Preoperatively predicting human epidermal growth factor receptor 2-low expression in breast cancer using neural network model based on multiparameter magnetic resonance imaging
Introduction
Breast cancer is prevalent among women in China and it has become one of the most common malignancies (1). In the individualized diagnosis and treatment of breast cancer, tumor biomarkers, such as progesterone receptor (PR), estrogen receptor (ER), tumor proliferation marker Ki-67, and human epidermal growth factor receptor 2 (HER2), not only have a suggestive effect on diagnosis and prognosis but also play an important role in the selection of treatment schemes and the evaluation of disease progression and tumor recurrence (2). HER2, in particular, is an important biomarker that affects the prognosis and decision-making of breast cancer. HER2 expression can be divided into HER2-positive and HER2-negative expression. When the immunohistochemistry (IHC) score is 3+ or the IHC score is 2+ with a positive in situ hybridization (ISH) result, HER2-positive expression is considered (3). HER2-positive breast cancer accounts for 15% of invasive breast cancer and, in most cases, has a poor prognosis; however, targeted therapy has considerably improved the prognoses of patients (4,5). When the IHC score is 0, 1+, or 2+ with a negative ISH result, HER2-negative expression is defined (3). HER2-negative expression is subdivided into HER2-low (IHC score 1+ or 2+ with a negative ISH result) and HER2-0 expression (IHC score 0) (6). HER2-low breast cancer accounts for approximately 55–60% of invasive breast cancer (7). Studies have shown that antibody-drug conjugates, such as trastuzumab-emtansine and trastuzumab-deruxtecan, can improve the prognosis of breast cancer patients with HER2-low expression (8-12). The prognosis, survival rate, and neoadjuvant therapy efficacy of HER2-low breast cancer patients are better than those of HER2-0 breast cancer patients (10-12). The HER2-low expression is mainly determined by biopsy, but due to the heterogeneity of the tumor, 7.3% of HER2-low tumors may be misclassified as HER2-positive (13). Predicting the HER2-low expression through imaging before surgery can facilitate the selection of clinical treatment strategies and improve patient prognosis (14).
Magnetic resonance imaging (MRI) is a noninvasive, multiparametric, and omnidirectional imaging technology that can provide rich imaging information and is now the primary diagnostic method for the study of breast cancer (15). Common diagnostic sequences include dynamic contrast-enhanced MRI (DCE-MRI) and diffusion-weighted imaging (DWI). DCE-MRI can reflect the dynamic distribution of contrast agents within tumors and display the morphological and hemodynamic characteristics of lesions (16) while DWI is a fast and inexpensive auxiliary diagnostic method that does not require the use of contrast agents. DWI can detect the Brownian motion of living water molecules and determine the motion restriction of water molecules by measuring the apparent diffusion coefficient (ADC). As cellular proliferation and density increase, the restriction of biological membrane structure on water molecule diffusion becomes more significant. This results in an increase in signal on the DWI and a decrease in ADC value (17,18). Many studies have shown that HER2-positive breast cancer usually manifests as irregular masses with or without non-mass enhancement, frequently with multiple foci, and centers; the MRI time-signal intensity curve (TIC) is of the washout pattern; and, the ADC value is higher than other molecular subtypes of breast cancer (19-23). Nevertheless, there is limited reporting on HER2-low expression breast cancer.
Bian et al. (20) conducted a study with a limited sample size, which revealed no significant statistical significance in MRI features between HER2-low and HER2-0 breast cancer subtypes. Additionally, they demonstrated that radiomics techniques utilizing multiparametric MRI could discriminate effectively between HER2-low and HER2-0 breast cancer subtypes. However, the procedures involved in image processing and modeling were found to be quite intricate. Herein, we constructed a neural network model with DCE-MRI features and ADC values based on a relatively larger sample size and explored its role in diagnosing HER2-low and HER2-0 breast cancer. Our findings may guide clinical treatment and prognosis assessment of HER2-low and HER2-0 breast cancer. We present this article in accordance with the TRIPOD reporting checklist (available at https://qims.amegroups.com/article/view/10.21037/qims-24-428/rc).
Methods
Study participants
This retrospective study was conducted across two centers: the Second Hospital of Shandong University (known as “Center 1”) and the Qilu Hospital of Shandong University (referred to as “Center 2”). We included 1,965 consecutive breast cancer patients who underwent MRI scans from January 2015 to December 2022 at “Center 1”. The inclusion criteria were: (I) breast cancer patients with HER2-low and HER2-0 confirmed by pathology; (II) patients who underwent DCE-MRI and DWI examination before surgery. The exclusion criteria were: (I) patients who received chemotherapy and radiotherapy; (II) patients with a history of biopsy or other surgeries before MRI examination; (III) patients with artifacts and poor image quality; (IV) patients with other molecular subtypes of breast cancer. Finally, 895 patients with 903 lesions were enrolled. According to a 7:3 ratio, the patients were divided into a training set of 626 cases (632 lesions, including 483 with HER2-low expression and 149 with HER2-0 expression) and an internal validation set of 269 cases (271 lesions, including 202 with HER2-low expression and 69 with HER2-0 expression). A cohort of 100 consecutive breast cancer patients (100 lesions) from Center 2 between June 2021 to December 2022 were enrolled as the external validation set. The study was conducted following the Declaration of Helsinki (as revised in 2013). The study was approved by the Ethics Committee of the Second Hospital of Shandong University (No. KYLL-2023LW040) and individual consent for this retrospective analysis was waived.
MRI scanning
In Center 1, the examinations were performed with a 3.0T MRI system (GE Discovery MR750; GE Healthcare, Chicago, IL, USA) and an 8-channel dedicated breast surface coil. The patient was in the prone position, and both breasts were scanned simultaneously. The examination was performed 7–14 days after the end of menstruation. The plain scan included T2 IDEAL (Iterative Dixon water-fat separation with echo asymmetry and least-squares estimation) and T1-weighted imaging—fast spin echo imaging sequences in the transverse plane. Following the plain scan, Vibrant-Flex technology was used to obtain a DCE-MRI scan in the transaxial plane. The scanning parameters were as follows: repetition time (TR): 3.9 ms, echo time (TE): 1.7 ms; flip angle: 5°; field of view (FOV): 360 mm × 360 mm; matrix: 348×348; slice thickness: 1.8 mm, number of excitation (NEX): 0.7. Following the plain scan, the contrast agent gadodiamide (0.2 mmol/kg body weight) was injected at a rate of 2 mL/s, followed by a 20 mL saline flush. A mask scan was carried out before the administration of the contrast agent, and dynamic enhancement images were acquired immediately after the injection of saline. A total of 7 sequences were acquired without intervals, each lasting for 60 s. For DWI, single-shot echo-planar imaging technology was used to conduct transverse plane scanning. The b values were 0 and 800 s/mm2, and the scanning parameters were as follows: TR: 3,000 ms, TE: 49.5 ms, slice thickness: 5 mm, slice gap: 1.0 mm, FOV: 360 mm × 360 mm, matrix: 128×96, NEX: 4.T.
In Center 2, breast MRI scans were also conducted with a 3.0T MRI system (GE Discovery MR750) and an 8-channel dedicated breast surface coil. The patients were positioned in a prone position. The breast MRI protocol included several sequences: an axial pre-contrast 2D fast spin echo T2-weighted fat-suppressed sequence with specific parameters (TR =9,000 ms, TE =120 ms, matrix =512×224, slice thickness =3–5 mm, FOV =35 mm × 35 cm, NEX =1, scan time =130 s); an axial pre-contrast diffusion-weighted echo-planar imaging sequence with parameters (TR =5,000 ms, TE =58 ms, matrix =150×150, slice thickness =3–5 mm, FOV =350 mm × 350 mm, NEX =2–4, scan time =230 s); and axial dynamic 3D spoiled gradient-echo T1-weighted fat-suppressed sequences with specific parameters (flip angle = 15°, TR =8 ms, TE =4 ms, matrix =512×256, slice thickness =1.40 mm, FOV =380 mm × 380 mm, NEX =1). Additionally, a sagittal 3D spoiled gradient-echo post-contrast T1-weighted sequence was performed. The DCE-MRI used axial imaging and comprised one pre-contrast and five post-contrast dynamic series. Contrast-enhanced axial images were captured at 1.5, 3, 4.5, and 6 min post-contrast injection, followed by a delayed sagittal image obtained 8 min after injection. A bolus of 0.2 mmol/kg of gadodiamide contrast agent was administered at a rate of 2 mL/s, accompanied by a 20 mL saline flush.
MRI image analysis
The scanned images were uploaded to the GE AW4.6 workstation and evaluated by two radiologists, with 25 and 7 years of experience respectively, using the Breast Imaging Reporting and Data System (BI-RADS) classification (24), in a blinded manner. Image preprocessing, which can improve image quality, diagnostic accuracy, and the overall performance of the recognition algorithms, was conducted using 3D Slicer software (version 5.0.3) and Python (version 3.11.4). The preprocessing steps involved N4 bias field correction to address intensity non-uniformity caused by differences in magnetic fields from various scanners, spatial registration through the Elastix registration module for automated alignment of a large image dataset for subsequent statistical analysis and comparison, resampling to achieve isotropic voxels of 1×1×1 mm3 using B-spline interpolation to standardize voxel dimensions across different sequences, and adaptive thresholding of pixel values to reduce the impact of extreme values and outliers.
For the dynamic enhanced images, the following features of the mass were evaluated: (I) size, which was calculated as the mean of three measurements on the maximum diameter of all lesions; (II) morphology (round or oval, irregular), edge (clear or unclear), and internal enhancement (uniform, non-uniform, and annular) were recorded; and (III) hemodynamic evaluation, during which the region of interest (ROI) was analyzed by the T1 perfusion software and the TIC of both early and delayed enhancement was obtained. During the ROI delineation, areas with liquefied necrosis and hemorrhage should be avoided. The ROI should be delineated in the most evident and prominent enhanced area of the lesion, and the size of the ROI should be smaller than the overall lesion size. The early enhancement rate of TIC was determined as (SIpost − SIpre) / SIpre × 100%. Here, SI stands for signal intensity, and SIpre and SIpost refer to the signal intensities before and after enhancement, respectively. The signal intensity at 2 min after enhancement or the highest peak signal intensity within 2 min of the lesion was defined as SIpost. The delayed enhancement of TIC referred to the enhancement type that arose either 2 min after the enhancement or when the curve was altered. TIC was categorized into three distinct types: progressive type (Type I, in which there was a consistent increase in signal intensity with time), platform type (Type II, in which signal intensity remained constant after initial enhancement), and washout type (Type III, in which signal intensity decreased after reaching peak enhancement). In addition, breast density and BI-RADS classification were also evaluated.
For ADC measurement, the ROI was delineated on the solid portion of the lesion (i.e., the low-signal area on the ADC map) while avoiding the liquefied necrotic areas by a radiologist with 25 years of experience in breast MRI (S.Z.). ROI size (450–550 pixel size) should be proportional to the lesion size to ensure consistency during repeated measurements. Multiple measurements were conducted, and for each lesion, the mean minimum ADC value was recorded. Another radiologist (P.C.) delineated a separate set of ROIs for 80 randomly selected patients to evaluate inter-observer reproducibility. The two radiologists were not aware of the patient’s actual diagnosis.
Histopathology analysis
All cases were confirmed by surgery or biopsy pathology, and molecular typing was performed using IHC. HER2-low and HER2-0 were evaluated based on IHC and ISH results (6). The criteria for evaluating the ER and PR were: positive percentage ≥10% was defined as positive, and <10% was negative. The discrepancy was resolved by discussion. The hormone receptor status was considered positive if either ER or PR, or both, were positive. Conversely, if both ER and PR were negative, the hormone receptor status was classified as negative.
Construction of neural network model
The univariate Logistic regression and the least absolute shrinkage and selection operator (LASSO) regression were used to screen the MRI features with statistically significant differences. These features (tumor morphology, early enhancement rate, and ADC value) were used as independent variables to establish a neural network model. Due to the use of three covariates for prediction, to account for potential nonlinear relationships, and to balance model complexity with prediction efficacy, the neural network model was constructed with two hidden layers. It is generally recommended to have the number of nodes in the hidden layers be less than twice the number of input variables. As a result, we selected hidden layers with 5 nodes in the first layer and 2 nodes in the second layer. The neural network model was constructed using the Neuralnet package in R, with the architecture specified as c(5,2) for the hidden layers. Default parameters were used for the remaining settings, and hyperparameter tuning was not conducted. The resilient backpropagation method without weight backtracking was used as the default construction method. For internal validation, we used Bootstrapping. The HER2-low expression probability obtained from the neural network model (a continuous variable between 0 and 1) was used for receiver operating characteristic (ROC) curve analysis, in which the discriminatory ability of variables for HER2-low expression was determined. The area under the ROC curve (AUC) was then calculated to quantify the predictive performance of the model. The decision curve analysis (DCA) was used to verify the clinical efficacy of the model. The inter-observer reproducibility for DWI/ADC measurement was assessed using the intra-class correlation coefficient (ICC). The ICC values indicated excellent agreement when ICC was >0.74, good agreement for ICC >0.6 to 0.74, fair agreement for ICC >0.4 to 0.6, and poor agreement for ICC <0.4.
Statistical analysis
R 4.1.3 software was used for statistical analysis. The Kolmogorov-Smirnov test determined the distribution of continuous variables (age, size, early enhancement, and ADC value). An independent samples t-test was used if there was a normal distribution, and the Wilcoxon rank-sum test was used otherwise. The Chi-squared test or Fisher’s exact test was used to analyze categorical data. Careful consideration was given to ensure adherence to the assumptions of the statistical tests employed. Specifically, for the Kolmogorov-Smirnov test, independent samples t-test, Wilcoxon rank-sum test, Chi-squared test, and Fisher’s exact test, we validated the assumptions of normality, homogeneity of variance, and independence as necessary. A P value less than 0.05 indicated statistical significance.
Results
Baseline data and pathological features of patients
The flowchart of patient enrollment is illustrated in Figure 1. The age range of the training set (626 cases/632 lesions) was 24 to 87 years old with a median age of 51 years old and a mean age of 52.065±11.042 years old. In the internal validation set (269 patients/271 lesions), the age range was 27 to 85 years old with a median age of 52 years old and a mean age of 52.413±11.438 years old. In the external validation set (100 patients/100 lesions), the age range was 29 to 80 years old with a median age of 53 years old and a mean age of 53.340±11.057 years old. There was no statistical difference in age between the patients in the training and validation sets (P=0.555).
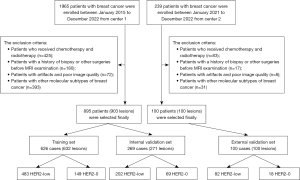
The demographic information of patients in the training set is presented in Table 1. The age, density, and BI-RADS classification between breast cancer patients with HER2-low expression and HER2-0 expression in the training set were not statistically significant (P=0.998, P=0.453, and P=0.687, respectively). Among the 632 lesions in the training set, 483 had HER2-low expression with lesion size ranging from 0.5 to 12.8 cm and an average size of 2.342±1.330 cm, while 149 had HER2-0 expression with lesion size ranging from 0.6 to 10.2 cm and an average size of 2.256±1.333 cm. Independent samples t-test showed that there was no statistically significant difference between patients with HER2-low and HER2-0 expression in terms of lesion size (P=0.492) (Table 1).
Table 1
Features | HER2-low (n=483) | HER2-0 (n=149) | Statistical value | P |
---|---|---|---|---|
Age (years) | 52.07±10.45 | 52.06±11.23 | −0.003 | 0.998 |
Density | 0.563 | 0.453 | ||
a/b | 108 (22.4) | 29 (19.5) | ||
c/d | 375 (77.6) | 120 (80.5) | ||
BI-RADS | 0.162 | 0.687 | ||
4 | 365 (75.6) | 115 (77.2) | ||
5 | 118 (24.4) | 34 (22.8) | ||
Tumor size (cm) | 2.342±1.330 | 2.256±1.333 | 0.688 | 0.492 |
Pathological type | 3.185 | 0.074 | ||
Invasive ductal carcinoma | 426 (88.2) | 123 (82.6) | ||
Others | 57 (11.8) | 26 (17.4) | ||
Hormone receptor expression | 14.371 | <0.001 | ||
Positive | 400 (82.8) | 102 (68.5) | ||
Negative | 83 (17.2) | 47 (31.5) |
Data are presented as mean ± standard deviation or n (%). a, the breasts are almost entirely fatty; b, there are scattered areas of fibroglandular density; c, the breasts are heterogeneously dense; d, the breasts are extremely dense. HER2, human epidermal growth factor receptor 2; BI-RADS, Breast Imaging Reporting and Data System.
Table 1 also listed the pathological features of breast cancer with HER2-low and HER2-0 expression in the training set. Among the lesions with HER2-low expression, 426 lesions were invasive ductal carcinoma, and 57 were other types of breast cancer. Among the lesions with HER2-0 expression, 123 lesions were invasive ductal carcinoma, and 26 were other types of breast cancer. The Chi-squared test indicated no statistically significant difference in pathological type between HER2-low expression and HER2-0 expression breast cancer (P=0.074). Specifically, 400 lesions of HER2-low expression breast cancer were HR-positive and 83 were HR-negative, while 102 cases of HER2-0 expression were HR-positive and 47 were HR-negative. The Chi-squared test showed a significant statistical difference in PR expression between HER2-low expression and HER2-0 expression (P<0.001).
MRI features of breast cancer with HER2-low and HER2-0 expression in the training set
The lesion morphology was related to the HER2 status (P<0.01), i.e., the lesion morphology of HER2-low expressing breast cancer was mostly irregular, while lesions expressing HER2-0 could be irregular, round, or oval, and the proportion of the two was similar. However, the edge, internal enhancement features, and TIC were not related to the HER2 status (P=0.124, P=0.719, and P=0.143) (Table 2, Figures 2,3). The early enhancement rates were 0.737±0.313 and 0.613±0.199 in HER2-low and HER2-0 breast cancer, respectively, with significant differences by the Wilcoxon rank-sum test (P<0.01) (Figures 2,3, Table 2). The ROC curve analysis revealed that the optimal cut-off value of the early enhancement rate for diagnosing HER2-low breast cancer was 0.555, with a sensitivity and specificity of 45.6% and 80.5%, respectively. The HER2-low breast cancer had a mean minimum ADC value of (0.987±0.203)×10−3 mm2/s and HER2-0 breast cancer had a mean minimum ADC value of (1.089±0.280)×10−3 mm2/s. The differences in ADC between HER2-low and HER2-0 were significant (P<0.01) (Figures 2,3, Table 2). Based on ROC curve analysis, the optimal cut-off value of ADC for diagnosing HER2-low breast cancer was 0.975×10−3 mm2/s, which corresponded to a sensitivity and specificity of 71.1% and 52.8%, respectively. Additionally, the inter-observer agreement between the two radiologists was almost perfect for the measured ADC values (ICC =0.964; 95% CI, 0.947–0.975). The confusion matrices showing the classification performance are presented in Table 3.
Table 2
Features | Training set | Internal validation set | External validation set | ||||||||
---|---|---|---|---|---|---|---|---|---|---|---|
HER2-low (n=483) |
HER2-0 (n=149) |
P | HER2-low (n=202) | HER2-0 (n=69) | P | HER2-low (n=82) | HER2-0 (n=18) |
P | |||
Morphology | <0.01 | 0.020 | 0.001 | ||||||||
Round or oval | 123 (25.5) | 69 (46.3) | 65 (32.2) | 33 (47.8) | 14 (17.1) | 8 (44.4) | |||||
Irregular | 360 (74.5) | 80 (53.7) | 137 (67.8) | 36 (52.2) | 68 (82.9) | 10 (55.6) | |||||
Edge | 0.124 | 0.573 | 0.329 | ||||||||
Clear | 14 (2.9) | 9 (6.0) | 3 (1.5) | 0 | 1 (1.2) | 1 (5.6) | |||||
Unclear | 469 (97.1) | 140 (94.0) | 199 (98.5) | 69 (100.0) | 81 (98.8) | 17 (94.4) | |||||
Internal enhancement | 0.719 | 0.207 | 1.0 | ||||||||
Uniform | 27 (5.6) | 9 (6.0) | 7 (3.5) | 0 | 3 (3.7) | 0 | |||||
Non-uniform | 420 (87.0) | 126 (84.6) | 183 (90.6) | 67 (97.1) | 73 (89.0) | 17 (94.4) | |||||
Annular | 36 (7.5) | 14 (9.4) | 12 (5.9) | 2 (2.9) | 6 (7.3) | 1 (5.6) | |||||
Early enhancement rates | 0.737±0.313 | 0.613±0.199 | <0.01 | 0.740±0.340 | 0.630±0.260 | 0.005 | 0.714±0.270 | 0.590±0.130 | 0.003 | ||
Time-signal intensity curve | 0.143 | 0.242 | 0.630 | ||||||||
I | 4 (0.8) | 3 (2.0) | 1 (0.5) | 1 (1.4) | 1 (1.2) | 0 | |||||
II | 98 (20.3) | 38 (25.5) | 53 (26.2) | 13 (18.8) | 21 (25.6) | 3 (16.7) | |||||
III | 381 (78.9) | 108 (72.5) | 148 (73.3) | 55 (79.7) | 60 (73.2) | 15 (83.3) | |||||
ADC value (10−3 mm2/s) | 0.987±0.203 | 1.089±0.280 | <0.01 | 0.987±0.203 | 0.980±0.180 | 0.006 | 0.968±0.232 | 1.120±0.296 | 0.001 |
Data are presented as mean ± standard deviation or n (%). HER2, human epidermal growth factor receptor 2; MRI, magnetic resonance imaging; ADC, apparent diffusion coefficient.
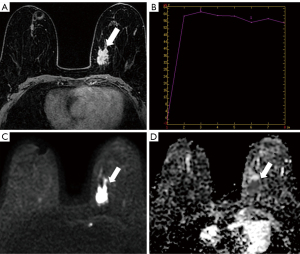
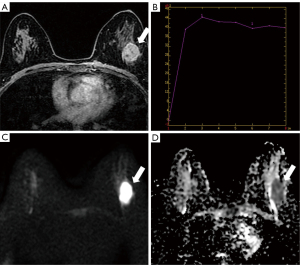
Table 3
Dataset | True positives | False positives | False negatives | True negatives |
---|---|---|---|---|
Training set | 100 | 102 | 49 | 381 |
Validation set | 62 | 102 | 7 | 100 |
External validation data | 18 | 17 | 6 | 59 |
True positives are the number of predicted positives that are actually positives. False positives are the number of predicted positives that are actually negatives. False negatives are the number of predicted negatives that are actually positives. True negatives are the number of predicted negatives that are actually negatives.
Features selection using the LASSO regression model
LASSO regression analysis was conducted to determine the key variables associated with low HER2 expression. Furthermore, 10-fold cross-validation was performed to mitigate the risk of overfitting. The distribution of LASSO regression coefficients and the cross-validation plots are presented in Figure 4A,4B. The optimal lambda value was determined as 0.0138. Finally, three variables (tumor morphology, early enhancement rate, and ADC value) with non-zero coefficients were identified.
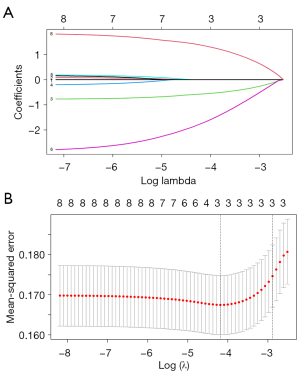
Neural network model construction and efficiency evaluation
The neural network model was constructed using tumor morphology, early enhancement rate, and ADC value (Figure 5A). The ROC curve showed that the AUC of the model for diagnosing HER2-low breast cancer was 0.757 (95% CI: 0.712–0.802) in the training set (Tables 4,5). The AUCs of ADC value, early enhancement rate, and morphology for diagnosing HER2-low breast cancer were 0.640 (95% CI: 0.589–0.691), 0.673 (95% CI: 0.622–0.724), and 0.600 (95% CI: 0.555–0.644), respectively (Tables 4,5, Figure 5B). Notably, the ADC value had the highest sensitivity for diagnosing HER2-low expression, and the early enhancement rate had the highest specificity. By using the ROC curve analysis, the optimal cut-off value for the neural network model in discriminating between HER2-low and HER2-0 expression breast cancer was 0.230. The diagnostic value of the MRI imaging features from 271 lesions in the internal validation set and 100 lesions in the external validation set was also analyzed with ROC analysis. The AUC of the model in the internal validation set and external validation set for diagnosing HER2-low expression breast cancer was 0.728 (95% CI: 0.658–0.798) and 0.791 (95% CI: 0.693–0.890), respectively (Figure 5C). The confusion matrices showing the classification performance are presented in Table 3.
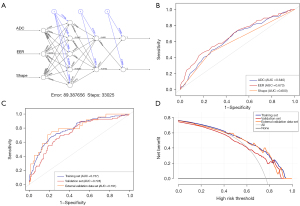
Table 4
Parameters | AUC (95% CI) | Sensitivity | Specificity | Positive predictive value |
Negative predictive value | Cut-off value |
---|---|---|---|---|---|---|
ADC | 0.640 (0.589–0.691) | 0.711 | 0.528 | 0.317 | 0.856 | 0.975 |
Early enhancement rate | 0.673 (0.622–0.724) | 0.456 | 0.805 | 0.420 | 0.828 | 0.555 |
Morphology | 0.600 (0.555–0.644) | 0.456 | 0.743 | 0.354 | 0.816 | 1.5 |
Combination | 0.757 (0.712–0.802) | 0.698 | 0.708 | 0.425 | 0.884 | 0.230 |
HER2, human epidermal growth factor receptor 2; DCE-MRI, dynamic contrast enhanced magnetic resonance imaging; ADC, apparent diffusion coefficient; AUC, area under the curve; CI, confidence interval.
Table 5
Parameters | AUC (95% CI) | P value |
---|---|---|
Combination* | 0.757 (0.712–0.802) | – |
ADC | 0.640 (0.589–0.691) | 5.194×10−6 |
Early enhancement rate | 0.673 (0.622–0.724) | 7.223×10−5 |
Morphology | 0.600 (0.555–0.644) | 8.460×10−8 |
*, the combination AUC is the reference. ADC, apparent diffusion coefficient; AUC, area under the curve; CI, confidence interval.
Clinical value evaluation of the neural network model
The DCA curve was plotted with the predicted probability on the x-axis and the net benefit rate on the y-axis. The results indicated that at a predicted probability of 0.764, the net benefit rate was greater than zero, indicating the clinical significance of the model. Furthermore, the smaller the predicted probability, the higher the net benefit rate, as shown in Figure 5D. Additionally, to facilitate direct validation and utilization by fellow researchers, we have made the trained model accessible as an RData file. The RData file, accompanied by the code for loading and applying the model, is accessible at https://github.com/YuYF97/neural_network_model.
Discussion
Alongside advancements in detection technology and the application of targeted drugs, breast cancer with HER2-low expression has gained increasing attention, leading to more clinical and pathological studies (3,6-14), but fewer studies in the field of imaging (20). A study by Bian et al. analyzed 213 lesions and found that MRI-based radiomics, but not conventional MRI features, could distinguish HER2-low and HER2-0 breast cancer (20). Here, we analyzed the DCE-MRI features and ADC value of HER2-low and HER2-0 breast cancer, identified the MRI features with differential significance, established a neural network model, and explored its value in evaluating HER2-low expression breast cancer.
Our study showed that the age, density, and BI-RADS classification between breast cancer patients with HER2-low expression and HER2-0 expression in the training set were not statistically significant. This is consistent with previous studies (10,11). Moreover, the pathological types and the lesion size of HER2-low and HER2-0 breast cancer were also not statistically different. This was similar to a clinical study, which showed that except that invasive lobular carcinoma was more commonly seen in HER2-0 breast cancer patients, other histopathological features such as tumor size had no significant difference between HER2-low and HER2-0 breast cancer (25). In this study, the pathological types of patients were mostly invasive ductal carcinoma. There was less invasive lobular carcinoma, mucinous carcinoma, etc., and thus they were classified into other types. In the future, we will expand the sample size for further analysis.
All lesions examined in this study were masses, and their morphology was correlated with HER2 status. This may be because a higher proportion of HR+ lesions (about 82.8%) were observed in lesions with HER2-low expression and HR+ breast cancer typically exhibits an irregular morphology (26,27). The ROC curve analysis revealed that the diagnostic efficacy of tumor morphology in identifying HER2-low breast cancer was low, with an AUC of 0.600 (95% CI: 0.555–0.644). The poor diagnostic efficacy may be attributable to the large difference in sample size between HER2-low and HER2-0 expression. Future research should involve expanding the sample size of HER2-0 expression.
In DCE-MRI, the enhanced permeability of tumor blood vessels facilitates rapid diffusion of contrast agents across the capillary wall, resulting in early peak enhancement and a delayed washout curve (28,29). In this study, while the TIC curve of breast cancer with HER2-low or HER2-0 expression did not show statistical significance, the early enhancement rate demonstrated statistical significance. The ROC curve analysis revealed that the optimal cut-off value of the early enhancement rate for diagnosing HER2-low breast cancer was 0.555, with a sensitivity and specificity of 45.6% and 80.5%, respectively. The washout curve is a typical characteristic of malignant tumors (30), which poses difficulty in distinguishing between breast cancer of HER2-low or HER2-0 expression. Our results showed a difference in the early enhancement rate of HER2-low or HER2-0 expression breast cancer, which requires further verification.
ADC values can quantitatively evaluate changes in tumor cell density and/or perfusion before changes in lesion morphology, providing a means for quantitative evaluation and early prediction of tumor characteristics on MRI (18). This study found a statistically significant difference in the mean minimum ADC of breast cancer between those with HER2-low expression and those with HER2-0 expression. Based on ROC curve analysis, the optimal cut-off value of ADC for diagnosing HER2-low breast cancer was 0.975×10−3 mm2/s, with a sensitivity and specificity of 71.1% and 52.8%, respectively. Breast cancer with HER2-low expression had an HR-positive rate of about 82.8%. The decreased ADC value may be attributed to HR-positive expression inhibiting angiogenesis, resulting in a reduction in perfusion and limited molecular diffusion (29). Conversely, HER2-0 expression is associated with an increased ADC value due to elevated neovascularization, higher vascular permeability, and greater continuity of extracellular fluid (31). In this study, the ADC value was measured at least three times, and the minimum value was selected to represent the most malignant region of the tumor. This method is straightforward and practical, as demonstrated in the study by Tuan et al. (32) and our previous study (33). However, DWI images and ADC values of the breast are easily affected by patient respiratory motion, cardiac pulsation, and machine sequence parameters. The equipment at the two centers in this study was of the same model, both with a field strength of 3.0T, and the selected b values were also the same. Therefore, we found that the inter-observer agreement between the two radiologists was almost perfect for the measured ADC values, indicating good and stable inter-observer consistency and repeatability. Using ICC to evaluate the consistency and repeatability of the measured ADC values may enhance the stability of the neural network prediction model and the reliability of the study, which is beneficial for future clinical classification, prognosis, and personalized diagnosis.
In statistical analysis, it has been found that neural network models excel in capturing complex nonlinear relationships between covariates and outcomes, while methods like logistic regression and random forest fail to achieve satisfactory predictive performance (34). Moreover, based on external validation data, the neural network model demonstrated good prediction performance while avoiding overfitting issues, with good extrapolation effects. Therefore, we developed a neural network model in this study. The tumor morphology, early enhancement rate, and ADC value in the training set were used as independent variables. Considering the number of variables and the HER2 classification categories, we selected two hidden layer units and conducted the ROC curve and DCA analyses. The results showed that the model exhibited a good diagnostic efficiency for HER2-low expression breast cancer with a superior AUC of 0.757 (95% CI: 0.712–0.802), which outweighed the AUC values of the ADC value, early enhancement rate, and tumor morphology. The variable values of MRI imaging features were applied to 271 lesions in the internal validation set and 100 lesions in the external validation set, which demonstrated a satisfactory fit to the training set and produced an AUC of 0.728 (95% CI: 0.658–0.798) and 0.791 (95% CI: 0.693–0.890) for diagnosing HER2-low expression breast cancer.
DCA is a method for evaluating the value of predictive models in actual clinical decision-making. In our study, the DCA curve analysis demonstrated that the model had clinical significance with a net benefit ratio greater than zero when the predicted probability was 0.764, and the magnitude of the net benefit ratio increased as the predicted probability decreased. Therefore, the neural network model may have good diagnostic efficiency and clinical application value for HER2-low expression breast cancer. This neural network model can be integrated into the existing diagnostic workflows by first identifying the HER2-low breast cancer for whom the model’s predictions are most beneficial. Moreover, healthcare professionals would need to be trained on interpreting and utilizing the model’s predictions effectively. This may involve educational sessions or workshops to ensure proper integration into clinical practice. In addition, this neural network model may provide risk scores or probabilities that categorize patients into low, moderate, or high-risk groups. Specific thresholds can be defined to guide clinical decisions such as treatment recommendations or further diagnostic testing for each risk category.
There have been comparatively few imaging studies on HER2-low breast cancer. A study involving 213 cases of HER2-negative breast cancer found no significant differences in conventional MRI features between HER2-low and HER2-0 breast cancer. However, radiomics techniques based on multiparametric MRI can differentiate between HER2-low and HER2-0 breast cancer (20). In our study, we found that DCE-MRI features and ADC values could serve as independent predictive factors for HER2-low or HER2-0 expression. By combining these variables, we developed a predictive model with good consistency and high accuracy. During the external validation, our model demonstrated good clinical efficacy. In comparison to other radiomics-based predictive models (20), our model was cost- and time-effective, which only required routine enhanced MR image features for feature extraction and prediction, eliminating the need for complex tumor three-dimensional target delineation at various stages of enhancement scans, which would otherwise impose a heavy computational burden. On the other hand, some researchers have utilized biopsy specimens and analyzed gene expression or radiogenomics to predict HER2-low or HER2-0 expression (13). While tissue biopsy has shown good predictive efficacy, it may be unnecessary for patients with typical imaging findings. Furthermore, invasive surgery may pose potential risks, such as tumor seeding and fatal bleeding, outweighing the benefits. Compared with biopsy, our model was non-invasive and had easy accessibility.
This study has several limitations. First, HER2-positive breast cancer cases were not included in this study. Second, all the lesions analyzed were masses, and the exclusion of non-mass lesions might limit the applicability of the findings. Future directions should include these lesion types to broaden the model’s utility. Third, the neural network model in our study used human-labeled ROIs as inputs. This process was time-consuming and error-prone. In future studies, we will develop a model that works with the entire MRI imaging without the need for human-labeled ROIs. Fourth, some features, like T2-weighted imaging signal features that are susceptible to variations between observers, were not evaluated. Fifth, there was a class imbalance. In future work, we plan to explore more methods, such as ensemble learning, cost-sensitive learning, etc., to further enhance the model’s robustness and fairness. Lastly, there were few statistically significant features extracted from DCE-MRI among HER2-low and HER2-0 breast cancer cases, and few HR-negative cases were identified. Therefore, there was no further analysis conducted to compare the differences in DCE-MRI and ADC values between HR+ and HR− in the two groups of breast cancer. Hence, future studies are warranted.
Conclusions
In conclusion, our study focused on the differentiation of HER2-low and HER2-0 expression breast cancer using MRI imaging features and ADC values. Compared to HER2-0 expression, HER2-low breast cancer has higher early enhancement rates and lower ADC values. The neural network model we constructed demonstrated promising diagnostic efficiency and clinical significance in evaluating HER2-low expression breast cancer, which may facilitate clinical decision-making and prognostic evaluation. Our findings highlighted the importance of incorporating DCE-MRI features and ADC values as independent predictive factors, which together provided a practical and non-invasive approach to predicting HER2-low expression. Future research should address study limitations and expand the scope to enhance breast cancer management.
Acknowledgments
Funding: This study was supported by
Footnote
Reporting Checklist: The authors have completed the TRIPOD reporting checklist. Available at https://qims.amegroups.com/article/view/10.21037/qims-24-428/rc
Conflicts of Interest: All authors have completed the ICMJE uniform disclosure form (available at https://qims.amegroups.com/article/view/10.21037/qims-24-428/coif). The authors have no conflicts of interest to declare.
Ethical Statement: The authors are accountable for all aspects of the work in ensuring that questions related to the accuracy or integrity of any part of the work are appropriately investigated and resolved. The study was conducted following the Declaration of Helsinki (as revised in 2013). The study was approved by the Ethics Committee of the Second Hospital of Shandong University (No. KYLL-2023LW040) and individual consent for this retrospective analysis was waived.
Open Access Statement: This is an Open Access article distributed in accordance with the Creative Commons Attribution-NonCommercial-NoDerivs 4.0 International License (CC BY-NC-ND 4.0), which permits the non-commercial replication and distribution of the article with the strict proviso that no changes or edits are made and the original work is properly cited (including links to both the formal publication through the relevant DOI and the license). See: https://creativecommons.org/licenses/by-nc-nd/4.0/.
References
- Cho N. Imaging features of breast cancer molecular subtypes: state of the art. J Pathol Transl Med 2021;55:16-25. [Crossref] [PubMed]
- Van Poznak C, Somerfield MR, Bast RC, Cristofanilli M, Goetz MP, Gonzalez-Angulo AM, Hicks DG, Hill EG, Liu MC, Lucas W, Mayer IA, Mennel RG, Symmans WF, Hayes DF, Harris LN. Use of Biomarkers to Guide Decisions on Systemic Therapy for Women With Metastatic Breast Cancer: American Society of Clinical Oncology Clinical Practice Guideline. J Clin Oncol 2015;33:2695-704. [Crossref] [PubMed]
- Burge CN, Chang HR, Apple SK. Do the histologic features and results of breast cancer biomarker studies differ between core biopsy and surgical excision specimens? Breast 2006;15:167-72. [Crossref] [PubMed]
- Slamon DJ, Clark GM, Wong SG, Levin WJ, Ullrich A, McGuire WL. Human breast cancer: correlation of relapse and survival with amplification of the HER-2/neu oncogene. Science 1987;235:177-82. [Crossref] [PubMed]
- Loibl S, Jassem J, Sonnenblick A, Parlier D, Winer E, Bergh J, Gelber RD, Restuccia E, Im YH, Huang C, Dalenc F, Calvo I, Procter M, Caballero C, Clark E. Gomez Moreno HL, Bliss J, Viale G, Bines J, Piccart M. VP6-2022: Adjuvant pertuzumab and trastuzumab in patients with early HER-2 positive breast cancer in APHINITY: 8.4 years' follow-up. Ann Oncol 2022;33:986-7.
- Modi S, Park H, Murthy RK, Iwata H, Tamura K, Tsurutani J, Moreno-Aspitia A, Doi T, Sagara Y, Redfern C, Krop IE, Lee C, Fujisaki Y, Sugihara M, Zhang L, Shahidi J, Takahashi S. Antitumor Activity and Safety of Trastuzumab Deruxtecan in Patients With HER2-Low-Expressing Advanced Breast Cancer: Results From a Phase Ib Study. J Clin Oncol 2020;38:1887-96. [Crossref] [PubMed]
- Marchiò C, Annaratone L, Marques A, Casorzo L, Berrino E, Sapino A. Evolving concepts in HER2 evaluation in breast cancer: Heterogeneity, HER2-low carcinomas and beyond. Semin Cancer Biol 2021;72:123-35. [Crossref] [PubMed]
- Modi S, Jacot W, Yamashita T, Sohn J, Vidal M, Tokunaga E, et al. Trastuzumab Deruxtecan in Previously Treated HER2-Low Advanced Breast Cancer. N Engl J Med 2022;387:9-20. [Crossref] [PubMed]
- Xin L, Wu Q, Zhan C, Qin H, Xiang H, Xu L, Ye J, Duan X, Liu Y. Multicenter study of the clinicopathological features and recurrence risk prediction model of early-stage breast cancer with low-positive human epidermal growth factor receptor 2 expression in China (Chinese Society of Breast Surgery 021). Chin Med J (Engl) 2022;135:697-706. [Crossref] [PubMed]
- Zhang G, Ren C, Li C, Wang Y, Chen B, Wen L, Jia M, Li K, Mok H, Cao L, Chen X, Lin J, Wei G, Li Y, Zhang Y, Balch CM, Liao N. Distinct clinical and somatic mutational features of breast tumors with high-, low-, or non-expressing human epidermal growth factor receptor 2 status. BMC Med 2022;20:142. [Crossref] [PubMed]
- Yang C, Zhang X, Chen Y, Li P, Zhang J, Xu A, Huang N, Liang M, Chen Y, Wang K. Survival differences between HER2-0 and HER2-low-expressing breast cancer - A meta-analysis of early breast cancer patients. Crit Rev Oncol Hematol 2023;185:103962. [Crossref] [PubMed]
- Denkert C, Seither F, Schneeweiss A, Link T, Blohmer JU, Just M, et al. Clinical and molecular characteristics of HER2-low-positive breast cancer: pooled analysis of individual patient data from four prospective, neoadjuvant clinical trials. Lancet Oncol 2021;22:1151-61. [Crossref] [PubMed]
- Chen R, Qi Y, Huang Y, Liu W, Yang R, Zhao X, Wu Y, Li Q, Wang Z, Sun X, Wei B, Chen J. Diagnostic value of core needle biopsy for determining HER2 status in breast cancer, especially in the HER2-low population. Breast Cancer Res Treat 2023;197:189-200. [Crossref] [PubMed]
- Molinelli C, Jacobs F, Marchiò C, Pitto F, Cosso M, Spinaci S, de Azambuja E, Schettini F, Agostinetto E, Lambertini M. HER2-Low Breast Cancer: Where Are We? Breast Care (Basel) 2022;17:533-45. [Crossref] [PubMed]
- Li Z, Ai T, Hu Y, Yan X, Nickel MD, Xu X, Xia L. Application of whole-lesion histogram analysis of pharmacokinetic parameters in dynamic contrast-enhanced MRI of breast lesions with the CAIPIRINHA-Dixon-TWIST-VIBE technique. J Magn Reson Imaging 2018;47:91-6. [Crossref] [PubMed]
- Li W, Wang S, Xie W, Feng C. A quantitative heterogeneity analysis approach to molecular subtype recognition of breast cancer in dynamic contrast-enhanced magnetic imaging images from radiomics data. Quant Imaging Med Surg 2023;13:4429-46. [Crossref] [PubMed]
- Partridge SC, McDonald ES. Diffusion weighted magnetic resonance imaging of the breast: protocol optimization, interpretation, and clinical applications. Magn Reson Imaging Clin N Am 2013;21:601-24. [Crossref] [PubMed]
- Yang SH, Lin J, Lu F, Han ZH, Fu CX, Lv P, Liu H, Gao DM. Evaluation of antiangiogenic and antiproliferative effects of sorafenib by sequential histology and intravoxel incoherent motion diffusion-weighted imaging in an orthotopic hepatocellular carcinoma xenograft model. J Magn Reson Imaging 2017;45:270-80. [Crossref] [PubMed]
- Portnow LH, Kochkodan-Self JM, Maduram A, Barrios M, Onken AM, Hong X, Mittendorf EA, Giess CS, Chikarmane SA. Multimodality Imaging Review of HER2-positive Breast Cancer and Response to Neoadjuvant Chemotherapy. Radiographics 2023;43:e220103. [Crossref] [PubMed]
- Bian X, Du S, Yue Z, Gao S, Zhao R, Huang G, Guo L, Peng C, Zhang L. Potential Antihuman Epidermal Growth Factor Receptor 2 Target Therapy Beneficiaries: The Role of MRI-Based Radiomics in Distinguishing Human Epidermal Growth Factor Receptor 2-Low Status of Breast Cancer. J Magn Reson Imaging 2023;58:1603-14. [Crossref] [PubMed]
- Chen H, Li W, Wan C, Zhang J. Correlation of dynamic contrast-enhanced MRI and diffusion-weighted MR imaging with prognostic factors and subtypes of breast cancers. Front Oncol 2022;12:942943. [Crossref] [PubMed]
- Nave O, Elbaz M, Bunimovich-Mendrazitsky S. Analysis of a breast cancer mathematical model by a new method to find an optimal protocol for HER2-positive cancer. Biosystems 2020;197:104191. [Crossref] [PubMed]
- Qian F, Mao Y, Dong J, Xie F, Fang X, Zhang Q, Xia P, Han X, Lu N. Development of a multiparametric model for predicting the response to neoadjuvant chemotherapy in breast cancer. Transl Cancer Res 2024;13:558-68. [Crossref] [PubMed]
- Pinker K, Bickel H, Helbich TH, Gruber S, Dubsky P, Pluschnig U, Rudas M, Bago-Horvath Z, Weber M, Trattnig S, Bogner W. Combined contrast-enhanced magnetic resonance and diffusion-weighted imaging reading adapted to the "Breast Imaging Reporting and Data System" for multiparametric 3-T imaging of breast lesions. Eur Radiol 2013;23:1791-802. [Crossref] [PubMed]
- Mutai R, Barkan T, Moore A, Sarfaty M, Shochat T, Yerushalmi R, Stemmer SM, Goldvaser H. Prognostic impact of HER2-low expression in hormone receptor positive early breast cancer. Breast 2021;60:62-9. [Crossref] [PubMed]
- Szep M, Pintican R, Boca B, Perja A, Duma M, Feier D, Fetica B, Eniu D, Dudea SM, Chiorean A. Multiparametric MRI Features of Breast Cancer Molecular Subtypes. Medicina (Kaunas) 2022.
- Seyfettin A, Dede I, Hakverdi S, Düzel Asıg B, Temiz M, Karazincir S. MR imaging properties of breast cancer molecular subtypes. Eur Rev Med Pharmacol Sci 2022;26:3840-8. [Crossref] [PubMed]
- Szabó BK, Aspelin P, Kristoffersen Wiberg M, Tot T, Boné B. Invasive breast cancer: correlation of dynamic MR features with prognostic factors. Eur Radiol 2003;13:2425-35. [Crossref] [PubMed]
- Ab Mumin N, Ramli Hamid MT, Wong JHD, Rahmat K, Ng KH. Magnetic Resonance Imaging Phenotypes of Breast Cancer Molecular Subtypes: A Systematic Review. Acad Radiol 2022;29:S89-S106. [Crossref] [PubMed]
- Kazama T, Takahara T, Hashimoto J. Breast Cancer Subtypes and Quantitative Magnetic Resonance Imaging: A Systemic Review. Life (Basel) 2022.
- Park SH, Choi HY, Hahn SY. Correlations between apparent diffusion coefficient values of invasive ductal carcinoma and pathologic factors on diffusion-weighted MRI at 3.0 Tesla. J Magn Reson Imaging 2015;41:175-82. [Crossref] [PubMed]
- Tuan Linh L, Minh Duc N, Minh Duc N, Tra My TT, Viet Bang L. Clin Ter 2021;172:218-24. [Crossref] [PubMed]
- Chen P, Zhao S, Guo W, Shao G. Dynamic contrast-enhanced magnetic resonance imaging features and apparent diffusion coefficient value of HER2-positive/HR-negative breast carcinoma. Quant Imaging Med Surg 2023;13:4816-25. [Crossref] [PubMed]
- Chen TJ, Hsu YH, Chen CH. Comparison of Neural Network and Logistic Regression Analysis to Predict the Probability of Urinary Tract Infection Caused by Cystoscopy. Biomed Res Int 2022;2022:5775447. [Crossref] [PubMed]