Development and validation of a dynamic contrast-enhanced magnetic resonance imaging-based habitat and peritumoral radiomic model to predict axillary lymph node metastasis in patients with breast cancer: a retrospective study
Introduction
Breast cancer is the most prevalent cancer worldwide and seriously endangers women’s health (1). Notably, axillary lymph node metastasis (ALNM) is the most common form of breast cancer invasion, which seriously affects the prognosis of breast cancer patients (2). The prognosis of breast cancer patients with ALNM has been demonstrated to be significantly poorer compared to those without ALNM, resulting in a decrease in the 5-year relative survival rate from 98.6% to 84.4% (3). It is imperative to develop a preoperative, non-invasive, and precise methodology for predicting ALNM in patients with breast cancer. The magnetic resonance imaging (MRI) technique, as a powerful non-invasive diagnostic modality for breast cancer, offers the potential to predict ALNM preoperatively (4). However, a meta-analysis revealed a low pooled sensitivity (0.55) and a relatively high specificity value (0.86) for preoperative MRI in detecting ALNM in breast cancer patients (5). The diagnostic performance of dynamic contrast-enhanced magnetic resonance imaging (DCE-MRI) in identifying ALNM in breast cancer patients was evaluated by Zhao et al., yielding an area under the curve (AUC) value of 0.722, which falls short of providing sufficient reliability for guiding clinical practice (6).
Radiomics is a powerful technology capable of extracting high-throughput quantitative features from medical images (7,8), demonstrating the predictive potential for lymph node metastases in various tumor types, including breast cancer (9-12), colorectal cancer (13), and lung cancer (14). Despite offering a non-invasive imaging biomarker for assessing ALNM, prior conventional radiomics studies have exhibited two prominent limitations. First, the majority of previous studies on radiomics in breast cancer primarily focused on intratumoral features (11,15-17), thereby neglecting the supplementary information provided by peritumoral radiomics features. Several studies have demonstrated that the peritumoral region harbors valuable information associated with tumor development and metastasis, such as lymphocyte infiltration (18-20), peritumoral lymphatic invasion (21), and lymphangiogenesis (22). A study has demonstrated a significant association between MRI-based peritumoral radiomics features and peritumoral lymphocyte density in breast cancer, highlighting the potential of radiomics as a non-invasive tool for predicting immune infiltration in the tumor microenvironment (23). Another study demonstrated that integrating intratumoral and 4 mm peritumoral radiomics signatures with clinicopathological features resulted in an AUC value of 0.869 for predicting sentinel lymph nodes (SLNs) in breast cancer patients (24). Subsequent research explored different sizes of the peritumoral region and found that expert-drawn regions measuring 6 mm or region of interest (ROI) drawn by another expert at 4 and 8 mm could potentially serve as optimal sizes for predicting SLN status in breast cancer (25). These results underscored the influence of peritumoral size selection on SLN prediction outcomes in breast cancer; however, they did not establish a definitive optimal peritumoral size.
Another notable limitation of conventional radiomics studies lies in their failure to capture intratumoral heterogeneity. The spatial heterogeneity of tumors may arise from the non-uniform distribution of tumor cell subsets harboring distinct gene mutations within the entire tumor, or it could be attributed to the emergence of dominant clone populations formed by differentiation grades established among tumor stem cells occupying specific subregions (26,27). The molecular reconstruction reveals that lymph node and distant metastases predominantly originate from independent subclones within the primary tumor (28,29). Repeating biopsy can confirm the spatiotemporal heterogeneity within tumors. However, relying solely on limited tissue samples for pathological analysis may not comprehensively elucidate the intratumor heterogeneity (30). Habitat analysis, also referred to as habitat imaging, offers the potential for non-invasively identifying tumor heterogeneity by accurately segmenting the tumor and its surrounding area into subregions that faithfully represent the diverse characteristics of the tumor (31-33). A study highlighted that habitat analysis enables the discrimination of tumor growth patterns and microvessel density within corresponding subregions (34). Using habitat analysis based on pre-treatment MRI for quantifying intratumoral heterogeneity has demonstrated favorable performance in predicting pathological complete response (pCR) to neoadjuvant therapy among breast cancer patients (35). Furthermore, the clustering parameters derived from subregions of habitat analysis based on DCE-MRI demonstrated their predictive potential for BReast CAncer gene 1 (BRCA1) mutation (36). However, there is currently no literature reporting on the utilization of DCE-MRI-based habitat analysis for predicting ALNM in breast cancer patients.
In this study, we used clinicopathological features, intra- and peri-tumoral radiomics features, and habitat features extracted from DCE-MRI to predict ALNM in breast cancer patients and compare their performances. This study aims to assess the value of DCE-MRI-based habitat analysis in predicting ALNM of breast cancer and determine the optimal peritumoral region size for accurate ALNM prediction of breast cancer. This study will provide insights for future investigations into habitat imaging and peritumoral radiomics. Furthermore, the findings of this study will be helpful for clinical decision-making and contribute to reducing unnecessary axillary invasive procedures in breast cancer patients. We present this article in accordance with the TRIPOD reporting checklist (available at https://qims.amegroups.com/article/view/10.21037/qims-24-558/rc).
Methods
Patients
The study was conducted in accordance with the Declaration of Helsinki (as revised in 2013). The present retrospective study was approved by the Institutional Ethics Committee of Shenzhen Yantian District People’s Hospital (No. 20210315001), and individual consent for this retrospective analysis was waived. A comprehensive retrospective search was conducted across clinical, pathological, and radiological databases at the Shenzhen People’s Hospital to enroll breast cancer patients between June 2019 and August 2021.
The inclusion criteria were: (I) female patients with a histopathological diagnosis of breast cancer, (II) patients who underwent preoperative bilateral breast MRI, and (III) patients who underwent axillary lymph node dissection (ALND) or sentinel lymph node biopsy (SLNB) for lymph node metastasis assessment. The exclusion criteria were: (I) history of prior breast surgery or chemotherapy before MRI examination, (II) missing or poor quality of MRI images.
The clinicopathological data were directly obtained from the medical record system and pathological reports. The collected clinicopathological information included age, multifocality, histopathological grade, lymphovascular invasion (LVI), estrogen receptor (ER) status, progesterone receptor (PR) status, human epidermal growth factor receptor 2 (HER-2) status, Ki67 expression level as determined by proliferation marker protein Ki67 staining, and lymph node involvement (axillary or SLNs). A cut-off point of 20% was applied to differentiate between low and high Ki67 expression levels in accordance with the St. Gallen 2015 recommendation (37).
In this retrospective study, 426 breast cancer patients were included. Patients were randomly assigned to the training set (n=338) and the test set (n=88) at a ratio of 4:1. The clinicopathological characteristics of the breast cancer patients in both the training and test sets are presented in Table 1. The flowchart depicting the patient recruitment process employed in this study is presented in Figure S1 in Appendix 1.
Table 1
Characteristics | Training set (n=338) | Test set (n=88) | |||||
---|---|---|---|---|---|---|---|
Non-metastasis (n=194) | Metastasis (n=144) | P value | Non-metastasis (n=51) | Metastasis (n=37) | P value | ||
Age (years), mean ± SD | 50.65±10.97 | 48.35±10.11 | 0.088 | 49.06±9.54 | 47.03±9.87 | 0.304 | |
Multifocality, n (%) | 0.004 | 0.610 | |||||
Negative | 175 (90.21) | 113 (78.47) | 47 (92.16) | 32 (86.49) | |||
Positive | 19 (9.79) | 31 (21.53) | 4 (7.84) | 5 (13.51) | |||
Histological grade, n (%) | <0.001 | 0.021 | |||||
1 | 25 (12.89) | 3 (2.08) | 9 (17.65) | – | |||
2 | 122 (62.89) | 121 (84.03) | 34 (66.67) | 32 (86.49) | |||
3 | 47 (24.23) | 20 (13.89) | 8 (15.69) | 5 (13.51) | |||
LVI, n (%) | 0.002 | 0.030 | |||||
Negative | 174 (89.69) | 110 (76.39) | 48 (94.12) | 28 (75.68) | |||
Positive | 20 (10.31) | 34 (23.61) | 3 (5.88) | 9 (24.32) | |||
ER status, n (%) | 1.000 | 0.341 | |||||
Negative | 47 (24.23) | 35 (24.31) | 6 (11.76) | 8 (21.62) | |||
Positive | 147 (75.77) | 109 (75.69) | 45 (88.24) | 29 (78.38) | |||
PR status, n (%) | 0.635 | 0.378 | |||||
Negative | 36 (18.56) | 23 (15.97) | 4 (7.84) | 6 (16.22) | |||
Positive | 158 (81.44) | 121 (84.03) | 47 (92.16) | 31 (83.78) | |||
HER-2 status, n (%) | 0.102 | 0.378 | |||||
Negative | 32 (16.49) | 14 (9.72) | 4 (7.84) | 6 (16.22) | |||
Positive | 162 (83.51) | 130 (90.28) | 47 (92.16) | 31 (83.78) | |||
Ki67, n (%) | 0.012 | 0.036 | |||||
Negative | 98 (50.52) | 52 (36.11) | 32 (62.75) | 14 (37.84) | |||
Positive | 96 (49.48) | 92 (63.89) | 19 (37.25) | 23 (62.16) |
SD, standard deviation; LVI, lymphovascular invasion; ER, estrogen receptor; PR, progesterone receptor; HER-2, human epidermal growth factor receptor 2; Ki67, proliferation marker protein Ki67.
MR image acquisition and image preprocessing
The MRI acquisition parameters are presented in Section S1 in Appendix 1. The third post-contrast MR image was utilized for analysis. Image preprocessing involved intensity standardization and spatial normalization, as described in Section S2 of Appendix 1. The comprehensive workflow employed in this study is depicted in Figure 1.
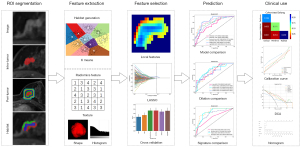
Tumor segmentation and peritumoral region generation
Two experienced radiologists (P.Q.W. and J.W., with 8 and 10 years of experience in MRI diagnosis of breast disease) utilized the 3DSlicer software (version 4.11.20200930) to perform precise tumor segmentation on the third post-contrast MR images without knowing the pathological results. The ROI for breast cancer was meticulously delineated layer by layer, enabling the acquisition of a comprehensive three-dimensional volume of interest (VOI) that accurately represents the entirety of the tumor. For breast cancer patients with multiple lesions, the largest lesion was selected for delineation and subsequent analysis. To comprehensively evaluate the predictive capabilities of the peritumoral regions, we expanded the ROI mask layer by layer at various radial distances on the OnekeyAI platform. The generated peritumoral regions, employing dilation intervals of 2 mm, are visually illustrated in Figure 2.
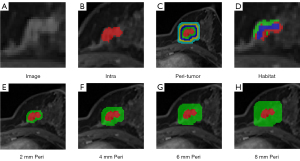
Feature extraction
In this study, handcrafted features were extracted from intra- and peri-tumoral regions and classified into three categories: (I) geometry, (II) intensity, and (III) texture (38). A total of 1,834 hand-crafted features were extracted from intratumoral and peritumoral regions, respectively, comprising 14 geometry features, 360 intensity features, and 1,460 texture features. The proportion of each group of handcrafted features is illustrated in Figure S2. The details of extracted radiomics features are shown in Section S3 in Appendix 1.
Regarding habitat features, local attributes were extracted from the three-dimensional (3D) VOI, encompassing local entropy and energy values. Subsequently, these attributes were amalgamated to form feature vectors, capturing distinct facets of each voxel’s attributes. To compute local attributes, a sliding window with dimensions of 3×3×3 was applied to each voxel within the MRI images, generating a 77-dimensional feature vector for each voxel. Following this, the K-means method was employed to cluster three subregions to avoid introducing excessive parameters that could lead to complexity (39). As demonstrated in Figure 3, the maximum feature value for each subregion was calculated to represent the final characteristics.
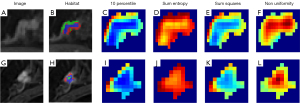
All features were extracted using the PyRadiomics tool (version 3.0.1; https://pyradiomics.readthedocs.io), with a majority aligning with the feature definitions outlined by the Imaging Biomarker Standardization Initiative (IBSI).
Feature selection
The test-retest and inter-rater analysis were used to assess segmentation uncertainty and ensure feature robustness. Features from multiple-segmented subregions were evaluated using the intraclass correlation coefficient (ICC). Features with an ICC ≥0.85 were included in the subsequent analysis. Following ICC screening, features were normalized using Z-scores for normal distribution. The P values for imaging features were calculated using a t-test, and only radiomic features with a P value <0.05 were retained. Pearson’s correlation coefficient was calculated to identify highly correlated features (exceeding 0.9). Subsequently, a recursive deletion strategy was employed to filter the features by iteratively removing the most redundant one at each step. The least absolute shrinkage and selection operator (LASSO) regression selected the final feature set for the radiomic signature (details presented in Section S4 in Appendix 1).
Model construction
Intratumoral radiomics signature (Intra)
Following LASSO feature screening, the finalized features underwent machine learning methods to establish the radiomics signature. The ALNM prediction model was formulated by employing six commonly utilized machine learning models: the support vector machine (SVM), K-nearest neighbors (KNN), RandomForest, XGBoost, LightGBM, and ExtraTrees. The specific hyperparameters for each model are presented in Section S5 in Appendix 1. Once the modeling was completed, a best-fit model was selected from the six constructed models for the subsequent analysis (Section S5 in Appendix 1).
Peritumoral radiomics signature (PeriXmm)
Here, ‘X’ denotes the peritumoral region of breast cancer in mm. Features extracted from the peritumoral areas of breast cancer were selected using the same feature selection process employed for the Intra Radiomics Signature, and the ultimate model was constructed using the identical machine learning algorithm. Similarly, an optimal model was selected for each specific peritumoral region.
Habitat signature (Habitat)
Given the reliance on unsupervised clustering algorithms for characterizing the internal tumor habitat, the mean feature values were computed. Furthermore, due to the unsupervised nature of clustering, the ICC assessment was omitted from the feature selection process of Habitat Signature. However, other configurations aligned with those of Intra and Peri models.
Clinical model and Fusion nomogram model
The Clinical models were constructed using the same machine learning algorithm for the Intra Radiomics Signature, while the Fusion nomogram model was developed employing a logistic regression algorithm.
Statistical analysis
Univariate analysis calculates the odds ratios (ORs) and corresponding P values for all clinicopathological features. The χ2 test was applied for categorical variables, whereas the independent samples t-test was used for continuous variables within a binary grouping. In addition to univariate analyses, stepwise multivariate analyses were performed on all clinical features. A correlation matrix was formed employing Pearson correlation analysis to facilitate the interpretation of correlations of the significant variables with ALNM. For the construction of the Clinical model, features with a P value <0.05 from the multivariable analysis were selected.
The diagnostic performance of each model was appraised in the test set via receiver operating characteristic (ROC) curves. The confidence interval (CI) uses 95% confidence. Additionally, we calculated the sensitivity, specificity, accuracy, positive predictive value (PPV), and negative predictive value (NPV) for each model. Calibration curves were generated to gauge calibration accuracy, and the Hosmer-Lemeshow (HL) goodness-of-fit test was employed to assess calibration ability. The net reclassification improvement (NRI) and integrated discrimination improvement (IDI) metrics were calculated to examine the incremental predictive value of the Fusion nomogram model for predicting ALNM. Additionally, decision curve analysis (DCA) was executed to evaluate the clinical utility of the predictive models.
Results
Patient characteristics
The study population was composed of 426 female patients, with ages ranging from 41 to 81 years old (mean, 48.9 years). The flowchart depicting the patient recruitment process employed in this study is presented in Figure S1 in Appendix 1. Among these patients, invasive ductal carcinoma of the breast was diagnosed in 360 patients, ductal carcinoma in situ in 43 patients, invasive lobular carcinoma in 13 patients, mucinous adenocarcinoma in 5 patients, intraductal papillary carcinoma in 2 patients, solid papillary carcinoma in 2 patients, and adenoid cystic carcinoma in 1 patient. Patients were randomly assigned to the training set (n=338) and the test set (n=88) at a ratio of 4:1. The clinicopathological characteristics of the breast cancer patients in both the training and test sets are presented in Table 1.
Clinicopathological features associated with ALNM
Univariable analysis showed that Ki67 [OR, 1.153 (95% CI: 1.055–1.260); P=0.008], multifocality [OR, 1.256 (95% CI: 1.110–1.420); P=0.003], and LVI [OR, 1.274 (95% CI: 1.131–1.436); P=0.001] were associated with ALNM in the training set (Table S1 in Appendix 1).
Multivariable analysis demonstrated that Ki67, multifocality, and LVI were independent predictors for ALNM (P<0.05) and thus were selected to construct the nomogram. The results of univariable and multivariable analyses on the clinicopathological features are presented in Figure 4.
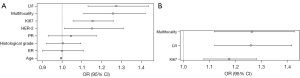
The correlation matrix, depicted in Figure S3 in Appendix 1, illustrates the interrelationships among distinct clinical features. The matrix reveals a positive correlation between Ki67, multifocality, and LVI and the metastasis task.
Features selection and model construction
By employing the LASSO algorithm, the Intra model identified 12 features, the Peri2mm model selected 12 features, the Peri4mm model extracted 15 features, the Peri6mm model recognized 18 features, the Peri8mm model pinpointed 15 features, and the Habitat model identified four features in our experiment. These selected features were then used to construct the habitat-based radiomics signature. The results of the LASSO 10-fold cross-validation are presented in Figure S4 in Appendix 1.
The development of 42 models was conducted using six algorithms to individually model clinical features, intratumoral features, peritumoral features within four specific ranges, and habitat features. Additionally, a fusion nomogram was constructed utilizing the logistic regression algorithm. Consequently, a total of 43 models were ultimately established. Ultimately, eight models were selected from the 43 constructed models for subsequent analysis: (I) clinicopathological features (Clinical model) employing the ExtraTrees algorithms; (II) intratumoral radiomic signatures (Intra model) employing the ExtraTrees algorithm; (III) peritumoral radiomic signatures of 2mm dilation (Peri2mm model) employing the LightGBM algorithm; (IV) peritumoral radiomic signatures of 4mm dilation (Peri4mm model) employing the XGBoost algorithm; (V) peritumoral radiomic signatures of 6mm dilation (Peri6mm model) employing RandomForest algorithm; (VI) peritumoral radiomic signatures of 8mm dilation (Peri8mm model) employing the ExtraTrees algorithm; (VII) habitat signatures (Habitat model) employing the XGBoost algorithm; (VIII) clinicopathological features, peritumoral radiomic signatures (choose the best one from 2–8 mm), and habitat signatures (Fusion nomogram model) employing the logistic regression algorithm.
Evaluation results of the prediction models
Intratumoral radiomic model vs. peritumoral radiomic model
The Peri4mm model demonstrates the highest AUC value among the Intra, Peri2mm, Peri6mm, and Peri8mm models while exhibiting minimal over-fitting. For discriminating ALNM, the Peri4mm model achieved AUCs of 0.871 (95% CI: 0.834–0.908) and 0.773 (95% CI: 0.672–0.873) in the training set and test set, respectively, while the Intra model achieved AUCs of 0.750 (95% CI: 0.699–0.802) and 0.745 (95% CI: 0.643–0.847) in the training set and test set, respectively (Figure 5). Other diagnostic metrics for the intra- and peri-tumoral radiomic models are shown in Table S2 in Appendix 1, including sensitivity, specificity, accuracy, PPV, and NPV. The results suggest that the radiomic signature derived from peritumoral regions with a dilation distance of 4 mm exhibited the most promising predictive performance. Consequently, we selected Peri4mm in the subsequent comparison of signatures and construction of the nomogram.
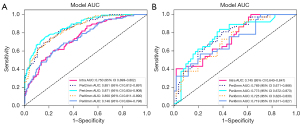
Habitat model vs. fusion nomogram
Compared to the Clinical model and Peri4mm model, the Habitat model exhibited superior diagnostic efficiency, with an AUC of 0.973 (95% CI: 0.959–0.987) in the training set and 0.854 (95% CI: 0.778–0.931) in the test set (Figure 6). Furthermore, by integrating clinicopathological signatures with the Peri4mm signatures and Habitat signatures, the fusion nomogram achieved improved predictive performance, with AUCs of 0.977 (95% CI: 0.965–0.989) and 0.873 (95% CI: 0.802–0.945) in the training set and test set, respectively. Other diagnostic metrics for the models, including sensitivity, specificity, accuracy, PPV, and NPV, are presented in Table S3 in Appendix 1. These results highlight the predictive power of the Nomogram and the Habitat signature, with narrow confidence intervals providing reliable performance estimates.
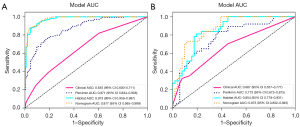
Calibration curve analysis
The calibration curve of the Fusion nomogram model exhibited satisfactory consistency in the test set (Figure 7), with a HL test statistic of 0.406. The HL test statistics for each signature are in Table S4 in Appendix 1.
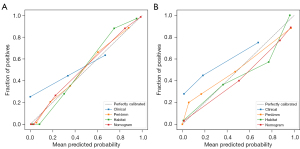
Accuracy improvement evaluation
The comparative assessment of the NRI and IDI metrics indicates that the Fusion nomogram model outperformed any individual signature (Figure S5 in Appendix 1). Notably, the Habitat signature displays improvements over both Peri4mm and clinical information.
DCA
The Fusion nomogram model yields noticeable benefits based on the predicted probabilities (Figure 8). Additionally, compared to other signatures, the Fusion nomogram model exhibits a larger potential for obtaining net benefit.
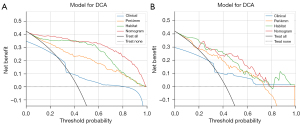
Fusion nomogram for clinical use
A radiomic nomogram for predicting ALNM in breast cancer patients was developed by integrating clinicopathological signatures, peritumoral radiomic signatures, and habitat signatures. The nomogram, which facilitates clinical utilization, is illustrated in Figure 9.
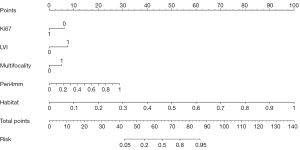
Discussion
The present study developed and validated a DCE-MRI-based habitat radiomics nomogram model to accurately predict ALNM in breast cancer patients. Notably, a peritumoral radiomics range of 4 mm was determined as optimal for ALNM prediction. The nomogram, which integrated clinicopathological signatures, peritumoral radiomic signatures within a 4 mm radius, and habitat signatures, exhibited superior diagnostic performance compared to other established models in our study. These findings have significant implications for guiding personalized treatment plans in clinical practice. This study stood out from previous radiomic studies because we not only used conventional intratumoral radiomic features but also used intratumoral habitat features and peritumoral radiomic features for more robustly interpreting intratumor heterogeneity and tumor microenvironment.
The present study reveals that multifocality, LVI, and Ki67 expression are significantly associated with ALNM in breast cancer patients, consistent with previous findings reported in the literature (40-42). The intratumoral radiomics model based on DCE-RMI in our study demonstrated a moderate predictive capability, as indicated by the AUC values of 0.750 and 0.745 in the training and test sets, respectively. Notably, the peritumoral model exhibited superior predictive performance compared to the intratumoral radiomics model, with Peri4mm achieving the highest AUC (0.871 and 0.773 in the training set and test set, respectively). The research findings suggest that extracting imaging features from peritumoral tissue can effectively capture tumor microenvironment information, thereby enhancing the ability to evaluate tumor biological behavior, such as lymph node metastasis. Previous studies have revealed that the combined utilization of intratumoral and peritumoral radiomics based on DCE-MRI enhances the precision in predicting ALNM among breast cancer patients. However, these studies have exhibited substantial variability in their selection of peritumoral range, ranging from 4 to 8 mm (25,43-45). The optimal range of peritumoral size also varies in other prediction tasks for breast cancer. This variability suggests that peritumoral range should be carefully considered based on specific prediction tasks. For example, the radiomics features extracted from the 3-mm peritumoral region based on X-ray images have demonstrated superior performance in distinguishing between benign and malignant breast lesions, surpassing both the 1-mm and 5-mm peritumoral ranges, as revealed by a recent study (46). Another study based on contrast-enhanced spectral mammography demonstrated that the intratumoral combined with a 5-mm peritumoral radiomics model exhibited superior predictive capability for neoadjuvant chemotherapy response in breast cancer patients, compared to both the intratumoral model and the intratumoral combined with a 10-mm peritumoral model (47).
Additionally, it is noteworthy that in the training set, both the sensitivity and NPV of the peritumoral radiomics model exhibited a decline as the peritumoral size increased. We hypothesize that this could be attributed to an accompanying increase in normal breast glands and/or adipose tissue within the expanding peritumoral area, consequently reducing predictive performance. However, this observed trend has yet to be replicated in either the validation set of our study or previous studies. Further investigation is warranted to elucidate the underlying factors contributing to this phenomenon.
The present study also highlights another significant finding that accurate prediction of ALNM in breast cancer can be achieved by employing habitat analysis of DCE-MRI, resulting in an impressive AUC value of 0.973 and 0.854 for the training set and validation set, respectively. Our ALNM prediction model based on habitat features significantly outperformed the intra- and peri-tumoral radiomics models regarding predictive efficacy. No other study has reported this result before. Habitat analysis technology, as a novel approach for investigating tumor heterogeneity, has been employed in some breast cancer research. For instance, Shi et al. (35) utilized habitat analysis to capture the heterogeneity of lesions on MRI, enabling the stratification of breast cancer patients into pCR and non-pCR groups based on treatment response. Furthermore, a model was developed using habitat features extracted from DCE-MRI to predict the presence of BRCA1 mutation in breast cancer patients (36). These studies highlight the potential of habitat analysis methods for predicting biological behavior and genetic biomarkers for breast cancer.
Different from conventional radiomics studies, we divided the tumor into three subregions to capture and characterize its spatial heterogeneity. The 3D segmentation method guarantees the spatial integrity of each subregion. It avoids the segmentation imbalance and partial loss of the overall structural features of the tumor due to the selection of the largest cross-section. The current study employed the K-means method, an unsupervised and unstructured partition clustering technique based on the distance minimization criterion, to achieve division by clustering the sub-regions (48). The K-means clustering method is widely utilized in habitat analysis by researchers due to its capability of effectively categorizing pixels with similar performance on each MRI images into distinct classes while remaining unaffected by spatial distribution (35,36,49). Currently, it is acknowledged that the tumor subregions identified through habitat analysis are intricately associated with the heterogeneity of tumors. The subregions generated by habitat analysis are a manifestation of the differences in environmental selection and cell fitness within the tumor (50). Distinct cellular populations and variations in blood perfusion have been observed across different subregions (51). This could potentially explain the exceptional predictive performance of the habitat analysis model established in this study.
Additionally, we explored whether an integrated model incorporating clinicopathologic factors, peritumoral radiomic signatures, and habitat signatures would surpass the performance of the signature alone. The findings demonstrated that the integrated model yielded optimal predictive performance for ALNM in breast cancer. The specificity and accuracy of the training set in the nomogram model were higher than those of other models. Still, the sensitivity and NPV were lower than those of the habitat analysis model, which may be related to the small number of cases included in the model, the collinearity between the models and the random grouping.
There are several limitations associated with this study. First, the data were collected retrospectively at one center in this study. The findings need to be validated in a more extensive and multicenter dataset to achieve high-level evidence. Furthermore, a prospective study is imperative to further validate the findings. Second, including only one MRI sequence (i.e., DCE-MRI) may not be enough to interpret intratumor heterogeneity and tumor microenvironment robustly, so future studies may utilize more MRI sequences (such as diffusion-weighted images) or even other modal images (like ultrasound) to conduct the research. In addition, segmenting DCE-MRI images requires manual intervention in this study, which is time-consuming.
Conclusions
In summary, we successfully identified the optimal size of the peritumoral region based on DCE-MRI radiomics for predicting ALNM in breast cancer patients to be 4 mm. And the nomogram developed and validated in this study, which combines clinicopathological factors, Peri4mm radiomic and habitat signatures derived from DCE-MRI achieved the best performance for predicting ALNM in breast cancer patients. These findings have significant implications for radiomics studies and may benefit clinical decision-making for breast cancer patients.
Acknowledgments
Funding: This study was supported by
Footnote
Reporting Checklist: The authors have completed the TRIPOD reporting checklist. Available at https://qims.amegroups.com/article/view/10.21037/qims-24-558/rc
Conflicts of Interest: All authors have completed the ICMJE uniform disclosure form (available at https://qims.amegroups.com/article/view/10.21037/qims-24-558/coif). P.Q.W. reports that this research was funded by the Guangdong Medical Science and Technology Research Fund Project (grant No. B2022071), and the Shenzhen Science and Technology Program (grant No. JCYJ20210324132809023). Y.B.L. reports that this research was funded by the National Natural Science Foundation of China (grant No. 82471941), the National High-Performance Medical Device Innovation Center Open Fund Project (grant No. NMED2021MS-01-003), and the Shenzhen Science and Technology Program (grant No. JCYJ20210324125403011). J.M. reports that this research was funded by the Shenzhen Science and Technology Research and Development Funds (grant Nos. GJHZ20210705142208024 & GJHZ20220913142613025). The other authors have no conflicts of interest to declare.
Ethical Statement: The authors are accountable for all aspects of the work in ensuring that questions related to the accuracy or integrity of any part of the work are appropriately investigated and resolved. The study was conducted in accordance with the Declaration of Helsinki (as revised in 2013). The study was approved by the Institutional Ethics Committee of Shenzhen Yantian District People’s Hospital (No. 20210315001), and individual consent for this retrospective analysis was waived.
Open Access Statement: This is an Open Access article distributed in accordance with the Creative Commons Attribution-NonCommercial-NoDerivs 4.0 International License (CC BY-NC-ND 4.0), which permits the non-commercial replication and distribution of the article with the strict proviso that no changes or edits are made and the original work is properly cited (including links to both the formal publication through the relevant DOI and the license). See: https://creativecommons.org/licenses/by-nc-nd/4.0/.
References
- Sung H, Ferlay J, Siegel RL, Laversanne M, Soerjomataram I, Jemal A, Bray F. Global Cancer Statistics 2020: GLOBOCAN Estimates of Incidence and Mortality Worldwide for 36 Cancers in 185 Countries. CA Cancer J Clin 2021;71:209-49. [Crossref] [PubMed]
- Miller KD, Nogueira L, Devasia T, Mariotto AB, Yabroff KR, Jemal A, Kramer J, Siegel RL. Cancer treatment and survivorship statistics, 2022. CA Cancer J Clin 2022;72:409-36. [Crossref] [PubMed]
- Matikas A, Kotsakis A, Apostolaki S, Politaki H, Perraki M, Kalbakis K, Nikolaou M, Economopoulou P, Hatzidaki D, Georgoulias V. Detection of circulating tumour cells before and following adjuvant chemotherapy and long-term prognosis of early breast cancer. Br J Cancer 2022;126:1563-9. [Crossref] [PubMed]
- Li Z, Gao Y, Gong H, Feng W, Ma Q, Li J, Lu X, Wang X, Lei J. Different Imaging Modalities for the Diagnosis of Axillary Lymph Node Metastases in Breast Cancer: A Systematic Review and Network Meta-Analysis of Diagnostic Test Accuracy. J Magn Reson Imaging 2023;57:1392-403. [Crossref] [PubMed]
- Zhang X, Liu Y, Luo H, Zhang J. PET/CT and MRI for Identifying Axillary Lymph Node Metastases in Breast Cancer Patients: Systematic Review and Meta-Analysis. J Magn Reson Imaging 2020;52:1840-51. [Crossref] [PubMed]
- Zhao M, Wu Q, Guo L, Zhou L, Fu K. Magnetic resonance imaging features for predicting axillary lymph node metastasis in patients with breast cancer. Eur J Radiol 2020;129:109093. [Crossref] [PubMed]
- Lambin P, Leijenaar RTH, Deist TM, Peerlings J, de Jong EEC, van Timmeren J, Sanduleanu S, Larue RTHM, Even AJG, Jochems A, van Wijk Y, Woodruff H, van Soest J, Lustberg T, Roelofs E, van Elmpt W, Dekker A, Mottaghy FM, Wildberger JE, Walsh S. Radiomics: the bridge between medical imaging and personalized medicine. Nat Rev Clin Oncol 2017;14:749-62. [Crossref] [PubMed]
- Liu Z, Wang S, Dong D, Wei J, Fang C, Zhou X, Sun K, Li L, Li B, Wang M, Tian J. The Applications of Radiomics in Precision Diagnosis and Treatment of Oncology: Opportunities and Challenges. Theranostics 2019;9:1303-22. [Crossref] [PubMed]
- Chai R, Ma H, Xu M, Arefan D, Cui X, Liu Y, Zhang L, Wu S, Xu K. Differentiating axillary lymph node metastasis in invasive breast cancer patients: A comparison of radiomic signatures from multiparametric breast MR sequences. J Magn Reson Imaging 2019;50:1125-32. [Crossref] [PubMed]
- Liu J, Sun D, Chen L, Fang Z, Song W, Guo D, Ni T, Liu C, Feng L, Xia Y, Zhang X, Li C. Radiomics Analysis of Dynamic Contrast-Enhanced Magnetic Resonance Imaging for the Prediction of Sentinel Lymph Node Metastasis in Breast Cancer. Front Oncol 2019;9:980. [Crossref] [PubMed]
- Dong Y, Feng Q, Yang W, Lu Z, Deng C, Zhang L, Lian Z, Liu J, Luo X, Pei S, Mo X, Huang W, Liang C, Zhang B, Zhang S. Preoperative prediction of sentinel lymph node metastasis in breast cancer based on radiomics of T2-weighted fat-suppression and diffusion-weighted MRI. Eur Radiol 2018;28:582-91. [Crossref] [PubMed]
- Yu Y, Tan Y, Xie C, Hu Q, Ouyang J, Chen Y, et al. Development and Validation of a Preoperative Magnetic Resonance Imaging Radiomics-Based Signature to Predict Axillary Lymph Node Metastasis and Disease-Free Survival in Patients With Early-Stage Breast Cancer. JAMA Netw Open 2020;3:e2028086. [Crossref] [PubMed]
- Li M, Zhang J, Dan Y, Yao Y, Dai W, Cai G, Yang G, Tong T. A clinical-radiomics nomogram for the preoperative prediction of lymph node metastasis in colorectal cancer. J Transl Med 2020;18:46. [Crossref] [PubMed]
- Thomas PA. Development of radiomics models to predict lymph node metastasis and de-escalated non-small-cell lung cancer surgery: a word of caution. Eur J Cardiothorac Surg 2021;60:72-3. [Crossref] [PubMed]
- Liang C, Cheng Z, Huang Y, He L, Chen X, Ma Z, Huang X, Liang C, Liu Z. An MRI-based Radiomics Classifier for Preoperative Prediction of Ki-67 Status in Breast Cancer. Acad Radiol 2018;25:1111-7. [Crossref] [PubMed]
- Han L, Zhu Y, Liu Z, Yu T, He C, Jiang W, Kan Y, Dong D, Tian J, Luo Y. Radiomic nomogram for prediction of axillary lymph node metastasis in breast cancer. Eur Radiol 2019;29:3820-9. [Crossref] [PubMed]
- Gao W, Chen J, Zhang B, Wei X, Zhong J, Li X, He X, Zhao F, Chen X. Automatic deep learning method for detection and classification of breast lesions in dynamic contrast-enhanced magnetic resonance imaging. Quant Imaging Med Surg 2023;13:2620-33. [Crossref] [PubMed]
- Xu Q, Chen S, Hu Y, Huang W. Landscape of Immune Microenvironment Under Immune Cell Infiltration Pattern in Breast Cancer. Front Immunol 2021;12:711433. [Crossref] [PubMed]
- Yu H, Meng X, Chen H, Han X, Fan J, Gao W, Du L, Chen Y, Wang Y, Liu X, Zhang L, Ma G, Yang J. Correlation Between Mammographic Radiomics Features and the Level of Tumor-Infiltrating Lymphocytes in Patients With Triple-Negative Breast Cancer. Front Oncol 2020;10:412. [Crossref] [PubMed]
- Takada K, Kashiwagi S, Asano Y, Goto W, Kouhashi R, Yabumoto A, Morisaki T, Shibutani M, Takashima T, Fujita H, Hirakawa K, Ohira M. Prediction of lymph node metastasis by tumor-infiltrating lymphocytes in T1 breast cancer. BMC Cancer 2020;20:598. [Crossref] [PubMed]
- Zhang S, Zhang D, Yi S, Gong M, Lu C, Cai Y, Tang X, Zou L. The relationship of lymphatic vessel density, lymphovascular invasion, and lymph node metastasis in breast cancer: a systematic review and meta-analysis. Oncotarget 2017;8:2863-73. [Crossref] [PubMed]
- Sethy C, Goutam K, Das B, Dash SR, Kundu CN. Nectin-4 promotes lymphangiogenesis and lymphatic metastasis in breast cancer by regulating CXCR4-LYVE-1 axis. Vascul Pharmacol 2021;140:106865. [Crossref] [PubMed]
- Braman N, Prasanna P, Whitney J, Singh S, Beig N, Etesami M, Bates DDB, Gallagher K, Bloch BN, Vulchi M, Turk P, Bera K, Abraham J, Sikov WM, Somlo G, Harris LN, Gilmore H, Plecha D, Varadan V, Madabhushi A. Association of Peritumoral Radiomics With Tumor Biology and Pathologic Response to Preoperative Targeted Therapy for HER2 (ERBB2)-Positive Breast Cancer. JAMA Netw Open 2019;2:e192561. [Crossref] [PubMed]
- Liu C, Ding J, Spuhler K, Gao Y, Serrano Sosa M, Moriarty M, Hussain S, He X, Liang C, Huang C. Preoperative prediction of sentinel lymph node metastasis in breast cancer by radiomic signatures from dynamic contrast-enhanced MRI. J Magn Reson Imaging 2019;49:131-40. [Crossref] [PubMed]
- Ding J, Chen S, Serrano Sosa M, Cattell R, Lei L, Sun J, Prasanna P, Liu C, Huang C. Optimizing the Peritumoral Region Size in Radiomics Analysis for Sentinel Lymph Node Status Prediction in Breast Cancer. Acad Radiol 2022;29:S223-S8. [Crossref] [PubMed]
- Dagogo-Jack I, Shaw AT. Tumour heterogeneity and resistance to cancer therapies. Nat Rev Clin Oncol 2018;15:81-94. [Crossref] [PubMed]
- Prasetyanti PR, Medema JP. Intra-tumor heterogeneity from a cancer stem cell perspective. Mol Cancer 2017;16:41. [Crossref] [PubMed]
- Naxerova K, Reiter JG, Brachtel E, Lennerz JK, van de Wetering M, Rowan A, Cai T, Clevers H, Swanton C, Nowak MA, Elledge SJ, Jain RK. Origins of lymphatic and distant metastases in human colorectal cancer. Science 2017;357:55-60. [Crossref] [PubMed]
- Reiter JG, Hung WT, Lee IH, Nagpal S, Giunta P, Degner S, Liu G, Wassenaar ECE, Jeck WR, Taylor MS, Farahani AA, Marble HD, Knott S, Kranenburg O, Lennerz JK, Naxerova K. Lymph node metastases develop through a wider evolutionary bottleneck than distant metastases. Nat Genet 2020;52:692-700. [Crossref] [PubMed]
- Inda MA, van Swinderen P, van Brussel A, Moelans CB, Verhaegh W, van Zon H, den Biezen E, Bikker JW, van Diest PJ, van de Stolpe A. Heterogeneity in Signaling Pathway Activity within Primary and between Primary and Metastatic Breast Cancer. Cancers (Basel) 2021;13:1345. [Crossref] [PubMed]
- Mandair D, Reis-Filho JS, Ashworth A. Biological insights and novel biomarker discovery through deep learning approaches in breast cancer histopathology. NPJ Breast Cancer 2023;9:21. [Crossref] [PubMed]
- Gillies RJ, Balagurunathan Y, Perfusion MR. Imaging of Breast Cancer: Insights Using "Habitat Imaging". Radiology 2018;288:36-7. [Crossref] [PubMed]
- Feng S, Yin J. Dynamic contrast-enhanced magnetic resonance imaging radiomics analysis based on intratumoral subregions for predicting luminal and nonluminal breast cancer. Quant Imaging Med Surg 2023;13:6735-49. [Crossref] [PubMed]
- Han Y, Chai F, Wei J, Yue Y, Cheng J, Gu D, Zhang Y, Tong T, Sheng W, Hong N, Ye Y, Wang Y, Tian J. Identification of Predominant Histopathological Growth Patterns of Colorectal Liver Metastasis by Multi-Habitat and Multi-Sequence Based Radiomics Analysis. Front Oncol 2020;10:1363. [Crossref] [PubMed]
- Shi Z, Huang X, Cheng Z, Xu Z, Lin H, Liu C, Chen X, Liu C, Liang C, Lu C, Cui Y, Han C, Qu J, Shen J, Liu Z. MRI-based Quantification of Intratumoral Heterogeneity for Predicting Treatment Response to Neoadjuvant Chemotherapy in Breast Cancer. Radiology 2023;308:e222830. [Crossref] [PubMed]
- Du T, Zhao H. Habitat Analysis of Breast Cancer-Enhanced MRI Reflects BRCA1 Mutation Determined by Immunohistochemistry. Biomed Res Int 2022;2022:9623173. [Crossref] [PubMed]
- Coates AS, Winer EP, Goldhirsch A, Gelber RD, Gnant M, Piccart-Gebhart M, Thurlimann B, Senn HJ, Panel M. Tailoring therapies--improving the management of early breast cancer: St Gallen International Expert Consensus on the Primary Therapy of Early Breast Cancer 2015. Ann Oncol 2015;26:1533-46. [Crossref] [PubMed]
- van Griethuysen JJM, Fedorov A, Parmar C, Hosny A, Aucoin N, Narayan V, Beets-Tan RGH, Fillion-Robin JC, Pieper S, Aerts H. Computational Radiomics System to Decode the Radiographic Phenotype. Cancer Res 2017;77:e104-7. [Crossref] [PubMed]
- Jeong SY, Park JE, Kim N, Kim HS. Hypovascular Cellular Tumor in Primary Central Nervous System Lymphoma is Associated with Treatment Resistance: Tumor Habitat Analysis Using Physiologic MRI. AJNR Am J Neuroradiol 2022;43:40-7. [Crossref] [PubMed]
- Wu P, Zhao K, Liang Y, Ye W, Liu Z, Liang C. Validation of Breast Cancer Models for Predicting the Nonsentinel Lymph Node Metastasis After a Positive Sentinel Lymph Node Biopsy in a Chinese Population. Technol Cancer Res Treat 2018;17:1533033818785032. [Crossref] [PubMed]
- Van Zee KJ, Manasseh DM, Bevilacqua JL, Boolbol SK, Fey JV, Tan LK, Borgen PI, Cody HS 3rd, Kattan MW. A nomogram for predicting the likelihood of additional nodal metastases in breast cancer patients with a positive sentinel node biopsy. Ann Surg Oncol 2003;10:1140-51. [Crossref] [PubMed]
- Chen JY, Chen JJ, Yang BL, Liu ZB, Huang XY, Liu GY, Han QX, Yang WT, Shen ZZ, Shao ZM, Wu J. Predicting sentinel lymph node metastasis in a Chinese breast cancer population: assessment of an existing nomogram and a new predictive nomogram. Breast Cancer Res Treat 2012;135:839-48. [Crossref] [PubMed]
- Zhan C, Hu Y, Wang X, Liu H, Xia L, Ai T. Prediction of Axillary Lymph Node Metastasis in Breast Cancer using Intra-peritumoral Textural Transition Analysis based on Dynamic Contrast-enhanced Magnetic Resonance Imaging. Acad Radiol 2022;29:S107-15. [Crossref] [PubMed]
- Liu Y, Li X, Zhu L, Zhao Z, Wang T, Zhang X, Cai B, Li L, Ma M, Ma X, Ming J. Preoperative Prediction of Axillary Lymph Node Metastasis in Breast Cancer Based on Intratumoral and Peritumoral DCE-MRI Radiomics Nomogram. Contrast Media Mol Imaging 2022;2022:6729473. [Crossref] [PubMed]
- Zhang S, Wang X, Yang Z, Zhu Y, Zhao N, Li Y, He J, Sun H, Xie Z. Intra- and Peritumoral Radiomics Model Based on Early DCE-MRI for Preoperative Prediction of Molecular Subtypes in Invasive Ductal Breast Carcinoma: A Multitask Machine Learning Study. Front Oncol 2022;12:905551. [Crossref] [PubMed]
- Wang S, Sun Y, Li R, Mao N, Li Q, Jiang T, Chen Q, Duan S, Xie H, Gu Y. Diagnostic performance of perilesional radiomics analysis of contrast-enhanced mammography for the differentiation of benign and malignant breast lesions. Eur Radiol 2022;32:639-49. [Crossref] [PubMed]
- Mao N, Shi Y, Lian C, Wang Z, Zhang K, Xie H, Zhang H, Chen Q, Cheng G, Xu C, Dai Y. Intratumoral and peritumoral radiomics for preoperative prediction of neoadjuvant chemotherapy effect in breast cancer based on contrast-enhanced spectral mammography. Eur Radiol 2022;32:3207-19. [Crossref] [PubMed]
- Yang Y, Han Y, Zhao S, Xiao G, Guo L, Zhang X, Cui G. Spatial heterogeneity of edema region uncovers survival-relevant habitat of Glioblastoma. Eur J Radiol 2022;154:110423. [Crossref] [PubMed]
- Park JE, Kim HS, Kim N, Park SY, Kim YH, Kim JH. Spatiotemporal Heterogeneity in Multiparametric Physiologic MRI Is Associated with Patient Outcomes in IDH-Wildtype Glioblastoma. Clin Cancer Res 2021;27:237-45. [Crossref] [PubMed]
- Gatenby RA, Grove O, Gillies RJ. Quantitative imaging in cancer evolution and ecology. Radiology 2013;269:8-15. [Crossref] [PubMed]
- Syed AK, Whisenant JG, Barnes SL, Sorace AG, Yankeelov TE. Multiparametric Analysis of Longitudinal Quantitative MRI data to Identify Distinct Tumor Habitats in Preclinical Models of Breast Cancer. Cancers (Basel) 2020;12:1682. [Crossref] [PubMed]