The causal effects of gut microbiota on quantitative susceptibility mapping (QSM) and T2* imaging-derived phenotypes: insights from a Mendelian randomization study
Introduction
Our understanding of the physiological and biochemical connectivity among the brain, digestive tract, and gut microbiota continues to increase (1). This bidirectional information transforming system is called the “gut-microbiota-brain axis” (2). Signaling molecules that originate from the gut microbiota and act on the central nervous system (CNS) can be classified as either food-related metabolites [e.g., short-chain fatty acids (SCFAs)], metabolites of endogenous molecules (e.g., secondary bile acids and tryptophan metabolites), and microbial cell wall components (e.g., lipopolysaccharides) (1-3). Abnormalities in the biological mechanisms of the microbiota microenvironment could cause CNS disorders [e.g., Parkinson’s disease (PD), Alzheimer’s disease (AD), and multiple sclerosis] (4). For example, patients with depression and anxiety have increased levels of pro-inflammatory bacteria, such as Enterobacteriaceae and Eggerthella, and lower levels of bacteria that excrete anti-inflammatory SCFAs, such as Faecalibacterium and Coprococcus, which can lead to abnormalities in the immune system (3). Currently, little is known about the biological mechanisms of the gut microbiota that cause cerebral physiological or pathological alterations; however, these alterations can be reflected in neuroimaging to some extent (5).
Magnetic resonance imaging (MRI) is a non-invasive neuroimaging technique that can acquire high-throughput information related to cerebral metabolism, function, and structure (6). Imaging data from large-scale neuroimaging investigations are usually extracted as interpretable imaging-derived phenotypes (IDPs). Examples of IDPs include regional volume and thickness, and functional connectivity data (7,8). These imaging characteristics have been observed to change in a variety of CNS disorders (9-11). Homeostatic disorders in gut microbiota are associated with cerebral IDPs. For example, fractional anisotropy, an indicator of brain structure integrity, has been found to be positively correlated with actinobacteria abundance in the thalamus and amygdala (12). Similarly, regional homogeneity, which is an index for detecting cerebral activity, in the right superior temporal cortex, left cuneus, and right middle temporal cortex of patients with schizophrenia has been found to be negatively correlated with the relative abundance of genus Roseburia (13). However, to date, little research has been conducted on the causal interaction between brain iron deposition and gut microbiota.
The disturbance of brain iron homeostasis can lead to oxidative stress and the generation of reactive oxygen species (14-16). Through quantitative susceptibility mapping (QSM), magnetic susceptibility sources like iron can be described and quantified (16,17). The magnetic susceptibility values of paramagnetic substances, including deoxyhemoglobin, iron (ferritin), and hemosiderin, present as high contrast, while those of diamagnetic substances, including myelin, oxygenated hemoglobin, and calcification, present as low contrast (18). Such values provide an indication of pathologic variations of myelin, calcification, and iron deposition (18). For example, both pre-symptomatic and symptomatic Huntington’s disease patients have abnormal iron deposition in the globus pallidus, putamen, and caudate (16). Further, QSM can be used to detect substantial cortical iron deposition in the brains of AD patients, which is associated with cognitive decline and brain atrophy (19).
Additionally, the magnetic sensitivity amplitude of tissues can be reflected in T2* mapping. Iron deposition destroys the homogeneity of the local magnetic field, resulting in a decrease in the T2* value, which is reflected in a decrease in the tissue signal intensity (20). In general, a lower T2* value corresponds to more iron deposition. T2* mapping is widely used to detect hemosiderin, methemoglobin, and deoxyhemoglobin in tissue lesions, such as superficial siderosis, cerebral hemorrhage, calcification, arteriovenous malformation, and cavernoma (20). Therefore, the process of generating IDPs from brain iron deposition indicators (i.e., T2* and QSM) is to transform the brain iron imaging data into visualized image phenotypes. A previous study showed that gut microbiota was associated with cerebral T2*-related IDPs (21). Specifically, the percentages of change in the relative abundance of Gemmatimonadetes, Bacteroidetes, and Proteobacteria have been linked to two-year changes in the brain iron deposition R2* value (R2* = 1/T2*) in the caudate (21). Figure 1 shows the relationship between the gut microbiota and brain QSM and T2* IDPs, as well as their possible roles in CNS diseases. Our investigation of their causal associations will extend understandings of the neurobiological mechanisms that underly the “gut-brain-microbiota axis”.
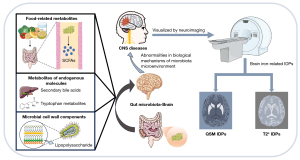
Observational studies face challenges in controlling confounding factors. Conversely, randomized control trials can be conducted to explore causal relationships, but face limitations related to technology and the study methods, and can also be overly expensive, unworkable, time consuming, and even unethical (22,23). Recently, Mendelian randomization (MR) has become an increasingly popular epidemiological analytic approach. MR employs genetic instrumental variables (IVs) to assess the causal relationships between exposure and outcome (22). During meiotic gametes’ formation, alleles from parents are randomly assigned to their offspring (22,24). Thus, confounding factors such as the external environment, socioeconomic status, and behavioral habits cannot affect the interactions between genes and phenotypes, ensuring the reliability of the causal relationships (25). In addition, the IVs used in MR analyses must meet the following assumptions (22,26): (I) the relevance assumption (i.e., IVs are strongly related to exposure variables); (II) the independence assumption: (i.e., IVs cannot be confounded by factors); and (III) the exclusivity assumption: (i.e., IVs have no direct influence on outcome variables).
Genome-wide association study (GWAS) data allow MR to be used to investigate causal relationships between the gut microbiota and brain iron-related IDPs (27). In the present study, we performed one-way two-sample MR analyses to estimate the causal relationships between the 196 gut microbiota, and 18 QSM IDPs and 10 T2* IDPs. It is feasible that MR can detect correlations between microbiome and brain iron-related IDPs by using genetic variations between individuals that underlie variations in gut microbiome abundance (28). This study’s preliminary findings could pave the way for future research to identify biomarkers and biotargets based on the gut microbiota that might aid in the diagnosis and therapeutic management of CNS diseases. We present this article in accordance with the STROBE-MR reporting checklist (available at https://qims.amegroups.com/article/view/10.21037/qims-24-318/rc).
Methods
In this study, one-way two-sample MR analyses were performed to assess the causal association between the gut microbiota and brain iron-related IDPs. The study was conducted in accordance with the Declaration of Helsinki (as revised in 2013).
Exposure data
MiBioGen is the most widely used GWAS database for human gut microbiota. It combines 16S ribosomal RNA (rRNA) gene sequencing profiles and genotyping data from 18,340 people across 24 cohorts (data link: https://mibiogen.gcc.rug.nl) (29). It contains 20 cohorts that comprise single ancestry samples, including European (16 cohorts, n=13,266), Middle Eastern (1 cohort, n=481), East Asian (1 cohort, n=811), American Hispanic/Latino (1 cohort, n=10,097), and African American (1 cohort, n=114) samples, and four cohorts that comprise polygenetic ancestry samples (n=2,571). Because these cohorts are heterogeneous in terms of ethnic background, age, gender ratios, and diet, per-cohort and whole-study filtering was used for the inclusion of taxa in the GWAS (29).
Gut microbiota composition was analyzed based on three different variable regions of the 16S rRNA gene (i.e., V4, V3–V4, and V1–V2), and direct taxonomic binning was carried out. Sequencing reads were processed and aligned to reference databases to identify and quantify the relative abundance of the different taxa in each sample, whose skewness was addressed by log transformation. For each cohort, only the taxa present in more than 10% of the samples were included in the quantitative microbiome trait loci mapping to identify the genetic loci that affected the relative abundance (29,30). Additionally, 15 unknown bacterial taxa were removed. Ultimately, as available online: https://cdn.amegroups.cn/static/public/qims-24-318-1.xlsx shows, 196 taxa were included in the MR analyses, comprising 9 phyla, 20 orders, 16 classes, 32 families, and 119 genera (29). Beta estimates were calculated using the least square method to examine the effects of the alleles on the exposure factors.
Outcome data
The GWAS data of the brain iron-related IDPs were obtained from the United Kingdom Biobank (UKB), which contains de-identified data and samples from half a million UK participants for health-related research (data link: https://www.fmrib.ox.ac.uk/ukbiobank/gwas_resources/index.html). Brain susceptibility-weighted MRI (swMRI) data from 35,885 participants released by the UKB in early 2020 were used for the QSM processing. At the time of the scans, all of the participants were in good health. QSM processing involves several procedures, starting with the combination, masking, and unwrapping of the phase data from each channel, followed by background field removal, and finally dipole inversion (18,31). After removing the unusable data from the QSM processing, QSM-based-IDPs were generated in various brain structures from 35,273 participants from a prospective epidemiological study. To prevent confounding effects related to environmental or demographic factors, 29,579 unrelated participants with recent UK ancestry were selected for the GWAS. We collected 18 QSM IDPs containing 16 subcortical regions in the bilateral cerebral hemisphere (i.e., the thalamus, caudate, putamen, pallidum, hippocampus, amygdala, accumbens, and substantia nigra), and two white matter hyperintensity (WMH) IDPs with/without regressing out WMH volume.
The median T2* IDPs were also obtained from the swMRI data from the UKB. The T2* values were calculated using the magnitude data. First, the R2* (i.e., the inverse of the T2*) was calculated based on two echo times, and the T2* images were then spatially filtered to reduce noise and converted to the T1 space by linear registration (32). Notably, in brain imaging, slice thickness exacerbates the bias caused by the existence of a macroscopic field gradient due to the air/tissue interface or poor magnetic field shim quality, which affects T2* estimates. To eliminate this confounding effect on the association analyses of the UKB T2* IDPs, the effect of the macroscopic field gradient was simulated using the UKB swMRI protocol to assess the bias caused by the T2* estimates. Macroscopic field gradient maps along the slice-direction were then generated for all the UKB participants, and the median gradient magnitude in each subcortical region of interest was calculated. Finally, the link between the macroscopic field gradient and the R2* was modeled using data from the UKB participants with large variances in the median gradient amplitude (18,33). As a result, a confounding variable for the macro field gradient that was unique to each participant and brain region was created, which was then regressed out from the T2* IDPs. No correlation was found between the field gradient and the QSM IDPs; hence, this particular confounding variable mainly applied to the T2* data. Thus, we obtained 10 T2* IDPs containing six subcortical regions in the bilateral cerebral hemisphere (i.e., the hippocampus, amygdala, and accumbens) with the additional deconfounding of the background field gradient, two substantia nigra in the bilateral cerebral hemisphere, and 2 WMH IDPs with/without regressing out WMH volume.
FMRIB’s Integrated Registration and Segmentation Tool (34) was used to segment each subcortical structure region of interest (excluding the substantia nigra), the atlas in the Montreal Neurological Institute space (35) was used to segment the substantia nigra, and Brain Intensity AbNormality Classification Algorithm processing was used to segment the WMH (36).
IV selection
To ensure the authenticity and precision of the results in relation to the causal association between the gut microbiota and the brain iron-related IDPs, the IVs were selected using the following selection criteria: (I) the single nucleotide polymorphisms (SNPs) were strongly correlated with the gut microbiota (P<1.0×10−5); (II) gene inheritance was completely random based on the linkage disequilibrium analysis and clumping procedure (R2<0.001 and clumping distance =10,000 kb) (22,26,37); and (III) the effects of the SNPs on exposure and outcome were related to the same allele (vague SNPs with inconsistent alleles and palindromic SNPs with uncorrectable vague chains during the coordination process were removed).
Statistical analysis
To guarantee the robustness of the results, we used two independent analysis processes by selecting the statistically significant IVs with distinct level of statistical strictness. In the first analysis, no alleles were flipped, as it was assumed that all the alleles were coded on the forward strand. In the second analysis, the strand for non-palindromic SNPs was corrected and all palindromic SNPs were removed from the analysis. The significant results at the intersection of these two analyses became our final results. A flow path of the study is shown in Figure 2.
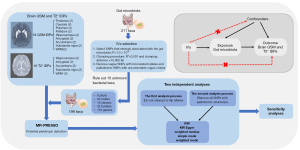
In the above two analysis processes, the following five MR analysis methods were used to estimate the causal relationships between the gut microbiota and brain iron-related IDPs: inverse variance weighting (IVW), MR-Egger regression, weighted median, simple mode, and weighted mode. IVW is a method for aggregating two or more random variables to minimize the variance of the sum, and it is used in preliminary analyses to assess causality. In this study, IVW was used as a primary analytical method to determine the MR estimates for each exposure-outcome relationship. If horizontal pleiotropy is not significant, IVW can provide a relatively stable causal assessment by means of a meta-analysis combining the Wald ratio of each SNP to exposure and outcome (38). Additionally, to further ensure the robustness of the result, the β estimate must meet the directional consistency of that of the other four MR analysis methods. To assess the reliability of our results, we performed heterogeneity and pleiotropy sensitivity analyses (with both p values set at 0.1) (25). We used Cochran’s Q test to assess the heterogeneity of the IVs (39). We then used MR-Egger regression and the MR-pleiotropy residual sum and outlier (MR-PRESSO) test to examine horizontal pleiotropy. In the MR-Egger intercept test, the 0 value is compared to the MR-egger intercept term. If the MR-Egger regression intercept is not 0, it indicates that there is no horizontal pleiotropy (37). MR-PRESSO evaluates the size of horizontal pleiotropy by summing the residuals of each SNP. The MR-PRESSO global test detects the presence of horizontal pleiotropy, while the MR-PRESSO outlier test corrects for horizontal pleiotropy by taking turns to compare the distance between a SNP and the total fitting line (residual sum of squares) to remove abnormal SNPs (outliers), and the MR-PRESSO distortion test determines whether there is a significant difference in the causal effect before and after the removal of the outliers. If there is no significant difference in the causal estimates before and after the removal of the outliers, it indicates that no horizontal pleiotropy exists (40). Finally, we calculated the F statistic (with the lowest threshold set at 10) to assess the strength of each IV (22,37).
Two-sample MR (version 0.5.6) and MR-PRESSO (version 1.0) packages in R version 4.2.1. (R Foundation for Statistical Computing, Vienna, Austria) were used for all the analyses to examine the causal relationships between the gut microbiota and brain iron-related IDPs.
Results
Effects of gut microbiota on QSM and T2* IDPs
Clumping and harmonizing was performed, and the details for all IVs of both analysis process are shown in available online: https://cdn.amegroups.cn/static/public/qims-24-318-2.xlsx; and https://cdn.amegroups.cn/static/public/qims-24-318-3.xlsx, respectively. The proportion of overlapping samples between the exposure and outcome was less than 5%. We set 0.05/(196×28) as the Bonferroni correction threshold of the p value. Overall, in the first analysis process, one bacteria order, two families, and six genera were found to be significantly associated with the QSM and T2* IDPs. Conversely, in the second analysis process, one phylum, one family, and 14 genera were found to be significantly associated with the QSM and T2* IDPs. The MR analysis results of both the analysis processes are presented in available online: https://cdn.amegroups.cn/static/public/qims-24-318-4.xlsx and https://cdn.amegroups.cn/static/public/qims-24-318-5.xlsx, respectively. After intersecting the results of these two analysis processes, one family and five genera were identified, and the intersected results were taken as the final outcomes.
In the intersection results of two MR analyses, we found that the genus Howardella was positively correlated with median susceptibility in the right caudate [β: 0.0935, 95% confidence interval (CI): 0.0601, 0.1269, PIVW=4.00E−08]. The genus Dialister was positively correlated with median susceptibility in the right accumbens (β: 0.0949, 95% CI: 0.0575, 0.1324, PIVW=6.90E−07). The genus Butyricicoccus was positively correlated with the median T2* in the left hippocampus with the additional deconfounding of the background field gradient (β: 0.1543, 95% CI: 0.0959, 0.2127, PIVW=2.20E−07). Both the family Defluviitaleaceae (β: −0.1215, 95% CI: −0.1604, −0.0827, PIVW=8.40E−10) and genus Defluviitaleaceae UCG011 (β: −0.1142, 95% CI: −0.1614, −0.0670, PIVW=2.10E−06) were negatively correlated with the median T2* in the right accumbens with the additional deconfounding of the background field gradient. The genus Desulfovibrio was positively correlated with the T2* WMH IDP with WMH volume regressed out (β: 0.1168, 95% CI: 0.0697, 0.1639, PIVW=1.20E−06).
The results of other supplemental MR methods were directionally consistent with the IVW method. Table 1 presents the MR analysis results of six bacterial taxa in the first analysis. Due to random variations, discrepancies in sensitivity to outliers, and different biases between the intrinsic properties of the statistical methods (rather than any violation of the assumptions of IVs), differences in the beta estimates between the IVW and MR-Egger approaches might exist (41). Additionally, among the six causal associations, the IVs had an F statistical range of 23.65 to 37.67, which indicates a low possibility of weak IV bias.
Table 1
Gut microbiome (exposure) | QSM/T2* IDPs (outcome) | MR method | No. SNP | F | β (95% CI) | P value |
---|---|---|---|---|---|---|
Genus Howardella | Median susceptibility in the right caudate | IVW (MRE) | 11 | 30.13 | 0.0935 (0.0601, 0.1269) | 4.00E−08 |
MR Egger | 11 | 0.0132 (−0.2473, 0.2736) | ||||
Weighted median | 11 | 0.0901 (0.0174, 0.1629) | ||||
Simple mode | 11 | 0.0824 (−0.0279, 0.1927) | ||||
Weighted mode | 11 | 0.0830 (−0.0294, 0.1954) | ||||
Genus Dialister | Median susceptibility in the right accumbens | IVW (MRE) | 12 | 24.66 | 0.0949 (0.0575, 0.1324) | 6.90E−07 |
MR Egger | 12 | 0.1355 (−0.2586, 0.5296) | ||||
Weighted median | 12 | 0.0992 (−0.0218, 0.2203) | ||||
Simple mode | 12 | 0.1083 (−0.0773, 0.2939) | ||||
Weighted mode | 12 | 0.1057 (−0.0811, 0.2925) | ||||
Genus Butyricicoccus | Median T2* in the left hippocampus | IVW (MRE) | 9 | 26.83 | 0.1543 (0.0959, 0.2127) | 2.20E−07 |
MR Egger | 9 | 0.1461 (−0.0434, 0.3357) | ||||
Weighted median | 9 | 0.1445 (0.0067, 0.2822) | ||||
Simple mode | 9 | 0.1235 (−0.0735, 0.3205) | ||||
Weighted mode | 9 | 0.1354 (−0.0175, 0.2882) | ||||
Family Defluviitaleaceae | Median T2* in the right accumbens | IVW (MRE) | 12 | 37.67 | −0.1215 (−0.1604, −0.0827) | 8.40E−10 |
MR Egger | 12 | −0.1447 (−0.4030, 0.1136) | ||||
Weighted median | 12 | −0.1244 (−0.2179, −0.0309) | ||||
Simple mode | 12 | −0.1341 (−0.2682, 4.169×10−5) | ||||
Weighted mode | 12 | −0.1306 (−0.2776, 0.0164) | ||||
Genus DefluvitaleaceaeUCG011 | Median T2* in the right accumbens | IVW (MRE) | 10 | 22.47 | −0.1142 (−0.1614, −0.0670) | 2.10E−06 |
MR Egger | 10 | −0.2219 (−0.5120, 0.0682) | ||||
Weighted median | 10 | −0.1170 (−0.2190, −0.0151) | ||||
Simple mode | 10 | −0.1246 (−0.2965, 0.0474) | ||||
Weighted mode | 10 | −0.1236 (−0.2947, 0.0476) | ||||
Genus Desulfovibrio | T2* white matter hyperintensity IDP with WMH volume regressed out | IVW (MRE) | 11 | 23.65 | 0.1168 (0.0697, 0.1639) | 1.20E−06 |
MR Egger | 11 | 0.1023 (−0.1557, 0.3602) | ||||
Weighted median | 11 | 0.0841 (−0.0295, 0.1977) | ||||
Simple mode | 11 | 0.0672 (−0.0974, 0.2318) | ||||
Weighted mode | 11 | 0.0680 (−0.0887, 0.2247) |
MR, Mendelian randomization; QSM, quantitative susceptibility mapping; IDPs, imaging-derived phenotypes; SNP, single nucleotide polymorphism; CI, confidence interval; IVW, inverse variance weighted; MRE, multiplicative random effects model; WMH, white matter hyperintensity.
Sensitivity analyses
Several sensitivity analyses were conducted to ensure the results were reliable (available online: https://cdn.amegroups.cn/static/public/qims-24-318-6.xlsx, Table 2). The results of the Cochran’s Q test revealed no heterogeneity in these six causal associations (all P>0.1). While the results of the MR-Egger regression intercept and MR-PRESSO analysis revealed no horizontal pleiotropy (all P>0.1). Thus, the results of the sensitivity analyses provided further evidence of the reliability of our MR analyses.
Table 2
Gut microbiome (exposure) | QSM/T2* IDPs (outcome) | No. SNP | Pleiotropy | Heterogeneity | |||
---|---|---|---|---|---|---|---|
MR-PRESSO global (P value) | MR-egger (P value) |
IVW test (P value) |
MR-egger (P value) |
||||
Genus Howardella | Median susceptibility in the right caudate | 11 | 0.9691 | 0.5512 | 0.9626 | 0.9538 | |
Genus Dialister | Median susceptibility in the right accumbens | 12 | 0.9995 | 0.8397 | 0.9993 | 0.9983 | |
Genus Butyricicoccus | Median T2* in the left hippocampus | 9 | 0.9664 | 0.9226 | 0.9560 | 0.9188 | |
Family Defluviitaleaceae | Median T2* in the right accumbens | 12 | 0.9938 | 0.8576 | 0.9931 | 0.9863 | |
Genus DefluvitaleaceaeUCG011 | Median T2* in the right accumbens | 10 | 0.9717 | 0.4697 | 0.9660 | 0.9671 | |
Genus Desulfovibrio | T2* white matter hyperintensity IDP with WMH volume regressed out | 11 | 0.9804 | 0.9094 | 0.9809 | 0.9640 |
QSM, quantitative susceptibility mapping; IDPs, imaging-derived phenotypes; SNP, single nucleotide polymorphism; MR-PRESSO, Mendelian randomization-pleiotropy residual sum and outlier; MR, Mendelian randomization; IVW, inverse variance weighted; WMH, white matter hyperintensity.
Discussion
Using MR analysis, we assessed the possible causal relationships between the gut microbiota and brain iron-related IDPs. Using the gut microbiota data from MiBioGen, the QSM IDPs, and the T2* IDPs data from UKB, we discovered six bacterial taxa that were causally related with the QSM IDPs and T2* IDPs.
The gut microbiome and its metabolites play an important role in gut-brain axis communication via immune, neuronal, and endocrine pathways (42). Changes in the gut microbiota of PD patients have been shown to induce neuroinflammation, activate microglia, and result in brain iron deposits (43). A previous study assessed the fecal samples from PD patients, and observed an increase in pro-inflammatory bacteria and a decrease in SCFAs-producing bacteria, particularly anti-inflammatory butyrate-producing bacteria (44). Compared to controls, the stools of PD patients have been found to have higher levels of multiple inflammatory mediators (45). Conversely, SCFAs have been shown to exert anti-inflammatory effects that affect the enteric nervous system, promote normal microglia growth, and may regulate neuroinflammation (44). Further, evidence suggests that the gut microbiota is involved in neuroinflammation, which is a key pathophysiological mechanism for AD (43). This suggests that the dysbiosis of the gut microbiota, particularly the ratio of pro-inflammatory to anti-inflammatory microbes, may be critical to the development of diseases.
Butyriciciccus is a microbe that produces butyrate (46). Butyricum exerts a neuroprotective effect, and can considerably reduce neurodegeneration via the modulation of metabolite butyrate, thereby preventing blood-brain-barrier dysfunction and inhibiting neuroinflammatory responses (47). Patients with early PD have been reported to have a lower abundance of Butyriciciccus (44). In this study, we found that the genus Butyricicoccus was positively correlated with the median T2* in the left hippocampus with the additional deconfounding of the background field gradient, indicating a negative association with iron deposition. Previous research has shown that neonatal iron overload contributes to alterations connected to hippocampus memory impairment (48). Silva et al. claimed that the sodium butyrate could entirely restore iron-induced deficiencies in recognition memory (48). Histone acetylation remolding of the chromatin is an important epigenetic process for controlling gene transcription during memory formation. Histone deacetylases induce chromatin condensation and limit gene transcription. As a histone deacetylase inhibitor, sodium butyrate eases memory damage and enhances memory formation (48). Our findings could inform cognitive impairment treatments and future research on the connections between the genus Butyricicoccus and brain iron deposition.
Conversely, Defluviitaleaceae has been found to be negatively correlated with the butyricum level (49). Notably, genera are members of families, and a family contains various genera. There was a strong overlap between the sets of IVs present at the low taxonomic level and the relevant sets of IVs contained at the high taxonomic level. Specifically, we found that both family Defluviitaleaceae and genus Defluviitaleaceae UCG011 were negatively correlated with the median T2* in the right accumbens with the additional deconfounding of the background field gradient. The ketogenic diet has been shown to exert a protective effect in neurodegenerative diseases (50). One study fed PD mice a ketogenic diet for eight weeks, and the results indicated that the PD group had a higher relative abundance of Defluviitaleacea, and the ketogenic diet significantly reduced this relative abundance (51). A higher abundance of Defluviitaleaceae has also been reported in permethrin-induced PD animal models (52), which suggests that Defluviitaleaceae may impair cognitive function. Additionally, we found that the genus Dialister was positively correlated with median susceptibility in the right accumbens. Thus, both Dialister and Defluviitaleaceae promote iron deposition in the right accumbens.
A previous study reported that PD patients treated with scalp-abdominal electroacupuncture therapy had significantly improved symptoms, accompanied by a significant decline in the relative abundance of the genus Dialister (53). This suggests that the genus Dialister is positively correlated with the severity of PD. PD and its motor abnormalities are brought on by the selective and gradual destruction of dopaminergic neurons in the substantia nigra or other basal ganglia structures (15). Iron levels have been found to be significantly increased in multiple brain regions in PD patients (54). The excessive iron deposition exacerbates PD by damaging dopaminergic neurons (55). According to Cuesta et al., there is a strong correlation between iron levels in the right accumbens and PD signs, which suggests that the right accumbens is engaged in the pathologic processes of PD’s motor abnormalities (56). Additionlly, Wang et al. have found that the increased iron levels in the right accumbens enhance the risk of PD (57). Our findings suggest that both Defluviitaleaceae and Dialister promote iron deposition in the right accumbens. Future studies should seek to examine other mechanisms between Defluviitaleaceae and Dialister and PD to aid in the development of therapeutic interventions for PD.
WMH has frequently been linked to impaired cognition and is a type of neuroimaging biomarker characterized by enhanced T2-weighted sequence signals (58). Various studies have shown that WMH is accompanied by increased specific motor symptoms or cognitive abnormalities in patients with PD (58-60), and that there is a link between total WMH volume and cognitive and/or motor dysfunction in PD (61). We found that the genus Desulfovibrio was positively correlated with the T2* WMH IDP with WMH volume regressed out. Desulfovibrio is a sulfate-reducing bacterium that gains energy by dissimilating sulfate reduction to generate sulfide (62). Hydrogen sulfide created by Desulfovibrio can cause severe CNS impairment and seriously disrupt iron metabolism by increasing iron accumulation in the cytoplasm (63). Additionally, Desulfovibrio creates magnetite, and both magnetite and hydrogen sulfide cause α-synuclein to oligomerize and aggregate (62,63). Recent research suggests that Desulfovibrio is a risk factor for PD (64). PD patients usually present with inflammation of the digestive tract, which tends to worsen with disease development (64). Our results provide further evidence that Desulfovibrio plays a role in PD and could serve as a potential therapeutic target.
Uchida et al. reported that the QSM value in the caudate of PD patients with mild cognitive impairment was negatively correlated with cognitive impairment scores and was higher than that in healthy controls and cognitively normal PD patients (65). Further, the iron content in the caudate and cortex of PD patients with cognitive disorders was found to be increased (15). Our results showed that the genus Howardella was positively correlated with median susceptibility in the right caudate. Interestingly, to date, no study appears to have sought to verify the association between the genus Howardella and brain iron deposition in the right caudate, which might be worthy of further study in the future.
This study had a number of strengths. First, we employed two independent analysis processes by selecting the statistically significant IVs with a distinct level of strictness, and we took the intersection of the results of these two MR analyses as our final result to ensure the robustness of our results. Second, in each analysis process, we employed five different MR estimate methods to guarantee the reliability of the results. Third MR-Egger and MR-PRESSO were used to eliminate effects of heterogeneity and horizontal pleiotropy. In addition, the sample size of the GWAS database used in this study was considerable.
However, this study also had several limitations. First, the lowest level of taxonomy in the exposure data was genus, which prevented us from further investigating the causal relationships between the gut microbiota and brain iron-related IDPs at the species level. Second, due to the limited number of SNPs reaching the genome-wide statistical significance, the threshold was relaxed (P<1.0×10–5) instead of the standard genome-wide statistical significance threshold (P<5.0×10–8). Third, the abundance of the gut microbiota can also be affected by external environmental, genetic, and lifestyle factors, which can result in subtle variations in genetic interpretation. Fourth, all the GWAS data included in this study were collected from Europe populations. As a result, further research is required to determine whether the findings of this study can be applied to other non-European populations. Fifth, skewed measurements like relative abundance might go against the linearity of MR assumptions. Hence, log, rank-based inverse normal, or other transformations could be applied to better meet the linearity assumption (66,67). Additionally, future studies should seek to employ advanced non-linear MR approaches. Sixth, MR studies can only be applied to risk factors with suitable genetic variants, which might have reduced the statistical power (Significance level >0.05) and increased the possibility of false-negative results. Consequently, the statistical power could be enhanced by using numerous genetic variations linked to risk factors as IVs to increase the proportion of variance interpretation. Further, while MR studies can provide valuable insights into the potential causal relationships between the exposure and outcome, it is challenging to thoroughly verify the hypothesis and determine the real causal effect. Therefore, the interpretation of MR findings should take into account evidence from other sources, including observational and experimental studies.
Conclusions
This study showed the causal effects of the gut microbiota on the brain iron-related IDPs by MR analysis. Our findings could provide novel insights into the management of CNS disorders from the perspective of the “brain-gut microbiota” axis. In our opinion, more importance should be attached to monitoring the gut microbiome in the early stage of neurodegenerative diseases to identify more risk predictors and potentially regulate beneficial microbiome groups.
Acknowledgments
We would like to thank the participants and investigators from the United Kingdom Biobank and MiBioGen Consortium for sharing the GWAS data. Additionally, we would like to thank Servier Medical Art (http://smart.servier.com/) for their assistance in creating Figures 1 and 2, under a Creative Common Attribution 3.0 Generic License (https://creativecommons.org/licenses/by/3.0/).
Funding: This work was supported by
Footnote
Reporting Checklist: The authors have completed the STROBE-MR reporting checklist. Available at https://qims.amegroups.com/article/view/10.21037/qims-24-318/rc
Conflicts of Interest: All authors have completed the ICMJE uniform disclosure form (available at https://qims.amegroups.com/article/view/10.21037/qims-24-318/coif). L.Y. reports that this work was supported by the Fundamental Research Funds for the Central Universities of Central South University (No. 1053320230244). S.G. is a current employee of Infervision Medical Technology Co., Ltd. J.L. reports that this work was supported by the National Natural Science Foundation of China (No. U22A20303), the Innovative Province Special Construction foundation of Hunan Province (No. 2019SK2131), the Science and Technology Innovation Program of Hunan province (No. 2021RC4016), and the Clinical Research Center for Medical Imaging in Hunan Province in China (No. 2020SK4001). The other authors have no conflicts of interest to declare.
Ethical Statement: The authors are accountable for all aspects of the work in ensuring that questions related to the accuracy or integrity of any part of the work are appropriately investigated and resolved. The study was conducted in accordance with the Declaration of Helsinki (as revised in 2013).
Open Access Statement: This is an Open Access article distributed in accordance with the Creative Commons Attribution-NonCommercial-NoDerivs 4.0 International License (CC BY-NC-ND 4.0), which permits the non-commercial replication and distribution of the article with the strict proviso that no changes or edits are made and the original work is properly cited (including links to both the formal publication through the relevant DOI and the license). See: https://creativecommons.org/licenses/by-nc-nd/4.0/.
References
- Mayer EA, Nance K, Chen S. The Gut-Brain Axis. Annu Rev Med 2022;73:439-53. [Crossref] [PubMed]
- Góralczyk-Bińkowska A, Szmajda-Krygier D, Kozłowska E. The Microbiota-Gut-Brain Axis in Psychiatric Disorders. Int J Mol Sci 2022;23:11245. [Crossref] [PubMed]
- Socała K, Doboszewska U, Szopa A, Serefko A, Włodarczyk M, Zielińska A, Poleszak E, Fichna J, Wlaź P. The role of microbiota-gut-brain axis in neuropsychiatric and neurological disorders. Pharmacol Res 2021;172:105840. [Crossref] [PubMed]
- Liu L, Huh JR, Shah K. Microbiota and the gut-brain-axis: Implications for new therapeutic design in the CNS. EBioMedicine 2022;77:103908. [Crossref] [PubMed]
- Ong IM, Gonzalez JG, McIlwain SJ, Sawin EA, Schoen AJ, Adluru N, Alexander AL, Yu JJ. Gut microbiome populations are associated with structure-specific changes in white matter architecture. Transl Psychiatry 2018;8:6. [Crossref] [PubMed]
- De Santis S, Moratal D, Canals S. Radiomicrobiomics: Advancing Along the Gut-brain Axis Through Big Data Analysis. Neuroscience 2019;403:145-9. [Crossref] [PubMed]
- Elliott LT, Sharp K, Alfaro-Almagro F, Shi S, Miller KL, Douaud G, Marchini J, Smith SM. Genome-wide association studies of brain imaging phenotypes in UK Biobank. Nature 2018;562:210-6. [Crossref] [PubMed]
- Miller KL, Alfaro-Almagro F, Bangerter NK, Thomas DL, Yacoub E, Xu J, et al. Multimodal population brain imaging in the UK Biobank prospective epidemiological study. Nat Neurosci 2016;19:1523-36. [Crossref] [PubMed]
- Hibar DP, Westlye LT, Doan NT, Jahanshad N, Cheung JW, Ching CRK, et al. Cortical abnormalities in bipolar disorder: an MRI analysis of 6503 individuals from the ENIGMA Bipolar Disorder Working Group. Mol Psychiatry 2018;23:932-42. [Crossref] [PubMed]
- van Rooij D, Anagnostou E, Arango C, Auzias G, Behrmann M, Busatto GF, et al. Cortical and Subcortical Brain Morphometry Differences Between Patients With Autism Spectrum Disorder and Healthy Individuals Across the Lifespan: Results From the ENIGMA ASD Working Group. Am J Psychiatry 2018;175:359-69. [Crossref] [PubMed]
- Kelly S, Jahanshad N, Zalesky A, Kochunov P, Agartz I, Alloza C, et al. Widespread white matter microstructural differences in schizophrenia across 4322 individuals: results from the ENIGMA Schizophrenia DTI Working Group. Mol Psychiatry 2018;23:1261-9. [Crossref] [PubMed]
- Fernandez-Real JM, Serino M, Blasco G, Puig J, Daunis-i-Estadella J, Ricart W, Burcelin R, Fernández-Aranda F, Portero-Otin M. Gut Microbiota Interacts With Brain Microstructure and Function. J Clin Endocrinol Metab 2015;100:4505-13. [Crossref] [PubMed]
- Li S, Song J, Ke P, Kong L, Lei B, Zhou J, Huang Y, Li H, Li G, Chen J, Li X, Xiang Z, Ning Y, Wu F, Wu K. The gut microbiome is associated with brain structure and function in schizophrenia. Sci Rep 2021;11:9743. [Crossref] [PubMed]
- Chang YZ. Brain Iron Metabolism and CNS Diseases. Singapore: Springer Singapore, 2019.
- D'Mello SR, Kindy MC. Overdosing on iron: Elevated iron and degenerative brain disorders. Exp Biol Med (Maywood) 2020;245:1444-73. [Crossref] [PubMed]
- Eskreis-Winkler S, Zhang Y, Zhang J, Liu Z, Dimov A, Gupta A, Wang Y. The clinical utility of QSM: disease diagnosis, medical management, and surgical planning. NMR Biomed 2017; [Crossref] [PubMed]
- Wang F, Zhang M, Li Y, Li Y, Gong H, Li J, Zhang Y, Zhang C, Yan F, Sun B, He N, Wei H. Alterations in brain iron deposition with progression of late-life depression measured by magnetic resonance imaging (MRI)-based quantitative susceptibility mapping. Quant Imaging Med Surg 2022;12:3873-88. [Crossref] [PubMed]
- Wang C, Martins-Bach AB, Alfaro-Almagro F, Douaud G, Klein JC, Llera A, Fiscone C, Bowtell R, Elliott LT, Smith SM, Tendler BC, Miller KL. Phenotypic and genetic associations of quantitative magnetic susceptibility in UK Biobank brain imaging. Nat Neurosci 2022;25:818-31. [Crossref] [PubMed]
- Yang A, Du L, Gao W, Liu B, Chen Y, Wang Y, Liu X, Lv K, Zhang W, Xia H, Wu K, Ma G. Associations of cortical iron accumulation with cognition and cerebral atrophy in Alzheimer's disease. Quant Imaging Med Surg 2022;12:4570-86. [Crossref] [PubMed]
- Chavhan GB, Babyn PS, Thomas B, Shroff MM, Haacke EM. Principles, techniques, and applications of T2*-based MR imaging and its special applications. Radiographics 2009;29:1433-49. [Crossref] [PubMed]
- Blasco G, Moreno-Navarrete JM, Rivero M, Pérez-Brocal V, Garre-Olmo J, Puig J. Daunis-I-Estadella P, Biarnés C, Gich J, Fernández-Aranda F, Alberich-Bayarri Á, Moya A, Pedraza S, Ricart W, López M, Portero-Otin M, Fernandez-Real JM. The Gut Metagenome Changes in Parallel to Waist Circumference, Brain Iron Deposition, and Cognitive Function. J Clin Endocrinol Metab 2017;102:2962-73. [Crossref] [PubMed]
- Sekula P, Del Greco M F, Pattaro C, Köttgen A. Mendelian Randomization as an Approach to Assess Causality Using Observational Data. J Am Soc Nephrol 2016;27:3253-65. [Crossref] [PubMed]
- Long Y, Tang L, Zhou Y, Zhao S, Zhu H. Causal relationship between gut microbiota and cancers: a two-sample Mendelian randomisation study. BMC Med 2023;21:66. [Crossref] [PubMed]
- Birney E. Mendelian Randomization. Cold Spring Harb Perspect Med 2022;12:a041302. [PubMed]
- Wang LN, Zhang Z. Mendelian randomization approach, used for causal inferences. Zhonghua Liu Xing Bing Xue Za Zhi 2017;38:547-52. [PubMed]
- Davey Smith G, Hemani G. Mendelian randomization: genetic anchors for causal inference in epidemiological studies. Hum Mol Genet 2014;23:R89-98. [Crossref] [PubMed]
- Dehghan A. Genome-Wide Association Studies. Methods Mol Biol 2018;1793:37-49. [Crossref] [PubMed]
- Thomas H. Mendelian randomization reveals causal effects of the gut microbiota. Nat Rev Gastroenterol Hepatol 2019;16:198-9. [Crossref] [PubMed]
- Kurilshikov A, Medina-Gomez C, Bacigalupe R, Radjabzadeh D, Wang J, Demirkan A, et al. Large-scale association analyses identify host factors influencing human gut microbiome composition. Nat Genet 2021;53:156-65. [Crossref] [PubMed]
- Wang Q, Dai H, Hou T, Hou Y, Wang T, Lin H, Zhao Z, Li M, Zheng R, Wang S, Lu J, Xu Y, Liu R, Ning G, Wang W, Bi Y, Zheng J, Xu M. Dissecting Causal Relationships Between Gut Microbiota, Blood Metabolites, and Stroke: A Mendelian Randomization Study. J Stroke 2023;25:350-60. [Crossref] [PubMed]
- Topiwala A, Wang C, Ebmeier KP, Burgess S, Bell S, Levey DF, Zhou H, McCracken C, Roca-Fernández A, Petersen SE, Raman B, Husain M, Gelernter J, Miller KL, Smith SM, Nichols TE. Associations between moderate alcohol consumption, brain iron, and cognition in UK Biobank participants: Observational and mendelian randomization analyses. PLoS Med 2022;19:e1004039. [Crossref] [PubMed]
- Alfaro-Almagro F, Jenkinson M, Bangerter NK, Andersson JLR, Griffanti L, Douaud G, et al. Image processing and Quality Control for the first 10,000 brain imaging datasets from UK Biobank. Neuroimage 2018;166:400-24. [Crossref] [PubMed]
- Hernando D, Vigen KK, Shimakawa A, Reeder SB R. *(2) mapping in the presence of macroscopic B0 field variations. Magn Reson Med 2012;68:830-40. [Crossref] [PubMed]
- Patenaude B, Smith SM, Kennedy DN, Jenkinson M. A Bayesian model of shape and appearance for subcortical brain segmentation. Neuroimage 2011;56:907-22. [Crossref] [PubMed]
- Pauli WM, Nili AN, Tyszka JM. A high-resolution probabilistic in vivo atlas of human subcortical brain nuclei. Sci Data 2018;5:180063. [Crossref] [PubMed]
- Griffanti L, Zamboni G, Khan A, Li L, Bonifacio G, Sundaresan V, Schulz UG, Kuker W, Battaglini M, Rothwell PM, Jenkinson M. BIANCA (Brain Intensity AbNormality Classification Algorithm): A new tool for automated segmentation of white matter hyperintensities. Neuroimage 2016;141:191-205. [Crossref] [PubMed]
- Wang YZ, Shen HB. Challenges and factors that influencing causal inference and interpretation, based on Mendelian randomization studies. Zhonghua Liu Xing Bing Xue Za Zhi 2020;41:1231-6. [PubMed]
- Chen J, Xu T, Wu M. Depression in systemic lupus erythematosus: Modifiable or inheritable? a two-sample mendelian randomization study. Front Genet 2022;13:988022. [Crossref] [PubMed]
- Bowden J, Holmes MV. Meta-analysis and Mendelian randomization: A review. Res Synth Methods 2019;10:486-96. [Crossref] [PubMed]
- Verbanck M, Chen CY, Neale B, Do R. Detection of widespread horizontal pleiotropy in causal relationships inferred from Mendelian randomization between complex traits and diseases. Nat Genet 2018;50:693-8. [Crossref] [PubMed]
- Boehm FJ, Zhou X. Statistical methods for Mendelian randomization in genome-wide association studies: A review. Comput Struct Biotechnol J 2022;20:2338-51. [Crossref] [PubMed]
- Ahmed H, Leyrolle Q, Koistinen V, Kärkkäinen O, Layé S, Delzenne N, Hanhineva K. Microbiota-derived metabolites as drivers of gut-brain communication. Gut Microbes 2022;14:2102878. [Crossref] [PubMed]
- Ma XZ, Chen LL, Qu L, Li H, Wang J, Song N, Xie JX. Gut microbiota-induced CXCL1 elevation triggers early neuroinflammation in the substantia nigra of Parkinsonian mice. Acta Pharmacol Sin 2024;45:52-65. [Crossref] [PubMed]
- Kwon D, Zhang K, Paul KC, Folle AD, Del Rosario I, Jacobs JP, Keener AM, Bronstein JM, Ritz B. Diet and the gut microbiome in patients with Parkinson's disease. NPJ Parkinsons Dis 2024;10:89. [Crossref] [PubMed]
- Tansey MG, Wallings RL, Houser MC, Herrick MK, Keating CE, Joers V. Inflammation and immune dysfunction in Parkinson disease. Nat Rev Immunol 2022;22:657-73. [Crossref] [PubMed]
- Horton MK, McCauley K, Fadrosh D, Fujimura K, Graves J, Ness J, et al. Gut microbiome is associated with multiple sclerosis activity in children. Ann Clin Transl Neurol 2021;8:1867-83. [Crossref] [PubMed]
- Stoeva MK, Garcia-So J, Justice N, Myers J, Tyagi S, Nemchek M, McMurdie PJ, Kolterman O, Eid J. Butyrate-producing human gut symbiont, Clostridium butyricum, and its role in health and disease. Gut Microbes 2021;13:1-28. [Crossref] [PubMed]
- Silva PF, Garcia VA, Dornelles Ada S, Silva VK, Maurmann N, Portal BC, Ferreira RD, Piazza FC, Roesler R, Schröder N. Memory impairment induced by brain iron overload is accompanied by reduced H3K9 acetylation and ameliorated by sodium butyrate. Neuroscience 2012;200:42-9. [Crossref] [PubMed]
- Zha Z, Lv Y, Tang H, Li T, Miao Y, Cheng J, Wang G, Tan Y, Zhu Y, Xing X, Ding K, Wang Y, Yin H. An orally administered butyrate-releasing xylan derivative reduces inflammation in dextran sulphate sodium-induced murine colitis. Int J Biol Macromol 2020;156:1217-33. [Crossref] [PubMed]
- Mentzelou M, Dakanalis A, Vasios GK, Gialeli M, Papadopoulou SK, Giaginis C. The Relationship of Ketogenic Diet with Neurodegenerative and Psychiatric Diseases: A Scoping Review from Basic Research to Clinical Practice. Nutrients 2023;15:2270. [Crossref] [PubMed]
- Jiang Z, Wang X, Zhang H, Yin J, Zhao P, Yin Q, Wang Z. Ketogenic diet protects MPTP-induced mouse model of Parkinson's disease via altering gut microbiota and metabolites. MedComm (2020) 2023;4:e268.
- Bordoni L, Gabbianelli R, Fedeli D, Fiorini D, Bergheim I, Jin CJ, Marinelli L, Di Stefano A, Nasuti C. Positive effect of an electrolyzed reduced water on gut permeability, fecal microbiota and liver in an animal model of Parkinson's disease. PLoS One 2019;14:e0223238. [Crossref] [PubMed]
- Nazarova L, Liu H, Xie H, Wang L, Ding H, An H, Huang D. Targeting gut-brain axis through scalp-abdominal electroacupuncture in Parkinson's disease. Brain Res 2022;1790:147956. [Crossref] [PubMed]
- Wang JY, Zhuang QQ, Zhu LB, Zhu H, Li T, Li R, Chen SF, Huang CP, Zhang X, Zhu JH. Meta-analysis of brain iron levels of Parkinson's disease patients determined by postmortem and MRI measurements. Sci Rep 2016;6:36669. [Crossref] [PubMed]
- Kumar H, Lim HW, More SV, Kim BW, Koppula S, Kim IS, Choi DK. The role of free radicals in the aging brain and Parkinson's Disease: convergence and parallelism. Int J Mol Sci 2012;13:10478-504. [Crossref] [PubMed]
- Cuesta MJ, Lecumberri P, Moreno-Izco L, López-Ilundain JM, Ribeiro M, Cabada T, Lorente-Omeñaca R, de Erausquin G, García-Martí G, Sanjuan J, Sánchez-Torres AM, Gómez M, Peralta V. Motor abnormalities and basal ganglia in first-episode psychosis (FEP). Psychol Med 2021;51:1625-36. [Crossref] [PubMed]
- Wang X, Zhu Z, Sun J, Jia L, Cai L, Chen Q, Yang W, Wang Y, Zhang Y, Guo S, Liu W, Yang Z, Zhao P, Wang Z, Lv H. Changes in iron load in specific brain areas lead to neurodegenerative diseases of the central nervous system. Prog Neuropsychopharmacol Biol Psychiatry 2024;129:110903. [Crossref] [PubMed]
- Liu H, Deng B, Xie F, Yang X, Xie Z, Chen Y, Yang Z, Huang X, Zhu S, Wang Q. The influence of white matter hyperintensity on cognitive impairment in Parkinson's disease. Ann Clin Transl Neurol 2021;8:1917-34. [Crossref] [PubMed]
- Carvalho de Abreu DC, Pieruccini-Faria F, Son S, Montero-Odasso M, Camicioli R. Is white matter hyperintensity burden associated with cognitive and motor impairment in patients with parkinson's disease? A systematic review and meta-analysis. Neurosci Biobehav Rev 2024;161:105677. [Crossref] [PubMed]
- Wu H, Hong H, Wu C, Qin J, Zhou C, Tan S. DuanMu X, Guan X, Bai X, Guo T, Wu J, Chen J, Wen J, Cao Z, Gao T, Gu L, Huang P, Xu X, Zhang B, Zhang M. Regional white matter hyperintensity volume in Parkinson's disease and associations with the motor signs. Ann Clin Transl Neurol 2023;10:1502-12. [Crossref] [PubMed]
- Scamarcia PG, Agosta F, Spinelli EG, Basaia S, Stojković T, Stankovic I, Sarasso E, Canu E, Markovic V, Petrović I, Stefanova E, Pagani E, Kostic VS, Filippi M. Longitudinal White Matter Damage Evolution in Parkinson's Disease. Mov Disord 2022;37:315-24. [Crossref] [PubMed]
- Fan HX, Sheng S, Zhang F. New hope for Parkinson's disease treatment: Targeting gut microbiota. CNS Neurosci Ther 2022;28:1675-88. [Crossref] [PubMed]
- Murros KE. Hydrogen Sulfide Produced by Gut Bacteria May Induce Parkinson's Disease. Cells 2022;11:978. [Crossref] [PubMed]
- Nie S, Jing Z, Wang J, Deng Y, Zhang Y, Ye Z, Ge Y. The link between increased Desulfovibrio and disease severity in Parkinson's disease. Appl Microbiol Biotechnol 2023;107:3033-45. [Crossref] [PubMed]
- Uchida Y, Kan H, Sakurai K, Inui S, Kobayashi S, Akagawa Y, Shibuya K, Ueki Y, Matsukawa N. Magnetic Susceptibility Associates With Dopaminergic Deficits and Cognition in Parkinson's Disease. Mov Disord 2020;35:1396-405. [Crossref] [PubMed]
- Fan J, Zhou Y, Meng R, Tang J, Zhu J, Aldrich MC, Cox NJ, Zhu Y, Li Y, Zhou D. Cross-talks between gut microbiota and tobacco smoking: a two-sample Mendelian randomization study. BMC Med 2023;21:163. [Crossref] [PubMed]
- Li Z, Chen Y, Ke H. Investigating the Causal Relationship Between Gut Microbiota and Crohn's Disease: A Mendelian Randomization Study. Gastroenterology 2024;166:354-55. [Crossref] [PubMed]