Exploring the optimal threshold of 3D consolidation tumor ratio value segmentation based on artificial intelligence for predicting the invasive degree of T1 lung adenocarcinoma
Introduction
With the widespread use of low-dose thin-slice chest computed tomography (CT) in lung health examinations, the detection rate of pulmonary nodules has significantly increased. These nodules are often early-stage lung adenocarcinomas, for which radical surgical resection is the preferred treatment method. However, there is some controversy in the clinical choice between wedge resection, segmentectomy, and lobectomy. Ginsberg’s randomized controlled study in 1995 showed that sublobar resection compared to lobectomy increased tumor-related mortality by 50% and the local recurrence rate by three times, thereby establishing the dominant position of lobectomy in radical lung cancer surgery (1). In recent years, with the increase of ground glass-like lung cancer and the earlier staging of lung cancer, sublobar resection has shown obvious advantages in these patients. The Japan Clinical Oncology Group (JCOG) series of studies, including JCOG0201 (2), JCOG0804/WJOG4507L (3), JCOG0802/WJOG4607L (4), and JCOG1211 (5), have provided important insights into lung cancer surgery treatment. Among them, the consolidation tumor ratio (CTR), as one of the key indicators for evaluating the surgical approach to lung cancer, defines non-invasive adenocarcinoma (non-IAC) on imaging as ≤20 mm in diameter and CTR ≤0.25. For peripheral early-stage lung adenocarcinomas primarily characterized by ground glass opacity (GGO), standardized limited resection or sublobar resection can achieve total survival rates comparable to or even better than standard lobectomy without increasing the risk of local recurrence. In addition, the study also proposed a new approach to determine the surgical method based on imaging performance, avoiding the dependence on preoperative small sample biopsies or intraoperative frozen pathology results for surgical strategy.
In clinical practice, due to the varying levels of intraoperative pathological diagnosis across different medical centers, the insufficient accuracy of intraoperative frozen sections, and the increased use of anesthetic drugs during the waiting period for pathology results (6), predicting the invasiveness of stage T1 lung adenocarcinoma based on preoperative imaging and planning preoperatively has significant clinical value (7,8). Sometimes, this method may even be more accurate than intraoperative frozen section diagnosis (9). In addition, preoperative imaging-assisted diagnosis can improve the accuracy of intraoperative frozen sections, which helps in planning the surgical resection and provides a basis for clinical decision-making when the intraoperative frozen results do not match preoperative considerations (10). This study aimed to predict the invasiveness of T1 stage lung adenocarcinoma by artificial intelligence technology based on the threshold segmentation method to determine the three-dimensional (3D) CTR. However, there is currently no clinical consensus on the setting of CT thresholds. This paper measures the 3D CTR at seven different CT thresholds (−550, −450, −350, −250, −150, −50, and 0 HU) to select the best predictive threshold and determine the optimal cut-off point at that threshold. Furthermore, this paper also conducts subgroup analysis on lung nodules of different sizes, including group of T1a, T1b, and T1c. We present this article in accordance with the STARD reporting checklist (available at https://qims.amegroups.com/article/view/10.21037/qims-24-1328/rc).
Methods
Collection of clinical data and determination of thresholds
In a comprehensive analysis of patients with pathologically confirmed stage T1 lung adenocarcinoma, a cohort was systematically assembled. The study period spanned from November 2017 to November 2023 at Zhengzhou People’s Hospital and from May 2012 to September 2018 at Huadong Hospital of Fudan University. Consecutive enrollment was conducted, resulting in the inclusion of 820 patients (936 nodules) and 236 patients (243 nodules) from the two centers, all of whom satisfied the predefined inclusion and exclusion criteria. The data included patient gender, age, and thin-slice chest CT images [digital imaging and communications in medicine (DICOM) format]. The collected thin-slice DICOM format images were compressed and imported into the Shukun Artificial Intelligence workstation for comprehensive thoracic diagnostic analysis. The software automatically segmented, identified, and quantified the 3D CTR (%) at different CT thresholds. A total of seven CT thresholds were set: −550, −450, −350, −250, −150, −50, and 0 HU. The study was conducted in accordance with the Declaration of Helsinki (as revised in 2013). The study was approved by the Institutional Review Boards of the Fifth Clinical Medical College of Henan University of Chinese Medicine (Zhengzhou People’s Hospital) (No. 2024011139) and Huadong Hospital of Fudan University (No. 2019K134), and the requirement for individual consent for this retrospective analysis was waived due to the retrospective nature of the study.
Inclusion and exclusion criteria
Inclusion criteria
The maximum diameter of the pulmonary opacity is ≤3 cm; no prior radiotherapy, chemotherapy, or other neoadjuvant therapies; confirmed as stage T1 lung adenocarcinoma by postoperative routine pathological diagnosis, including atypical adenomatous hyperplasia (AAH), adenocarcinoma in situ (AIS), minimally invasive adenocarcinoma (MIA) and IAC; complete thin-slice chest CT data within 1 month prior to surgery, with a slice thickness of ≤1.5 mm; complete clinical data, and all imaging data analyzed by the artificial Shukun Technology intelligence workstation for whole chest diagnostic analysis, ensuring that resected lung nodules can be accurately identified and quantified for detailed radiomic features.
Exclusion criteria
The maximum diameter of the pulmonary opacity is >3 cm; received radiotherapy, chemotherapy, or other neoadjuvant therapies prior to surgery; confirmed as other types of tumors or benign lesions by postoperative routine pathological diagnosis; incomplete thin-slice chest CT data within 1 month prior to surgery or with a slice thickness >1.5 mm; incomplete clinical data; imaging data that cannot be accurately identified or detailed radiomic features not quantified by the artificial Shukun Technology intelligence workstation for whole chest diagnostic analysis.
Grouping strategy
Patients were categorized into two groups based on postoperative pathological results: the Invasive Group, which includes patients diagnosed with IAC; and the Non-Invasive Group, comprising patients diagnosed with AAH, AIS, and MIA. Furthermore, a subgroup analysis was conducted for stage T1 lung cancer patients based on the size of the pulmonary nodule, dividing them into three subgroups: T1a (nodule diameter ≤10 mm), T1b (nodule diameter between >10 and 20 mm), and T1c (nodule diameter between >20 and 30 mm). The detailed flowchart of the study is presented in Figure 1, while the variations in the solid components of the nodule on chest CT under different threshold settings are illustrated in Figure 2.
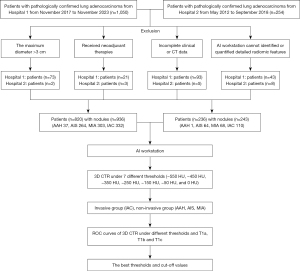
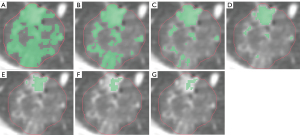
Statistical analysis
Statistical analysis in this study was performed using SPSS 23.0 software (IBM, Armonk, New York, USA). For continuous variables, data are presented as mean ± standard deviation, and differences between groups were compared using independent samples t-tests. Categorical variables are expressed as counts and percentages, with group comparisons made using chi-square tests (χ2 tests). A P value of less than 0.05 was considered statistically significant based on a two-tailed test. The 3D CTR values at different CT thresholds were used to plot the receiver operating characteristic (ROC) curves, and the predictive performance at different thresholds was compared, specifically the area under the curve (AUC). This method was employed to determine the optimal CT threshold and identify the cut-off point with the highest predictive efficacy at that threshold. Furthermore, data were re-analyzed after stratifying the nodules by size.
Results
General characteristics analysis
A total of 1,179 pulmonary nodules from two centers were included in this study, with the non-invasive lesion group comprising 737 nodules (AAH 38, AIS 328, MIA 371), and the invasive group consisting of 442 nodules (IAC 442). Regardless of the CT threshold settings, the 3D CTR showed significant statistical differences between the two groups, indicating that the 3D CTR is a stable and effective predictive factor for the invasiveness of stage T1 lung adenocarcinoma. Detailed data characteristics are presented in Table 1, which illustrates the judgment of the invasiveness of stage T1 lung adenocarcinoma based on the 3D CTR at different thresholds.
Table 1
Variables | Total (n=1,179) | Non-invasive group (n=737) | Invasive group (n=442) | t/χ2 | P |
---|---|---|---|---|---|
Age, years | 56.67±11.51 | 53.87±11.83 | 61.36±9.25 | −12.10 | <0.001 |
Maximum 3D diameter, mm | 13.22±6.28 | 12.55±5.95 | 14.35±6.63 | −4.70 | <0.001 |
3D CTR, % | |||||
−550 HU | 53.99±34.39 | 39.68±30.40 | 77.85±26.54 | −22.62 | <0.001 |
−450 HU | 39.73±35.70 | 23.02±25.89 | 67.60±32.26 | −24.68 | <0.001 |
−350 HU | 29.73±34.27 | 12.51±19.34 | 58.45±34.51 | −25.67 | <0.001 |
−250 HU | 22.43±31.26 | 6.90±13.93 | 48.32±34.81 | −23.90 | <0.001 |
−150 HU | 16.82±27.72 | 3.40±9.39 | 39.21±33.20 | −22.15 | <0.001 |
−50 HU | 11.60±22.64 | 1.51±6.00 | 28.44±29.22 | −19.14 | <0.001 |
0 HU | 8.63±18.67 | 0.91±4.35 | 21.52±25.17 | −17.06 | <0.001 |
Sex | 7.22 | 0.007 | |||
Male | 392 (33.25) | 224 (30.39) | 168 (38.01) | ||
Female | 787 (66.75) | 513 (69.61) | 274 (61.99) | ||
Lobe | 2.95 | 0.566 | |||
RUL | 400 (33.93) | 246 (33.38) | 154 (34.84) | ||
RML | 83 (7.04) | 54 (7.33) | 29 (6.56) | ||
RLL | 227 (19.25) | 138 (18.72) | 89 (20.14) | ||
LUL | 290 (24.60) | 192 (26.05) | 98 (22.17) | ||
LLL | 179 (15.18) | 107 (14.52) | 72 (16.29) |
Data are presented as mean ± standard deviation or number (percentage). CT, computed tomography; 3D CTR, three-dimensional consolidation tumor ratio; RUL, right upper lobe; RML, right middle lobe; RLL, right lower lobe; LUL, left upper lobe; LLL, left lower lobe; HU, Housefield unit.
ROC curve analysis of 3D CTR predicting the infiltration of stage T1 lung adenocarcinoma based on artificial intelligence threshold segmentation at different thresholds
This study utilized the artificial intelligence threshold segmentation method to calculate the 3D CTR at various CT threshold settings to predict the infiltration of stage T1 lung adenocarcinoma. The corresponding ROC curves are presented in Figure 3. Specifically, as the CT threshold was adjusted from −550 to −150 HU, an enhancement in predictive performance was observed with the increase in threshold. However, when the CT threshold was further increased from −150 to 0 HU, the predictive performance decreased. This indicates that the optimal predictive performance for lung adenocarcinoma invasion is achieved at a CT threshold of −150 HU. The AUC and the 95% confidence interval (CI) were 0.901 (0.883–0.919). The corresponding cut-off value was 2.75%, with a sensitivity of 86.878%, specificity of 77.883%, accuracy of 81.255%, and Youden’s index of 0.64761. These results demonstrate that the 3D CTR shows a high predictive accuracy at the threshold of −150 HU. When the threshold is set to −150 HU, the CTR distribution between the two groups is shown in Figure 4.
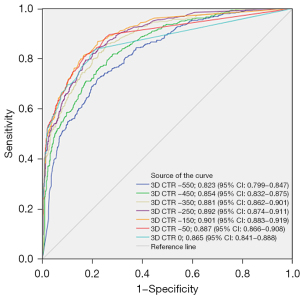
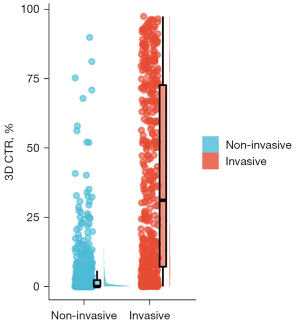
Subgroup analysis by lung nodule size
This study categorized patients with stage T1 lung cancer into three subgroups based on the size of the pulmonary nodules: the T1a group (507 nodules, with 353 non-invasive and 154 invasive), the T1b group (495 nodules, with 292 non-invasive and 203 invasive), and the T1c group (177 nodules, with 92 non-invasive and 85 invasive). For each subgroup, we calculated the 3D CTR at different CT threshold settings and plotted the corresponding ROC curves.
In the T1a subgroup, the predictive performance improved as the CT threshold increased from −550 to 0 HU, with the optimal threshold at −150 HU, yielding an AUC of 0.887, a cut-off value of 2.75%, a sensitivity of 77.620%, a specificity of 85.714%, an accuracy of 80.079%, and a Youden’s index of 0.63335 (Figure 5).
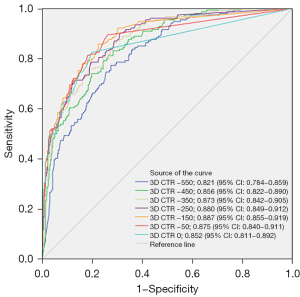
For the T1b subgroup, a similar trend of increasing predictive performance with threshold increase was observed, with the optimal threshold also at −150 HU, resulting in an AUC of 0.903, a cut-off value of 5.4%, a sensitivity of 87.671%, a specificity of 80.296%, an accuracy of 84.646%, and a Youden’s index of 0.67967 (Figure 6).
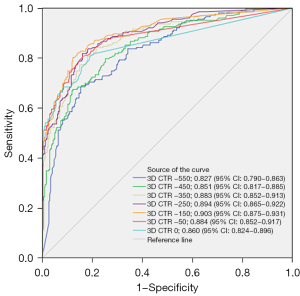
The analysis of the T1c subgroup showed that the predictive performance peaked at the −150 HU threshold, with an AUC of 0.928, a cut-off value of 7.1%, a sensitivity of 88.043%, a specificity of 81.176%, an accuracy of 84.746%, and a Youden’s index of 0.69220 (Figure 7).
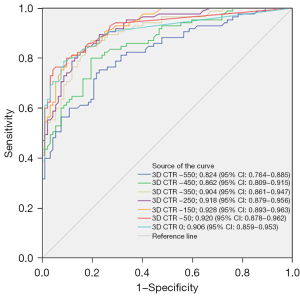
Discussion
In January 2024, the ninth edition of the Lung Cancer TNM Staging was officially implemented in clinical practice. Compared to the eighth edition, there have been no changes to the T staging criteria, which still depend on the size of the tumor and its invasion of adjacent organs. The new standard specifically includes only the solid components of the tumor as the invasive part, excluding the ground-glass opacity (11). This shift underscores the importance of quantifying the solid components within malignant pulmonary nodules, especially in assessing the pathological invasiveness of subsolid nodules (12). The CTR has thus become a pivotal indicator, but a unified measurement standard is still lacking (13).
Due to the uneven density distribution and potential diversity of solid components within lung nodules, the traditional 1D CTR, based on the ratio of the maximum diameter of the assumed single solid component to the maximum diameter of the lung nodule, may deviate to some extent. The JCOG series of studies made breakthroughs in this area, defining the imaging criteria for non-invasive lung cancer: the tumor’s longest diameter should not exceed 2 cm and the CTR should not exceed 0.25, achieving a specificity of 98.7% in predicting the absence of lymph node metastasis and vascular invasion pathologically.
Based on CTR measurements, JCOG (2-5) and other studies (14-17) have further explored the optimal surgical strategy for early-stage non-small cell lung cancer (NSCLC). They found that for lung cancer patients with a diameter not exceeding 3 cm and a CTR not exceeding 0.5, the prognosis of limited pulmonary resection is not inferior to that of lobectomy. These findings have challenged the traditional gold standard of lung cancer surgery—lobectomy plus systematic lymph node dissection—and have sparked extensive discussions in clinical practice. However, it is important to note that the CTR measurements in these studies were all based on one-dimensional CTR.
Threshold segmentation is a fundamental technique in image processing, which categorizes image pixels into different classes or regions based on their pixel values. Leveraging this technique, artificial intelligence-based automated measurement methods have demonstrated higher precision in obtaining the 3D CTR of lung nodules compared to traditional manual measurement of 1D CTR. Researchers such as Zhu (18) have evaluated the performance of automatic deep learning algorithms in measuring the volume of lung adenocarcinoma for predicting prognosis and compared them with manual measurement methods, finding the automatic measurement to be superior in predictive accuracy and more effective for stratified analysis. Sun (19) and colleagues developed an artificial intelligence algorithm for measuring the solid component ratio in lung nodules, comparing it with traditional manual measurement methods, 1D diameter ratio, 2D area ratio, and 3D volume ratio, and confirmed that the 3D volume ratio method is more accurate. Ren (20) also emphasized the significant importance of 3D radiomics features in distinguishing the invasiveness of lung adenocarcinoma.
These research outcomes provide robust support for the theoretical foundation of this study.
Although there is no consensus on the optimal threshold for measuring the solid components of lung nodules, this study employed seven different CT thresholds (−550, −450, −350, −250, −150, −50, 0 HU) to measure 3D CTR using artificial intelligence and predict the invasiveness of stage T1 lung adenocarcinoma. The study found that regardless of the threshold setting, 3D CTR showed statistical differences between non-invasive and invasive groups, indicating its potential as a stable predictive factor for invasive cancer. Particularly, when the CT threshold was set to −150 HU, the predictive performance was optimal, with an AUC and 95% CI of 0.901 (0.883–0.919). Furthermore, when subdivided into T1a, T1b, and T1c subgroups based on the size of the lung nodules, the analysis still identified −150 HU as the optimal threshold.
Currently, surgical approaches for lung nodules mainly include lobectomy, segmentectomy, and wedge resection as subtypes of sublobar resection. Among these, sublobar resection demonstrates unique advantages due to its minimal adverse impact on lung function, inflammatory response, and immune function in patients with NSCLC, leading to faster postoperative recovery (21). Therefore, selecting the appropriate surgical method while ensuring patient prognosis is particularly crucial.
According to some consensus and recommendations from the JCOG series of studies (2-5), for mixed GGO or pure GGO lung nodules with a diameter of less than 2 cm and a CTR not exceeding 0.25, wedge resection with a tumor-free margin greater than 5 mm can be selected. If the intraoperative frozen pathology indicates a non-invasive tumor, lymph node dissection may not be necessary. For lesions with unclear pathology before surgery, wedge resection is recommended as the priority, with further surgery determined by intraoperative pathology. For multiple nodules, the principle is to remove the main lesion as the primary goal while preserving lung function as much as possible, with the optimal site for wedge resection being the best choice.
Artificial intelligence software has developed rapidly in recent years (22). This study utilizes the artificial intelligence-based threshold segmentation method to calculate the 3D CTR at the optimal threshold setting to predict the invasiveness of stage T1 lung adenocarcinoma. This method has significant clinical value in preoperative planning for surgical methods and, combined with intraoperative frozen pathology results, provides more basis for clinical decision-making in cases where intraoperative frozen does not align with clinical considerations.
Study limitation
However, we also recognize that this study has some limitations. All cases included in the analysis are retrospective data, and there are differences in the conditions for CT collection between the two centers. This paper did not include the surgical methods of the included data in the research scope. In addition, this study did not include lung nodules larger than 3 cm in diameter, which may have an impact on the accuracy of the results. Therefore, we look forward to future studies to complement and improve in these aspects.
Conclusions
In summary, we believe that setting the CT threshold at −150 HU to distinguish whether stage T1 lung adenocarcinoma is IAC is reasonable, with the optimal cut-off point being 2.75%. Based on this, we propose that follow-up and intervention strategies be formulated for pulmonary nodules with varying sizes and 3D CTRs at a threshold setting of −150 HU (less than 2.75% for a maximum diameter ≤10 mm, less than 5.4% for maximum diameter >10 to 20 mm, and less than 7.1% for maximum diameter >20 to 30 mm), in accordance with the characteristics of non-invasive adenocarcinoma.
Acknowledgments
Funding: None.
Footnote
Reporting Checklist: The authors have completed the STARD reporting checklist. Available at https://qims.amegroups.com/article/view/10.21037/qims-24-1328/rc
Conflicts of Interest: All authors have completed the ICMJE uniform disclosure form (available at https://qims.amegroups.com/article/view/10.21037/qims-24-1328/coif). L.Z. is an employee of Shukun (Beijing) Technology Co. The other authors have no conflicts of interest to declare.
Ethical Statement: The authors are accountable for all aspects of the work in ensuring that questions related to the accuracy or integrity of any part of the work are appropriately investigated and resolved. The study was conducted in accordance with the Declaration of Helsinki (as revised in 2013). The study was approved by the Institutional Review Boards of The Fifth Clinical Medical College of Henan University of Chinese Medicine (Zhengzhou People’s Hospital) (No. 2024011139) and Huadong Hospital of Fudan University (No. 2019K134), and the requirement for individual consent for this retrospective analysis was waived due to the retrospective nature of the study.
Open Access Statement: This is an Open Access article distributed in accordance with the Creative Commons Attribution-NonCommercial-NoDerivs 4.0 International License (CC BY-NC-ND 4.0), which permits the non-commercial replication and distribution of the article with the strict proviso that no changes or edits are made and the original work is properly cited (including links to both the formal publication through the relevant DOI and the license). See: https://creativecommons.org/licenses/by-nc-nd/4.0/.
References
- Ginsberg RJ, Rubinstein LV. Randomized trial of lobectomy versus limited resection for T1 N0 non-small cell lung cancer. Lung Cancer Study Group. Ann Thorac Surg 1995;60:615-22; discussion 622-3. [Crossref] [PubMed]
- Suzuki K, Koike T, Asakawa T, Kusumoto M, Asamura H, Nagai K, Tada H, Mitsudomi T, Tsuboi M, Shibata T, Fukuda H, Kato HJapan Lung Cancer Surgical Study Group. (JCOG LCSSG). A prospective radiological study of thin-section computed tomography to predict pathological noninvasiveness in peripheral clinical IA lung cancer (Japan Clinical Oncology Group 0201). J Thorac Oncol 2011;6:751-6. [Crossref] [PubMed]
- Suzuki K, Watanabe SI, Wakabayashi M, Saji H, Aokage K, Moriya Y, Yoshino I, Tsuboi M, Nakamura S, Nakamura K, Mitsudomi T, Asamura HWest Japan Oncology Group and Japan Clinical Oncology Group. A single-arm study of sublobar resection for ground-glass opacity dominant peripheral lung cancer. J Thorac Cardiovasc Surg 2022;163:289-301.e2. [Crossref] [PubMed]
- Saji H, Okada M, Tsuboi M, Nakajima R, Suzuki K, Aokage K, et al. Segmentectomy versus lobectomy in small-sized peripheral non-small-cell lung cancer (JCOG0802/WJOG4607L): a multicentre, open-label, phase 3, randomised, controlled, non-inferiority trial. Lancet 2022;399:1607-17. [Crossref] [PubMed]
- Aokage K, Suzuki K, Saji H, Wakabayashi M, Kataoka T, Sekino Y, et al. Segmentectomy for ground-glass-dominant lung cancer with a tumour diameter of 3 cm or less including ground-glass opacity (JCOG1211): a multicentre, single-arm, confirmatory, phase 3 trial. Lancet Respir Med 2023;11:540-9. [Crossref] [PubMed]
- Konno H, Isaka M, Mizuno T, Kojima H, Nagata T, Kawata T, Nakajima T, Endo M, Ohde Y. Validity of surgical decision based on intraoperative frozen section diagnosis for unconfirmed pulmonary nodules with previous malignancy. Gen Thorac Cardiovasc Surg 2022;70:472-8. [Crossref] [PubMed]
- Wu G, Woodruff HC, Sanduleanu S, Refaee T, Jochems A, Leijenaar R, Gietema H, Shen J, Wang R, Xiong J, Bian J, Wu J, Lambin P. Preoperative CT-based radiomics combined with intraoperative frozen section is predictive of invasive adenocarcinoma in pulmonary nodules: a multicenter study. Eur Radiol 2020;30:2680-91. [Crossref] [PubMed]
- Mori S, Noda Y, Shibazaki T, Kato D, Matsudaira H, Hirano J, Ohtsuka T. Definitive lobectomy without frozen section analysis is a treatment option for large or deep nodules selected carefully with clinical diagnosis of malignancy. Thorac Cancer 2020;11:1996-2004. [Crossref] [PubMed]
- Tang J, Cui Y, Li B, Xue X, Tian F. Mathematical prediction model of computed tomography signs is superior to intraoperative frozen section in the diagnosis of ground-glass nodular invasive adenocarcinoma of the lung. Thorac Cancer 2021;12:2382-7. [Crossref] [PubMed]
- Xinli W, Xiaoshuang S, Chengxin Y, Qiang Z. CT-Assisted Improvements in the Accuracy of the Intraoperative Frozen Section Examination of Ground-Glass Density Nodules. Comput Math Methods Med 2022;2022:8967643. [Crossref] [PubMed]
- Van Schil PE, Asamura H, Nishimura KK, Rami-Porta R, Kim YT, Bertoglio P, Cangir AK, Donington J, Fang W, Giroux DJ, Lievens Y, Liu H, Lyons G, Sakai S, Travis WD, Ugalde P, Jeffrey Yang CF, Yotsukura M, Detterbeck F, et al. The International Association for the Study of Lung Cancer Lung Cancer Staging Project: Proposals for the Revisions of the T-Descriptors in the Forthcoming Ninth Edition of the TNM Classification for Lung Cancer. J Thorac Oncol 2024;19:749-65.
- Lin B, Wang R, Chen L, Gu Z, Ji C, Fang W. Should resection extent be decided by total lesion size or solid component size in ground glass opacity-containing lung adenocarcinomas? Transl Lung Cancer Res 2021;10:2487-99. [Crossref] [PubMed]
- Jing W, Liu M, Li W, Li D, Wu Y, Lv F. Prognostic implication of consolidation-to-tumor ratio in early lung adenocarcinoma: a retrospective cross-sectional study. Quant Imaging Med Surg 2024;14:3366-80. [Crossref] [PubMed]
- Zhang C, Pan Y, Li H, Zhang Y, Li B, Zhang Y, et al. Extent of surgical resection for radiologically subsolid T1N0 invasive lung adenocarcinoma: When is a wedge resection acceptable? J Thorac Cardiovasc Surg 2024;167:797-809.e2. [Crossref] [PubMed]
- Lin H, Peng Z, Zhou K, Liang L, Cao J, Huang Z, Chen L, Mei J. Differential efficacy of segmentectomy and wedge resection in sublobar resection compared to lobectomy for solid-dominant stage IA lung cancer: a systematic review and meta-analysis. Int J Surg 2024;110:1159-71. [Crossref] [PubMed]
- Al-Thani S, Nasar A, Villena-Vargas J, Harrison S, Lee B, Port JL, Altorki N, Chow OS. Wedge resection, segmentectomy, and lobectomy: oncologic outcomes based on extent of surgical resection for ≤2 cm stage IA non-small cell lung cancer. J Thorac Dis 2024;16:1875-84. [Crossref] [PubMed]
- Handa Y, Tsutani Y, Okada M. Transition of Treatment for Ground Glass Opacity-Dominant Non-Small Cell Lung Cancer. Front Oncol 2021;11:655651. [Crossref] [PubMed]
- Zhu Y, Chen LL, Luo YW, Zhang L, Ma HY, Yang HS, Liu BC, Li LJ, Zhang WB, Li XM, Xie CM, Yang JC, Wang DL, Li Q. Prognostic impact of deep learning-based quantification in clinical stage 0-I lung adenocarcinoma. Eur Radiol 2023;33:8542-53. [Crossref] [PubMed]
- Sun J, Zhang L, Hu B, Du Z, Cho WC, Witharana P, Sun H, Ma D, Ye M, Chen J, Wang X, Yang J, Zhu C, Shen J. Deep learning-based solid component measuring enabled interpretable prediction of tumor invasiveness for lung adenocarcinoma. Lung Cancer 2023;186:107392. [Crossref] [PubMed]
- Ren H, Xiao Z, Ling C, Wang J, Wu S, Zeng Y, Li P. Development of a novel nomogram-based model incorporating 3D radiomic signatures and lung CT radiological features for differentiating invasive adenocarcinoma from adenocarcinoma in situ and minimally invasive adenocarcinoma. Quant Imaging Med Surg 2023;13:237-48. [Crossref] [PubMed]
- Shin S, Kong S, Kang D, Lee G, Cho JH, Shim YM, Cho J, Kim HK, Park HY. Longitudinal changes in pulmonary function and patient-reported outcomes after lung cancer surgery. Respir Res 2022;23:224. [Crossref] [PubMed]
- Kerdvibulvech C, Dong ZY. Roles of Artificial Intelligence and Extended Reality Development in the Post-COVID-19 Era. International Conference on Human-Computer Interaction. Springer, Cham, 2021.