Alterations in the white matter fiber tracts of preschool-aged children with autism spectrum disorder: an automated fiber quantification study
Introduction
Autism spectrum disorder (ASD) is a specific type of neurodevelopmental disorder in clinical practice. The core symptoms of ASD include deficits in social interaction, communication impairments, and restricted interests or stereotyped behaviours. Previous neuroimaging studies have indicated that the neuropathology of ASD involves atypical neural connectivity, including functional connectivity and structural connectivity among multiple brain regions (1,2). Abnormal functional connections are related to impairments in the coherence of white matter (WM) trajectories, which typically facilitate rapid and effective neuronal communication across multiple brain regions (3). Abnormal structural connectivity of the WM leads to decreased functional connectivity between brain regions in ASD patients. The findings of previous neuroimaging studies have suggested that deficits in the WM could contribute to specific neurodevelopmental abnormalities observed in individuals with ASD, thereby affecting their social and language ability (4,5). Hence, investigating the microstructural properties of WM in ASD patients could improve our understanding of the underlying neural mechanisms of this disorder.
Diffusion tensor imaging (DTI) technique is a noninvasive magnetic resonance imaging (MRI) modality that is highly sensitive to subtle WM fiber tract abnormalities (6). Fractional anisotropy (FA), mean diffusivity (MD), axial diffusivity (AD), and radial diffusivity (RD) are often measured with DTI to characterize the microstructural properties of the WM, such as the density, coherence, and orientation of fiber tracts, as well as the degree of myelination and WM integrity (7). Combining these measures provided multiple ways to examine diffusion alterations in WM fiber tracts, thus allowing for the study of potential changes in the WM microstructures in individuals with neurodevelopmental disorders such as ASD (8). Despite the extensive application of DTI in ASD research, the findings remain inconclusive. The majority of DTI studies conducted in adolescent and adult ASD patients have consistently reported that compared with the healthy population, individuals with ASD exhibit decreased FA, and increased MD and RD within several WM fiber tracts, including the corpus callosum (CC), cingulum bundle, uncinate fasciculus (UF), inferior frontal-occipital fasciculus (IFOF), and superior longitudinal fasciculus (SLF) in the brain, as determined with voxel-based analysis (VBA) and tract-based spatial statistics (TBSS), compared with the healthy population (9,10). A recent meta-analysis of DTI studies examining major WM fiber tracts in infants and young children with ASD revealed increased FA and decreased RD in the CC, UF, and inferior longitudinal fasciculus (ILF), whereas reduced FA and increased RD were observed in the IFOF (11). However, several studies have reported that there are no significant differences in the DTI metrics of WM fiber tracts between patients with ASD and non-ASD individuals (12,13). These inconsistent findings may be attributed to differences in the methodologies employed and in the age ranges of individuals with ASD included in the studies. In addition, conventional DTI methods only examine the microstructural properties of WM fiber tracts at the global level, while the impaired segments of WM fiber tracts cannot be identified (14), as WM fiber tracts in the brain of ASD brain may differ at different developmental stages (15).
The automated fiber quantification (AFQ) technique is a novel DTI approach that enables automated reconstruction of WM fiber tracts and yields measurements obtained at anatomically equivalent locations along their trajectories (16). The diffusion properties of tissues within the fiber tract may differ at different locations due to the presence of diseases or injuries in specific, local portions of the tracts. Compared with TBSS and VBA, AFQ can more sensitively detect local abnormalities that may be masked by the whole, while also providing more comprehensive insights into the microstructural characteristics of WM (17). AFQ has been widely used to examine a variety of psychiatric disorders, such as mild cognitive impairment (18), Alzheimer’s disease (19), and cerebral small vessel diseases (20).
In a previous DTI study of adolescents and adults with ASD, AFQ was used to localize impairments of the left SLF at the anterior portion of the fiber tract (21). However, as ASD is an early-onset disorder, the studies of older ASD patients may reveal causal or compensatory differences, but not disorder-related differences. Thus, knowledge of the precise location of impaired WM fiber tracts in preschool-aged children with ASD will enhance our understanding of the pathogenesis of the disorder and provide more effective diagnostic guidance. In the present study, we used AFQ to (I) investigate the potential WM impairment in preschool-aged children with ASD located at 20 major WM fiber tracts along with 100 continued nodes; and (II) explore the relationships between the diffusion metrics and the severity of clinical manifestations. We present this article in accordance with the STROBE reporting checklist (available at https://qims.amegroups.com/article/view/10.21037/qims-24-950/rc).
Methods
The study was conducted in accordance with the Declaration of Helsinki (as revised in 2013). The study was approved by the Research Ethics Committee of the Children’s Hospital of Chongqing Medical University (IRB number: [2022] Ethical Review [Research] No. [582]). The legal guardians of all participants provided informed consent for their participation before the MRI scans.
Participants
A total of 50 children who were diagnosed for the first time with ASD and 50 age- and sex-matched typically developing children (TDC), aged between 2 and 6 years, were recruited for this study between December 2022 and January 2024. Clinical and imaging data were collected from all participants. ASD was diagnosed in strict accordance with the Diagnostic and Statistical Manual of Mental Disorders (DSM-V) (22) and the Childhood Autism Rating Scale (CARS). The inclusion criteria for the ASD group were as follows: (I) the individual with ASD met the diagnostic criteria outlined in the DSM-V; and (II) a CARS score ≥30 points. The ASD participants were excluded if they met any of the following criteria: (I) neurological diseases (such as seizures or encephalitis), mental diseases, or genetic syndromes commonly associated with ASD, such as fragile X syndrome and Rett syndrome; (II) receiving treatment with medication that included psychoactive compounds; and (III) focal or diffuse lesions on MRI. The TDC group was recruited through advertisements and underwent a general physical examination and a routine MRI sequence scan. The inclusion criterion was no significant growth or developmental abnormalities on the general physical examination. The exclusion criteria for the TDC group were the same as those for the ASD group. After visual quality control of the MRI data, 43 children were included in the ASD group and 42 were included in the TDC group (Figure 1).
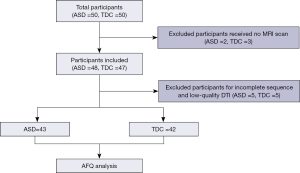
MRI data acquisition
Each participant underwent MRI examinations with a 3.0 Tesla MR scanner (Achieva, Philips; The Netherlands) equipped with an 8-channel head coil. All patients in the ASD group and some participants in the TDC group were sedated to reduce motion artifacts during scanning. After the subjects were evaluated by anesthesiologists and showed no contraindications and parental informed consent was obtained, propofol (loading dose of 1 mg/kg, followed by 200 µg/kg/min) was administered intravenously to achieve mild to moderate sedation.
A three-dimensional (3D), high-resolution sagittal T1-weighted imaging (T1WI) scan with turbo-fast echo acquisition was performed. The parameters were as follows: repetition time (TR) =7.7 ms, echo time (TE) =3.4 ms, flip angle =8°, slice thickness =1.1 mm, gap =0 mm, field of view (FOV) =250 mm × 250 mm, matrix size =228×227, voxel size =1.04 mm × 1.04 mm × 1.04 mm, and total time = 5 min 35 s. The DTI scan was acquired via a single-shot, echo planar imaging (EPI) sequence with the following parameters: TR =9,611 ms, TE =65 ms, matrix size =128×126, FOV = 230 mm × 230 mm, slice thickness =2 mm, and voxel size = 1.8 mm × 1.8 mm × 2.0 mm with both 32-directional diffusion encoding (b=1,000 s/mm2 for each direction) and no diffusion encoding (b =0 s/mm2).
Data preprocessing
All the images were converted from DICOM format to NIFTI format files with MRIcron (http://www.mricron.com). For DTI data, FMRIB Software Library (FSL) software (version 5.0.9; http://fsl.fmrib.ox.ac.uk/fsl/fslwiki/) was used for preprocessing, which included the following steps. First, the diffusion-weighted images were aligned with the b0 image to maximize the normalized mutual information. Second, the eddy correction tool from FIMRIB’s diffusion toolbox (FDT) was utilized to correct for eddy currents and head movement. Third, individual brain masks were generated with the brain extraction tool (BET) at a threshold of 0.20 (23). Finally, the DTIFIT tool from FDT was employed to fit the tensor model to each voxel within the brain mask, enabling computation of the eigenvalues (λ1, λ2, and λ3) and eigenvectors on a voxel-wise basis. By the mentioned steps, the maps of diffusion metrics, such as the FA, AD, RD, and MD, and a raw T2-weighted image without diffusion weighting (S0) were acquired.
The 3D T1WI image was subsequently preprocessed according to the following steps. First, non-brain structures were removed with the BET. Second, the anterior commissure-posterior commissure (AC-PC) plane was calibrated with the mrAnatAverageAcpcNifti function in the VistaSoft package (http://github.com/vistalab/vistasoft) for subsequent alignment. Finally, the T1WI images for each participant were utilized as a reference for aligning with the S0 image to minimize the displacement of DTI data. The DTI data were transformed into a format suitable for AFQ processing in MATLAB R2012a.
The pipeline of AFQ
Twenty major WM fiber tracts across the whole brain were automatically extracted with the AFQ1.2 package (https://github.com/yeatmanlab/AFQ) running in MATLAB R2012a. The main processing steps of AFQ were as follows (24): (I) whole-brain fiber tracts tracking with deterministic fiber tractography, with thresholds of FA >0.2, turning angle <30°, and fiber length between 50 and 250 mm; (II) segmentation of the candidate fiber tracts based on the waypoint region of interest; (III) refinement of the candidate fiber tracts based on probability maps; (IV) use of five iterations to clean fiber tracts, and removing fiber tracts with deviated properties (fiber length exceeding the mean length ± 4 standard deviation (SD); distance from the tract core exceeding the 5 SD); and (V) quantification and measurement of DTI metrics (FA, MD, RD, and AD) at 100 equidistant nodes along each fiber tract.
In the present study, the identified WM included the anterior thalamic radiation (ATR), corticospinal tract (CST), cingulum cingulate (CGC), cingulum hippocampus (CGH), IFOF, ILF, SLF, UF, arcuate fasciculus (AF), callosum forceps major (FMA), and callosum forceps minor (FMI).
Statistical analysis
The clinical data and DTI metrics were compared between the ASD and TDC groups with SPSS 26.0 software (SPSS Inc, Chicago, IL, USA). The independent sample t-test or Mann-Whitney U test was used to assess between-group differences for normally or nonnormally distributed data, respectively. The sex distributions of the groups were compared with Pearson’s chi-square tests. The level of statistical significance was set at P<0.05. The four DTI metrics (FA, MD, RD, and AD) of 16 identified WM fiber tracts were compared between the ASD and TDC groups at the entire and point-wise levels with a general linear model while controlling for age and sex. The significance level was set at P<0.05 after the false discovery rate (FDR) correction was applied for multiple comparisons. At the entire level, the number of tests for FDR correction was 16. At the point-wise level, the number of tests for FDR correction was 1,600. For the point-wise level analysis, we reported the results only when more than three adjacent nodes demonstrated significant differences (25).
After controlling for age and sex as potential covariates, we evaluated the relationships between the mean diffusion metrics in altered fiber tracts at the entire level and the CARS score in the ASD group with Pearson’s correlation analysis. Additional correlation analyses were performed between the diffusion profiles of significantly altered fiber segments and the CARS score in the ASD group. The significance level was set at P<0.05.
Results
Demographic data and CARS scores
The demographic characteristics and CARS assessments of the participants are presented in Table 1. There were no significant differences in age (P=0.124) or sex (P=0.759) between the children in the ASD and TDC groups.
Table 1
Characteristics | ASD (N=43) | TDC (N=42) | P |
---|---|---|---|
Age, years | 3.85±1.55 | 4.40±1.70 | 0.124a |
Sex (male/female) | 30/13 | 28/14 | 0.759b |
CARS | 36.81±4.01 | None |
Values are reported as number or mean ± standard deviation. a, the P value was obtained by two-sample t-tests; b, the P value was obtained by Chi-squared test. ASD, autism spectrum disorder; TDC, typical developing children; CARS, Childhood Autism Rating Scale.
Identification of WM fiber tracts in participants
Due to the strict criteria employed by AFQ for identifying WM fiber tracts, the percentage of successful tracts of the bilateral CGH and AF was less than 80%. Consequently, we excluded four fiber tracts and included the remaining 16 for further analysis (Table 2, Figure 2).
Table 2
Item | Tract | Number of successful tract | Ratio of successful tract | |||||
---|---|---|---|---|---|---|---|---|
ASD | TDC | Total | ASD (%) | TDC (%) | Total (%) | |||
1 | ATR.L | 43 | 42 | 85 | 100 | 100 | 100 | |
2 | ATR.R | 42 | 41 | 83 | 97.6 | 97.6 | 97.6 | |
3 | CST.L | 43 | 41 | 84 | 100 | 97.6 | 98.8 | |
4 | CST.R | 43 | 41 | 84 | 100 | 97.6 | 98.8 | |
5 | CGC.L | 42 | 40 | 82 | 97.6 | 95.2 | 96.5 | |
6 | CGC.R | 38 | 38 | 76 | 88.4 | 90.5 | 89.4 | |
7 | CGH.L | 15 | 14 | 29 | 34.9 | 33.3 | 34.1 | |
8 | CGH.R | 16 | 15 | 31 | 37.2 | 35.7 | 36.5 | |
9 | FMA | 41 | 41 | 82 | 95.3 | 97.6 | 96.5 | |
10 | FMI | 43 | 42 | 85 | 100 | 100 | 100 | |
11 | IFOF.L | 42 | 42 | 84 | 97.6 | 100 | 98.8 | |
12 | IFOF.R | 42 | 42 | 84 | 97.6 | 100 | 98.8 | |
13 | ILF.L | 42 | 41 | 83 | 97.6 | 97.6 | 97.6 | |
14 | ILF.R | 39 | 41 | 80 | 90.7 | 97.6 | 94.1 | |
15 | SLF.L | 38 | 38 | 76 | 88.4 | 90.5 | 89.4 | |
16 | SLF.R | 42 | 40 | 82 | 97.6 | 95.2 | 96.5 | |
17 | UF. L | 43 | 42 | 85 | 100 | 100 | 100 | |
18 | UF. R | 43 | 42 | 85 | 100 | 100 | 100 | |
19 | AF. L | 34 | 30 | 64 | 79.1 | 71.4 | 75.3 | |
20 | AF. R | 35 | 27 | 62 | 81.4 | 64.3 | 72.9 |
WM, white matter; ASD, autism spectrum disorder; TDC, typical developing children; ATR, anterior thalamic radiation; CST, corticospinal tract; CGC, cingulum cingulate; CGH, cingulum hippocampus; FMA, callosum forceps major; FMI, callosum forceps minor; IFOF, inferior frontal-occipital fasciculus; ILF, inferior longitudinal fasciculus; SLF, superior longitudinal fasciculus; UF, uncinate fasciculus; AF, arcuate fasciculus; L, left; R, right.
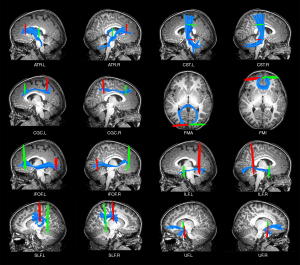
Alterations of the WM fiber tracts at the entire level
Compared with the TDC group, the ASD group presented significant decreases in the FA values of the left ILF and FMI (all P<0.05 after FDR correction). For MD, the ASD groups showed a significant increment in the left IFOF and left ILF (all P<0.05 after FDR correction). For RD, the ASD groups showed a significant increment in the left ILF, FMI, and left IFOF (all P<0.05 after FDR correction). The AD values of whole WM fiber tracts were not significantly different between the two groups (P>0.05 after FDR correction). The group differences in all DTI metrics of whole WM fiber tracts, including FA, MD, and RD, are displayed in Table 3 and Figure 3.
Table 3
Metrics | Fiber tracts | ASD | TDC | t | P | P (after FDR correction) |
---|---|---|---|---|---|---|
FA | FMI | 0.530±0.027 | 0.551±0.029 | −2.687 | 0.001 | 0.02 |
ILF.L | 0.364±0.030 | 0.385±0.031 | −3.301 | 0.009 | 0.04 | |
MD | IFOF.L | 0.874±0.028 | 0.850±0.029 | 3.349 | 0.001 | 0.016 |
ILF.L | 0.908±0.046 | 0.879±0.038 | 2.572 | 0.012 | 0.048 | |
RD | FMI | 0.558±0.035 | 0.532±0.036 | 3.035 | 0.003 | 0.024 |
IFOF.L | 0.671±0.038 | 0.642±0.042 | 2.912 | 0.005 | 0.026 | |
ILF.L | 0.720±0.047 | 0.685±0.042 | 3.134 | 0.002 | 0.024 |
The comparison of DTI metrics between ASD and TDC groups showed a significant difference (P<0.05, after FDR correction). FA, MD, and RD values are reported as mean ± standard deviation. DTI, diffusion tensor imaging; WM, white matter; ASD, autism spectrum disorder; TDC, typical developing children; FA, fractional anisotropy; MD, mean diffusion; RD, radial diffusivity; FMI, callosum forceps minor; ILF, inferior longitudinal fasciculus; IFOF, inferior frontal-occipital fasciculus; FDR, false discovery rate; L, left; R, right.
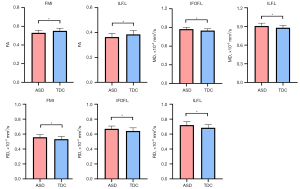
Alterations of the WM fiber tracts at the point-wise level
The results of the point-wise comparisons of the DTI metrics are displayed in Figure 4.
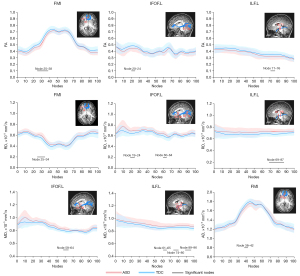
Compared with the TDC group, the ASD group exhibited a significantly decreased FA in the fiber tracts, namely the FMI (nodes 25 to 38), left IFOF (nodes 20 to 24), and left ILF (nodes 71 to 76) (all P<0.05 after FDR correction). Similarly, the AD also exhibited a significant decrease in the FMI (nodes 38 to 42). Conversely, there were significantly increased MD in the left IFOF (nodes 58 to 64), FMI (nodes 38 to 42), and left ILF (nodes 61 to 65, nodes 74 to 80, and nodes 89 to 96) (all P<0.05 after FDR correction). Moreover, the increases of RD were also observed in the FMI (nodes 25 to 34), left IFOF (nodes 19 to 24, nodes 60 to 64), and left ILF (nodes 69 to 87) (all P < 0.05 after FDR correction).
Correlations between DTI metrics and CARS scores
Figure 5 shows that the mean FA in the posterior part of the left ILF was negatively correlated with the CARS score in preschool-aged children with ASD (r=−0.475, P<0.001). No significant correlations were identified between any of the other DTI metrics of the other fiber tracts at either the global or point levels and the CARS score (all P>0.05).
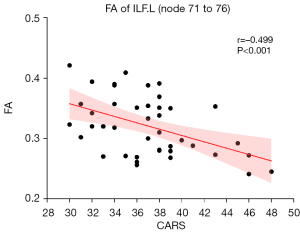
Discussion
In the present study, we investigated the integrity of WM fiber tracts in preschool-aged children with ASD via AFQ and examined the relationships between the DTI metrics of WM fiber tracts and the severity of autism manifestations. The results displayed that impairment of WM microstructure in preschool-aged children with ASD showed characteristics of segments, and was distributed mainly in the left cerebral hemisphere. The segmental impairment of the FMI, left ILF, and IFOF may be associated with high-order visual processing and emotional response dysfunction observed in preschool-aged children with ASD. In addition, the lower FA of the posterior segment of the left ILF was inversely associated with the CARS score in preschool-aged children with ASD. These findings indicate that the impairment of the FMI, left ILF, and left IFOF, especially the posterior segment of the left ILF, plays an important role in the neuropathology of ASD.
Previous studies have demonstrated significantly abnormal left-lateralization of structural and functional brain organization in individuals with ASD (26,27). Abnormal lateralization of WM fiber tracts in language-related brain regions, including the left UF, SLF, ILF, and IFOF, which are integral to core language functions, may be associated with the language impairments observed in individuals with ASD (28). In the current study, the entire analysis of the WM fiber tracts revealed that the impaired WM fiber tracts in preschool-aged children with ASD were distributed mainly in the left hemisphere, similar to the results mentioned above. Moreover, we successfully identified the specific regions containing impaired fiber tracts in individuals with ASD. These findings imply that the localized impairment of fiber tracts within the left hemisphere may serve as an underlying pathogenic mechanism contributing to the abnormal behaviours observed in individuals with ASD. However, unlike the findings from a previous study (29), the right hemisphere did not contain impaired WM fiber tracts in the current study. Fu et al. reported that the increased FA in preschool-aged children with ASD was mainly found in the WM fiber tracts of the right cerebral hemisphere, suggesting rightward asymmetry (30). We speculate that the differences may be due to the age range of the participants and the processing methods employed in our study, which differ from those in other studies. The underlying mechanism requires further investigation in future studies.
Our findings are similar to the results reported by Naigles et al. (31) in younger males with ASD, where a decreased FA and increased MD were observed in the bilaterally ILF. However, previous DTI studies employing TBSS suggested that the FA value of WM fiber tracts in children with ASD aged 2 to 7 years was either greater than or not significantly different from that of children in the TDC (30,32). We speculate that this may be due to differences between the research methods or cohorts among the studies. Previous DTI studies focusing on ASD from mid-childhood to adulthood have consistently reported a decrease in FA and an increase in MD within WM fiber tracts when compared with healthy individuals (33,34). At the entire level, the left ILF and FMI exhibited a decreased FA in the current study. These findings may suggest that FA decreases previously observed in older children, adolescents, and adults with ASD may develop across younger childhood as well (35). In the future, longitudinal and multicenter studies should be conducted to elucidate the distinctive characteristics of WM developmental alterations in individuals with ASD.
The ILF is the main WM fiber tract that connects the temporal lobe with the fusiform gyrus and dorsolateral part of the occipital lobe (36). This part of the brain is related to the recognition of objects, faces, and emotional processing (37). Ameis et al. (38) reported that injury to the left ILF played an important role in facial expression recognition dysfunction in individuals with ASD. Yin et al. (39) reported that the decreased FA of left ILF was significantly associated with poorer comprehension ability. Similarly, at the entire level, the left ILF also exhibited a reduction in FA and an increase in MD in ASD children, suggesting the presence of aberrant structural integrity of the left ILF in our study cohort. In addition, the microstructural abnormalities of the ILF might vary along its WM trajectory in younger children with ASD. Through point-wise analysis, we found that the main impaired region of the left ILF was located at its posterior segment, which was structurally connected with the anterior segments of the superior, middle, and inferior temporal gyri (40). Impairment of the ILF may disrupt the connectivity between visual inputs originating from the occipital and temporal lobes, resulting in impaired emotional responses to visual stimuli (41,42). We speculate that the reduced integrity in the posterior part of the left ILF may be a structural basis of the dysfunction in visual inputs and emotional responses in ASD patients. More importantly, we found that the integrity of the posterior segment of the left ILF was inversely related to the severity of autistic symptoms. Therefore, the left ILF may be a target fiber tract for further study of ASD.
The FMI is a part of the CC that is closely linked to cognitive function and emotional regulation. The atypical development of the FMI may impact the integration and exchange of information between the bilateral frontal lobes, subsequently leading to dysfunction of cognitive and social abilities in individuals with ASD (43,44). Similar to the findings of previous studies (9), our study also suggested that the FMI displayed a reduction in integrity, which might be responsible for the altered coordination of movement and perception in the ASD group. In the present study, according to AFQ, the impaired segment of the FMI was located at the anterior part of the WM fiber tract, which was connected to the right frontal lobe. This part of the brain is associated with various cognitive, social, and executive functions. Another study revealed that ASD patients had significantly reduced interhemispheric functional connectivity between the bilateral medial prefrontal lobes (45). Moreover, our study revealed the decreased AD of the anterior part of the FMI, which indicated axonal impairment and altered axonal integrity (46). Therefore, we speculate that abnormalities in the exchange of information between the bilateral frontal lobes in ASD individuals are related to the impairment of the FMI.
The IFOF is a long-range intrahemispheric tract that originates in the occipital cortex, traverses the posterior inferior temporal cortex, and terminates in the inferior frontal gyrus and dorsolateral prefrontal cortex (47). This fiber tract plays key roles in visual semantic processing, syntactic integration, and emotional recognition (48-50). Previous studies have suggested that impairment of the IFOF is related to atypical semantic processing and difficulty in emotion recognition in individuals with ASD (51,52). The findings of our study suggested that the left IFOF exhibited atypical myelination, which manifests as elevated RD and MD values in preschool-aged children with ASD. The inconsistency among related studies may be attributed to the ASD subtypes or age ranges employed. In particular, in the point-wise analysis conducted in the present study, the impairment of the left IFOF was located at the anterior part of the WM fiber tract, which was connected with the left occipital lobe and fusiform gyrus (53). Therefore, impairment to the anterior part of the left IFOF could hinder the integration of affective responses and early visual information, resulting in impaired emotion recognition from facial expressions among individuals with ASD and potentially contributing to their social impairments.
There are several limitations in the current study. First, there was a slight disparity in the sex distribution between the two groups. However, importantly, this is a common phenomenon in ASD studies because of differences in symptom prevalence between the sexes. Although we accounted for sex variations across the groups, it is possible that the sex disparity could have influenced the findings. Future studies on ASD should take into careful consideration the impact of sex on brain structure and function. Second, the current study employed a moderate sample size, potentially impacting the statistical power. Future research with a larger sample size is needed to validate these results. Finally, we used only the CARS to diagnose ASD and to assess the severity of the disease. A variety of assessment tools, such as the Autism Diagnostic Observation Scale and Social Responsiveness Scale, should be used to diagnose and evaluate the clinical symptoms of ASD. This would aid in establishing correlations between core symptoms of ASD and DTI metrics.
Conclusions
AFQ has the potential to accurately identify the specific aberrant patterns within the WM fiber tracts of individuals with ASD. The present study provides evidence of the aberrant development of lateralization in the left hemisphere in preschool-aged children with ASD. These affected fiber tracts may be implicated in social and language impairments, thereby establishing an anatomical basis for the observed neurological dysfunction in individuals with ASD. Additionally, the decreased integrity of the posterior part of the left ILF may reflect the severity of autistic symptoms.
Acknowledgments
We especially appreciate Professor Tingyu Li for assisting us in clinical expertise and data collection.
Funding: This work was supported by
Footnote
Reporting Checklist: The authors have completed the STROBE reporting checklist. Available at https://qims.amegroups.com/article/view/10.21037/qims-24-950/rc
Conflicts of Interest: All authors have completed the ICMJE uniform disclosure form (available at https://qims.amegroups.com/article/view/10.21037/qims-24-950/coif). The authors have no conflicts of interest to declare.
Ethical Statement: The authors are accountable for all aspects of the work in ensuring that questions related to the accuracy or integrity of any part of the work are appropriately investigated and resolved. The study was conducted in accordance with the Declaration of Helsinki (as revised in 2013). The study was approved by the Research Ethics Committee of the Children’s Hospital of Chongqing Medical University (IRB number: [2022] Ethical Review [Research] No. [582]). The legal guardians of all participants provided informed consent for their participation before the MRI scans.
Open Access Statement: This is an Open Access article distributed in accordance with the Creative Commons Attribution-NonCommercial-NoDerivs 4.0 International License (CC BY-NC-ND 4.0), which permits the non-commercial replication and distribution of the article with the strict proviso that no changes or edits are made and the original work is properly cited (including links to both the formal publication through the relevant DOI and the license). See: https://creativecommons.org/licenses/by-nc-nd/4.0/.
References
- Lepping RJ, McKinney WS, Magnon GC, Keedy SK, Wang Z, Coombes SA, Vaillancourt DE, Sweeney JA, Mosconi MW. Visuomotor brain network activation and functional connectivity among individuals with autism spectrum disorder. Hum Brain Mapp 2022;43:844-59. [Crossref] [PubMed]
- Peterson BS, Liu J, Dantec L, Newman C, Sawardekar S, Goh S, Bansal R. Using tissue microstructure and multimodal MRI to parse the phenotypic heterogeneity and cellular basis of autism spectrum disorder. J Child Psychol Psychiatry 2022;63:855-70. [Crossref] [PubMed]
- Yang Q, Huang P, Li C, Fang P, Zhao N, Nan J, Wang B, Gao W, Cui LB. Mapping alterations of gray matter volume and white matter integrity in children with autism spectrum disorder: evidence from fMRI findings. Neuroreport 2018;29:1188-92. [Crossref] [PubMed]
- Vogan VM, Morgan BR, Leung RC, Anagnostou E, Doyle-Thomas K, Taylor MJ. Widespread White Matter Differences in Children and Adolescents with Autism Spectrum Disorder. J Autism Dev Disord 2016;46:2138-47. [Crossref] [PubMed]
- Blanken LME, Muetzel RL, Jaddoe VWV, Verhulst FC, van der Lugt A, Tiemeier H, White T. White matter microstructure in children with autistic traits. Psychiatry Res Neuroimaging 2017;263:127-34. [Crossref] [PubMed]
- Feng YZ, Ye YJ, Cheng ZY, Hu JJ, Zhang CB, Qian L, Lu XH, Cai XR. Non-invasive assessment of early stage diabetic nephropathy by DTI and BOLD MRI. Br J Radiol 2020;93:20190562. [Crossref] [PubMed]
- Ding S, Guo Y, Chen X, Du S, Han Y, Yan Z, Zhu Q, Li Y. Demyelination and remyelination detected in an alternative cuprizone mouse model of multiple sclerosis with 7.0 T multiparameter magnetic resonance imaging. Sci Rep 2021;11:11060. [Crossref] [PubMed]
- Haigh SM, Keller TA, Minshew NJ, Eack SM. Reduced White Matter Integrity and Deficits in Neuropsychological Functioning in Adults With Autism Spectrum Disorder. Autism Res 2020;13:702-14. [Crossref] [PubMed]
- Weber CF, Lake EMR, Haider SP, Mozayan A, Mukherjee P, Scheinost D, Bamford NS, Ment L, Constable T, Payabvash S. Age-dependent white matter microstructural disintegrity in autism spectrum disorder. Front Neurosci 2022;16:957018. [Crossref] [PubMed]
- Ouyang M, Cheng H, Mishra V, Gong G, Mosconi MW, Sweeney J, Peng Y, Huang H. Atypical age-dependent effects of autism on white matter microstructure in children of 2-7 years. Hum Brain Mapp 2016;37:819-32. [Crossref] [PubMed]
- Faraji R, Ganji Z, Zamanpour SA, Nikparast F, Akbari-Lalimi H, Zare H. Impaired white matter integrity in infants and young children with autism spectrum disorder: What evidence does diffusion tensor imaging provide? Psychiatry Res Neuroimaging 2023;335:111711. [Crossref] [PubMed]
- Karahanoğlu FI, Baran B, Nguyen QTH, Meskaldji DE, Yendiki A, Vangel M, Santangelo SL, Manoach DS. Diffusion-weighted imaging evidence of altered white matter development from late childhood to early adulthood in Autism Spectrum Disorder. Neuroimage Clin 2018;19:840-7. [Crossref] [PubMed]
- Kato Y, Kagitani-Shimono K, Matsuzaki J, Hanaie R, Yamamoto T, Tominaga K, Watanabe Y, Mohri I, Taniike M. White Matter Tract-Cognitive Relationships in Children with High-Functioning Autism Spectrum Disorder. Psychiatry Investig 2019;16:220-33. [Crossref] [PubMed]
- Hu R, Tan F, Chen W, Wu Y, Jiang Y, Du W, Zuo Y, Gao B, Song Q, Miao Y. Microstructure abnormalities of the diffusion quantities in children with attention-deficit/hyperactivity disorder: an AFQ and TBSS study. Front Psychiatry 2023;14:1237113. [Crossref] [PubMed]
- McLaughlin K, Travers BG, Dadalko OI, Dean DC 3rd, Tromp D, Adluru N, Destiche D, Freeman A, Prigge MD, Froehlich A, Duffield TC, Zielinski BA, Bigler ED, Lange N, Anderson JS, Alexander AL, Lainhart JE. Longitudinal development of thalamic and internal capsule microstructure in autism spectrum disorder. Autism Res 2018;11:450-62. [Crossref] [PubMed]
- Huang H, Ma X, Yue X, Kang S, Li Y, Rao Y, Feng Y, Wu J, Long W, Chen Y, Lyu W, Tan X, Qiu S. White Matter Characteristics of Damage Along Fiber Tracts in Patients with Type 2 Diabetes Mellitus. Clin Neuroradiol 2023;33:327-41. [Crossref] [PubMed]
- Zhang P, Feng Y, Xu T, Li Y, Xia J, Zhang H, Sun Z, Tian W, Zhang J. Brain white matter microstructural alterations in patients with systemic lupus erythematosus: an automated fiber quantification study. Brain Imaging Behav 2024;18:622-9. [Crossref] [PubMed]
- Qu Y, Wang P, Yao H, Wang D, Song C, Yang H, et al. Reproducible Abnormalities and Diagnostic Generalizability of White Matter in Alzheimer's Disease. Neurosci Bull 2023;39:1533-43. [Crossref] [PubMed]
- Zhang X, Sun Y, Li W, Liu B, Wu W, Zhao H, Liu R, Zhang Y, Yin Z, Yu T, Qing Z, Zhu B, Xu Y, Nedelska Z, Hort J, Zhang B. Characterization of white matter changes along fibers by automated fiber quantification in the early stages of Alzheimer's disease. Neuroimage Clin 2019;22:101723. [Crossref] [PubMed]
- Huang L, Chen X, Sun W, Chen H, Ye Q, Yang D, Li M, Luo C, Ma J, Shao P, Xu H, Zhang B, Zhu X, Xu Y. Early Segmental White Matter Fascicle Microstructural Damage Predicts the Corresponding Cognitive Domain Impairment in Cerebral Small Vessel Disease Patients by Automated Fiber Quantification. Front Aging Neurosci 2020;12:598242. [Crossref] [PubMed]
- Libero LE, Burge WK, Deshpande HD, Pestilli F, Kana RK. White Matter Diffusion of Major Fiber Tracts Implicated in Autism Spectrum Disorder. Brain Connect 2016;6:691-9. [Crossref] [PubMed]
- Mukherjee SB. Identification, Evaluation, and Management of Children With Autism Spectrum Disorder: American Academy of Pediatrics 2020 Clinical Guidelines. Indian Pediatr 2020;57:959-62.
- Smith SM. Fast robust automated brain extraction. Hum Brain Mapp 2002;17:143-55. [Crossref] [PubMed]
- Yeatman JD, Dougherty RF, Myall NJ, Wandell BA, Feldman HM. Tract profiles of white matter properties: automating fiber-tract quantification. PLoS One 2012;7:e49790. [Crossref] [PubMed]
- Long X, Zhou Y, Zhang F, Li F, Wang X, Meng Y, Roberts N, Cheng B, Jia Z. Altered MRI Diffusion Properties of the White Matter Tracts Connecting Frontal and Thalamic Brain Regions in First-Episode, Drug-Naive Patients With Postpartum Depression. J Magn Reson Imaging 2023;57:899-906. [Crossref] [PubMed]
- Nielsen JA, Zielinski BA, Fletcher PT, Alexander AL, Lange N, Bigler ED, Lainhart JE, Anderson JS. Abnormal lateralization of functional connectivity between language and default mode regions in autism. Mol Autism 2014;5:8. [Crossref] [PubMed]
- Postema MC, van Rooij D, Anagnostou E, Arango C, Auzias G, Behrmann M, et al. Altered structural brain asymmetry in autism spectrum disorder in a study of 54 datasets. Nat Commun 2019;10:4958. [Crossref] [PubMed]
- Li M, Wang Y, Tachibana M, Rahman S, Kagitani-Shimono K. Atypical structural connectivity of language networks in autism spectrum disorder: A meta-analysis of diffusion tensor imaging studies. Autism Res 2022;15:1585-602. [Crossref] [PubMed]
- Andrews DS, Lee JK, Solomon M, Rogers SJ, Amaral DG, Nordahl CW. A diffusion-weighted imaging tract-based spatial statistics study of autism spectrum disorder in preschool-aged children. J Neurodev Disord 2019;11:32. [Crossref] [PubMed]
- Fu L, Wang Y, Fang H, Xiao X, Xiao T, Li Y, Li C, Wu Q, Chu K, Xiao C, Ke X. Longitudinal Study of Brain Asymmetries in Autism and Developmental Delays Aged 2-5 Years. Neuroscience 2020;432:137-49. [Crossref] [PubMed]
- Naigles LR, Johnson R, Mastergeorge A, Ozonoff S, Rogers SJ, Amaral DG, Nordahl CW. Neural correlates of language variability in preschool-aged boys with autism spectrum disorder. Autism Res 2017;10:1107-19. [Crossref] [PubMed]
- Huang X, Ming Y, Zhao W, Feng R, Zhou Y, Wu L, Wang J, Xiao J, Li L, Shan X, Cao J, Kang X, Chen H, Duan X. Developmental prediction modeling based on diffusion tensor imaging uncovering age-dependent heterogeneity in early childhood autistic brain. Mol Autism 2023;14:41. [Crossref] [PubMed]
- Koolschijn PC, Caan MW, Teeuw J, Olabarriaga SD, Geurts HM. Age-related differences in autism: The case of white matter microstructure. Hum Brain Mapp 2017;38:82-96. [Crossref] [PubMed]
- Bletsch A, Schäfer T, Mann C, Andrews DS, Daly E, Gudbrandsen M, Ruigrok ANV, Dallyn R, Romero-Garcia R, Lai MC, Lombardo MV, Craig MC, Suckling J, Bullmore ET, Baron-Cohen S, Murphy DGM, Dell'Acqua F, Ecker C. Atypical measures of diffusion at the gray-white matter boundary in autism spectrum disorder in adulthood. Hum Brain Mapp 2021;42:467-84. [Crossref] [PubMed]
- Andrews DS, Lee JK, Harvey DJ, Waizbard-Bartov E, Solomon M, Rogers SJ, Nordahl CW, Amaral DG. A Longitudinal Study of White Matter Development in Relation to Changes in Autism Severity Across Early Childhood. Biol Psychiatry 2021;89:424-32. [Crossref] [PubMed]
- Wang Y, Metoki A, Smith DV, Medaglia JD, Zang Y, Benear S, Popal H, Lin Y, Olson IR. Multimodal mapping of the face connectome. Nat Hum Behav 2020;4:397-411. [Crossref] [PubMed]
- Zemmoura I, Burkhardt E, Herbet G. The inferior longitudinal fasciculus: anatomy, function and surgical considerations. J Neurosurg Sci 2021;65:590-604. [Crossref] [PubMed]
- Ameis SH, Catani M. Altered white matter connectivity as a neural substrate for social impairment in Autism Spectrum Disorder. Cortex 2015;62:158-81. [Crossref] [PubMed]
- Yin Y, Xu S, Li C, Li M, Liu M, Yan J, Lan Z, Zhan W, Jiang G, Tian J. Association of Reduced Tract Integrity with Social Communication Deficits in Preschool Autism Children: A Tract-Based Spatial Statistics Study. Neuropsychiatr Dis Treat 2021;17:2003-10. [Crossref] [PubMed]
- Lin YH, Young IM, Conner AK, Glenn CA, Chakraborty AR, Nix CE, Bai MY, Dhanaraj V, Fonseka RD, Hormovas J, Tanglay O, Briggs RG, Sughrue ME. Anatomy and White Matter Connections of the Inferior Temporal Gyrus. World Neurosurg 2020;143:e656-66. [Crossref] [PubMed]
- Catani M, Jones DK, Donato R, Ffytche DH. Occipito-temporal connections in the human brain. Brain 2003;126:2093-107. [Crossref] [PubMed]
- Catani M, Thiebaut de Schotten M. A diffusion tensor imaging tractography atlas for virtual in vivo dissections. Cortex 2008;44:1105-32. [Crossref] [PubMed]
- Kirkovski M, Singh M, Dhollander T, Fuelscher I, Hyde C, Albein-Urios N, Donaldson PH, Enticott PG. An Investigation of Age-related Neuropathophysiology in Autism Spectrum Disorder Using Fixel-based Analysis of Corpus Callosum White Matter Micro- and Macrostructure. J Autism Dev Disord 2024;54:2198-210. [Crossref] [PubMed]
- Porcu M, Cocco L, Cau R, Suri JS, Mannelli L, Manchia M, Puig J, Qi Y, Saba L. Correlation of Cognitive Reappraisal and the Microstructural Properties of the Forceps Minor: A Deductive Exploratory Diffusion Tensor Imaging Study. Brain Topogr 2024;37:63-74. [Crossref] [PubMed]
- Li Q, Becker B, Jiang X, Zhao Z, Zhang Q, Yao S, Kendrick KM. Decreased interhemispheric functional connectivity rather than corpus callosum volume as a potential biomarker for autism spectrum disorder. Cortex 2019;119:258-66. [Crossref] [PubMed]
- Minnigulova A, Davydova E, Pereverzeva D, Sorokin A, Tyushkevich S, Mamokhina U, Danilina K, Dragoy O, Arutiunian V. Corpus callosum organization and its implication to core and co-occurring symptoms of Autism Spectrum Disorder. Brain Struct Funct 2023;228:775-85. [Crossref] [PubMed]
- Panesar SS, Yeh FC, Deibert CP, Fernandes-Cabral D, Rowthu V, Celtikci P, Celtikci E, Hula WD, Pathak S, Fernández-Miranda JC. A diffusion spectrum imaging-based tractographic study into the anatomical subdivision and cortical connectivity of the ventral external capsule: uncinate and inferior fronto-occipital fascicles. Neuroradiology 2017;59:971-87. [Crossref] [PubMed]
- Friederici AD. Pathways to language: fiber tracts in the human brain. Trends Cogn Sci 2009;13:175-81. [Crossref] [PubMed]
- Sefcikova V, Sporrer JK, Juvekar P, Golby A, Samandouras G. Converting sounds to meaning with ventral semantic language networks: integration of interdisciplinary data on brain connectivity, direct electrical stimulation and clinical disconnection syndromes. Brain Struct Funct 2022;227:1545-64. [Crossref] [PubMed]
- Roux A, Lemaitre AL, Deverdun J, Ng S, Duffau H, Herbet G. Combining Electrostimulation With Fiber Tracking to Stratify the Inferior Fronto-Occipital Fasciculus. Front Neurosci 2021;15:683348. [Crossref] [PubMed]
- Saaybi S, AlArab N, Hannoun S, Saade M, Tutunji R, Zeeni C, Shbarou R, Hourani R, Boustany RM. Pre- and Post-therapy Assessment of Clinical Outcomes and White Matter Integrity in Autism Spectrum Disorder: Pilot Study. Front Neurol 2019;10:877. [Crossref] [PubMed]
- Bakhtiari R, Zürcher NR, Rogier O, Russo B, Hippolyte L, Granziera C, Araabi BN, Nili Ahmadabadi M, Hadjikhani N. Differences in white matter reflect atypical developmental trajectory in autism: A Tract-based Spatial Statistics study. Neuroimage Clin 2012;1:48-56. [Crossref] [PubMed]
- Martino J, Brogna C, Robles SG, Vergani F, Duffau H. Anatomic dissection of the inferior fronto-occipital fasciculus revisited in the lights of brain stimulation data. Cortex 2010;46:691-9. [Crossref] [PubMed]