Research trends of artificial intelligence and radiomics in lung cancer: a bibliometric analysis
Introduction
Lung cancer was the leading cause of worldwide cancer-related mortality, according to the Global Cancer Statistics 2022 report (1). Lung cancer has the highest proportion (20.4%) of all cancers in China, and a mortality rate of 27.2% (2). Due to its prevalence and mortality rates, lung cancer has had a great impact on the worldwide burden (3). To reduce lung cancer mortality, the National Lung Screening Trial was conducted by low-dose computed tomography (CT) screening (4); however, conventional CT still has limitations. Notably, conventional CT screening relies on the manual reading of many CT images and data for diagnosis, which is laborious, inefficient, and prone to omission and misdiagnosis in clinical practice; thus, more accurate lung cancer diagnosis methods need to be established (5).
In 2012, Lambin et al. proposed the radiomics to extract high-throughput image features from medical images (6). The steps of radiomics mainly include image acquisition, lesion segmentation, feature extraction, and model construction (7). With the increase in the number of studies in recent years, radiomics has become a potentially powerful tool for non-invasive precision medicine (8).
Due to the rapid development of advanced artificial intelligence (AI), technologies of radiomics have advanced continually (9,10). As a branch of computer science (11), AI primarily includes machine learning (ML), such as classical support vector machines, and emerging technologies, such as deep learning (DL) (12). DL, as a subtype of ML, processes data using multilayer network algorithms and can extract deeper imaging features, significantly enhancing the performance of predictive models compared to traditional radiomics approaches (10,12,13). In recent years, the integration of AI and radiomics has seen continued growth, achieving effective applications in the detection, diagnosis, and prognosis prediction of lung cancer and lung nodules (14-17).
Retrospective reviews of the field have been conducted; however, most have only focused on the technical process of AI and radiomics, and their application in lung cancer (18-20). Thus, research needs to be conducted to show the current status, hotspots, and trends in the field, and to provide guidance on research directions. Compared with a systematic review, a bibliometric analysis is more extensive, and includes all relevant literature in a field (21-23). It also quantitatively analyzes the data, which can help to identify trends and provide unique insights into research trends and collaborations in the field (21-23).
Therefore, this study aimed to use a bibliometric method to comprehensively investigate and identify research trends in the field of AI and radiomics in lung cancer. It comprehensively analyzed the current research status of the field in terms of countries/regions, institutions, authors, journals and keywords, assessed the quality of the existing research, and explored potential research directions. We present this article in accordance with the BIBLIO reporting checklist (24) (available at https://qims.amegroups.com/article/view/10.21037/qims-24-1316/rc).
Methods
Data source and retrieval strategies
A search was conducted of the Web of Science Core Collection (WoSCC) to retrieve all relevant publications on the application of AI and radiomics in lung cancer; no search time period was set. The search strategy was mainly related to AI, ML, DL, radiomics, lung cancer, and CT images. Details about the search strategy are provided in Appendix 1. Non-English literature and other types of literature, such as conference abstracts, newspapers, news, books, and book chapters, were excluded; only articles or reviews were included. To minimize subjective bias, two independent readers reviewed the titles and abstracts, and any disagreements were resolved through discussion with the third reviewer with seven years of radiology experience. Using the BIBLIO checklists, three authors evaluated the quality of the included publications. Figure 1 shows the study protocol from the initial search strategy to the final studies included in the analysis.
Data collection
The search period ended on May 19, 2024, and all downloads were completed on the same day to prevent the daily update of the database from distorting the measurement results. The following data were extracted: abstract, publishing year, journal, country/region, institution, author, keywords, type of literature, and literature citations. The full record of the search results and the cited references were exported directly in plain text file. In addition, Journal Citation Reports 2023 and journal websites were used to obtain details of each journal’s country/region and impact factor (IF). The data were downloaded directly from the database, and as the study did not include any data on human or animal subjects, ethical approval was not required.
Analysis method
This study used CiteSpace (version 6.3.R2, Advanced) to process the filtered records exported by the WoSCC (25). These records were imported into CiteSpace to construct institution, author, and keyword visualization maps. The time slice was set from “2008–2024”, and all other parameters were set as the default values. After examining the original map, manual adjustments were made to the position and size of the nodes and labels. CiteSpace was also used to perform a burst analysis of keywords; the top 45 keywords with the strongest citation bursts were identified to analyze the research hotspots and development trends in this field. In this study, a reference timeline view was generated by CiteSpace, which explores a particular discipline’s development and evolutionary dynamics.
Bibliometrix, a software package built with R software (version 4.4.0) for bibliometric analysis, was used in this study to undertake analyses of the number of publications by country/region of the corresponding author, the number of citations by country/region, and the number of publications by journal (26).
In addition, the relevant data analysis results were derived using CiteSpace and Bibliometrix. R software (version 4.4.0) was used to draw figures and statistical charts to analyze the basic characteristic data for the country/region, institution, author, and journal.
Results
Study selection and overview
As of May 19, 2024, 5,632 publications were retrieved from the WoSCC. A total of 2,989 publications were included in the bibliometric analysis, of which 2,804 were articles (93.8%) and 185 were reviews (6.2%). The general metrics and overview of the included literature are provided in Table 1.
Table 1
Metric | Value |
---|---|
Document types | |
Article | 2,804 |
Review | 185 |
Main information | |
Timespan of publications | 2008–2024 |
Number of publications included | 2,989 |
Number of citations | 67,570 |
Average annual number of citations | 3,974.7 |
Country/region | 61 |
Institution | 485 |
Author | 689 |
Keywords | 639 |
Annual publication
Based on the analysis results of Bibliometrix, a table of the number of publications issued per year was produced (Table 2). The number of publications did not change significantly in the first eight years. The number of publications showed rapid increase from 2016, and despite a decrease in the annual growth rate in recent years, the literature in the field continues to grow.
Table 2
Year | Number of publications |
---|---|
2008 | 15 |
2009 | 20 |
2010 | 25 |
2011 | 17 |
2012 | 20 |
2013 | 31 |
2014 | 31 |
2015 | 31 |
2016 | 66 |
2017 | 102 |
2018 | 174 |
2019 | 263 |
2020 | 404 |
2021 | 465 |
2022 | 582 |
2023 | 559 |
2024 | 184 |
Distribution by country/region
According to the Bibliometrix statistics and the analysis of the corresponding authors’ country/region information (Figure 2A), most publications came from China (n=1,365, 45.7%), followed by the United States of America (USA) (n=393, 13.1%), India (n=235, 7.9%), South Korea (n=147, 4.9%), and Japan (n=119, 4.0%). As Figure 2B shows, Ireland had the highest average number of citations, far exceeding that of other countries/regions, while European countries/regions produced fewer publications but tended to have more citations. The USA ranked second in terms of the number of published publications, and fourth in terms of the average citation count. Detailed data on the top 40 countries/regions for publication volume and citation averages can be found in Tables S1,S2, respectively.
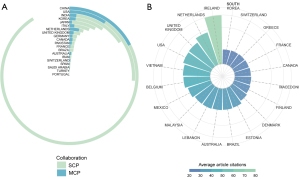
Distribution by institution
The visualization map of institutions (Figure 3A) showed 485 nodes and 2,282 lines, indicating that 485 institutions had contributed to the field. Among all institutions, Fudan University had the highest number of publications (n=109, 3.6%), followed by Shanghai Jiao Tong University (n=105, 3.5%), Chinese Academy of Sciences (n=97, 3.2%), and General Electric (n=75, 2.5%). The nodes of Harvard University (centrality =0.14), Harvard Medical School (centrality =0.11), National Yunlin University Science and Technology (centrality =0.11), and Islamic Azad University (centrality =0.11) had purple circles, indicating their important role as bridging institutions. The centrality of the 20 most productive institutions is shown in Figure 3B. The frequency of the 40 most published institutions and their centrality is shown in Table S3.
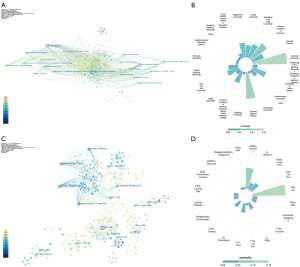
Analysis of authors
CiteSpace was used to analyze the relevant information of the authors to obtain the visualization map (Figure 3C), which showed 689 nodes and 1,562 lines. Gillies published the most publications, participating in the publication of 28 of the included publications (0.9%), followed by Li (27 publications, 0.9%), Aerts (26 publications, 0.9%), Lee (21 publications, 0.7%), and Tian (21 publications, 0.7%). It is also evident that some of the authors worked very closely with each other, as displayed by the multiple groups in the figure. Figure 3D shows the centrality of the 20 authors with the most publications. Tian had the strongest centrality (centrality =0.14), indicating his important role in this field. In addition, this study analyzed the authors in terms of co-citations and ranked the top 40 authors (Table S4).
Distribution by journal
A statistical analysis of the top 30 journals in terms of the number of publications was conducted to explore the relationship among IF (2023), number of publications, and total number of citations of the journals (Table S5), and a bubble diagram was drawn (Figure 4). Eight journals were found to have published more than 50 relevant publications. Frontiers in Oncology contributed the most publications (n=177, 5.9%), followed by Medical Physics (n=109, 3.6%), European Radiology (n=92, 3.1%), Scientific Reports (n=89, 3.0%), and Cancers (n=78, 2.6%). Among the top 30 journals with the highest number of publications, Radiology (IF =12.1) had the highest IF. Among them, 10 journals were from the USA. In addition, the two most cited journals were also American; that is, Radiology (5,152 citations) and Medical Physics (3,487 citations).
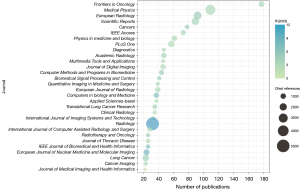
Analysis of co-cited references
From 2008 to 2024, the total number of citations was 67,570, and the average annual number of citations was 3,974.7. As Table 3 shows, this study ranked the top 20 cited literature results and manually obtained the country/region and IF of the corresponding journals. Only four publications were cited more than 500 times, while the others were cited between 270 and 500 times. The most cited article titled “End-to-End Lung Cancer Screening with Three-Dimensional Deep Learning on Low-Dose Chest Computed Tomography” (922 citations), which was published in Nature Medicine, was also the most cited article on average per year (27).
Table 3
Rank | Total citations | Citations per annual | Year | Title of publication | Journal | ||
---|---|---|---|---|---|---|---|
Name | Country/region | IF (2023) | |||||
1 | 922 | 153.67 | 2019 | End-to-end lung cancer screening with three-dimensional deep learning on low-dose chest computed tomography | Nature Medicine | USA | 58.7 |
2 | 783 | 87 | 2016 | Pulmonary nodule detection in CT images: false positive reduction using multi-view convolutional networks | IEEE Transactions on Medical Imaging | USA | 8.9 |
3 | 635 | 63.5 | 2015 | Machine-learning methods for quantitative radiomic biomarkers | Scientific Reports | England | 3.8 |
4 | 507 | 50.7 | 2015 | CT-based radiomic signature predicts distant metastasis in lung adenocarcinoma | Radiotherapy and Oncology | Netherlands | 4.9 |
5 | 456 | 45.6 | 2015 | Measuring computed tomography scanner variability of radiomics features | Investigative Radiology | USA | 7.0 |
6 | 432 | 39.27 | 2014 | Robust radiomics feature quantification using semiautomatic volumetric segmentation | PLoS One | USA | 2.9 |
7 | 404 | 31.08 | 2012 | Tumour heterogeneity in non-small cell lung carcinoma assessed by CT texture analysis: a potential marker of survival | European Radiology | Austria | 4.7 |
8 | 392 | 49 | 2017 | Multi-crop convolutional neural networks for lung nodule malignancy suspiciousness classification | Pattern Recognition | England | 7.5 |
9 | 388 | 48.5 | 2017 | Intrinsic dependencies of CT radiomic features on voxel size and number of gray levels | Medical Physics | USA | 3.2 |
10 | 359 | 29.92 | 2013 | Non-small cell lung cancer: histopathologic correlates for texture parameters at CT | Radiology | USA | 12.1 |
11 | 328 | 32.8 | 2015 | Computer-aided classification of lung nodules on computed tomography images via deep learning technique | Oncotargets and Therapy | England | 2.7 |
12 | 326 | 46.57 | 2018 | Deep learning for lung cancer prognostication: a retrospective multi-cohort radiomics study | PLoS Medicine | USA | 10.5 |
13 | 326 | 27.17 | 2013 | Are pretreatment 18f-FDG-PET tumor textural features in non-small cell lung cancer associated with response and survival after chemoradiotherapy? | Journal of Nuclear Medicine | USA | 9.1 |
14 | 323 | 26.92 | 2013 | Stability of FDG-PET radiomics features: an integrated analysis of test-retest and inter-observer variability | Acta Oncologica | England | 2.7 |
15 | 314 | 44.86 | 2018 | Radiomics and radiogenomics in lung cancer: a review for the clinician | Lung Cancer | Netherlands | 4.5 |
16 | 313 | 52.17 | 2019 | Development and validation of deep learning-based automatic detection algorithm for malignant pulmonary nodules on chest radiographs | Radiology | USA | 12.1 |
17 | 299 | 37.38 | 2017 | Central focused convolutional neural networks: Developing a data-driven model for lung nodule segmentation | Medical Image Analysis | Netherlands | 10.7 |
18 | 282 | 47 | 2019 | Deep learning predicts lung cancer treatment response from serial medical imaging | Clinical Cancer Research | USA | 10.0 |
19 | 271 | 33.88 | 2017 | Somatic mutations drive distinct imaging phenotypes in lung cancer | Cancer Research | USA | 12.5 |
20 | 271 | 30.11 | 2016 | Exploratory study to identify radiomics classifiers for lung cancer histology | Frontiers in Oncology | Switzerland | 3.5 |
CT, computed tomography; 18F-FDG-PET, 18F-fluorodeoxyglucose positron emission tomography; IF, impact factor.
A timeline visualization analysis of the co-cited references was also performed (Figure 5). The network had a total of 1,167 nodes and 6,327 lines, forming eight clusters, as follows: “radiomics”, “non-small cell lung cancer”, “pulmonary nodule detection”, “deep learning”, “medical image”, “artificial intelligence”, “computerized tomography”, and “texture extraction”.
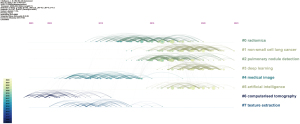
Analysis of keyword co-occurrence clusters
To intuitively show the research hotspots and trends, the keywords were analyzed. The results of the co-occurrence keyword network showed that there were 639 nodes and 2,817 connections (Figure 6A). In terms of frequency, “lung cancer” ranked first, with 824 occurrences, followed by “deep learning”, “classification”, “cancer”, “computed tomography”, “pulmonary nodules”, “images”, “CT”, and “features”. Detailed data on the top 40 keywords and the centrality is provided in Table S6. In addition, we also performed a cluster analysis of the keywords (Figure 6B) and obtained five cluster results, from #0 to #4 as follows: “radiomics”, “non-small cell lung cancer”, “epidermal growth factor receptor”, “lung adenocarcinoma”, and “machine learning”.
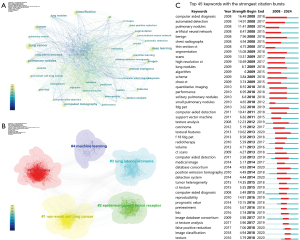
The top 45 keywords with the strongest burst sorted by time of appearance were determined, and the keyword burst plot showed that computer-aided diagnosis had the strongest burst intensity. In addition, “texture”, “image classification”, and “false positive reduction” have recently emerged on the frontier of research (Figure 6C).
Discussion
This study conducted a comprehensive bibliometric analysis on the application of AI and radiomics in lung cancer based on 2,989 publications. In 2016, this field entered a phase of rapid development. This might be due to a milestone study published online in 2015 titled “Radiomics: Images Are More than Pictures, They Are Data” (28), which led to a rapid acceleration in research into radiomics. Gillies, the first author of this milestone article, was also the author with the highest number of publications in the author analysis of this field. Additionally, Alpha Dog’s 2016 victory over the Go World Champion Lee (29), sparked significant research interest in AI. Despite a slowdown in the annual growth of publications in recent years, AI and radiomics remain a vital frontier of research for the future diagnosis and treatment of lung cancer patients.
In terms of impact, European and American countries/regions have higher citation rates for literature in this field, of research typically published in journals with higher IFs, and generally have a higher level of international scientific standing. According to previous studies (1), Eastern Asia has the highest age-standardized incidence rates of lung cancer, and this region also has the highest number of publications. In part, the severity of lung cancer may have driven each country’s/region’s research in this field. The qualitative leadership of the USA in this field might be due to the larger size of its economy and its greater investment in scientific research (30). However, the influence of Chinese research is generally not very high, as such, relevant Chinese researchers may need to seek to improve the novelty and relevance of their research. Additionally, the study revealed that research involving collaborations between different countries/regions and institutions generally exhibited a higher IF among the 2,989 studies analyzed (31-33). It may be that collaborations between different countries/regions and institutions lead to discussions about research methods, bridge gaps in thinking, and result in the sharing of multicenter data and some AI techniques, which in turn lead to improved reliability and quality, and a greater likelihood of the publications being accepted by high IF journals; however, the long-term value of these studies needs to be examined over time.
Based on the clustered timeline map of the co-cited literature and the keyword analysis, non-small cell lung cancer (NSCLC) is a major focus of research in this field. This may be because NSCLC is more prevalent than small cell lung cancer, and accounts for the majority of lung cancers (34), and thus also accounts for a much larger proportion of clinical requirement. Review results from Evangelista et al. on patients with lung cancer also showed that 12 of the 15 publications included in their analysis were studies on NSCLC (35). NSCLC has been a major hotspot in the field over the past years, and might be a continuing hotspot. Relevant researchers could improve clinical studies of NSCLC with AI and radiomics. As small cell lung cancer accounts for a relatively small proportion of lung cancer cases, research in this field is limited, making it a potential direction for future studies.
The keyword clustering results suggested that the epidermal growth factor receptor (EGFR) may also be a research hotspot in the field of lung cancer. The EGFR gene is regarded as a proto-oncogene, and mutations in this gene cause abnormal cell proliferation, which is associated with the pathogenesis of lung cancer (36,37). These findings have led to multiple studies being conducted in this area, in recent years, some studies aimed to use non-invasive radiomics to identify EGFR gene mutations for predicting the survival and treatment responses of lung cancer patients (38-40). Studies seeking to elucidate the relationship between genes and radiomics have been termed, radiogenomics (41). In addition to radiogenomics, recent attempts have been made to combine radiomics with pathomics and transcriptomics with successful results (42-44). The idea of multi-omics is gradually coming into view, which may be an emerging field and a novel development trend (45). Multi-omics may achieve better results, which in turn could promote the development and integration of diagnostic and treatment modalities for lung cancer in the clinic in the future.
The time visualization maps and keyword analysis of the co-cited publications showed that AI is important. Recently, DL has emerged as a valuable strategy in radiomics, and many advanced DL frameworks have been established, including the U-Net and transformer models (46-49). Continuous innovations in DL technologies promote the continuous progress of radiomics in lung cancer. Scholars in this field should pay more attention to the development of DL in the engineering field, and learn more interdisciplinary medicine and engineering skills to achieve leading research results in this field.
As evident from the clustered timeline map of the co-cited literature and the keyword analysis, texture of imaging is a prominent topic. Feature extraction is a step in radiomics, but it still faces many challenges related to its standardization. Currently, DL features are not interpretable, and the internal algorithms and decision-making processes of DL, which are often referred to as “black boxes”, raise questions about the results (7). The interpretability of DL could become a direction for further exploration of the field (50). In addition, the Image Biomarker Standardization Initiative proposed in 2020 also noted that the current process of feature extraction is not standardized (51). In the future, related research in this field could be based on the Image Biomarker Standardization Initiative for feature extraction and model training to increase the reproducibility of models, thus enhancing their clinical value.
In recent years, a limited number of bibliometric studies have been published in similar fields. These research topics include one or both of AI, radiomics, and cancer or lung cancer. Consistent with our findings, these studies showed rapid growth, beginning around 2016, with China and the USA leading in terms of publication volume (23,52-55). Similar to our results, “deep learning” appeared as a frequent keyword across most of these articles, with authors like Karger et al. and Liu et al. advocating for improved reproducibility and interpretability in DL research, and Liang et al. emphasizing the need for further integration of DL and radiomics in the field of radiological imaging for lung cancer (23,52-55). Further, in the studies of Volpe et al. and Zhong et al., “radiogenomics”, “immunotherapy”, and other multi-omics applications have emerged as research hotspots in recent years (53,54). These trends align with our study’s recognition of radiogenomics and multi-omics as promising future directions. Additionally, “textural feature” is another significant keyword in the work of Liang et al. and Liu et al., emphasizing the importance of feature extraction in radiomics, which also aligns with our findings (23,55).
This study had several limitations. First, as it is difficult for bibliometric visualization software to merge literature from different database sources, this study only included literature from the WoSCC, and searches of other databases were not conducted. Second, excluding non-English literature might have influenced the results to some extent, but we believe that the results remain reliable, given that non-English literature comprises only a small percentage of the literature. Additionally, the manual screening of the retrieved studies introduces the potential of bias by the screeners. Finally, this search ended on May 19, 2024, but research in this field is constantly and dynamically updated, which might have led to the omission of newly emerging hot topics.
Conclusions
This study employed a bibliometric method to analyze the global distribution and citations of literature across various countries/regions, authors, and institutions. It also analyzed the specific content of the literature, such as the keyword co-occurrence networks. It highlighted future research directions in the field, including new AI techniques, EGFR mutations, and standardized feature extraction, while emphasizing the importance of increased collaboration between regions and institutions. Our findings may provide valuable insights for researchers and organizations into the current state of the field, and help them to identify key areas for future research and support.
Acknowledgments
The authors would like to thank the team at CiteSpace, which was led by Professor Chaomei Chen, for providing technical support.
Funding: This research was supported by
Footnote
Reporting Checklist: The authors have completed the BIBLIO reporting checklist. Available at https://qims.amegroups.com/article/view/10.21037/qims-24-1316/rc
Conflicts of Interest: All authors have completed the ICMJE uniform disclosure form (available at https://qims.amegroups.com/article/view/10.21037/qims-24-1316/coif). The authors have no conflicts of interest to declare.
Ethical Statement: The authors are accountable for all aspects of the work in ensuring that questions related to the accuracy or integrity of any part of the work are appropriately investigated and resolved.
Open Access Statement: This is an Open Access article distributed in accordance with the Creative Commons Attribution-NonCommercial-NoDerivs 4.0 International License (CC BY-NC-ND 4.0), which permits the non-commercial replication and distribution of the article with the strict proviso that no changes or edits are made and the original work is properly cited (including links to both the formal publication through the relevant DOI and the license). See: https://creativecommons.org/licenses/by-nc-nd/4.0/.
References
- Bray F, Laversanne M, Sung H, Ferlay J, Siegel RL, Soerjomataram I, Jemal A. Global cancer statistics 2022: GLOBOCAN estimates of incidence and mortality worldwide for 36 cancers in 185 countries. CA Cancer J Clin 2024;74:229-63. [Crossref] [PubMed]
- Maomao C, He L, Dianqin S, Siyi H, Xinxin Y, Fan Y, Shaoli Z, Changfa X, Lin L, Ji P, Wanqing C. Current cancer burden in China: epidemiology, etiology, and prevention. Cancer Biol Med 2022;19:1121-38. [Crossref] [PubMed]
- Qi J, Li M, Wang L, Hu Y, Liu W, Long Z, Zhou Z, Yin P, Zhou M. National and subnational trends in cancer burden in China, 2005-20: an analysis of national mortality surveillance data. Lancet Public Health 2023;8:e943-55. [Crossref] [PubMed]
- National Lung Screening Trial Research Team. Aberle DR, Adams AM, Berg CD, Black WC, Clapp JD, Fagerstrom RM, Gareen IF, Gatsonis C, Marcus PM, Sicks JD. Reduced lung-cancer mortality with low-dose computed tomographic screening. N Engl J Med 2011;365:395-409. [Crossref] [PubMed]
- Lan CC, Hsieh MS, Hsiao JK, Wu CW, Yang HH, Chen Y, Hsieh PC, Tzeng IS, Wu YK. Deep Learning-based Artificial Intelligence Improves Accuracy of Error-prone Lung Nodules. Int J Med Sci 2022;19:490-8. [Crossref] [PubMed]
- Lambin P, Rios-Velazquez E, Leijenaar R, Carvalho S, van Stiphout RG, Granton P, Zegers CM, Gillies R, Boellard R, Dekker A, Aerts HJ. Radiomics: extracting more information from medical images using advanced feature analysis. Eur J Cancer 2012;48:441-6. [Crossref] [PubMed]
- Wu G, Jochems A, Refaee T, Ibrahim A, Yan C, Sanduleanu S, Woodruff HC, Lambin P. Structural and functional radiomics for lung cancer. Eur J Nucl Med Mol Imaging 2021;48:3961-74. [Crossref] [PubMed]
- Lambin P, Leijenaar RTH, Deist TM, Peerlings J, de Jong EEC, van Timmeren J, Sanduleanu S, Larue RTHM, Even AJG, Jochems A, van Wijk Y, Woodruff H, van Soest J, Lustberg T, Roelofs E, van Elmpt W, Dekker A, Mottaghy FM, Wildberger JE, Walsh S. Radiomics: the bridge between medical imaging and personalized medicine. Nat Rev Clin Oncol 2017;14:749-62. [Crossref] [PubMed]
- Tunali I, Gillies RJ, Schabath MB. Application of Radiomics and Artificial Intelligence for Lung Cancer Precision Medicine. Cold Spring Harb Perspect Med 2021;11:a039537. [Crossref] [PubMed]
- Yip SS, Aerts HJ. Applications and limitations of radiomics. Phys Med Biol 2016;61:R150-66. [Crossref] [PubMed]
- Hosny A, Parmar C, Quackenbush J, Schwartz LH, Aerts HJWL. Artificial intelligence in radiology. Nat Rev Cancer 2018;18:500-10. [Crossref] [PubMed]
- Jiang F, Jiang Y, Zhi H, Dong Y, Li H, Ma S, Wang Y, Dong Q, Shen H, Wang Y. Artificial intelligence in healthcare: past, present and future. Stroke Vasc Neurol 2017;2:230-43. [Crossref] [PubMed]
- Guiot J, Vaidyanathan A, Deprez L, Zerka F, Danthine D, Frix AN, Lambin P, Bottari F, Tsoutzidis N, Miraglio B, Walsh S, Vos W, Hustinx R, Ferreira M, Lovinfosse P, Leijenaar RTH. A review in radiomics: Making personalized medicine a reality via routine imaging. Med Res Rev 2022;42:426-40. [Crossref] [PubMed]
- Chetan MR, Gleeson FV. Radiomics in predicting treatment response in non-small-cell lung cancer: current status, challenges and future perspectives. Eur Radiol 2021;31:1049-58. [Crossref] [PubMed]
- Binczyk F, Prazuch W, Bozek P, Polanska J. Radiomics and artificial intelligence in lung cancer screening. Transl Lung Cancer Res 2021;10:1186-99. [Crossref] [PubMed]
- Yang L, Cai H, Luo X, Wu J, Tang R, Chen Y, Li W. A lightweight neural network for lung nodule detection based on improved ghost module. Quant Imaging Med Surg 2023;13:4205-21. [Crossref] [PubMed]
- Ren H, Xiao Z, Ling C, Wang J, Wu S, Zeng Y, Li P. Development of a novel nomogram-based model incorporating 3D radiomic signatures and lung CT radiological features for differentiating invasive adenocarcinoma from adenocarcinoma in situ and minimally invasive adenocarcinoma. Quant Imaging Med Surg 2023;13:237-48. [Crossref] [PubMed]
- Wilson R, Devaraj A. Radiomics of pulmonary nodules and lung cancer. Transl Lung Cancer Res 2017;6:86-91. [Crossref] [PubMed]
- Ladbury C, Amini A, Govindarajan A, Mambetsariev I, Raz DJ, Massarelli E, Williams T, Rodin A, Salgia R. Integration of artificial intelligence in lung cancer: Rise of the machine. Cell Rep Med 2023;4:100933. [Crossref] [PubMed]
- Chen M, Copley SJ, Viola P, Lu H, Aboagye EO. Radiomics and artificial intelligence for precision medicine in lung cancer treatment. Semin Cancer Biol 2023;93:97-113. [Crossref] [PubMed]
- Lu C, Liu M, Shang W, Yuan Y, Li M, Deng X, Li H, Yang K. Knowledge Mapping of Angelica sinensis (Oliv.) Diels (Danggui) Research: A Scientometric Study. Front Pharmacol 2020;11:294. [Crossref] [PubMed]
- Ke L, Lu C, Shen R, Lu T, Ma B, Hua Y. Knowledge Mapping of Drug-Induced Liver Injury: A Scientometric Investigation (2010-2019). Front Pharmacol 2020;11:842. [Crossref] [PubMed]
- Liu X, Hu X, Yu X, Li P, Gu C, Liu G, Wu Y, Li D, Wang P, Cai J. Frontiers and hotspots of (18)F-FDG PET/CT radiomics: A bibliometric analysis of the published literature. Front Oncol 2022;12:965773. [Crossref] [PubMed]
- Montazeri A, Mohammadi S, M, Hesari P, Ghaemi M, Riazi H, Sheikhi-Mobarakeh Z. Preliminary guideline for reporting bibliometric reviews of the biomedical literature (BIBLIO): a minimum requirements. Syst Rev 2023;12:239. [Crossref] [PubMed]
- Chen C. CiteSpace II: Detecting and visualizing emerging trends and transient patterns in scientific literature. J Am Soc Inf Sci Technol 2016;57:359-377.
- Aria M, Cuccurullo C. bibliometrix: An R-tool for comprehensive science mapping analysis. Journal of Informetrics 2017;11:959-975.
- Ardila D, Kiraly AP, Bharadwaj S, Choi B, Reicher JJ, Peng L, Tse D, Etemadi M, Ye W, Corrado G, Naidich DP, Shetty S. End-to-end lung cancer screening with three-dimensional deep learning on low-dose chest computed tomography. Nat Med 2019;25:954-61. Erratum in: Nat Med 2019;25:1319. [Crossref] [PubMed]
- Gillies RJ, Kinahan PE, Hricak H. Radiomics: Images Are More than Pictures, They Are Data. Radiology 2016;278:563-77. [Crossref] [PubMed]
- Silver D, Huang A, Maddison CJ, Guez A, Sifre L, van den Driessche G, Schrittwieser J, Antonoglou I, Panneershelvam V, Lanctot M, Dieleman S, Grewe D, Nham J, Kalchbrenner N, Sutskever I, Lillicrap T, Leach M, Kavukcuoglu K, Graepel T, Hassabis D. Mastering the game of Go with deep neural networks and tree search. Nature 2016;529:484-9. [Crossref] [PubMed]
- McIntosh SA, Alam F, Adams L, Boon IS, Callaghan J, Conti I, et al. Global funding for cancer research between 2016 and 2020: a content analysis of public and philanthropic investments. Lancet Oncol 2023;24:636-45. [Crossref] [PubMed]
- Wang S, Zhou M, Liu Z, Liu Z, Gu D, Zang Y, Dong D, Gevaert O, Tian J. Central focused convolutional neural networks: Developing a data-driven model for lung nodule segmentation. Med Image Anal 2017;40:172-83. [Crossref] [PubMed]
- Xu Y, Hosny A, Zeleznik R, Parmar C, Coroller T, Franco I, Mak RH, Aerts HJWL. Deep Learning Predicts Lung Cancer Treatment Response from Serial Medical Imaging. Clin Cancer Res 2019;25:3266-75. [Crossref] [PubMed]
- Rios Velazquez E, Parmar C, Liu Y, Coroller TP, Cruz G, Stringfield O, Ye Z, Makrigiorgos M, Fennessy F, Mak RH, Gillies R, Quackenbush J, Aerts HJWL. Somatic Mutations Drive Distinct Imaging Phenotypes in Lung Cancer. Cancer Res 2017;77:3922-30. [Crossref] [PubMed]
- Herbst RS, Morgensztern D, Boshoff C. The biology and management of non-small cell lung cancer. Nature 2018;553:446-54. [Crossref] [PubMed]
- Evangelista L, Fiz F, Laudicella R, Bianconi F, Castello A, Guglielmo P, Liberini V, Manco L, Frantellizzi V, Giordano A, Urso L, Panareo S, Palumbo B, Filippi L. PET Radiomics and Response to Immunotherapy in Lung Cancer: A Systematic Review of the Literature. Cancers (Basel) 2023.
- da Cunha Santos G, Shepherd FA, Tsao MS. EGFR mutations and lung cancer. Annu Rev Pathol 2011;6:49-69. [Crossref] [PubMed]
- Paez JG, Jänne PA, Lee JC, Tracy S, Greulich H, Gabriel S, Herman P, Kaye FJ, Lindeman N, Boggon TJ, Naoki K, Sasaki H, Fujii Y, Eck MJ, Sellers WR, Johnson BE, Meyerson M. EGFR mutations in lung cancer: correlation with clinical response to gefitinib therapy. Science 2004;304:1497-500. [Crossref] [PubMed]
- Mu W, Jiang L, Zhang J, Shi Y, Gray JE, Tunali I, Gao C, Sun Y, Tian J, Zhao X, Sun X, Gillies RJ, Schabath MB. Non-invasive decision support for NSCLC treatment using PET/CT radiomics. Nat Commun 2020;11:5228. [Crossref] [PubMed]
- Zhang J, Zhao X, Zhao Y, Zhang J, Zhang Z, Wang J, Wang Y, Dai M, Han J. Value of pre-therapy 18F-FDG PET/CT radiomics in predicting EGFR mutation status in patients with non-small cell lung cancer. Eur J Nucl Med Mol Imaging 2020;47:1137-46.
- Dong Y, Jiang Z, Li C, Dong S, Zhang S, Lv Y, Sun F, Liu S. Development and validation of novel radiomics-based nomograms for the prediction of EGFR mutations and Ki-67 proliferation index in non-small cell lung cancer. Quant Imaging Med Surg 2022;12:2658-71. [Crossref] [PubMed]
- Liu Z, Duan T, Zhang Y, Weng S, Xu H, Ren Y, Zhang Z, Han X. Radiogenomics: a key component of precision cancer medicine. Br J Cancer 2023;129:741-53. [Crossref] [PubMed]
- Wang R, Dai W, Gong J, Huang M, Hu T, Li H, Lin K, Tan C, Hu H, Tong T, Cai G. Development of a novel combined nomogram model integrating deep learning-pathomics, radiomics and immunoscore to predict postoperative outcome of colorectal cancer lung metastasis patients. J Hematol Oncol 2022;15:11. [Crossref] [PubMed]
- Ferreira JR Junior, Koenigkam-Santos M, Cipriano FEG, Fabro AT, Azevedo-Marques PM. Radiomics-based features for pattern recognition of lung cancer histopathology and metastases. Comput Methods Programs Biomed 2018;159:23-30. [Crossref] [PubMed]
- Lin P, Lin YQ, Gao RZ, Wan WJ, He Y, Yang H. Integrative radiomics and transcriptomics analyses reveal subtype characterization of non-small cell lung cancer. Eur Radiol 2023;33:6414-25. [Crossref] [PubMed]
- Zanfardino M, Franzese M, Pane K, Cavaliere C, Monti S, Esposito G, Salvatore M, Aiello M. Bringing radiomics into a multi-omics framework for a comprehensive genotype-phenotype characterization of oncological diseases. J Transl Med 2019;17:337. [Crossref] [PubMed]
- Avanzo M, Wei L, Stancanello J, Vallières M, Rao A, Morin O, Mattonen SA, El Naqa I. Machine and deep learning methods for radiomics. Med Phys 2020;47:e185-202. [Crossref] [PubMed]
- Mukherjee P, Zhou M, Lee E, Schicht A, Balagurunathan Y, Napel S, Gillies R, Wong S, Thieme A, Leung A, Gevaert O. A Shallow Convolutional Neural Network Predicts Prognosis of Lung Cancer Patients in Multi-Institutional CT-Image Data. Nat Mach Intell 2020;2:274-82. [Crossref] [PubMed]
- Lin X, Jiao H, Pang Z, Chen H, Wu W, Wang X, Xiong L, Chen B, Huang Y, Li S, Li L. Lung Cancer and Granuloma Identification Using a Deep Learning Model to Extract 3-Dimensional Radiomics Features in CT Imaging. Clin Lung Cancer 2021;22:e756-66. [Crossref] [PubMed]
- Niu C, Wang G. Unsupervised contrastive learning based transformer for lung nodule detection. Phys Med Biol 2022;
- Qian L, Wu T, Kong S, Lou X, Jiang Y, Tan Z, Wu L, Gao C. Could the underlying biological basis of prognostic radiomics and deep learning signatures be explored in patients with lung cancer? A systematic review. Eur J Radiol 2024;171:111314. [Crossref] [PubMed]
- Zwanenburg A, Vallières M, Abdalah MA, Aerts HJWL, Andrearczyk V, Apte A, et al. The Image Biomarker Standardization Initiative: Standardized Quantitative Radiomics for High-Throughput Image-based Phenotyping. Radiology 2020;295:328-38. [Crossref] [PubMed]
- Karger E, Kureljusic M. Artificial Intelligence for Cancer Detection-A Bibliometric Analysis and Avenues for Future Research. Curr Oncol 2023;30:1626-47. [Crossref] [PubMed]
- Volpe S, Mastroleo F, Krengli M, Jereczek-Fossa BA. Quo vadis Radiomics? Bibliometric analysis of 10-year Radiomics journey. Eur Radiol 2023;33:6736-45. [Crossref] [PubMed]
- Zhong R, Gao T, Li J, Li Z, Tian X, Zhang C, Lin X, Wang Y, Gao L, Hu K. The global research of artificial intelligence in lung cancer: a 20-year bibliometric analysis. Front Oncol 2024;14:1346010. [Crossref] [PubMed]
- Liang H, Chen Z, Wei F, Yang R, Zhou H. Bibliometrics research on radiomics of lung cancer. Transl Cancer Res 2021;10:3757-71. [Crossref] [PubMed]