Analysis of automatic fetal intracranial volume (ICV) measurement based on the optimized ultrasound Smart ICV method at 16–34 weeks of gestation
Introduction
The fetal central nervous system (CNS) undergoes a complex developmental and maturation process throughout pregnancy, which persists after birth (1-4). Fetal brain development in the perinatal period represents a key research area. By assessing the development of the fetal CNS, it is possible to predict the health status and neurodevelopmental outcome of the fetus after birth.
In the past, biparietal diameter and head circumference measurement were commonly used to evaluate fetal brain development (5). Compared to the indirect assessment of the two-dimensional (2D) diameter, the fetal intracranial volume (ICV) can help evaluate the development of the prenatal CNS from the three-dimensional (3D) attributes of the cranial structure. It can also be used to evaluate the actual growth of the fetal brain directly and accurately and to assess any abnormalities in the development of the brain.
Research has demonstrated that magnetic resonance imaging (MRI) has excellent tissue resolution and distinct advantages in volume measurement, and it is frequently employed to evaluate the fetal brain volume (6). However, MRI is relatively expensive, complex, and time-consuming. Therefore, it has not been routinely used in clinical practice for ICV measurement. Instead, it is often employed as a supplementary diagnostic tool when abnormalities are detected by ultrasound examination (7,8). VOCAL has been applied to measure the volumes of the fetal brain and other organs (9-13) and has considerable clinical implications, but its operation is complex.
In recent years, with the rapid development of ultrasound imaging technology and medical image-processing methodology, a software tool for automatic measurement of fetal ICV, the Smart ICV, has been developed (14). This tool includes a function that provides automatic 3D fetal ICV calculation for fetuses at a gestational age (GA) of 19–34 weeks (8) with a single press of a button. However, previous studies on this technology have primarily consisted of consistency analyses between Smart ICV and VOCAL (8,14), and these investigations have mainly included fetuses aged between 20 and 34 weeks, as Smart ICV is not applicable to fetuses at an earlier GA (16–19 weeks). However, the growth curve of fetal ICV automatically measured via Smart ICV has not been examined.
This study thus aimed to optimize and validate the performance of the Smart ICV method using the 3D ultrasound volume data of 200 cases at 16–19 weeks of gestation. To verify its clinical efficiency, the optimized Smart ICV was compared with the VOCAL at 16–34 weeks of gestation. Finally, by using 950 sets of ultrasound volume data, we established the growth curve of fetal ICV at 16–34 weeks of gestation based on the optimized Smart ICV to analyze changes in ICV at different GAs and to provide a more suitable basis for the diagnosis of fetal craniocerebral abnormalities. We present this article in accordance with the GRRAS reporting checklist (available at https://qims.amegroups.com/article/view/10.21037/qims-24-1379/rc).
Methods
Patients
A total of 1,140 healthy pregnant women attending the Hefei Women and Children’s Healthcare Hospital from June 2021 to March 2023 were randomly selected. The pregnant women were aged 22–40 (30.45±4.25) years. Among these cases, 190 were lost to follow-up or had poor image quality, and the remaining 950 cases were enrolled in the study.
To be eligible for inclusion in this study, the participants had to meet the following inclusion criteria: be pregnant with a single fetus with a GA of 16–34 weeks (calculated according to the last menstrual period or crown-rump length measured by ultrasound at 11–13 weeks of gestation). Participants were excluded from the study if they met any of the following exclusion criteria: (I) pregnancy complications, such as gestational diabetes, and gestational hypertension; (II) diseases such as chronic hypertension and systemic lupus erythematosus; (III) a fetus with chromosomal abnormalities, structural malformations, fetal growth restriction (FGR), etc.; and (IV) poor imaging of the fetus due to respiratory movement or thick abdominal wall fat. This study was conducted in accordance with the Declaration of Helsinki (as revised in 2013) and approved by the Ethics Committee of the Hefei Women and Children’s Healthcare Hospital (No. YYLL2021-yj008-02-01). Informed consent was obtained from all the patients.
Ultrasound examination
The Nuewa R9 ultrasound imaging system with the SD8-1U transabdominal volume probe (1–8 MHz; Mindray Medical, Shenzhen, China) was used for the ultrasound examination. In each examination, fetal biometry was measured to estimate the GA, and structural screening was also captured. The ultrasound system was operated by two physicians with more than 5 years’ experience in prenatal ultrasound screening.
For the 2D mode, the transthalamic cross-section was selected as the initial plane with fixed levels for gain (=55), frame rate (=23 frame per second), and dynamic range (=120) to ensure the consistency of data acquisition. For the 3D mode, the surface mode was chosen, the scanning quality was set to high, the sampling frame was adjusted to include the entire fetal cranium surface, and the scanning angle was set to 50–70°. The Smart Scene 3D brain mode was then selected, and during the acquisition of 3D ICV images, the participants were asked to hold their breath. The “automatic ICV measurement” option was selected under the S-Planes CNS menu. The 3D fetal ICV images were obtained and stored. The average value of the data was recorded after three successive measurements.
Optimization of ICV measurement with Smart ICV at 16–19 weeks of gestation
A total of 200 healthy fetuses at 16–19 weeks of gestation were selected for algorithm optimization (n=120) and validation (n=80). The Hausdorff distance was applied to evaluate the improvement of the Smart ICV algorithm in identifying the boundary of the fetal brain.
The Smart ICV is a fast and robust software program that segments the cranial contour and calculates the ICV in 3D fetal ultrasound images. The algorithm flowchart is shown in Figure 1. First, the 3D fetal brain volume data were radially resampled into multiple 2D planes at a fixed angle. Radial sampling has its unique advantages: (I) since 3D ultrasound involves fan-shaped scanning, radial sampling conforms to the imaging principle of 3D ultrasound. (II) When carried out with a single organ as the axis, radial sampling can guarantee that the organ is relatively intact on each sampling section (in contrast to slice-by-slice sampling). (III) Owing to the constraints of the ultrasound imaging principle, acoustic shadow artifacts will be generated behind the highly echogenic region. Numerous artifacts can appear on the sections sampled slice by slice, leading to segmentation errors. However, radial sampling can reduce this artifact. Second, each 2D plane was segmented using a graph cut algorithm. In graph cut, the image is modeled as a graph structure, where nodes represent pixels and the weights of the edges reflect the similarity between pixels. By minimizing the cost function and solving the minimum cut problem, the graph cut can achieve effective image segmentation. Third, the 3D segmentation mask was further reconstructed from the masks of all the resampled 2D planes, and the ICV measurement was calculated.
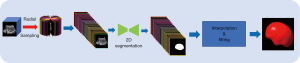
As reported previously (8,14), Smart ICV is commonly used for the ICV calculation of fetuses at 19–34 weeks of gestation and has shown excellent performance. Compared to that after 20 weeks, the region of the fossa before 20 weeks differs in terms of both texture and morphology, leading to inconsistency in the segmenting boundary. Therefore, to improve the segmentation accuracy of the region of fossa for fetal brains at earlier GAs, we introduced several strategies, including redesign of edge detectors, data augmentation, and fine-tuning of parameters to improve segmentation performance, particularly at the boundaries of the segmented regions. (I) We optimized the model by employing a combination of loss functions tailored for better boundary segmentation. The loss function we used included edge loss, Dice loss, and focal loss. Edge loss was incorporated to enhance the model’s focus on the boundaries of the segmented regions. By emphasizing the importance of edges, the model could better capture the fine details and reduce boundary ambiguity. Dice loss helped maximize the overlap between the predicted and true segmentation, improving overall segmentation accuracy, particularly in complex regions. Focal loss was included to address the issue of class imbalance in the dataset. This loss function allows the model to focus on harder-to-classify regions, thus improving segmentation in regions where boundary detection is more challenging. (II) To further enhance the model’s generalization capability, we used cycle-consistent generative adversarial networks (CycleGAN) for data augmentation. Ultrasound images from different subjects often exhibit variations in image characteristics due to differences in machine settings, probes, and manufacturers. CycleGAN was employed to learn these variations and augment the training data with images that simulate different styles. (III) We conducted an unsupervised pretraining of our model using large volumes of unlabeled ultrasound images from different organs. Through contrastive learning, the model was trained to distinguish between different image features, even in the absence of labeled data.
Comparison of fetal ICV measured using Smart ICV and VOCAL
Both the optimized Smart ICV and VOCAL were employed to validate the accuracy of the fetal ICV calculation. For the Smart ICV, the whole process and calculation were fully automated to single-click operation. For the VOCAL method, three sampling settings (6, 12, and 18 planes) were used for the ICV calculation. At each selected plane, the boundary of the fetal brain was manually traced, and the total ICV was reconstructed in the 3D space based on all of the 2D delineations. This manual measurement process was repeated three times for each participant. All the measurement results and time duration were recorded. Figure 2 shows the ICV measurement results derived from the 6-plane VOCAL (Figure 2A), 12-plane VOCAL (Figure 2B), 18-plane VOCAL (Figure 2C), and optimized Smart ICV method (Figure 2D) on a fetus at 20 weeks of gestation. The performance of the two methods was then compared and analyzed.
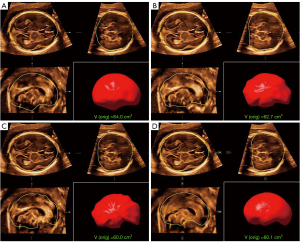
Additionally, the data of 60 healthy fetuses at 16–34 weeks of gestation were collected to validate the intra- and inter-observer consistency. Under the condition that the fetal position was appropriate and relatively fixed, the first examiner obtained the 3D ICV images twice to evaluate the intra-observer consistency. Without knowing the measurement results of the first examiner, a second examiner then obtained ICV images for the same participants on the same day to evaluate the inter-observer measurement consistency. The two examiners independently obtained the ICV results using the optimized Smart ICV. Finally, at least 2 weeks later, they obtained the ICV using the VOCAL method.
ICV growth curve and Z-score
First, Pearson correlation analysis was used to analyze the correlation coefficients between ICV with GA and head circumference. Then, to analyze the growth of the fetal ICV in the second and third trimesters, 950 cases of healthy fetuses at 16–34 weeks of gestation were randomly selected and divided into 19 groups of 50 cases per week. The best-fit growth curve for the Smart ICV data was established, and the normal range for the Z-score was then calculated. Finally, the ICV variation trend for fetuses with different GAs was analyzed. The best-fit growth curve for the Smart ICV data was established, and the normal range for the Z-score was then calculated. Finally, the ICV variation trend for fetuses with different GAs was analyzed.
Statistical analysis
SPSS 24 (IBM Corp., Armonk, NY, USA) was used for the statistical analysis. The normally distributed measurement data are expressed as the mean ± standard deviation (SD). If the measurement data conformed to a normal distribution and the variance was homogeneous, one-way analysis of variance (ANOVA) was used for comparisons between groups. The intra- and inter-observer consistencies were analyzed using the intraclass correlation coefficient (ICC) and Bland-Altman analyses, respectively. The optimal regression model for the Smart ICV measurement was established for the GA, and the Z-score scatter plot was obtained. The Shapiro-Wilk test was used to verify the normality of the Z-scores. A P value <0.05 was considered statistically significant.
Results
Population characteristics
For the 950 healthy pregnant women, the (I) mean age was 30.45±4.25 years; (II) the body mass index was 26.23±2.31 kg/m2; and (III) the GA at delivery was 38±3 weeks.
Smart ICV optimization
Based on the validation dataset of 80 fetuses at earlier GAs (16–19 weeks), each fetal ICV was processed with both the original and optimized versions of the Smart ICV. The 18-plane VOCAL (manual annotation) was also analyzed. The segmentation results of the optimized Smart ICV showed a higher correlation with the 18-plane VOCAL than did the original version, and had a lower mean Hausdorff distance (optimized: 1.15±0.25 mm; original: 1.31±0.93 mm). Figure 3 shows the 3D mapping of the Hausdorff distance between the Smart ICV and 18-plane VOCAL before and after the algorithm optimization on a randomly selected fetal brain. Meanwhile, as seen in Figure 4, the boundary of the fetal brain obtained by the optimized Smart ICV was closer to the manual annotation than was the original version, especially in the region indicated by the red arrow.
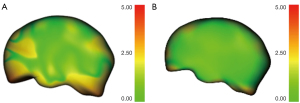
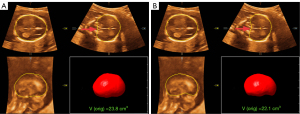
Intra- and inter-observer measurements for Smart ICV and VOCAL modes
For the ICV data obtained with the Smart ICV and the 6-, 12-, and 18-plane VOCAL, the ICC values for the same observer were 0.998 [95% confidence interval (CI): 0.996–0.999], 0.962 (95% CI: 0.934–0.978), 0.982 (95% CI: 0.968–0.989), and 0.997 (95% CI: 0.995–0.998), respectively; meanwhile, the ICC values for two observers were 0.991 (95% CI: 0.988–0.996), 0.941 (95% CI: 0.920–0.969), 0.951 (95% CI: 0.932–0.976), and 0.981 (95% CI: 0.979–0.991), respectively. The Bland-Altman analysis showed that the ICV data for both modes had good agreement, and there was no statistically significant difference between the two modes in terms of the mean difference, intercept of the regression equation, or slope (P>0.05). Moreover, in terms of the mean values, the smallest difference in the mean values and the 95% CI was between the Smart ICV and 18-plane VOCAL (Table 1). The ICV data obtained using these methods were in good agreement (Figure 5), confirming the accuracy of the optimized Smart ICV.
Table 1
Parameters | Mean difference (95% CI) |
P value | Regression equation | ||||
---|---|---|---|---|---|---|---|
Intercept | Slope | ||||||
95% CI | P value | 95% CI | P value | ||||
Smart ICV and 6-plane ICV (cm3) | −3.18 (−7.99, 1.63) | 0.19 | 0.52 (−8.93, 9.96) | 0.91 | −0.03 (−0.08, 0.03) | 0.37 | |
Smart ICV and 12-plane ICV (cm3) | −3.16 (−6.37, 0.04) | 0.06 | −3.75 (−10.15, 2.65) | 0.24 | 0.004 (−0.04, 0.04) | 0.83 | |
Smart ICV and 18-plane ICV (cm3) | 0.23 (−1.51, 1.96) | 0.79 | 1.24 (−2.16, 4.65) | 0.47 | −0.007 (−0.03, 0.01) | 0.49 |
ICV, intracranial volume; CI, confidence interval.
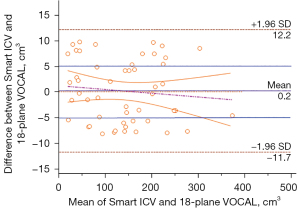
The time durations for obtaining the ICV data with the Smart ICV and the 6-, 12-, and 18-plane VOCAL were 3.7±0.7, 80.5±11.4, 120.0±26.5, and 153.1±29.5 s, respectively. The one-way ANOVA showed that there were significant differences in the time duration for ICV acquisition between the different measurement methods (P<0.05). The Smart ICV not only required the shortest amount of time but also had the highest inspection efficiency compared to the VOCAL method. Among the different VOCAL methods, the 6-plane VOCAL had shortest duration while the 18-plane VOCAL had the longest one due to the increased number of traced planes.
ICV growth curve and Z-score analysis based on Smart ICV
Smart ICV correlation analysis
In the Pearson correlation analysis, the coefficients for the correlation of ICV with GA and head circumference were 0.984 and 0.969, respectively (all P values <0.001), and the correlation between Smart ICV and GA was stronger.
Growth curve analysis of the Smart ICV results based on GA
We found that as the GA increased, the ICV increased. Among the functions fitted using the different modes, the cubic, S-curves, and quadratic curves had the highest values R2 values of 0.982, 0.981, and 0.978, respectively. The best-fit model for the Smart ICV with GA was the cubic function, which is expressed as follows: y = −44.2445 + 0.1427x2 + 0.0052x3, where y is the ICV, and x is the gestational week (Figure 6). The fetal ICV relative to GA based on Smart ICV was established (Table 2), and the growth trend of the fetal Smart ICV, obtained through the derivative of the growth curve, was evaluated. The results showed that the fetal Smart ICV exhibited accelerated in growth in the second trimester, which became steady in the late-pregnancy period (Figure 7).
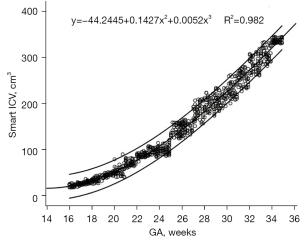
Table 2
GA (weeks) | 5th (cm3) | 50th (cm3) | 95th (cm3) |
---|---|---|---|
16 | 17.40 | 22.58 | 26.29 |
17 | 23.46 | 27.99 | 33.19 |
18 | 28.89 | 36.42 | 44.69 |
19 | 38.37 | 45.44 | 57.42 |
20 | 47.44 | 55.65 | 73.71 |
21 | 60.85 | 68.32 | 85.07 |
22 | 73.95 | 84.51 | 96.26 |
23 | 86.84 | 97.64 | 114.58 |
24 | 100.50 | 112.08 | 133.57 |
25 | 119.03 | 138.05 | 148.37 |
26 | 130.38 | 144.40 | 161.94 |
27 | 151.16 | 167.54 | 201.62 |
28 | 170.06 | 193.43 | 220.95 |
29 | 183.70 | 215.23 | 238.46 |
30 | 200.33 | 231.97 | 269.27 |
31 | 221.90 | 255.36 | 290.23 |
32 | 263.17 | 284.75 | 319.24 |
33 | 281.81 | 305.08 | 335.30 |
34 | 331.64 | 345.33 | 364.22 |
ICV, intracranial volume; GA, gestational age.
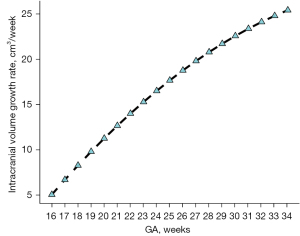
Analysis of the normal range of Z-scores of the Smart ICV based on GA
The Z-score was used to verify the goodness of fit of the regression models. With GA and the ICV, the following Z-scoring equation was established according to the following regression equation:
The Shapiro-Wilk test was used to verify the normality of the Z-score (Figure 8). The Z-score of the ICV from the Smart ICV calculated according to the equation above revealed no obvious change in the trend. All the residual changes in the SD fell within the normal range (Figure 9). These results indicated that the regression model had a good fit.
Discussion
Smart ICV is a fast and powerful software-based method that can automatically segment the brain and calculate 3D fetal ICV. In this study, 120 cases with a GA of 16–19 weeks were included for algorithm optimization, which included redesigning the edge detector, data enhancement, and parameter fine-tuning. In redesigning edge detectors, we used edge loss, Dice loss, and focal loss to optimize the model. The combined effect of these loss functions could ensure that the model not only maintained high overall accuracy but also excelled in segmenting boundary regions, which is crucial for clinical applications. With data enhancement implemented via CycleGAN, this process enriched the diversity of the training dataset and allowed our model to better generalize across different datasets, improving robustness in clinical settings. We perform fine-tuning of the parameters via unsupervised pretraining using contrastive learning. This pretraining step allowed the model to learn generalizable features from ultrasound images, improving its ability to segment 3D ultrasound images with minimal labeled data. These optimization strategies improved segmentation performance and enhanced the segmentation accuracy of the fetal brain fossa area at earlier GAs. Subsequently, in the algorithm verification, when compared with the original version, the optimized Smart ICV produced a segmentation result closer to that of the VOCAL-18 plane, and the Hausdorff distance was lower. This demonstrates that Smart ICV was improved in its ability to recognize fetal brain boundaries at 16 to 19 weeks. The early detection and diagnosis of diseases are crucial to effective treatment, and thus there is an inclination to conduct prenatal ultrasound structural screening as early as is feasible. The International Society of Ultrasound in Obstetrics and Gynecology (ISUOG) has formulated guidelines for early-pregnancy fetal structural screening (15). The accuracy of diagnosis of complex and severe congenital heart disease (CHD) in early pregnancy and the echocardiographic features of various CHDs have been reported (16,17). Therefore, the assessment of brain development of fetuses with CHD needs to be improved. The expansion of the application scope of Smart ICV can meet this clinical need.
In previous studies, the VOCAL method was often used to measure the volume of organs (8-12). VOCAL can accurately measure fetal ICV, but extensive training is required in the early stage. The accuracy of the manual characterization of ICV improves with the increase in operator experience (18). In this study, 60 healthy fetuses at 16–34 weeks were included to evaluate the consistency of Smart ICV and VOCAL. Other studies (8,14) have conducted similar analyses and confirmed that 6-plane VOCAL is in good agreement with the Smart ICV, reporting a shorter examination time (8.2±4.5 vs. 121.3±19.0 s, P<0.0001). The novelty of our study is that in addition to analyzing the 6-plane VOCAL method, we also examined 12- and 18-plane VOCAL. With the increase of the number of VOCAL manual delineation planes, the fitting of is more precise and closer to the actual condition, with the 18-plane VOCAL being superior to the 6- and 12-plane VOCAL in this regard but requiring more time. The consistency analysis in our study indicated that the Smart ICV and 6-, 12-, and 18-plane VOCAL groups had good intra- and inter-observer consistency. In addition, Smart ICV and 18-plane VOCAL had the highest consistency, with Smart ICV requiring the shorter examination time (3.7±0.7 vs. 153.1±29.5 s, P<0.05). Therefore, Smart ICV can accurately evaluate fetal ICV and has greater examination efficiency, which is convenient for clinical promotion. It can be applied in the automatic evaluation of fetal ICV development in the second and third trimesters of pregnancy, providing additional diagnostic evidence for clinical practice.
Quantitative analysis of ICV is crucial to brain development assessment and clinical decision-making. The newly developed and optimized Smart ICV can achieve accurate and automatic separation of the fetal brain, obtain fetal ICV automatically, and reduce the time required for manual segmentation and editing. As a result, in this study, a large sample size (n=950) was used and provided growth curves and percentiles of automatic ICV from a GA of 16–34 weeks. This period is when obstetric screening commonly occurs and can critically inform the management of fetal brain development. In a previous VOCAL study (19), the number of cases was not as large as that in our study due the complexity of the VOCAL workflow. In VOCAL, the reference axis is set manually, and multiple 2D planes (6, 12, and 18 planes) are obtained by rotating the reference axis. The 2D contours of the brain in all planes are manually traced. Finally, all the 2D contours are matched to form a 3D brain volume. Even highly trained operators require extensive time to complete the measurement of a single ICV, which greatly limits its application in clinical studies.
In addition, the ICV growth curve and ICV growth rate generated from the optimized Smart ICV indicated that ICV followed a cubic growth pattern. The growth in ICV accelerated in the second and third trimesters of pregnancy, and the craniocerebral structure gradually developed and improved. In our study, the ICV increased by more than 12 times from 16 to 34 weeks. Another VOCAL study (19) reported that from 18 to 40 weeks, ICV increased by more than 10 times. This may be related to the complex development and growth of the brain. During the fetal period, the brain undergoes extensive reorganization, which includes neuron cell proliferation, neurogenesis, gliogenesis, cell migration, cell differentiation, synaptogenesis and connection formation, cortical folding, and axonal myelination (4). Ren et al. (20) examined 188 fetuses ranging in GA from 19 to 37 weeks for analysis and found that with the change of GA, ICV followed a quadratic growth pattern. This differs from the ICV growth pattern observed in our study. The reasons for this may be that the gestational weeks included were not completely overlapping, the number of cases in each group was different, and the imaging methods were not the same. In addition, the ICV measured by ultrasound is smaller than that measured by MRI, and there are some differences between these methods. As MRI has better spatial resolution and tissue contrast, the estimated fetal ICV may be more accurate. However, the advantages of our approach are also significant. The Smart ICV method can analyze ICV changes more quickly, which is conducive to the rapid identification of abnormal fetuses and is also suitable for earlier GAs; meanwhile, MRI is relatively time-consuming, complicated, and expensive. In addition, due to the small fetal brain size, poor tissue differentiation, and frequent fetal movement at the earlier GA, MRI is often used in the fetuses after 19 weeks in clinical practice. Only a few studies (21,22) have explored MRI brain development assessment in the first trimester, and one study (21) included only fetal specimens that had already stopped developing.
Furthermore, in our study, the ICV based on 6-plane VOCAL was greater than that based on 12- and 18-VOCAL and on Smart ICV. Moreover, the ICV of 18-plane VOCAL was similar to that obtained by Smart ICV. Compared with the ICV obtained by VOCAL in a previous study (19), that in our study was smaller, but not significantly so. This slight discrepancy most likely stems from the disparity in the number of planes. In our study, the growth curve adopted was that of 6-plane VOCAL, and the number of depicted planes was not as numerous and the fitting degree not as accurate as that of 18-plane VOCAL. Moreover, the ICV in our study was in line with one reported elsewhere (19), supporting the validity of our results and confirming the reliability of using the optimized Smart ICV method to quantify ICV. In addition, we established a Z-score based on Smart ICV and GA and found that SD for all residual changes was ±3. The Z-score provides accurate quantitative evaluation of parameters and can be easily applied in clinical settings. The regression model can further be verified as having a good fit if the effectiveness of the Z-score is evaluated, which provides an accurate diagnostic basis for clinical quantitative evaluation. The Z-score has been used in quantitative studies of fetal heart in the past (23-27).
At any stage in the process of brain growth and maturation, when influenced by certain unfavorable factors, the structure and function of the brain may be altered. Previous studies have demonstrated that in cases in which the fetuses have FGR or CHD or in which pregnant women have gestational diabetes mellitus (GDM) or preeclampsia (28-31), the development of the fetal brain undergoes changes to varying extents. Impaired placental circulation, which leads to insufficient placental blood perfusion and subsequently causes fetal ischemia and hypoxia, constitutes a pathophysiological alteration in the pathogenesis of FGR. Chronic ischemia and hypoxia in the fetus lead to blood redistribution. Changes in brain metabolism and remodeling of the fetal brain may directly influence fetal brain growth and functional changes. The total brain volume of FGR fetuses is decreased, the development of gray matter and white matter is hindered, and the brain tissue and connectivity are altered. The severity is mediated by the timing of fetal damage in relation to the impact on brain development. Among the related conditions, early-onset FGR (occurring before 32 weeks) emerges earlier. The extent of brain damage is broader and more complex, manifesting as diffuse neuroinflammation. This gives rise to diffuse white-matter damage and extensive loss of mature myelin, thereby leading to a reduction in the number of mature oligodendrocytes (32). For fetuses with CHD, anatomical and hemodynamic changes impair the delivery of nutrients to the brain, consequently resulting severe impediments to brain development and maturation. This optimized technology (Smart ICV) can be more readily used to assess brain volume changes in these fetuses. Compared with the traditional VOCAL, it has higher efficiency and better repeatability and will be further investigated in our future prospective studies.
Marra et al. (33) evaluated the development of the cerebral cortex structure in healthy fetuses at 19 to 34 weeks of gestation and found that the depth of the Sylvian fissure (SF), parieto-occipital fissure (POF), and calcarine fissure (CF) increased significantly with greater GA and head circumference but were more closely related to head circumference. Meanwhile, in our study, ICV increased significantly with the increase in GA and head circumference. Pearson correlation analysis showed that the correlation coefficients between ICV and GA and head circumference were 0.984 and 0.969, respectively. Sex may also play a role in brain development. A fetal brain MRI study (34) reported that the growth of brain volume and different cranial-cerebral structures were related to GA and sex. Mappa et al. (35) demonstrated that sex is also a factor in the development of the healthy fetal cerebral cortex, exerting a more significant influence in fetuses after 28 weeks. However, in a different study (20), no significant difference in the fetal ICV of different genders was reported. In our study, we did not analyze the gender factor. In the future, we can also conduct a secondary analysis on the correlation between gender and ICV obtained via Smart ICV.
Certain limitations to this study should be noted. First, after 34 weeks, due to the interference of the skull shadow, the difficulty in automatic tracing of the fetal ICV increased while its accuracy decreased significantly. Second, our analysis centered on the ICV based on the Smart ICV and its changes in healthy fetuses; however, abnormal fetuses must also be considered in future research. Third, the accuracy of ICV can be influenced by fetal movement and the thickness of abdominal wall fat in pregnant women, which led to the blurring of images with numerous motion artifacts, making it difficult for the Smart ICV to obtain ICV data. Finally, the relationship between Smart ICV and gender was not scrutinized, while the devices used were restricted to a single manufacturer. However, only Mindray has developed an ultrasound diagnostic instrument that can automatically measure fetal ICV.
Conclusions
The study optimized the Smart ICV to render it more suitable for the rapid acquisition of the fetal ICV at 16–34 weeks of pregnancy, thereby improving its clinical applicability. The results of the optimized Smart ICV showed excellent consistency with those of the 18-plane VOCAL. The optimized Smart ICV method has the potential to be a reliable tool for fetal growth assessment during the second and third trimesters. Moreover, a cubic growth curve of ICV and GA derived from a sample of 950 cases from a single center was established based on the optimized Smart ICV.
Acknowledgments
Funding: This work was supported by
Footnote
Reporting Checklist: The authors have completed the GRRAS reporting checklist. Available at https://qims.amegroups.com/article/view/10.21037/qims-24-1379/rc
Conflicts of Interest: All authors have completed the ICMJE uniform disclosure form (available at https://qims.amegroups.com/article/view/10.21037/qims-24-1379/coif). The authors have no conflicts of interest to declare.
Ethical Statement: The authors are accountable for all aspects of the work in ensuring that questions related to the accuracy or integrity of any part of the work are appropriately investigated and resolved. This study was conducted in accordance with the Declaration of Helsinki (as revised in 2013) and was approved by the Ethics Committee of the Hefei Women and Children’s Healthcare Hospital (No. YYLL2021-yj008-02-01). Informed consent was obtained from all the patients.
Open Access Statement: This is an Open Access article distributed in accordance with the Creative Commons Attribution-NonCommercial-NoDerivs 4.0 International License (CC BY-NC-ND 4.0), which permits the non-commercial replication and distribution of the article with the strict proviso that no changes or edits are made and the original work is properly cited (including links to both the formal publication through the relevant DOI and the license). See: https://creativecommons.org/licenses/by-nc-nd/4.0/.
References
- Urbanik A, Cichocka M, Kozub J, Karcz P, Herman-Sucharska I. Brain Maturation-Differences in Biochemical Composition of Fetal and Child's Brain. Fetal Pediatr Pathol 2017;36:380-6. [Crossref] [PubMed]
- Nishikuni K, Ribas GC. Study of fetal and postnatal morphological development of the brain sulci. J Neurosurg Pediatr 2013;11:1-11. [Crossref] [PubMed]
- Andescavage NN, du Plessis A, McCarter R, Serag A, Evangelou I, Vezina G, Robertson R, Limperopoulos C. Complex Trajectories of Brain Development in the Healthy Human Fetus. Cereb Cortex 2017;27:5274-83. [PubMed]
- Dudink I, Hüppi PS, Sizonenko SV, Castillo-Melendez M, Sutherland AE, Allison BJ, Miller SL. Altered trajectory of neurodevelopment associated with fetal growth restriction. Exp Neurol 2022;347:113885. [Crossref] [PubMed]
- Malinger G, Paladini D, Haratz KK, Monteagudo A, Pilu GL, Timor-Tritsch IE. ISUOG Practice Guidelines (updated): sonographic examination of the fetal central nervous system. Part 1: performance of screening examination and indications for targeted neurosonography. Ultrasound Obstet Gynecol 2020;56:476-84. [Crossref] [PubMed]
- Harkey T, Baker D, Hagen J, Scott H, Palys V. Practical methods for segmentation and calculation of brain volume and intracranial volume: a guide and comparison. Quant Imaging Med Surg 2022;12:3748-61. [Crossref] [PubMed]
- Jarvis DA, Finney CR, Griffiths PD. Normative volume measurements of the fetal intra-cranial compartments using 3D volume in utero MR imaging. Eur Radiol 2019;29:3488-95. [Crossref] [PubMed]
- Prayer D, Malinger G, De Catte L, De Keersmaecker B, Gonçalves LF, Kasprian G, Laifer-Narin S, Lee W, Millischer AE, Platt L, Prayer F, Pugash D, Salomon LJ, Sanz Cortes M, Stuhr F, Timor-Tritsch IE, Tutschek B, Twickler D, Raine-Fenning NISUOG Clinical Standards Committee. ISUOG Practice Guidelines (updated): performance of fetal magnetic resonance imaging. Ultrasound Obstet Gynecol 2023;61:278-87. [Crossref] [PubMed]
- Kalache KD, Espinoza J, Chaiworapongsa T, Londono J, Schoen ML, Treadwell MC, Lee W, Romero R. Three-dimensional ultrasound fetal lung volume measurement: a systematic study comparing the multiplanar method with the rotational (VOCAL) technique. Ultrasound Obstet Gynecol 2003;21:111-8. [Crossref] [PubMed]
- Resta S, Scandella G, Mappa I, Pietrolucci ME, Maqina P, Rizzo G. Placental Volume and Uterine Artery Doppler in Pregnancy Following In Vitro Fertilization: A Comprehensive Literature Review. J Clin Med 2022;11:5793. [Crossref] [PubMed]
- Wang H, Yan B, Yue L, He M, Liu Y, Li H. The Diagnostic Value of 3D Power Doppler Ultrasound Combined With VOCAL in the Vascular Distribution of Breast Masses. Acad Radiol 2020;27:198-203. [Crossref] [PubMed]
- Zhang J, Lyu G, Qiu J, Qiu S, Li Z, Lin M, Xiao X, Tang L, He J, Li X, Li S. Three-dimensional ultrasound VOCAL combined with contrast-enhanced ultrasound: an alternative to contrast-enhanced magnetic resonance imaging for evaluating ablation of benign uterine lesions. Int J Hyperthermia 2022;39:1360-70. [Crossref] [PubMed]
- Bravo-Valenzuela NJ, Peixoto AB, Carrilho MC, Siqueira Pontes AL, Chagas CC, Simioni C, Araujo Júnior E. Fetal cardiac function by three-dimensional ultrasound using 4D-STIC and VOCAL - an update. J Ultrason 2019;19:287-94. [Crossref] [PubMed]
- Grisolia G, Pinto A. Smart ICV™ versus VOCAL™ in fetal brain volume assessment: Can we begin to trust artificial intelligence in clinical practice? J Clin Ultrasound 2023;51:1152-4. [Crossref] [PubMed]
- Bilardo CM, Chaoui R, Hyett JA, Kagan KO, Karim JN, Papageorghiou AT, Poon LC, Salomon LJ, Syngelaki A, Nicolaides KH. ISUOG Practice Guidelines (updated): performance of 11-14-week ultrasound scan. Ultrasound Obstet Gynecol 2023;61:127-43. [Crossref] [PubMed]
- Yu D, Sui L, Zhang N. Performance of First-Trimester Fetal Echocardiography in Diagnosing Fetal Heart Defects: Meta-analysis and Systematic Review. J Ultrasound Med 2020;39:471-80. [Crossref] [PubMed]
- Ximenes RS, Bravo-Valenzuela NJ, Pares DBS, Araujo Júnior E. The use of cardiac ultrasound imaging in first-trimester prenatal diagnosis of congenital heart diseases. J Clin Ultrasound 2023;51:225-39. [Crossref] [PubMed]
- Ioannou C, Sarris I, Salomon LJ, Papageorghiou AT. A review of fetal volumetry: the need for standardization and definitions in measurement methodology. Ultrasound Obstet Gynecol 2011;38:613-9. [Crossref] [PubMed]
- He H, Shu S, Lan W, Peng C, Ma M, Li K. Three-dimensional ultrasound virtual organ computer-aided analysis to monitor fetal intracranial volume development characteristics: A multi-center study in a Chinese population. J Clin Ultrasound 2023;51:74-81. [Crossref] [PubMed]
- Ren JY, Zhu M, Wang G, Gui Y, Jiang F, Dong SZ. Quantification of Intracranial Structures Volume in Fetuses Using 3-D Volumetric MRI: Normal Values at 19 to 37 Weeks' Gestation. Front Neurosci 2022;16:886083. [Crossref] [PubMed]
- Zhan J, Dinov ID, Li J, Zhang Z, Hobel S, Shi Y, Lin X, Zamanyan A, Feng L, Teng G, Fang F, Tang Y, Zang F, Toga AW, Liu S. Spatial-temporal atlas of human fetal brain development during the early second trimester. Neuroimage 2013;82:115-26. [Crossref] [PubMed]
- Cortes-Albornoz MC, Calixto C, Bedoya MA, Didier RA, Estroff JA, Jaimes C. Fetal Brain Growth in the Early Second Trimester. AJNR Am J Neuroradiol 2023;44:1440-4. [Crossref] [PubMed]
- Rocha LA, Zielinsky P, Nicoloso LHS, Araujo E Junior. Development of the Z-score for the measurement of myocardial thickness by two-dimensional echocardiography in normal fetuses. Echocardiography 2021;38:97-102. [Crossref] [PubMed]
- Mao YK, Zhao BW, Zhou L, Wang B, Chen R, Wang SS. Z-score reference ranges for pulsed-wave Doppler indices of the cardiac outflow tracts in normal fetuses. Int J Cardiovasc Imaging 2019;35:811-25. [Crossref] [PubMed]
- Luewan S, Srisupundit K, Tongprasert F, Traisrisilp K, Jatavan P, Tongsong T. Z Score Reference Ranges of Fetal Cardiac Output From 12 to 40 Weeks of Pregnancy. J Ultrasound Med 2020;39:515-27. [Crossref] [PubMed]
- DeVore GR. Computing the Z Score and Centiles for Cross-sectional Analysis: A Practical Approach. J Ultrasound Med 2017;36:459-73. [Crossref] [PubMed]
- Mao YK, Lou HY, Pan M, Zhao BW. Z-Score Reference Ranges for the Offset of the Tricuspid Septal Leaflet in Normal Fetuses. Fetal Diagn Ther 2019;46:58-66. [Crossref] [PubMed]
- Marra MC, Mappa I, Pietrolucci ME, Lu JLA, D' Antonio F, Rizzo G. Fetal brain development in pregnancies complicated by gestational diabetes mellitus. J Perinat Med 2024;52:310-6. [Crossref] [PubMed]
- Basso A, Youssef L, Nakaki A, Paules C, Miranda J, Casu G, Salazar L, Gratacos E, Eixarch E, Crispi F, Crovetto F. Fetal neurosonography at 31-35 weeks reveals altered cortical development in pre-eclampsia with and without small-for-gestational-age fetus. Ultrasound Obstet Gynecol 2022;59:737-46. [Crossref] [PubMed]
- Mappa I, Marra MC, Pietrolucci ME, Lu JLA, D'Antonio F, Rizzo G. Midline structures and cortical development in late-onset fetal growth restriction according to Doppler status: prospective study. Ultrasound Obstet Gynecol 2024;64:228-35. [Crossref] [PubMed]
- Rizzo G, Pietrolucci ME, De Vito M, Pavjola M, Capponi A, Mappa I. Fetal brain biometry and cortical development in congenital heart disease: A prospective cross sectional study. J Clin Ultrasound 2023;51:84-90. [Crossref] [PubMed]
- Alves de Alencar Rocha AK, Allison BJ, Yawno T, Polglase GR, Sutherland AE, Malhotra A, Jenkin G, Castillo-Melendez M, Miller SL. Early- versus Late-Onset Fetal Growth Restriction Differentially Affects the Development of the Fetal Sheep Brain. Dev Neurosci 2017;39:141-55. [Crossref] [PubMed]
- Marra MC, Pietrolucci ME, Mappa I, Lu JLA, Di Mascio D, D'Antonio F, Rizzo G. Modeling fetal cortical development by quantile regression for gestational age and head circumference: a prospective cross sectional study. J Perinat Med 2023;51:1212-9. [Crossref] [PubMed]
- Machado-Rivas F, Gandhi J, Choi JJ, Velasco-Annis C, Afacan O, Warfield SK, Gholipour A, Jaimes C. Normal Growth, Sexual Dimorphism, and Lateral Asymmetries at Fetal Brain MRI. Radiology 2022;303:162-70. [Crossref] [PubMed]
- Mappa I, Marra MC, Pietrolucci ME, Lu JLA, Di Mascio D, D'Antonio F, Rizzo G. Effects of gender on fetal cortical development: a secondary analysis of a prospective cross-sectional study. J Perinat Med 2024;52:114-6. [Crossref] [PubMed]