Differentiation of early-stage endometrial carcinoma from benign endometrial lesions: a comparative study of six diffusion models
Introduction
Endometrial carcinoma (EC) is the fourth most prevalent gynecological malignancy, with its morbidity and mortality rates growing annually and an increasing incidence observed in younger populations (1). Endometrial hyperplasia and polyps are common benign endometrial lesions (2). Although they share similar clinical manifestations with EC, their treatment approaches and prognoses differ significantly (3). A medication or minimally invasive approach to hysterectomy is preferred for benign endometrial lesions (4), whereas primary treatment of early-stage EC consists of hysterectomy and bilateral salpingo-oophorectomy with or without pelvic and para-aortic lymphadenectomy (5). The gold standard for preoperative differentiation between benign and malignant endometrial lesions is biopsy (6). However, biopsy is an invasive procedure and may be unsuitable for patients with cervical or vaginal stenosis (7). Additionally, sampling errors can occur, potentially compromising the diagnostic accuracy (8). Therefore, in order to achieve precise diagnosis and personalized treatment, it is imperative to develop a noninvasive method for accurately differentiating between benign and malignant endometrial lesions before surgery.
Magnetic resonance imaging (MRI), especially diffusion-weighted imaging (DWI), plays a crucial role in the preoperative diagnosis and staging of EC (2,9). The apparent diffusion coefficient (ADC) is the most common quantitative parameter of monoexponential DWI, capable of reflecting the biological characteristics of different tissues by characterizing the Gaussian diffusion of water molecules. However, the diffusion displacement of water molecules significantly deviates from the Gaussian distribution in highly heterogeneous tumors, and ADC values cannot reflect the non-Gaussian diffusion characteristics of water molecules, nor can they truly reflect the diffusion properties of water molecules in tumors (10). In order to accurately analyze the abnormal diffusion movement of water molecules in tumors, some researchers have developed the following mathematical models based on multiple-b-value DWI: diffusion kurtosis imaging (DKI) (11), intravoxel incoherent motion (IVIM) (12), stretched exponential model (SEM) (13), continuous-time random walk (CTRW) (14), and fractional order calculus (FROC) (15). Recently, the DKI, IVIM, and SEM models have been incrementally applied in the diagnosis and assessment in patients with EC (16-20). Previous studies have demonstrated that some non-Gaussian models surpass the monoexponential model in the histopathological assessment of malignant tumors (20,21). Nevertheless, the applicability of CTRW and FROC models for preoperative diagnosis and evaluation of EC remains uncertain, and there is a lack of comparative studies involving multiple diffusion models.
The purpose of this study was to compare the diffusion parameters of six diffusion models (DWI, DKI, IVIM, SEM, CTRW, and FROC) in distinguishing early-stage EC from benign endometrial lesions and to identify potential predictors for early-stage EC. We present this article in accordance with the STROBE reporting checklist (available at https://qims.amegroups.com/article/view/10.21037/qims-24-896/rc).
Methods
Patients
This prospective study received approval from the Ethics Committee of the First People’s Hospital of Yunnan Province (No. KHLL2023-KY104) and was conducted in accordance with the Declaration of Helsinki (as revised in 2013). Informed consent was obtained from all individual participants. Between April 2021 and July 2023, a total of 150 consecutive patients with suspected benign or malignant endometrial lesions were enrolled in the prospective study based on the results of the initial calculation of sample size. The inclusion criteria were as follows: (I) patients with stage I–II EC, endometrial hyperplasia, or endometrial polyps confirmed by surgery and pathology; (II) completion of conventional MRI and multiple-b-value (0–3,000 s/mm2) DWI scans within 2 weeks before surgery; and (III) availability of complete clinical data. Meanwhile, the exclusion criteria were as follows: (I) poor MRI quality; (II) completion of preoperative chemoradiotherapy, hormone therapy, or other treatments; (III) lesions with a maximum diameter of less than 1 cm; and (IV) a history of other pelvic malignancies. Finally, a total of 72 eligible patients were included in the study. The flowchart of the patient selection process is displayed in Figure S1.
MRI protocol
All patients underwent preoperative pelvic MRI scanning using a 3-T MRI scanner (MAGNETOM Prisma, Siemens Healthineers, Erlangen, Germany). The imaging protocol comprised conventional MRI sequences and multiple-b-value DWI using a single-shot echo-planar imaging that included 13 b-values: 0, 10, 20, 30, 50, 70, 100, 150, 200, 400, 1,000, 2,000, and 3,000 s/mm2. The parameters of multiple-b-value DWI were as follows: repetition time (TR) =3,000 ms, echo time (TE) =64 ms, field of view (FOV) =360×360 mm2, matrix =164×164, slice thickness =5 mm, and slice gap =1 mm. The conventional MRI sequences and parameters were as follows: sagittal T2-weighted imaging (T2WI) (TR =5,470 ms, TE =89 ms, FOV =210×210 mm2, matrix =384×384, slice thickness =3 mm, slice gap =3.6 mm); axial T1WI (TR =480 ms, TE =10 ms, FOV =346×313 mm2, matrix =384×384, slice thickness =5 mm, slice gap =6 mm), axial T2WI (TR =8,230 ms, TE =97 ms, FOV =360×360 mm2, matrix =384×384, slice thickness =5 mm, slice gap =6 mm), sagittal contrast-enhanced T1WI (TR =3.92 ms, TE =1.46 ms, FOV =260×260 mm2, matrix =320×320, slice thickness =3 mm, slice gap =0 mm), and axial contrast-enhanced T1WI (TR =2.9 ms, TE =1.09 ms, FOV =346×313 mm2, matrix =290×320, slice thickness =3 mm, slice gap =0 mm). The contrast agent was administered intravenously with gadolinium meglumine (0.2 mL/kg) at a rate of 1.5 mL/s, which was followed by a rinse with 10 ml of normal saline at a rate of 2 mL/s.
Image processing and analysis
Body-DiffusionLab software on MR Station (BoDiLab; Chengdu ZhongYing Medical Technology Co., Ltd., Chengdu, China) was used to generate diffusion parameter maps of six diffusion models (DWI, DKI, IVIM, SEM, CTRW, and FROC) from multiple-b-value DWI. For image processing, DWI used b-values of 0 and 1,000 s/mm2, IVIM employed b-values ranging from 0 and 200 s/mm2, and the remaining models used b-values of 0, 1,000, 2,000, and 3,000 s/mm2. The formulae for the respective models are as follows:
- Monoexponential DWI model:
where S(b) and S0 are the signal intensities when b>0 s/mm2 and b=0 s/mm2 are applied, respectively. - DKI model:
where D represents the mean diffusivity, and K represents the mean kurtosis (MK). - IVIM model:
where D represents the true diffusion coefficient (D) of the water molecules, D*represents the pseudodiffusion coefficient (D*) of microcirculation, and f represents the perfusion fraction. - SEM model:
where DDC represents the distributed diffusion coefficient, and α represents the intravoxel diffusion heterogeneity index. - CTRW model:
where Eα represents the Mittag-Leffler function of α order; D represents the anomalous diffusion coefficient; and α and β represent the temporal diffusion heterogeneity index (αCTRW) and spatial diffusion heterogeneity index, respectively. - FROC model:
where D represents diffusion coefficient, µ represents the spatial constant, β represents the fractional order derivative in space, Gd represents diffusion gradient amplitude, δ represents diffusion gradient pulse width, and ∆ represents the gradient lobe separation.
Fourteen diffusion parameter maps were obtained from the above six models (Figure 1): ADC map derived from the DWI model; mean diffusivity (MD) and MK maps derived from the DKI model; D, pseudo diffusion coefficient (D*), and perfusion fraction (f) maps derived from the IVIM model; intravoxel diffusion heterogeneity index (αSEM) and distributed diffusion coefficient (DDC) maps derived from the SEM model; αCTRW, spatial diffusion heterogeneity index (βCTRW), and the anomalous diffusion coefficient (DCTRW) map derived from the CTRW model; and fractional order derivative in space (βFROC), diffusion coefficient (DFROC), and spatial constant (µFROC) maps derived from the FROC model. Two radiologists (A and B, with 5 and 10 years of experience in pelvic MRI, respectively) blinded to the histopathological findings independently delineated the regions of interest (ROIs) of the lesions using ITK-SNAP (http://www.itksnap.org/pmwiki/pmwiki.php). The ROIs were outlined along the lesion contour at the maximum slice of the lesions on DWI (b=1,000 s/mm2) or ADC maps with reference to other MRI sequences. Care was taken to exclude the surrounding normal myometrium and any obvious cystic, hemorrhagic, or necrotic areas. Subsequently, the delineated ROIs were applied to other diffusion parameter maps, and the mean value of each diffusion parameter was derived.
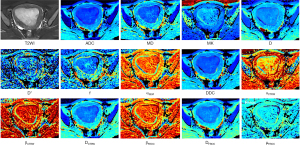
Statistical analysis
We used PASS version 15 software (NCSS, Kaysville, UT, USA) to calculate the initial sample size. Statistical analyses were performed using SPSS version 26 software (IBM Corp., Armonk, NY, USA). Continuous variables for normal and skewed distributions are presented as the mean ± standard deviation and as the median and interquartile range (IQR = percentiles 75 – percentiles 25), respectively. Categorical variables are presented as numbers and percentages. The interclass correlation coefficient (ICC) was used to assess the interobserver agreement between radiologists A and B in the measurement of the diffusion parameters (ICC <0.50, poor; 0.50–0.75, moderate; 0.75–0.90, good; and >0.90, excellent). The measurements of senior radiologist B were used for subsequent analysis. The Kolmogorov-Smirnov test was conducted to assess the normal distribution of continuous variables. If the variables followed a normal distribution, the t test was used to compare differences between benign and malignant lesions; otherwise, the Mann-Whitney test was used. Categorical variables were compared using the chi-square test or the Fisher exact test. A P value <0.05 was considered to indicate statistical difference, while a P value <0.001 was deemed to represent a highly significant difference. Univariate and multivariate logistic regression analyses were performed on diffusion parameters that demonstrated highly significant differences (P<0.001) to select independent predictors of early-stage EC. These were then integrated with clinical parameters (P<0.05) to establish combined models. The performance of each parameter or model was evaluated using the area under the curve (AUC) of the receiver operating characteristic (ROC) curve. The diagnostic threshold was determined with maximum Youden Index, and then sensitivity, specificity, and accuracy were calculated. The Delong test is used to compare the predictive performance of different models.
Results
Clinicopathologic characteristics
A total of 72 patients (17 patients with benign endometrial lesions and 55 patients with EC) were included in this study. The clinicopathological characteristics are presented in Table 1. The patients with EC consisted of 50 patients with endometrioid adenocarcinoma and 5 patients with nonendometrioid adenocarcinoma, while the benign patients comprised 7 patients with endometrial polyps, 9 patients with endometrial hyperplasia, and 1 patient with both hyperplasia and polyps. The median age or mean age of the patients with EC and the benign patients was 55.4±10.7 and 48±10.3 years, respectively, representing a significant difference (P=0.015). The proportion of menopausal patients in the EC group was significantly higher than that of patients with benign lesions (P=0.011). No significant differences were observed between the benign and malignant groups in terms of primary manifestations (P=0.090), hypertension (P=0.361), diabetes (P=1.000), body mass index (BMI) (P=0.388), cancer antigen (CA)125 (P=0.909), or CA199 (P=0.095).
Table 1
Parameter | EC (n=55) | Benign endometrial lesions (n=17) | P |
---|---|---|---|
Age (years) | 55.4±10.7 | 48.0±10.3 | 0.015* |
Histological type, n (%) | NA | ||
Endometrioid adenocarcinoma | 50 (90.9) | NA | |
Non-endometrioid adenocarcinoma | 5 (9.1) | NA | |
Endometrial polyp | NA | 7 (41.2) | |
Endometrial hyperplasia | NA | 9 (52.9) | |
Endometrial polyp + hyperplasia | NA | 1 (5.9) | |
Deep myometrial invasion (yes/no), n (%) | 10 (18.2)/45 (81.8) | NA | NA |
Cervical stromal invasion (yes/no), n (%) | 5 (9.1)/50 (90.9) | NA | NA |
Histologic grade, n (%) | NA | ||
Grade 1 | 17 (30.9) | NA | |
Grade 2 | 25 (45.4) | NA | |
Grade 3 | 9 (16.4) | NA | |
Unknown | 4 (7.3) | NA | |
Chief manifestation, n (%) | 0.090 | ||
Irregular vaginal bleeding | 43 (78.2) | 13 (76.5) | |
Menstrual disorder | 7 (12.7) | 0 (0.0) | |
Asymptomatic | 0 (0.0) | 1 (5.9) | |
Other symptoms | 5 (9.1) | 3 (17.6) | |
Menopause (yes/no), n (%) | 30 (54.4)/25 (45.5) | 3 (17.6)/14 (82.4) | 0.011* |
Hypertension (yes/no), n (%) | 18 (32.7)/37 (67.3) | 3 (17.6)/14 (82.4) | 0.361 |
Diabetes (yes/no), n (%) | 5 (9.1)/50 (90.9) | 1 (5.9)/16 (94.1) | 1.000 |
BMI (kg/m2) | 24.7 (4.4) | 24.612±4.265 | 0.388 |
CA125 (U/mL) | 16.0 (20.0) | 19.0 (13.3) | 0.909 |
CA199 (U/mL) | 19.1 (30.2) | 13.876±12.229 | 0.095 |
FIGO stage, n (%) | NA | ||
IA | 42 (76.4) | NA | |
IB | 8 (14.5) | NA | |
II | 5 (9.1) | NA |
Continuous variables for normal and skew distributions are presented as mean ± standard deviation and as median (interquartile range), respectively. Interquartile range = percentiles 75 – percentiles 25. *, P<0.05. EC, endometrial carcinoma; NA, not applicable; BMI, body mass index; CA, cancer antigen; FIGO, Federation International of Gynecology and Obstetrics.
Diffusion parameters
The ICC range for the diffusion parameters was 0.933–0.991, indicating a high level of consistency across all parameters. The comparison of various diffusion parameters between the EC group and the benign group is shown in Table 2 and Figure 2. All the diffusion parameters were statistically different between the EC group and the benign group (P=0.05), except for D* [median 4.204 (IQR 0.970) vs. 3.738±1.144; P=0.268] and f [0.164±0.058 vs. median 0.180 (IQR 0.100); P=0.235] values. Additionally, the EC group and the benign group showed highly significant differences (P<0.001) in terms of ADC [0.741 (0.300)×10−3 vs. (1.276±0.416)×10−3 mm2/s], MK [median 0.926 (IQR 0.240) vs. 0.609±0.214], D [median 0.615 (IQR 0.220)×10−3 vs. (1.032±0.306)×10−3 mm2/s], DDC [median 0.799 (IQR 0.370)×10−3 vs. median 1.215 (IQR 0.580)×10−3 mm2/s], αCTRW [0.736±0.094 vs. median 0.856 (IQR 0.080)], DCTRW [median 1.279 (IQR 0.580)×10−3 vs. median 0.885 (IQR 0.360)×10−3 mm2/s], and DFROC [median 0.653 (IQR 0.260)×10−3 vs. (1.180±0.438)×10−3 mm2/s] values. According to univariate and multivariate logistic regression analyses (Table 3), only MK, D, and αCTRW could be used as independent predictors of early-stage EC (P<0.05) among the above seven parameters with a significant difference.
Table 2
Diffusion model | Diffusion parameter | EC group | Benign endometrial lesion group | P |
---|---|---|---|---|
DWI | ADC (10−3 mm2/s) | 0.741 (0.300) | 1.276±0.416 | <0.001** |
DKI | MD (10−3 mm2/s) | 1.054 (0.460) | 1.427 (0.680) | 0.001* |
MK | 0.926 (0.240) | 0.609±0.214 | <0.001** | |
IVIM | D (10−3 mm2/s) | 0.615 (0.220) | 1.032±0.306 | <0.001** |
D* (10−3 mm2/s) | 4.204 (0.970) | 3.738±1.144 | 0.268 | |
F | 0.164±0.058 | 0.180 (0.100) | 0.235 | |
SEM | αSEM | 0.786 (0.07) | 0.858 (0.050) | 0.001* |
DDC (10−3 mm2/s) | 0.799 (0.370) | 1.215 (0.580) | <0.001** | |
CTRW | αCTRW | 0.736±0.094 | 0.856 (0.080) | <0.001** |
βCTRW | 0.906±0.052 | 0.937±0.046 | 0.033* | |
DCTRW (10−3 mm2/s) | 1.279 (0.580) | 0.885 (0.360) | <0.001** | |
FROC | βFROC | 0.829±0.062 | 0.887±0.062 | 0.001* |
DFROC (10−3 mm2/s) | 0.653 (0.260) | 1.180±0.438 | <0.001** | |
μFROC (mm) | 3.244 (0.430) | 2.943 (0.900) | 0.025* |
Continuous variables for normal and skew distributions are presented as the mean ± standard deviation and as median (interquartile range), respectively. *, P<0.05; **, P<0.001. EC, endometrial carcinoma; DWI, diffusion-weighted imaging; ADC, apparent diffusion coefficient; DKI, diffusion kurtosis imaging; MD, mean diffusivity; MK, mean kurtosis; IVIM, intravoxel incoherent motion; D, true diffusion coefficient; D*, pseudodiffusion coefficient; f, perfusion fraction; SEM, stretched exponential model; αSEM, intravoxel diffusion heterogeneity index; DDC, distributed diffusion coefficient; CTRW, continuous-time random walk; αCTRW, temporal diffusion heterogeneity index; βCTRW, spatial diffusion heterogeneity index; DCTRW, anomalous diffusion coefficient; FROC, fractional order calculus; βFROC, fractional order derivative in space; DFROC, diffusion coefficient; μFROC, spatial constant.
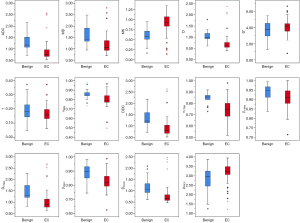
Table 3
Diffusion model | Diffusion parameter | Univariable LR | Multivariable LR | |||
---|---|---|---|---|---|---|
OR (95% CI) | P | OR (95% CI) | P | |||
DWI | ADC (10−3 mm2/s) | 0.998 (0.997–1.000) | 0.009* | 0.999 (0.952–1.047) | 0.954 | |
DKI | MK | 1.005 (1.002–1.007) | <0.001* | 1.010 (1.002–1.018) | 0.014* | |
IVIM | D (10−3 mm2/s) | 0.998 (0.997–1.000) | 0.016* | 1.008 (1.003–1.012) | 0.002* | |
SEM | DDC (10−3 mm2/s) | 0.998 (0.997–1.000) | 0.006* | 0.998 (0.992–1.003) | 0.443 | |
CTRW | αCTRW | 0.984 (0.975–0.993) | <0.001* | 0.973 (0.950–0.996) | 0.022* | |
DCTRW (10−3 mm2/s) | 0.998 (0.997–1.000) | 0.007* | 1.022 (0.982–1.064) | 0.294 | ||
FROC | DFROC (10−3 mm2/s) | 0.998 (0.997–1.000) | 0.009* | 1.001 (0.987–1.015) | 0.913 |
*, P<0.05. LR, logistic regression; EC, endometrial carcinoma; OR, odds ratio; CI, confidence interval; DWI, diffusion-weighted imaging; ADC, apparent diffusion coefficient; DKI, diffusion kurtosis imaging; MK, mean kurtosis; IVIM, intravoxel incoherent motion; D, true diffusion coefficient; SEM, stretched exponential model; DDC, distributed diffusion coefficient; CTRW, continuous-time random walk; αCTRW, temporal diffusion heterogeneity index; DCTRW, anomalous diffusion coefficient; FROC, fractional order calculus; DFROC, diffusion coefficient; μFROC, spatial constant.
Predictive performance
ROC analyses of various diffusion parameters and clinical parameters with statistical differences are shown in Table 4 and Figure 3. The AUC, accuracy, sensitivity, and specificity of these diffusion parameters in differentiating early-stage EC and benign endometrial lesions had ranges of 0.677–0.848, 65.3–86.1%, 64.7–82.4%, and 65.5–89.1%, respectively. The AUC of αCTRW was the highest [0.848 (95% CI: 0.751–0.945)], followed by MK [0.839 (0.737–0.940)]. The AUC, accuracy, sensitivity, and specificity of age and menopause in predicting early-stage EC was 0.696 (95% CI: 0.545–0.848) and 0.684 (95% CI: 0.547–0.822), 69.4% and 61.1%, 69.1% and 54.5%, and 70.6% and 82.4%, respectively, with an age threshold of 50.5 years. The differential performance of the combined models constructed by independent predictors and effective clinical parameters for distinguishing between early-stage EC and benign endometrial lesions is shown in Table 4, Figures 3-5. The ranges of the AUC, accuracy, sensitivity, and specificity of the combined models were 0.903–0.922, 86.1–93.1%, 85.5–100.0%, and 70.6–88.2%, respectively. Specifically, the AUC of the model combining MK, D, and αCTRW was 0.903 (0.824–0.982), which surpassed that of any single diffusion parameter. Meanwhile, the model combining MK, D, αCTRW, and menopausal status (MK + D + αCTRW + menopausal status) exhibited the highest AUC [0.922 (95% CI 0.845–0.999)], accuracy (93.1%), and sensitivity (100.0%) among all the models. According to the Delong tests (Figure S2), the AUCs of the MK + D + αCTRW + menopausal status model were significantly different from those of other models (P<0.05) except the combined models and the αCTRW model.
Table 4
Model | Parameter | Cutoff values | AUC (95% CI) | Accuracy | Sensitivity | Specificity | Youden index |
---|---|---|---|---|---|---|---|
DWI | ADC (10−3 mm2/s) | 1.056 | 0.826 (0.724–0.927) | 83.3% | 76.5% | 85.5% | 0.620 |
DKI | MD (10−3 mm2/s) | 1.329 | 0.781 (0.668–0.894) | 76.4% | 82.4% | 74.5% | 0.569 |
MK | 0.718 | 0.839 (0.737–0.940) | 84.7% | 76.5% | 87.3% | 0.638 | |
IVIM | D (10−3 mm2/s) | 0.926 | 0.821 (0.714–0.929) | 86.1% | 76.5% | 89.1% | 0.656 |
SEM | αSEM | 0.839 | 0.759 (0.621–0.897) | 73.6% | 76.5% | 74.5% | 0.510 |
DDC (10−3 mm2/s) | 1.133 | 0.824 (0.720–0.928) | 83.3% | 76.5% | 85.5% | 0.620 | |
CTRW | αCTRW | 0.833 | 0.848 (0.751–0.945) | 86.1% | 76.5% | 89.1% | 0.656 |
βCTRW | 0.927 | 0.677 (0.526–0.828) | 65.3% | 64.7% | 65.5% | 0.302 | |
DCTRW (10−3 mm2/s) | 1.140 | 0.810 (0.699–0.920) | 79.2% | 82.4% | 78.2% | 0.606 | |
FROC | βFROC | 0.886 | 0.765 (0.631–0.898) | 81.9% | 64.7% | 87.3% | 0.520 |
DFROC (10-3 mm2/s) | 0.912 | 0.829 (0.727–0.931) | 83.3% | 76.5% | 85.5% | 0.620 | |
μFROC (mm) | 3.155 | 0.680 (0.530–0.830) | 68.1% | 70.6% | 67.3% | 0.379 | |
Clinical parameters | Age (years) | 50.5 | 0.696 (0.545–0.848) | 69.4% | 69.1% | 70.6% | 0.397 |
Menopausal status | 0.500 | 0.684 (0.547–0.822) | 61.1% | 54.5% | 82.4% | 0.369 | |
Combined models | MK + D + αCTRW | 0.699 | 0.903 (0.824–0.982) | 86.1% | 87.3% | 82.4% | 0.697 |
MK + D + αCTRW + age | 0.729 | 0.918 (0.834–1.000) | 86.1% | 85.5% | 88.2% | 0.737 | |
MK + D + αCTRW + menopausal status | 0.352 | 0.922 (0.845–0.999) | 93.1% | 100.0% | 70.6% | 0.706 | |
MK + D + αCTRW + age + menopausal status |
0.659 | 0.922 (0.842–1.000) | 88.9% | 90.9% | 82.4% | 0.733 |
ROC, receiver operating characteristic; EC, endometrial carcinoma; AUC, area under the curve; CI, confidence interval; DWI, diffusion-weighted imaging; ADC, apparent diffusion coefficient; DKI, diffusion kurtosis imaging; MD, mean diffusivity; MK, mean kurtosis; IVIM, intravoxel incoherent motion; D, true diffusion coefficient; SEM, stretched exponential model; αSEM, intravoxel diffusion heterogeneity index; DDC, distributed diffusion coefficient; CTRW, continuous-time random walk; αCTRW, temporal diffusion heterogeneity index; βCTRW, spatial diffusion heterogeneity index; DCTRW, anomalous diffusion coefficient; FROC, fractional order calculus; βFROC, fractional order derivative in space; DFROC, diffusion coefficient; μFROC, spatial constant.
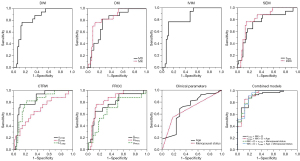
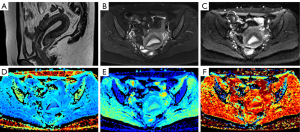
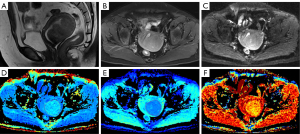
Discussion
In this study, except for D* and f of IVIM model, all the diffusion parameters derived from the DWI, DKI, IVIM, SEM, CTRW, and FROC models proved valuable in distinguishing early-stage EC from benign endometrial lesions. Specifically, MK, D, and αCTRW emerged as independent predictors for the diagnosis of early-stage EC, achieving a combined AUC of 0.903. When independent predictors were modeled in conjunction with menopausal status, the AUC was the highest (0.922).
Irregular vaginal bleeding is the most common clinical manifestation of benign and malignant endometrial lesions, often leading to overtreatment of benign endometrial lesions or undertreatment of early-stage EC (22). Uglietti et al. (23) confirmed that age and menopausal status could distinguish early-stage EC from benign endometrial lesions, noting that women over the age of 50 years and postmenopausal women exhibit a heightened risk of developing EC, which is consistent with our study. However, the performance of clinical indicators in predicting early-stage EC is low, and more reliable independent predictors needed to be identified.
We further found that there were significant differences in ADC, MD, and MK values between the benign and malignant groups, aligning with findings from previous studies (17,18). The cell density of early-stage EC is higher compared to that of benign endometrial lesions, resulting in more restricted water molecule diffusion, so the ADC values of early-stage EC are significantly lower than those of benign endometrial lesions (24). However, the T2 relaxation time may affect the accuracy of the ADC values (25). MD is a diffusion parameter obtained from the ADC and corrected by non-Gaussian distribution (26). The tumor cells of EC are densely packed, and their extracellular volume is reduced, leading to significantly limited diffusion of water molecules. In contrast, benign endometrial lesions are characterized by sparse cellular distribution and enlarged glands, resulting in less limited diffusion of water molecules (18). Hence, the MD values of early-stage EC were lower than those of benign endometrial lesions. MK is positively correlated with the complexity and heterogeneity of microstructures (27). We found that the MK values of early-stage EC were significantly higher than those of benign endometrial lesions. The possible reason for this is that EC cells exhibit more active proliferation compared to benign endometrial lesions, with more neovascularization, more complex internal components, and greater deviation of diffusion, resulting in higher MK values (18).
The IVIM model facilitates the simultaneous assessment the diffusion of water molecules and the perfusion of capillaries (28). D values are one of the independent predictors of early-stage EC, potentially due to the exclusion of the microcirculation perfusion effect (29). The growth and metabolism of EC cells are more vigorous than are those of benign endometrial lesions. EC cells are also characterized by higher cell density and a greater abundance of macromolecular substances such as intracellular proteins, which increases the resistance of water molecules to movement both intra- and extracellularly, ultimately leading to lower D values in the EC (17). Nevertheless, because the IVIM model is susceptible to the biochemical composition of tissues, the observed IVIM parameters may not represent the physiological measures (30). The SEM model is considered to be more suitable for assessing diffusion-weighted signal attenuation on account of the heterogeneous environment of spin, which not only fits the diffusion signal curve more accurately but also more aptly reflects the microstructure of biological tissues (31,32). In line with our study, Meng et al. (33) also posited that αSEM and DDC could be used to diagnose EC, but the performance of these values was lower than that of ADC values in their study. Recent research suggests no significant difference in DDC between high and low proliferative EC (16) and no significant difference in αSEM between high- and low-grade EC (19), suggesting that the utility of the SEM model in the evaluation of EC requires further exploration.
Recently, the CTRW and FROC models have been applied in the identification of benign and malignant tumors, gene expression, and histopathological evaluation (34-39). This study found that all diffusion parameters of the CTRW and FROC models showed significant differences between the early-stage EC group and the benign group. A previous study suggested that the FROC model was capable of detecting detect microstructural information at high b-values, thereby enhancing the accuracy of identifying malignant breast lesions (35). βFROC values are negatively associated with increased tissue heterogeneity (40). Because of the more complex tissue of EC lesion, βFROC values are lower than those of benign endometrial lesions. Additionally, µFROC values exhibit a negative correlation with the mean diffusion length of water molecules (41). Malignant tumor cells will proliferate indefinitely, and the mean free diffusion length of water molecules is shorter, and so the µFROC values are higher than those of benign lesions. Consistent with our findings, Chang et al. (39) also found that αCTRW, βCTRW, and DCTRW values could differentiate between benign and malignant lesions. βCTRW described the heterogeneity of the diffusion “jump” length during displacement, and the greater the spatial heterogeneity in malignant lesions is, the lower the βCTRW values, potentially reflecting the immaturity and curvature of the newly formed capillaries in the malignant tumor (14,39). αCTRW indicates the probability of water molecules being retained or released during diffusion within tissue structures, and a lower αCTRW values in malignant tumors suggest a greater variation in the time of diffusion of water molecules (42). Mao et al. (34) reported αCTRW to be an independent predictor for distinguishing between high- and low-expression levels of human epidermal growth factor receptor 2 in breast cancer and proposed that αCTRW has the potential to be an alternative indicator for biopsy. Similarly, we found that αCTRW was one of the independent predictors of early-stage EC. For one, αCTRW has the characteristics of non-Gaussian distribution, which can directly reflect the underlying structural complexity of tumor. For another, early-stage EC cells demonstrate vigorous proliferation and active mitosis, and the tumor cells in different cycles may increase the temporal heterogeneity of water molecule diffusion.
Previous studies have demonstrated that the combination of multiple parameters can simultaneously capture multiple tissue characteristics such as cell structure and heterogeneity, thereby improving diagnostic efficacy (36,37,39). Li et al. (36) demonstrated that MK and αCTRW were superior to single diffusion indicators in predicting vessels encapsulating tumor clusters of hepatocellular carcinoma. We also found that the combined model incorporating MK, D, and αCTRW exhibited a higher AUC than did any single diffusion parameter, indicating that different parameters have the potential to provide complementary predictive information. Clinical parameters offer a macroscopic reflection of the overall physiological and pathological information of patients, while diffusion parameters indicate the growth and metabolism of tumor cells at the microscopic level. Therefore, when MK, D, and αCTRW were combined with effective clinical parameters in the construction of the model, the AUC was the highest.
This study involved several limitations which should be mentioned. First, the prospective nature of patient collection and the limited sample size precluded a comprehensive analysis of each parameter in EC staging and histopathological evaluation. Second, we only delineated the ROI of the slice with the maximum area of the lesion due to the limitation of the image signal-to-noise ratio, and thus the whole lesion should be studied after the imaging quality is further improved. Third, this study only involved one center and lacked external validation. In the future, prospective studies will be conducted in collaboration with multiple hospitals to improve the clinical applicability and generalizability of the model.
Conclusions
Age, menopausal status, and the diffusion parameters of the DWI, DKI, IVIM, SEM, CTRW, and FROC models are helpful in differentiating early-stage EC from benign endometrial lesions. MK, D, and αCTRW can serve as independent predictors for the diagnosis of early-stage EC. The combined model incorporating independent predictors and menopausal status yielded the highest performance.
In summary, this study examined several diffusion models, which complemented one another. We preliminarily confirmed the application value of different diffusion models in the identification of endometrial lesions. The application of the combined model has the potential to become a novel and noninvasive diagnostic biomarker for differentiating between benign and malignant endometrial lesions. These promising results are a step toward subsequent research involving larger patient groups.
Acknowledgments
Funding: This work was supported by
Footnote
Reporting Checklist: The authors completed the STROBE reporting checklist. Available at https://qims.amegroups.com/article/view/10.21037/qims-24-896/rc
Conflicts of Interest: All authors have completed the ICMJE uniform disclosure form (available at https://qims.amegroups.com/article/view/10.21037/qims-24-896/coif). S.W. is employed by Siemens Healthineers. The other authors have no conflicts of interest to declare.
Ethical Statement: The authors are accountable for all aspects of the work in ensuring that questions related to the accuracy or integrity of any part of the work are appropriately investigated and resolved. This prospective study was conducted in accordance with the Declaration of Helsinki (as revised in 2013) and received approval from the Ethics Committee of the First People’s Hospital of Yunnan Province (No. KHLL2023-KY104). Informed consent was obtained from all individual participants.
Open Access Statement: This is an Open Access article distributed in accordance with the Creative Commons Attribution-NonCommercial-NoDerivs 4.0 International License (CC BY-NC-ND 4.0), which permits the non-commercial replication and distribution of the article with the strict proviso that no changes or edits are made and the original work is properly cited (including links to both the formal publication through the relevant DOI and the license). See: https://creativecommons.org/licenses/by-nc-nd/4.0/.
References
- Siegel RL, Miller KD, Fuchs HE, Jemal A. Cancer Statistics, 2021. CA Cancer J Clin 2021;71:7-33. [Crossref] [PubMed]
- Lee Y, Kim KA, Song MJ, Park YS, Lee J, Choi JW, Lee CH. Multiparametric magnetic resonance imaging of endometrial polypoid lesions. Abdom Radiol (NY) 2020;45:3869-81. [Crossref] [PubMed]
- Shor S, Pansky M, Maymon R, Vaknin Z, Smorgick N. Prediction of Premalignant and Malignant Endometrial Polyps by Clinical and Hysteroscopic Features. J Minim Invasive Gynecol 2019;26:1311-5. [Crossref] [PubMed]
- Auclair MH, Yong PJ, Salvador S, Thurston J, Colgan TTJ, Sebastianelli A. Guideline No. 390-Classification and Management of Endometrial Hyperplasia. J Obstet Gynaecol Can 2019;41:1789-800. [Crossref] [PubMed]
- D'Oria O, Giannini A, Besharat AR, Caserta D. Management of Endometrial Cancer: Molecular Identikit and Tailored Therapeutic Approach. Clin Exp Obstet Gynecol 2023;50:210.
- Koh WJ, Abu-Rustum NR, Bean S, Bradley K, Campos SM, Cho KR, et al. Uterine Neoplasms, Version 1.2018, NCCN Clinical Practice Guidelines in Oncology. J Natl Compr Canc Netw 2018;16:170-99. [Crossref] [PubMed]
- Narice BF, Delaney B, Dickson JM. Endometrial sampling in low-risk patients with abnormal uterine bleeding: a systematic review and meta-synthesis. BMC Fam Pract 2018;19:135. [Crossref] [PubMed]
- Svirsky R, Smorgick N, Rozowski U, Sagiv R, Feingold M, Halperin R, Pansky M. Can we rely on blind endometrial biopsy for detection of focal intrauterine pathology? Am J Obstet Gynecol 2008;199:115.e1-3. [Crossref] [PubMed]
- Bi Q, Chen Y, Wu K, Wang J, Zhao Y, Wang B, Du J. The Diagnostic Value of MRI for Preoperative Staging in Patients with Endometrial Cancer: A Meta-Analysis. Acad Radiol 2020;27:960-8. [Crossref] [PubMed]
- Le Bihan D. Apparent diffusion coefficient and beyond: what diffusion MR imaging can tell us about tissue structure. Radiology 2013;268:318-22. [Crossref] [PubMed]
- Jensen JH, Helpern JA, Ramani A, Lu H, Kaczynski K. Diffusional kurtosis imaging: the quantification of non-gaussian water diffusion by means of magnetic resonance imaging. Magn Reson Med 2005;53:1432-40. [Crossref] [PubMed]
- Le Bihan D, Breton E, Lallemand D, Aubin ML, Vignaud J, Laval-Jeantet M. Separation of diffusion and perfusion in intravoxel incoherent motion MR imaging. Radiology 1988;168:497-505. [Crossref] [PubMed]
- Bennett KM, Schmainda KM, Bennett RT, Rowe DB, Lu H, Hyde JS. Characterization of continuously distributed cortical water diffusion rates with a stretched-exponential model. Magn Reson Med 2003;50:727-34. [Crossref] [PubMed]
- Ingo C, Magin RL, Colon-Perez L, Triplett W, Mareci TH. On random walks and entropy in diffusion-weighted magnetic resonance imaging studies of neural tissue. Magn Reson Med 2014;71:617-27. [Crossref] [PubMed]
- Zhou XJ, Gao Q, Abdullah O, Magin RL. Studies of anomalous diffusion in the human brain using fractional order calculus. Magn Reson Med 2010;63:562-9. [Crossref] [PubMed]
- Zhang G, Yan R, Liu W, Jin X, Wang X, Wang H, Li Z, Shang J, Wang K, Guo J, Han D. Use of biexponential and stretched exponential models of intravoxel incoherent motion and dynamic contrast-enhanced magnetic resonance imaging to assess the proliferation of endometrial carcinoma. Quant Imaging Med Surg 2023;13:2568-81. [Crossref] [PubMed]
- Meng X, Tian S, Zhang Q, Chen L, Lin L, Li J, Shen Z, Wang J, Zhang Y, Song Q, Liu A. Improved differentiation between stage I-II endometrial carcinoma and endometrial polyp with combination of APTw and IVIM MR imaging. Magn Reson Imaging 2023;102:43-8. [Crossref] [PubMed]
- Tian S, Chen A, Li Y, Wang N, Ma C, Lin L, Wang J, Liu A. The combined application of amide proton transfer imaging and diffusion kurtosis imaging for differentiating stage Ia endometrial carcinoma and endometrial polyps. Magn Reson Imaging 2023;99:67-72. [Crossref] [PubMed]
- Zhang Q, Ouyang H, Ye F, Chen S, Xie L, Zhao X, Yu X. Multiple mathematical models of diffusion-weighted imaging for endometrial cancer characterization: Correlation with prognosis-related risk factors. Eur J Radiol 2020;130:109102. [Crossref] [PubMed]
- Chen T, Li Y, Lu SS, Zhang YD, Wang XN, Luo CY, Shi HB. Quantitative evaluation of diffusion-kurtosis imaging for grading endometrial carcinoma: a comparative study with diffusion-weighted imaging. Clin Radiol 2017;72:995.e11-20. [Crossref] [PubMed]
- Yang H, Ge X, Zheng X, Li X, Li J, Liu M, Zhu J, Qin J. Predicting Grade of Esophageal Squamous Carcinoma: Can Stretched Exponential Model-Based DWI Perform Better Than Bi-Exponential and Mono-Exponential Model? Front Oncol 2022;12:904625. [Crossref] [PubMed]
- Bi Q, Wang Y, Deng Y, Liu Y, Pan Y, Song Y, Wu Y, Wu K. Different multiparametric MRI-based radiomics models for differentiating stage IA endometrial cancer from benign endometrial lesions: A multicenter study. Front Oncol 2022;12:939930. [Crossref] [PubMed]
- Uglietti A, Mazzei C, Deminico N, Somigliana E, Vercellini P, Fedele L. Endometrial polyps detected at ultrasound and rate of malignancy. Arch Gynecol Obstet 2014;289:839-43. [Crossref] [PubMed]
- Fujii S, Matsusue E, Kigawa J, Sato S, Kanasaki Y, Nakanishi J, Sugihara S, Kaminou T, Terakawa N, Ogawa T. Diagnostic accuracy of the apparent diffusion coefficient in differentiating benign from malignant uterine endometrial cavity lesions: initial results. Eur Radiol 2008;18:384-9. [Crossref] [PubMed]
- Wang Y, Zhao KX, Ma FZ, Xiao BH. The contribution of T2 relaxation time to MRI-derived apparent diffusion coefficient (ADC) quantification and its potential clinical implications. Quant Imaging Med Surg 2023;13:7410-6. [Crossref] [PubMed]
- Granata V, Fusco R, Risi C, Ottaiano A, Avallone A, De Stefano A, Grimm R, Grassi R, Brunese L, Izzo F, Petrillo A. Diffusion-Weighted MRI and Diffusion Kurtosis Imaging to Detect RAS Mutation in Colorectal Liver Metastasis. Cancers (Basel) 2020.
- Thaler C, Kyselyova AA, Faizy TD, Nawka MT, Jespersen S, Hansen B, Stellmann JP, Heesen C, Stürner KH, Stark M, Fiehler J, Bester M, Gellißen S. Heterogeneity of multiple sclerosis lesions in fast diffusional kurtosis imaging. PLoS One 2021;16:e0245844. [Crossref] [PubMed]
- Zhang Q, Yu X, Lin M, Xie L, Zhang M, Ouyang H, Zhao X. Multi-b-value diffusion weighted imaging for preoperative evaluation of risk stratification in early-stage endometrial cancer. Eur J Radiol 2019;119:108637. [Crossref] [PubMed]
- Iima M, Le Bihan D. Clinical Intravoxel Incoherent Motion and Diffusion MR Imaging: Past, Present, and Future. Radiology 2016;278:13-32. [Crossref] [PubMed]
- Yu WL, Xiao BH, Ma FZ, Zheng CJ, Tang SN, Wáng YXJ. Underestimation of the spleen perfusion fraction by intravoxel incoherent motion MRI. NMR Biomed 2023;36:e4987. [Crossref] [PubMed]
- Hall MG, Barrick TR. From diffusion-weighted MRI to anomalous diffusion imaging. Magn Reson Med 2008;59:447-55. [Crossref] [PubMed]
- Zheng L, Jiang P, Lin D, Chen X, Zhong T, Zhang R, Chen J, Song Y, Xue Y, Lin L. Histogram analysis of mono-exponential, bi-exponential and stretched-exponential diffusion-weighted MR imaging in predicting consistency of meningiomas. Cancer Imaging 2023;23:117. [Crossref] [PubMed]
- Meng N, Yan R, Ren J, Wang H, Jin X, Han D. Single Index. Double exponential and tensile density index model diffusion weighted imaging in diagnosis of endometrial carcinoma. Chin J Med Imaging 2017;25:609-12+616.
- Mao C, Hu L, Jiang W, Qiu Y, Yang Z, Liu Y, Wang M, Wang D, Su Y, Lin J, Yan X, Cai Z, Zhang X, Shen J. Discrimination between human epidermal growth factor receptor 2 (HER2)-low-expressing and HER2-overexpressing breast cancers: a comparative study of four MRI diffusion models. Eur Radiol 2024;34:2546-59. [Crossref] [PubMed]
- Wang C, Wang G, Zhang Y, Dai Y, Yang D, Wang C, Li J. Differentiation of benign and malignant breast lesions using diffusion-weighted imaging with a fractional-order calculus model. Eur J Radiol 2023;159:110646. [Crossref] [PubMed]
- Li C, Wen Y, Xie J, Chen Q, Dang Y, Zhang H, Guo H, Long L. Preoperative prediction of VETC in hepatocellular carcinoma using non-Gaussian diffusion-weighted imaging at high b values: a pilot study. Front Oncol 2023;13:1167209. [Crossref] [PubMed]
- Shao X, An L, Liu H, Feng H, Zheng L, Dai Y, Yu B, Zhang J. Cervical Carcinoma: Evaluation Using Diffusion MRI With a Fractional Order Calculus Model and its Correlation With Histopathologic Findings. Front Oncol 2022;12:851677. [Crossref] [PubMed]
- Zhang A, Hu Q, Song J, Dai Y, Wu D, Chen T. Value of non-Gaussian diffusion imaging with a fractional order calculus model combined with conventional MRI for differentiating histological types of cervical cancer. Magn Reson Imaging 2022;93:181-8. [Crossref] [PubMed]
- Chang H, Wang D, Li Y, Xiang S, Yang YX, Kong P, Fang C, Ming L, Wang X, Zhang C, Jia W, Yan Q, Liu X, Zeng Q. Evaluation of breast cancer malignancy, prognostic factors and molecular subtypes using a continuous-time random-walk MR diffusion model. Eur J Radiol 2023;166:111003. [Crossref] [PubMed]
- Sui Y, Wang H, Liu G, Damen FW, Wanamaker C, Li Y, Zhou XJ. Differentiation of Low- and High-Grade Pediatric Brain Tumors with High b-Value Diffusion-weighted MR Imaging and a Fractional Order Calculus Model. Radiology 2015;277:489-96. [Crossref] [PubMed]
- Magin RL, Akpa BS, Neuberger T, Webb AG. Fractional Order Analysis of Sephadex Gel Structures: NMR Measurements Reflecting Anomalous Diffusion. Commun Nonlinear Sci Numer Simul 2011;16:4581-7. [Crossref] [PubMed]
- Karaman MM, Sui Y, Wang H, Magin RL, Li Y, Zhou XJ. Differentiating low- and high-grade pediatric brain tumors using a continuous-time random-walk diffusion model at high b-values. Magn Reson Med 2016;76:1149-57. [Crossref] [PubMed]