Radiomics for predicting grades, isocitrate dehydrogenase mutation, and oxygen 6-methylguanine-DNA methyltransferase promoter methylation of adult diffuse gliomas: combination of structural MRI, apparent diffusion coefficient, and susceptibility-weighted imaging
Introduction
Gliomas are the most common type of primary intracranial tumors. In 2016, the 4th edition of World Health Organization Classification Criteria of Tumors of the Central Nervous System Tumor (WHO CNS4) first introduced isocitrate dehydrogenase (IDH) and 1p19q as diagnostic molecular markers for adult diffuse gliomas (1). In the latest edition WHO CNS5, adult diffuse gliomas are categorized into three subtypes: glioblastoma, IDH wildtype, astrocytoma, IDH mutant, and oligodendroglioma, IDH mutant with 1p19q co-delated (2). IDH-mutant glioma patients show better outcomes than IDH-wildtype patients. Oxygen 6-methylguanine-DNA methyltransferase (MGMT) promoter methylation is an epigenetic prognostic biomarker for gliomas (3). MGMT is a DNA damage repair enzyme which decreases the efficacy of the chemotherapy drug temozolomide (4). Methylated MGMT is deactivated and MGMT promoter-methylated patients have longer progression-free survival (PFS) and overall survival (OS) than unmethylated patients (5,6).
The detection of the molecular signature plays an important role in the diagnosis and treatment of glioma patients (7,8). Currently, the genetic information of adult diffuse gliomas is mainly obtained by sequencing the tumor sample resected in the surgery, which is invasive, expensive, and time-consuming. It is valuable to provide a quick, noninvasive, and low-expense method to identify the meaningful biomarkers of gliomas.
Magnetic resonance imaging (MRI) is important in clinical diagnosis and follow-up of glioma patients. In recent years, with the rapid progress of computer vision and artificial intelligence techniques, radio-genomics has illustrated considerable potential to detect tumor biomarkers through multi-parameter MRI (9,10). Radiomic features extracted from conventional structural MRI sequences such as T1-weighted imaging (T1WI), T2-weighted imaging (T2WI), T1 contrast-enhanced imaging (T1CE), and fluid-attenuated inversion recovery (FLAIR) have been shown to be effective to predict grades and IDH mutation status of gliomas (11,12). Diffusion-weighted imaging (DWI) and apparent diffusion coefficient (ADC) reflect the cell density in the tumor regions, which indicates microstructural differences between tumors. Features extracted from multiple diffusion metrics can also identify IDH mutation with high accuracy(13). Nevertheless, the value of radiomic features from structural MRI and ADC in predicting MGMT promoter methylation status is controversial. Some researchers obtain excellent results using structural MRI, ADC, or combined sequences (14-16). However, in a recent multi-center retrospective study, Kim et al. validated 420 structural MRI-based models to predict MGMT promoter methylation status, from which approximately 80% of models achieved no significant difference with the chance level of 50% (17). The research in predicting glioma MGMT promoter status with MRI radiomics is still insufficient and needs further investigation.
Susceptibility-weighted imaging (SWI) is a valuable MRI sequence to depict tumor microvasculature and microhemorrhage (18). SWI can hemi-quantitatively assess tumor vessels and blood products through intra-tumoral susceptibility signals (ITSS) (19). IDH wildtype, MGMT promoter unmethylated, and high-grade gliomas tend to have higher ITSS (20-22). However, there has been no research investigating the value of radiomic features extracted from SWI in predicting grade, IDH mutation, and MGMT promoter methylation status, which deserves further exploration.
The purpose of this study was to investigate the value of conventional structural MRI, ADC, and especially, SWI radiomic features, to predict glioma grade and molecular markers. We present this article in accordance with the TRIPOD reporting checklist (available at https://qims.amegroups.com/article/view/10.21037/qims-24-1110/rc).
Methods
Patients
The study was conducted in accordance with the Declaration of Helsinki (as revised in 2013). The study was approved by the Ethical Committee of Nanjing Drum Tower Hospital (No. 2022-364-02) and the requirement for individual consent for this retrospective analysis was waived. The publicly available University of California San Francisco Preoperative Diffuse Glioma (UCSF-PDGM) dataset was downloaded from The Cancer Imaging Archive (23,24) (TCIA, https://www.cancerimagingarchive.net/).
A total of 1,145 patients from Nanjing Drum Tower Hospital between January 2010 and December 2022 and UCSF between January 2015 and December 2021 with pathologically confirmed diagnosis of adult diffuse glioma according to WHO CNS5 were screened. The exclusion criteria were as follows: (I) patients received chemotherapy or radiation therapy prior to the MRI scan; (II) poor MRI quality due to inferior image quality; and (III) missing sequences on baseline MRI, including ADC and SWI. Finally, 539 patients were enrolled in this study. In UCSF-PDGM, 426 patients who did not receive burr-hole biopsy before imaging were included as the training cohort and the other 67 patients who received preoperational burr-hole biopsy were included as the internal validation cohort. A further 46 patients in our hospital were included as the external test cohort, as illustrated in Figure 1.
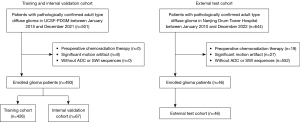
The clinical characteristic and genetic data of patients then UCSF-PDGM were available online. For patients in our hospital, patient age and sex were retrieved from electronic records. Grade 2 and 3 patients were defined as “low-grade”, whereas Grade 4 patients were defined as “high-grade”. IDH mutation was tested using Sanger sequencing. MGMT methylation status of patients in our hospital was unknown.
MRI protocol
MRI data of UCSF-PDFM were acquired using a 3.0T MRI scanner Discovery 750 (GE Healthcare, Milwaukee, WI, USA), which were downloaded from TCIA. MRI data in our hospital were acquired using two 3.0T MRI scanners, including Achieva (Philips Medical Systems, Best, Netherlands) and Ingenia (Philips Medical Systems). Details of the MRI protocol are listed in Appendix 1.
Image processing and radiomic feature extraction
The same preprocessing procedure using software SimpleITK (version 2.2.0; https://simpleitk.org/) was applied to the training cohort, internal validation cohort, and external test cohort. Each sequence was registered and resampled to a 1×1×1 mm3 voxel resolution represented by FLAIR imaging utilizing automated nonlinear registration. Subsequently, the resampled data was skull stripped. Fuzzy C-means-based intensity normalization was used to eliminate the greyscale distribution differences and made the MRI image histograms more consistent among patients.
We defined 2 regions of interest (ROIs) in this research. Tumor core (TC) consists of enhancing tumor parenchyma, non-enhancing tumor parenchyma, and necrosis area. Whole tumor (WT) consists of the TC and surrounding edema area. WT was delineated on T2WI. For those patients with strongly enhancing tumors, TC was delineated on T1CE, as illustrated in Figure 2. For those patients with non-enhancing tumors, we considered TC identical to WT due to poorly discriminated edges, as illustrated in Figure 3. The researchers participating in the delineation were blinded to the patients’ pathological diagnosis, WHO grade, and other molecular markers.
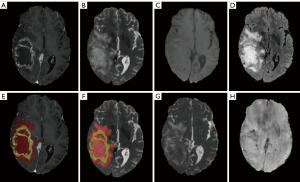
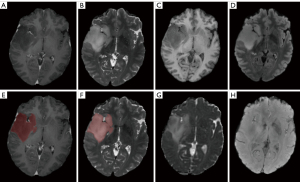
For patients in UCSF-PDGM, all the image data was segmented by an ensemble model based on previous Brain Tumor Segmentation (BraTS) challenge deep learning algorithms. The automated segmentation labels were reviewed and corrected manually by groups of annotators with different experience and finally approved by two neuroradiologists with over 15 years of experience in UCSF. For patients in our hospital, all the segmentation labels were manual segmented by a board-certificated neuroradiologist with more than 15 years of experience.
We extracted radiomics features in the two types of ROIs among the six sequences of each patients utilizing the open-source python package Pyradiomics (https://github.com/Radiomics/pyradiomics). The extracted features consisted of 18 first-order features, 14 three-dimensional (3D) shape-based features, 16 gray level run length matrix (GLRLM) features, 16 gray level size zone matrix (GLSZM) features, 14 gray level dependence matrix (GLDM) features, 5 neighboring gray tone difference matrix (NGTDM) features, and 23 gray level cooccurrence matrix (GLCM) features. Features were extracted on six MRI sequences after transformation applying seven different image filters, which are original, square, square root, logarithm, exponential, gradient, exponential Laplacian of Gaussian, and wavelet. For each patient, 658 features in two ROIs among six sequences were extracted for a total of 7,896 features.
Radiomics features selection and model construction
The extracted features among the six sequences were selected based on the training cohort before the model construction process. Pearson correlation was utilized to reduce feature redundancy. Radiomic features exhibiting a correlation coefficient greater than 0.95 were excluded from further analysis. Then, we utilized the analysis of variance (ANOVA) F-test as the feature selection method due to its outstanding performance and high efficacy. We computed the F-value for all the features. For each combination of sequences, different sets of 40 features with highest F-value were selected to train the machine learning model. Features with low correlation were dropped out because they hardly improved the accuracy. The number of features was 40 and consistent across different radiomics models (Tables S1-S6).
WHO grade 4, IDH mutated, and MGMT promoter methylated are considered as the positive class, respectively. Random forest was utilized to establish multiple radiomics classification models, the area under the receiver operating curve (AUC), accuracy (ACC), sensitivity (SENS), specificity (SPEC), positive predictive value (PPV), negative predictive value (NPV), F1 Score, and Matthew’s correlation coefficient (MCC) were utilized to compare the performance of different radiomics models.
Two radiologists with 1- and 5-years of respective experience in neuro-oncology imaging also evaluated WHO grade and genetic information with all six sequences provided, as a comparison with multiple radiomics models. The study design of this research is shown in Figure 4.
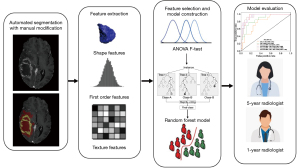
Statistical analysis
Mann-Whitney-U test was performed to determine the differences of age distribution between the training cohort and internal validation cohort. Patients’ age was reported as median (Q1, Q3). Nominal variables were reported as number (percentage). Chi-squared test was performed to evaluate the differences in sex, tumor grade, IDH, and MGMT promoter methylation among groups. Delong test was performed to compare the AUCs of different radiomics models. A P value <0.05 was considered statistically significant in this research. All statistical analyses were performed using Python software version 3.7.13 (http://www.python.org).
Results
Baseline information
Table 1 summarizes the baseline characteristic of the 539 patients enrolled in this study. Totals of 426 and 67 patients from UCSF-PDGM were enrolled in the training cohort and the internal validation cohort, respectively. There were no significant differences in the distribution of age, sex, tumor WHO grade, IDH mutation, and MGMT promoter methylation status between the training cohort and internal validation cohort. An additional 46 patients in our hospital were included in the external test cohort. MGMT promoter methylation status was unknown in the external test cohort.
Table 1
Characteristics | Overall (n=493) | Training (n=426) | Internal validation (n=67) | P value | External test (n=46) |
---|---|---|---|---|---|
Age (years) | 59 [47–68] | 60 [48–69] | 56 [44–65] | 0.054a | 55 [47–62] |
Sex | 0.289b | ||||
Female | 199 (40.4) | 168 (39.4) | 31 (46.3) | 22 (47.8) | |
Male | 294 (59.6) | 258 (60.6) | 36 (53.7) | 24 (52.2) | |
Grade | 0.321b | ||||
2 | 54 (11.0) | 50 (11.7) | 4 (6.0) | 19 (41.3) | |
3 | 43 (8.7) | 38 (8.9) | 5 (7.5) | 6 (13.0) | |
4 | 396 (80.3) | 338 (79.3) | 58 (86.6) | 21 ( (45.7) | |
IDH | 0.847b | ||||
Wild-type | 393 (79.7) | 339 (79.6) | 54 (80.6) | 23 (50.0) | |
Mutant | 100 (20.3) | 87 (20.4) | 13 (19.4) | 23 (50.0) | |
MGMT | 0.292b | ||||
Unmethylated | 117 (28.2) | 104 (29.1) | 13 (22.4) | 0 | |
Methylated | 298 (71.8) | 253 (70.89) | 45 (77.6) | 0 |
Patients’ age is reported as median [interquartile range]. Nominal variables are reported as n (%). a, Mann-Whitney-U test; b, chi-squared test. IDH, isocitrate dehydrogenase; MGMT, oxygen 6-methylguanine-DNA methyltransferase.
Multiparametric MRI radiomics model capacity to predict WHO grade of adult diffuse gliomas
The modality combination of structural MRI plus SWI and structural MRI plus ADC plus SWI exhibited the highest AUC of 0.987 [95% confidence interval (CI): 0.964–1.000] on the internal validation cohort and AUC of 0.951 (95% CI: 0.886–1.000) on the external test cohort. On the internal validation cohort, the 1- and 5-year radiologist achieved accuracies of 0.761 and 0.866, respectively. On the external test cohort, the 1-year radiologist and 5-year radiologist achieved accuracies of 0.783 and 0.848, respectively. The metrics of the multiparametric MRI radiomics models predicting tumor grades are illustrated in Table 2 and Figure 5.
Table 2
Cohort | Modality | AUC (95% CI) | ACC | F1 score | SENS | SPEC | PPV | NPV | MCC |
---|---|---|---|---|---|---|---|---|---|
Internal validation | Structural MRI | 0.975 (0.938–1.000) | 0.970 | 0.983 | 1.000 | 0.778 | 0.967 | 1.000 | 0.867 |
ADC | 0.824 (0.692–0.956) | 0.821 | 0.895 | 0.879 | 0.444 | 0.911 | 0.364 | 0.298 | |
SWI | 0.960 (0.915–1.000) | 0.910 | 0.949 | 0.966 | 0.556 | 0.933 | 0.714 | 0.581 | |
Structural MRI + ADC | 0.975 (0.938–1.000) | 0.970 | 0.983 | 1.000 | 0.778 | 0.967 | 1.000 | 0.867 | |
Structural MRI + SWI | 0.987 (0.964–1.000) | 0.970 | 0.983 | 1.000 | 0.778 | 0.967 | 1.000 | 0.867 | |
Structural MRI + ADC + SWI | 0.987 (0.964–1.000) | 0.970 | 0.983 | 1.000 | 0.778 | 0.967 | 1.000 | 0.867 | |
1-year radiologist | – | 0.761 | 0.717 | 0.776 | 0.667 | 0.938 | 0.316 | 0.335 | |
5-year radiologist | – | 0.866 | 0.875 | 0.862 | 0.889 | 0.980 | 0.500 | 0.601 | |
External test | Structural MRI | 0.918 (0.830–1.000) | 0.618 | 0.698 | 1.000 | 0.316 | 0.536 | 1.000 | 0.411 |
ADC | 0.895 (0.791–0.999) | 0.735 | 0.743 | 0.867 | 0.632 | 0.650 | 0.857 | 0.503 | |
SWI | 0.805 (0.654–0.957) | 0.647 | 0.714 | 1.000 | 0.368 | 0.556 | 1.000 | 0.452 | |
Structural MRI + ADC | 0.918 (0.830–1.000) | 0.618 | 0.698 | 1.000 | 0.316 | 0.536 | 1.000 | 0.411 | |
Structural MRI + SWI | 0.951 (0.886–1.000) | 0.676 | 0.732 | 1.000 | 0.421 | 0.577 | 1.000 | 0.493 | |
Structural MRI + ADC + SWI | 0.951 (0.886–1.000) | 0.676 | 0.732 | 1.000 | 0.421 | 0.577 | 1.000 | 0.493 | |
1-year radiologist | – | 0.783 | 0.780 | 0.800 | 0.762 | 0.800 | 0.762 | 0.562 | |
5-year radiologist | – | 0.848 | 0.848 | 0.840 | 0.857 | 0.875 | 0.818 | 0.695 |
AUC, area under the curve; CI, confidence interval; ACC, accuracy; SENS, sensitivity; SPEC, specificity; PPV, positive predictive value; NPV, negative predictive value; MCC, Matthew’s correlation coefficient; ADC, apparent diffusion coefficient; SWI, susceptibility-weighted imaging.
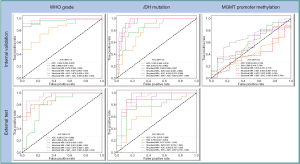
Multiparametric MRI radiomics model capacity to predict IDH mutation status of adult diffuse gliomas
The modality combination of structural MRI plus ADC plus SWI exhibited the highest AUC of 0.953 (95% CI: 0.904–1.000) on the internal validation cohort; structural MRI exhibited the highest AUC of 0.917 (95% CI: 0.801–1.000) on the external test cohort. On the internal validation cohort, the 1- and 5-year radiologist achieved accuracies of 0.776 and 0.791, respectively; on the external test cohort, the 1- and 5-year radiologist achieved accuracies of 0.761 and 0.783, respectively. The metrics of the multiparametric MRI radiomics models in predicting IDH mutation are illustrated in Table 3 and Figure 5.
Table 3
Cohort | Modality | AUC (95% CI) | ACC | F1 score | SENS | SPEC | PPV | NPV | MCC |
---|---|---|---|---|---|---|---|---|---|
Internal validation | Structural MRI | 0.946 (0.895–0.997) | 0.866 | 0.710 | 0.846 | 0.870 | 0.611 | 0.959 | 0.639 |
ADC | 0.790 (0.666–0.914) | 0.701 | 0.524 | 0.846 | 0.667 | 0.379 | 0.947 | 0.409 | |
SWI | 0.862 (0.762–0.962) | 0.836 | 0.593 | 0.615 | 0.889 | 0.571 | 0.906 | 0.490 | |
Structural MRI + ADC | 0.936 (0.876–0.995) | 0.866 | 0.571 | 0.462 | 0.963 | 0.750 | 0.881 | 0.518 | |
Structural MRI + SWI | 0.944 (0.891–0.998) | 0.896 | 0.696 | 0.615 | 0.963 | 0.800 | 0.912 | 0.642 | |
Structural MRI + ADC + SWI | 0.953 (0.904–1.000) | 0.851 | 0.667 | 0.769 | 0.870 | 0.588 | 0.940 | 0.581 | |
1-year radiologist | – | 0.776 | 0.634 | 1.000 | 0.464 | 0.722 | 1.000 | 0.579 | |
5-year radiologist | – | 0.791 | 0.749 | 0.815 | 0.692 | 0.917 | 0.474 | 0.445 | |
External test | Structural MRI | 0.917 (0.801–1.000) | 0.853 | 0.857 | 0.833 | 0.875 | 0.882 | 0.824 | 0.707 |
ADC | 0.701 (0.515–0.888) | 0.559 | 0.694 | 0.944 | 0.125 | 0.548 | 0.667 | 0.122 | |
SWI | 0.795 (0.637–0.954) | 0.618 | 0.435 | 0.278 | 1.000 | 1.000 | 0.552 | 0.391 | |
Structural MRI + ADC | 0.882 (0.755–1.000) | 0.618 | 0.480 | 0.333 | 0.938 | 0.857 | 0.556 | 0.334 | |
Structural MRI + SWI | 0.872 (0.733–1.000) | 0.588 | 0.417 | 0.278 | 0.938 | 0.833 | 0.536 | 0.282 | |
Structural MRI + ADC + SWI | 0.887 (0.755–1.000) | 0.794 | 0.800 | 0.778 | 0.813 | 0.824 | 0.765 | 0.589 | |
1-year radiologist | – | 0.761 | 0.760 | 0.739 | 0.783 | 0.773 | 0.750 | 0.522 | |
5-year radiologist | – | 0.783 | 0.780 | 0.739 | 0.826 | 0.810 | 0.760 | 0.567 |
IDH, isocitrate dehydrogenase; AUC, area under the curve; CI, confidence interval; ACC, accuracy; SENS, sensitivity; SPEC, specificity; PPV, positive predictive value; NPV, negative predictive value; MCC, Matthew’s correlation coefficient; ADC, apparent diffusion coefficient; SWI, susceptibility-weighted imaging; MRI, magnetic resonance imaging.
Multiparametric MRI radiomics model capacity to predict MGMT promoter methylation status of adult diffuse gliomas
The modality combination of structural MRI plus SWI exhibited the highest AUC of 0.650 (95% CI: 0.485–0.814) on internal validation cohort, as shown in Table 4. The 1- and 5-year radiologist achieved accuracies of 0.552 and 0.672, respectively. The metrics of the multiparametric MRI radiomics models in predicting tumor MGMT status are illustrated in Table 4 and Figure 5. Neither the multiparametric radiomics models or the radiologists achieved high performance in predicting MGMT promoter status.
Table 4
Modality | AUC (95% CI) | ACC | F1 score | SENS | SPEC | PPV | NPV | MCC |
---|---|---|---|---|---|---|---|---|
Structural MRI | 0.613 (0.451–0.775) | 0.672 | 0.804 | 0.867 | 0.000 | 0.750 | 0.000 | −0.183 |
ADC | 0.566 (0.403–0.729) | 0.672 | 0.804 | 0.867 | 0.000 | 0.750 | 0.000 | −0.183 |
SWI | 0.599 (0.418–0.780) | 0.810 | 0.891 | 1.000 | 0.154 | 0.804 | 1.000 | 0.352 |
Structural MRI + ADC | 0.575 (0.397–0.754) | 0.707 | 0.813 | 0.822 | 0.308 | 0.804 | 0.333 | 0.134 |
Structural MRI + SWI | 0.650 (0.485–0.814) | 0.707 | 0.828 | 0.911 | 0.000 | 0.759 | 0.000 | −0.146 |
Structural MRI + ADC + SWI | 0.581 (0.399-0.764) | 0.759 | 0.857 | 0.933 | 0.154 | 0.792 | 0.400 | 0.130 |
1-year radiologist | – | 0.552 | 0.471 | 0.692 | 0.357 | 0.600 | 0.455 | 0.052 |
5-year radiologist | – | 0.672 | 0.595 | 0.735 | 0.500 | 0.800 | 0.409 | 0.222 |
MGMT, oxygen 6-methylguanine-DNA methyltransferase; AUC, area under the curve; CI, confidence interval; ACC, accuracy; SENS, sensitivity; SPEC, specificity; PPV, positive predictive value; NPV, negative predictive value; MCC, Matthew’s correlation coefficient; ADC, apparent diffusion coefficient; SWI, susceptibility-weighted imaging; MRI, magnetic resonance imaging.
Comparation of different radiomics models in predicting WHO grade and IDH mutation status
All the P values of multiple Delong test were larger than 0.05. There were no significant differences in predicting WHO grade and IDH mutation status for these radiomics models, as indicated in Table 5.
Table 5
Comparisons | P value | |
---|---|---|
WHO Grade | IDH mutation | |
Structural MRI vs. structural MRI + SWI | 0.171 | 0.179 |
Structural MRI vs. structural MRI + ADC | 0.209 | 0.163 |
Structural MRI vs. structural MRI + SWI + ADC | 0.171 | 0.198 |
Structural MRI + SWI vs. structural MRI + ADC | 0.263 | 0.752 |
Structural MRI + SWI vs. structural MRI + SWI + ADC | 1.000 | 0.485 |
Structural MRI + ADC vs. structural MRI + SWI + ADC | 0.263 | 0.837 |
WHO, World Health Organization; MRI, magnetic resonance imaging; IDH, isocitrate dehydrogenase; ADC, apparent diffusion coefficient; SWI, susceptibility-weighted imaging.
Discussion
In this study, we explored the application of radiomics features based on preoperative multiparametric MRI to establish multiple radiomics models to predict the WHO grades and key molecular markers of adult diffuse gliomas. The results showed that the constructed models had excellent predictive performance in WHO grade and IDH mutation task, which indicated promising clinical application in the future. However, none of the established models in this research succeeded to differentiate MGMT promoter methylation efficiently.
To the best of our knowledge, this is the first study investigating the value of SWI radiomics features in predicting WHO grades, IDH mutation, and MGMT promoter status of adult diffuse gliomas. In previous research, investigators tried to apply ITSS, which is a semi-quantitative metric depicting the vasculature and micro-hemorrhage within the tumor, to evaluate the grade, IDH mutation, 1p19q codeletion, and MGMT promoter status of glioma (25). Jitender revealed that grade IV gliomas tend to have higher ITSS values compared with Grade II and Grade III gliomas. However, there were no significant differences of ITSS between Grade II and Grade III gliomas (18). High-grade gliomas appear to have more neovascularization than low-grade gliomas, which is reflected by more foci of susceptibility within the tumor on SWI sequences. The performance of structural MRI sequence radiomics features is sufficient; adding SWI and ADC cannot further increase the efficacy to predict glioma grade.
IDH is the most important molecular marker to define diffuse gliomas in WHO CNS5 (26). Numerous articles have reported that models based on multiparametric MRI radiomics features are useful to predict IDH mutation status (27,28). IDH mutation refers to better treatment response and longer OS, regardless of the histopathological grade. Prior multimodal radiomics machine learning studies have predicted IDH mutation with AUCs ranging from 0.76 to 0.96 (10,29). IDH-wildtype glioblastomas appear to be obviously enhanced and cystic with abundant vasculature and high cellular density, represented by low ADC and high perfusion on MRI (30,31). IDH-wildtype glioblastomas also tend to have more micro-hemorrhage foci within the tumor parenchyma, resulting in high ITSS on SWI. In our study, the SWI radiomics model achieved an AUC of 0.862 on the internal validation cohort and 0.701 on the external test cohort. However, the structural MRI model achieved an AUC of 0.917, which was higher than that of the combined structural MRI, ADC, and SWI model. The Delong test illustrated that there were no significant differences of model performance after adding ADC and SWI features.
We discovered that incorporating ADC and SWI radiomics features cannot provide significant improvement in predicting WHO grade and IDH mutation. The results contradict neuroradiologists’ experience and perceptions. ADC reflects tumor cell density and SWI is associated with neovascularization within tumor parenchyma. Both ADC and SWI are popular parameters to characterize tumor grade and IDH mutation. Here are some potential explanations. First is the redundancy of information in radiomic features. Structural MRI sequences already encompass a wide range of tumor characteristics, such as mass morphology, peritumor edema, and contrast enhancement. It is possible that structural sequences have captured enough relevant features for predicting WHO grade and IDH with sufficient accuracy, making additional information from ADC and SWI. Secondly, there may be some correlation among features from different sequences. Although SWI reflects neovascularization and ADC highlights cell density, these characteristics may already be represented indirectly in structural MRI. For example, IDH-wildtype glioblastomas usually have massive necrosis with bleeding. The micro-hemorrhage foci commonly exist in necrotic areas, which can be detected well as hyperintensity on T1WI. In addition, high-grade gliomas with high cellular density tend to have cysts and a high degree of enhancement. Therefore, the additional features from ADC and SWI may not provide substantially new information beyond what has already been presented in structural MRI. The final possible reasons may be image quality and resolution. Clinically, structural MRIs are usually obtained in 3D with high resolution and high signal-to-noise ratio (SNR), whereas SWI and ADC are much more likely to be obtained in relatively lower resolution with lower SNR. The relatively lower image quality compared with structural MRI sequences could overshadow some potential useful information which ADC and SWI may contain, thus not improving model performance significantly.
In this study, we did not expect to obtain the results that all the radiomics models we trained failed to predict MGMT promoter methylation effectively. Among all the models, combined structural MRI and SWI model performed best on the internal validation cohort with an AUC of 0.650, which is almost no different from random chance. All of the established models are not effective for clinical application. Since we obtained high robustness on the WHO grading and IDH mutation tasks, we trust that our model construction methodology involved no significant errors. We tend to believe that it is impossible to predict MGMT promoter methylation status precisely using structural MRI, ADC, and SWI with current radiomics and machine learning techniques. Two previous studies established multiple deep learning models from structural MRI data to determine MGMT promoter status and finally drew the conclusion that current deep learning algorithms are unable to predict MGMT promoter methylation merely using structural MRI data (17,32). However, some other studies claimed to predict MGMT promoter methylation successfully (15,33). Currently, the question whether MRI features can reflect MGMT status is still under debate. More studies based on advanced MRI techniques are needed for further investigation.
MGMT is a DNA mismatch repair protein, preventing gliomas from the damage caused by alkylating agent temozolomide (34). The methylation of the promoter site of this gene will silence its expression. Patients with MGMT promoter methylation are more likely to benefit from temozolomide therapy and have longer OS. In several previous studies, it was revealed that gliomas without MGMT promoter methylation tends to have thick enhancement and infiltrative edema, whereas the other gliomas with MGMT promoter methylation usually present with nodular enhancement and mass-like edema (35). Based on this image manifestation, various researchers devoted to establishing a feasible model from MRI radiomics features to predict MGMT promoter status pre-operationally. The results were controversial. Robineteven tried the exact methylation percentage as the training label but still did not obtain any significant results (32). In this study, we discovered that even adding ADC and SWI to structural MRI information cannot improve model efficacy to a clinically applicable level. The biological nature of MGMT promoter methylation is complex and might not be fully captured by the imaging features used in our models. The status of MGMT methylation is highly spatially heterogeneous and different parts of tumors may have different methylation percentages. Therefore, clinically, the determination of MGMT promoter methylation status is sample-dependent and it is hard to define MGMT methylation using a binary label. There are many intermediate methylation statuses with radiology manifestations similar to MGMT methylated status or MGMT unmethylated status, which are easily misclassified.
The models constructed in this research were able to predict IDH mutation and WHO grade with high efficacy. The established prediction model may help neuro-oncologists to make more precise diagnoses and guide treatment plans for glioma patients. More efforts are required in the investigation of the full value of multiparametric MRI features based on deep learning frameworks to predict core molecular marks, especially MGMT, in the future.
There were also several limitations in this research. (I) The MGMT status was unknown for patients in the external test cohort. However, considering that all the radiomics models failed to discriminate MGMT-methylated patients from MGMT-unmethylated patients in the internal validation cohort, we can reasonably speculate that the radiomics models derived from the combination of structural MRI, ADC, and SWI cannot differentiate MGMT promoter status effectively. (II) The ADC value used in this study was calculated with b0 and b1000 maps, which was heavily contributed by T2-weighted effects rather than true diffusion. This can result in higher ADC values in regions where T2-relaxation is prolonged, making it difficult to distinguish between restricted diffusion and T2-related signal changes (36,37). We will try other advanced diffusion models such as diffusion tensor imaging and diffusion kurtosis imaging to better define diffusion restriction. (III) The labels used in training cohort were skewed. There were obviously more high-grade glioma patients than low-grade glioma patients, more IDH-wildtype patients than IDH-mutant patients, and more MGMT-methylated patients than MGMT-unmethylated patients. In the future, we will try to implement some data augmentation techniques such as synthetic minority over-sampling technique (SMOTE) to oversample the minority class, which may help to balance the dataset and provide a model with more cases of underrepresented classes to broaden the knowledge. We will also attempt to collect and integrate data from multiple institutions to increase the diversity of the dataset. This diversity is crucial for training models that are more generalizable and less prone to overfitting specific to a single center’s characteristics. Models trained with multiple institutional datasets will have better robustness, generalizability, and wider clinical application.
Conclusions
We developed and validated radiomics models which achieved promising performance in assessing WHO grade and IDH mutation status but had no efficacy in predicting MGMT methylation status. Adding SWI and ADC features cannot provide extra information to structural MRI in predicting WHO grade and IDH mutation.
Acknowledgments
This work was presented as an oral presentation in the 2024 International Society for Magnetic Resonance in Medicine.
Funding: This work was supported by
Footnote
Reporting Checklist: The authors have completed the TRIPOD reporting checklist. Available at https://qims.amegroups.com/article/view/10.21037/qims-24-1110/rc
Conflicts of Interest: All authors have completed the ICMJE uniform disclosure form (available at https://qims.amegroups.com/article/view/10.21037/qims-24-1110/coif). The authors have no conflicts of interest to declare.
Ethical Statement: The authors are accountable for all aspects of the work in ensuring that questions related to the accuracy or integrity of any part of the work are appropriately investigated and resolved. The study was conducted in accordance with the Declaration of Helsinki (as revised in 2013). The study was approved by the Ethics Committee of Nanjing Drum Tower Hospital (No. 2022-364-02) and the requirement for individual consent for this retrospective analysis was waived.
Open Access Statement: This is an Open Access article distributed in accordance with the Creative Commons Attribution-NonCommercial-NoDerivs 4.0 International License (CC BY-NC-ND 4.0), which permits the non-commercial replication and distribution of the article with the strict proviso that no changes or edits are made and the original work is properly cited (including links to both the formal publication through the relevant DOI and the license). See: https://creativecommons.org/licenses/by-nc-nd/4.0/.
References
- Louis DN, Perry A, Reifenberger G, von Deimling A, Figarella-Branger D, Cavenee WK, Ohgaki H, Wiestler OD, Kleihues P, Ellison DW. The 2016 World Health Organization Classification of Tumors of the Central Nervous System: a summary. Acta Neuropathol 2016;131:803-20. [Crossref] [PubMed]
- Louis DN, Perry A, Wesseling P, Brat DJ, Cree IA, Figarella-Branger D, Hawkins C, Ng HK, Pfister SM, Reifenberger G, Soffietti R, von Deimling A, Ellison DW. The 2021 WHO Classification of Tumors of the Central Nervous System: a summary. Neuro Oncol 2021;23:1231-51. [Crossref] [PubMed]
- Butler M, Pongor L, Su YT, Xi L, Raffeld M, Quezado M, Trepel J, Aldape K, Pommier Y, Wu J. MGMT Status as a Clinical Biomarker in Glioblastoma. Trends Cancer 2020;6:380-91. [Crossref] [PubMed]
- Cropper JD, Alimbetov DS, Brown KTG, Likhotvorik RI, Robles AJ, Guerra JT, He B, Chen Y, Kwon Y, Kurmasheva RT. PARP1-MGMT complex underpins pathway crosstalk in O(6)-methylguanine repair. J Hematol Oncol 2022;15:146. [Crossref] [PubMed]
- Haque W, Teh C, Butler EB, Teh BS. Prognostic and predictive impact of MGMT promoter methylation status in high risk grade II glioma. J Neurooncol 2022;157:137-46. [Crossref] [PubMed]
- Do DT, Yang MR, Lam LHT, Le NQK, Wu YW. Improving MGMT methylation status prediction of glioblastoma through optimizing radiomics features using genetic algorithm-based machine learning approach. Sci Rep 2022;12:13412. [Crossref] [PubMed]
- Yang K, Wu Z, Zhang H, Zhang N, Wu W, Wang Z, Dai Z, Zhang X, Zhang L, Peng Y, Ye W, Zeng W, Liu Z, Cheng Q. Glioma targeted therapy: insight into future of molecular approaches. Mol Cancer 2022;21:39. [Crossref] [PubMed]
- Yang H, Zhu Z, Long C, Niu F, Zhou J, Chen S, Ye M, Peng S, Zhang X, Chen Y, Wei L, Wang H, Liu D, Yao M, Zhang X, Zhang B. Quantitative and Qualitative Parameters of DCE-MRI Predict CDKN2A/B Homozygous Deletion in Gliomas. Acad Radiol 2024;31:3355-65. [Crossref] [PubMed]
- Buda M, AlBadawy EA, Saha A, Mazurowski MA. Deep Radiogenomics of Lower-Grade Gliomas: Convolutional Neural Networks Predict Tumor Genomic Subtypes Using MR Images. Radiol Artif Intell 2020;2:e180050. [Crossref] [PubMed]
- Rathore S, Mohan S, Bakas S, Sako C, Badve C, Pati S, et al. Multi-institutional noninvasive in vivo characterization of IDH, 1p/19q, and EGFRvIII in glioma using neuro-Cancer Imaging Phenomics Toolkit (neuro-CaPTk). Neurooncol Adv 2020;2:iv22-34. [Crossref] [PubMed]
- Li Y, Wei D, Liu X, Fan X, Wang K, Li S, Zhang Z, Ma K, Qian T, Jiang T, Zheng Y, Wang Y. Molecular subtyping of diffuse gliomas using magnetic resonance imaging: comparison and correlation between radiomics and deep learning. Eur Radiol 2022;32:747-58. [Crossref] [PubMed]
- Choi YS, Bae S, Chang JH, Kang SG, Kim SH, Kim J, Rim TH, Choi SH, Jain R, Lee SK. Fully automated hybrid approach to predict the IDH mutation status of gliomas via deep learning and radiomics. Neuro Oncol 2021;23:304-13. [Crossref] [PubMed]
- Chen F, Zhang X, Li M, Wang R, Wang HT, Zhu F, Lu DJ, Zhao H, Li JW, Xu Y, Zhu B, Zhang B. Axial diffusivity and tensor shape as early markers to assess cerebral white matter damage caused by brain tumors using quantitative diffusion tensor tractography. CNS Neurosci Ther 2012;18:667-73. [Crossref] [PubMed]
- Yogananda CGB, Shah BR, Nalawade SS, Murugesan GK, Yu FF, Pinho MC, Wagner BC, Mickey B, Patel TR, Fei B, Madhuranthakam AJ, Maldjian JA. MRI-Based Deep-Learning Method for Determining Glioma MGMT Promoter Methylation Status. AJNR Am J Neuroradiol 2021;42:845-52. [Crossref] [PubMed]
- Chen S, Xu Y, Ye M, Li Y, Sun Y, Liang J, Lu J, Wang Z, Zhu Z, Zhang X, Zhang B. Predicting MGMT Promoter Methylation in Diffuse Gliomas Using Deep Learning with Radiomics. J Clin Med 2022;11:3445. [Crossref] [PubMed]
- Xi YB, Guo F, Xu ZL, Li C, Wei W, Tian P, Liu TT, Liu L, Chen G, Ye J, Cheng G, Cui LB, Zhang HJ, Qin W, Yin H. Radiomics signature: A potential biomarker for the prediction of MGMT promoter methylation in glioblastoma. J Magn Reson Imaging 2018;47:1380-7. [Crossref] [PubMed]
- Kim BH, Lee H, Choi KS, Nam JG, Park CK, Park SH, Chung JW, Choi SH. Validation of MRI-Based Models to Predict MGMT Promoter Methylation in Gliomas: BraTS 2021 Radiogenomics Challenge. Cancers (Basel) 2022.
- Saini J, Gupta PK, Sahoo P, Singh A, Patir R, Ahlawat S, Beniwal M, Thennarasu K, Santosh V, Gupta RK. Differentiation of grade II/III and grade IV glioma by combining "T1 contrast-enhanced brain perfusion imaging" and susceptibility-weighted quantitative imaging. Neuroradiology 2018;60:43-50. [Crossref] [PubMed]
- Yang X, Lin Y, Xing Z, She D, Su Y, Cao D. Predicting 1p/19q codeletion status using diffusion-, susceptibility-, perfusion-weighted, and conventional MRI in IDH-mutant lower-grade gliomas. Acta Radiol 2021;62:1657-65. [Crossref] [PubMed]
- Kong LW, Chen J, Zhao H, Yao K, Fang SY, Wang Z, Wang YY, Li SW. Intratumoral Susceptibility Signals Reflect Biomarker Status in Gliomas. Sci Rep 2019;9:17080. [Crossref] [PubMed]
- Lin Y, Xing Z, She D, Yang X, Zheng Y, Xiao Z, Wang X, Cao D. IDH mutant and 1p/19q co-deleted oligodendrogliomas: tumor grade stratification using diffusion-, susceptibility-, and perfusion-weighted MRI. Neuroradiology 2017;59:555-62. [Crossref] [PubMed]
- Yang X, Xing Z, She D, Lin Y, Zhang H, Su Y, Cao D. Grading of IDH-mutant astrocytoma using diffusion, susceptibility and perfusion-weighted imaging. BMC Med Imaging 2022;22:105. [Crossref] [PubMed]
- Calabrese E, Villanueva-Meyer JE, Rudie JD, Rauschecker AM, Baid U, Bakas S, Cha S, Mongan JT, Hess CP. The University of California San Francisco Preoperative Diffuse Glioma MRI Dataset. Radiol Artif Intell 2022;4:e220058. [Crossref] [PubMed]
- Clark K, Vendt B, Smith K, Freymann J, Kirby J, Koppel P, Moore S, Phillips S, Maffitt D, Pringle M, Tarbox L, Prior F. The Cancer Imaging Archive (TCIA): maintaining and operating a public information repository. J Digit Imaging 2013;26:1045-57. [Crossref] [PubMed]
- Lasocki A, Anjari M, Ӧrs Kokurcan S, Thust SC. Conventional MRI features of adult diffuse glioma molecular subtypes: a systematic review. Neuroradiology 2021;63:353-62. [Crossref] [PubMed]
- Yu W, Wu P, Wang F, Miao L, Han B, Jiang Z. Construction of Novel Methylation-Driven Gene Model and Investigation of PARVB Function in Glioblastoma. Front Oncol 2021;11:705547. [Crossref] [PubMed]
- Xie Z, Li J, Zhang Y, Zhou R, Zhang H, Duan C, Liu S, Niu L, Zhao J, Liu Y, Song S, Liu X. The diagnostic value of ADC histogram and direct ADC measurements for coexisting isocitrate dehydrogenase mutation and O6-methylguanine-DNA methyltransferase promoter methylation in glioma. Front Neurosci 2022;16:1099019. [Crossref] [PubMed]
- Kihira S, Mei X, Mahmoudi K, Liu Z, Dogra S, Belani P, Tsankova N, Hormigo A, Fayad ZA, Doshi A, Nael K. U-Net Based Segmentation and Characterization of Gliomas. Cancers (Basel) 2022.
- Maynard J, Okuchi S, Wastling S, Busaidi AA, Almossawi O, Mbatha W, Brandner S, Jaunmuktane Z, Koc AM, Mancini L, Jäger R, Thust S. World Health Organization Grade II/III Glioma Molecular Status: Prediction by MRI Morphologic Features and Apparent Diffusion Coefficient. Radiology 2021;298:E61. [Crossref] [PubMed]
- Zhang HW, Lyu GW, He WJ, Lei Y, Lin F, Wang MZ, Zhang H, Liang LH, Feng YN, Yang JH. DSC and DCE Histogram Analyses of Glioma Biomarkers, Including IDH, MGMT, and TERT, on Differentiation and Survival. Acad Radiol 2020;27:e263-71. [Crossref] [PubMed]
- Cao M, Wang X, Liu F, Xue K, Dai Y, Zhou Y. A three-component multi-b-value diffusion-weighted imaging might be a useful biomarker for detecting microstructural features in gliomas with differences in malignancy and IDH-1 mutation status. Eur Radiol 2023;33:2871-80. [Crossref] [PubMed]
- Robinet L, Siegfried A, Roques M, Berjaoui A, Cohen-Jonathan Moyal E. MRI-Based Deep Learning Tools for MGMT Promoter Methylation Detection: A Thorough Evaluation. Cancers (Basel) 2023;15:2253. [Crossref] [PubMed]
- Chang P, Grinband J, Weinberg BD, Bardis M, Khy M, Cadena G, Su MY, Cha S, Filippi CG, Bota D, Baldi P, Poisson LM, Jain R, Chow D. Deep-Learning Convolutional Neural Networks Accurately Classify Genetic Mutations in Gliomas. AJNR Am J Neuroradiol 2018;39:1201-7. [Crossref] [PubMed]
- Jian A, Jang K, Manuguerra M, Liu S, Magnussen J, Di Ieva A. Machine Learning for the Prediction of Molecular Markers in Glioma on Magnetic Resonance Imaging: A Systematic Review and Meta-Analysis. Neurosurgery 2021;89:31-44. [Crossref] [PubMed]
- Rudie JD, Rauschecker AM, Bryan RN, Davatzikos C, Mohan S. Emerging Applications of Artificial Intelligence in Neuro- Oncology. Radiology 2019;290:607-18. [Crossref] [PubMed]
- Wáng YXJ, Ma FZ. A tri-phasic relationship between T2 relaxation time and magnetic resonance imaging (MRI)-derived apparent diffusion coefficient (ADC). Quant Imaging Med Surg 2023;13:8873-80. [Crossref] [PubMed]
- Wáng YXJ, Zhao KX, Ma FZ, Xiao BH. The contribution of T2 relaxation time to MRI-derived apparent diffusion coefficient (ADC) quantification and its potential clinical implications. Quant Imaging Med Surg 2023;13:7410-6. [Crossref] [PubMed]