H, Hasegawa T, Kosaka M, Shirai N, Shimada K, Yoshikawa J, Tanaka A. High-sensitive cardiac troponin T as a novel predictor for recurrence of atrial fibrillation after radiofrequency catheter ablation. Europace 2017;19:1951-7. [Crossref] [PubMed]
- Rask-Madsen C, Kahn CR. Tissue-specific insulin signaling, metabolic syndrome, and cardiovascular disease. Arterioscler Thromb Vasc Biol 2012;32:2052-9. [Crossref] [PubMed]
- Ormazabal V, Nair S, Elfeky O, Aguayo C, Salomon C, Zuñiga FA. Association between insulin resistance and the development of cardiovascular disease. Cardiovasc Diabetol 2018;17:122. [Crossref] [PubMed]
- Zhang Y, Ding X, Hua B, Liu Q, Gao H, Chen H, Zhao XQ, Li W, Li H. High Triglyceride-Glucose Index is Associated with Poor Cardiovascular Outcomes in Nondiabetic Patients with ACS with LDL-C below 1.8 mmol/L. J Atheroscler Thromb 2022;29:268-81. [Crossref] [PubMed]
- Karadeniz FÖ, Sancaktepe EA, Karadeniz Y. High Triglyceride-Glucose Index is Associated With Poor Prognosis in Patients With Acute Coronary Syndrome in Long-Term Follow-Up. Angiology 2023;74:139-48. [Crossref] [PubMed]
- Bohne LJ, Johnson D, Rose RA, Wilton SB, Gillis AM. The Association Between Diabetes Mellitus and Atrial Fibrillation: Clinical and Mechanistic Insights. Front Physiol 2019;10:135. [Crossref] [PubMed]
- Chan YH, Chang GJ, Lai YJ, Chen WJ, Chang SH, Hung LM, Kuo CT, Yeh YH. Atrial fibrillation and its arrhythmogenesis associated with insulin resistance. Cardiovasc Diabetol 2019;18:125. [Crossref] [PubMed]
- Maria Z, Campolo AR, Scherlag BJ, Ritchey JW, Lacombe VA. Dysregulation of insulin-sensitive glucose transporters during insulin resistance-induced atrial fibrillation. Biochim Biophys Acta Mol Basis Dis 2018;1864:987-96. [Crossref] [PubMed]
- Seewöster T, Kosich F, Sommer P, Bertagnolli L, Hindricks G, Kornej J. Prediction of low-voltage areas using modified APPLE score. Europace 2021;23:575-80. [Crossref] [PubMed]
- Tsao HM, Hu WC, Wu MH, Tai CT, Lin YJ, Chang SL, Lo LW, Hu YF, Tuan TC, Wu TJ, Sheu MH, Chang CY, Chen SA. Quantitative analysis of quantity and distribution of epicardial adipose tissue surrounding the left atrium in patients with atrial fibrillation and effect of recurrence after ablation. Am J Cardiol 2011;107:1498-503. [Crossref] [PubMed]
- Takahashi K, Okumura Y, Watanabe I, Nagashima K, Sonoda K, Sasaki N, Kogawa R, Iso K, Kurokawa S, Ohkubo K, Nakai T, Nakahara S, Hori Y, Nikaido M, Hirayama A. Anatomical proximity between ganglionated plexi and epicardial adipose tissue in the left atrium: implication for 3D reconstructed epicardial adipose tissue-based ablation. J Interv Card Electrophysiol 2016;47:203-12. [Crossref] [PubMed]
- Ciuffo L, Nguyen H, Marques MD, Aronis KN, Sivasambu B, de Vasconcelos HD, Tao S, Spragg DD, Marine JE, Berger RD, Lima JAC, Calkins H, Ashikaga H. Periatrial Fat Quality Predicts Atrial Fibrillation Ablation Outcome. Circ Cardiovasc Imaging 2019;12:e008764. [Crossref] [PubMed]
- Iacobellis G. Local and systemic effects of the multifaceted epicardial adipose tissue depot. Nat Rev Endocrinol 2015;11:363-71. [Crossref] [PubMed]
- Nogami K, Sugiyama T, Kanaji Y, Hoshino M, Hara S, Yamaguchi M, Hada M, Sumino Y, Misawa T, Hirano H, Ueno H, Miwa N, Yamao K, Kusa S, Hachiya H, Kakuta T. Association between pericoronary adipose tissue attenuation and outcome after second-generation cryoballoon ablation for atrial fibrillation. Br J Radiol 2021;94:20210361. [Crossref] [PubMed]