Relationship between autism spectrum disorder and peripapillary intraretinal layer thickness: a pediatric retrospective cross-sectional study
Introduction
Autism spectrum disorder (ASD) is a profound neurodevelopmental condition characterized by enduring deficits in social communication and interaction, coupled with restricted, repetitive patterns of behavior, interests, or activities (1). The global prevalence of ASD has reached 1% and exhibits a persistent upward trajectory (2-4). The retina, serving as an extension of the central nervous system (CNS), shares structural and functional parallels with the brain. Specifically, the retinal plexiform and nerve fiber layers bear semblance to the white matter, while the nuclear and ganglion cell layers resemble the cerebral gray matter (GM) (5). Neurophysiologically, the retina and brain predominantly consist of neurons and glial cells, exhibiting akin interconnections among glial cells (6,7). Furthermore, the receptors for the main neurotransmitters of both the retina and brain are nearly identical (8,9). The retina and brain also share similar mechanisms in neuroimmune defence and response (10,11). In addition, both the retina and the brain necessitate analogous blood supply owing to comparable angiogenesis (12). The inner blood-retina barrier mirrors the structure, function, and mechanism of the blood-brain barrier (10,13). These shared characteristics position the retina as a promising avenue for ASD exploration.
As the starting point of the visual system, the retina is susceptible to changes of the body. Individuals with ASD commonly face challenges, manifesting as hypersensitivity or insensitivity to visual stimuli and reduced attention to social gaze and facial expressions (14-17). Previous electroretinogram (ERG) studies on ASD individuals have reported functional retinal changes in ASD (18,19). And a recent ERG-based research holds that sensory processing differences in ASD individuals are upstream of more complex autistic traits and potential intervention targets and report that the autistic retina displays hyper-responsive reaction to single white flashes, indicating altered retinal sensitivity in ASD individuals (20). These findings suggest that differences in retinal dynamics may correlate with the severity of autistic traits. Moreover, early visual abnormalities may significantly impact learning processes and contribute to the social and communicative development of infants (21). Therefore, the retina exploration in the context of ASD biomarker holds potential and may provide additional insights into ASD. This paper focuses on retinal structure changes near the optic disc, which serves as the gateway of the optic nerve to the brain and the inlet of the primary blood supply to the retina.
The unique advantage of the retina over other CNS structures lies in its direct observability and the retinal neurons are unmyelinated unlike the brain. While traditional imaging modalities, such as color fundus photographs and fundus fluorescein angiography, have been pivotal in ophthalmology, their two-dimensional (2D) limitations and potential adverse reactions due to dyes are still salient. Optical coherence tomography (OCT), utilizing low-coherence light, offers non-invasive, three-dimensional (3D) imaging with micro-scale resolution, emerging as a powerful tool for diagnosing, observing, and quantifying retinal diseases (22-24). In light of these advancements, our study utilizes OCT to investigate the relationship between intraretinal layer thickness and ASD.
To the best of our knowledge, only a limited number of studies have focused on alterations near the optic disc in autism (25-30). These studies have predominantly explored the peripapillary retinal nerve fiber layer (pRNFL), without sufficiently investigating other intraretinal layers. Additionally, as the study of retinal changes in autism is an emerging field, previous studies have exhibited considerable variability in sample sizes and age distributions and have not reached consistent results. Revisiting previous studies with a larger sample size, this study seeks to clarify the relationship between intraretinal layer thickness and ASD.
Notably, OCT imaging devices are primarily crafted for the examination of retinal diseases, prioritizing pRNFL measurements in optic disc related region due to their intimate connection with ocular conditions. This emphasis resulted in a predominant focus on RNFL in previous ASD studies conducted in this region. However, as the pathological mechanisms underlying ASD remain unclear, the exclusive association of observed changes with RNFL alterations remains uncertain. Therefore, it is imperative to comprehensively investigate all retinal sublayers within the peripapillary region. This study aims to conduct thorough global and quadrantal thickness analyses of all intraretinal layers within the peripapillary region, incorporating clinical parameters related to social functioning and cognitive level in exploratory experiments. We present this article in accordance with the STROBE reporting checklist (available at https://qims.amegroups.com/article/view/10.21037/qims-24-753/rc).
Methods
Participants
This cross-sectional study received approval from the Medical Ethics Committee of Nanjing Medical University (Approval ID: 2020-SR-363) and adhered to the principles of the Declaration of Helsinki (as revised in 2013). Recruitment transpired from December 2019 to August 2023 and encompassed both an ASD group and a neurotypical (NT) control group. ASD participants were sourced from the Specific Disease Cohort of ASD in the Children’s Mental Health Research Center, Nanjing Brain Hospital/The Affiliated Brain Hospital of Nanjing Medical University, Nanjing, China, while NT subjects were recruited from the local communities through the Department of Ophthalmology, Jiangsu Province Hospital/The First Affiliated Hospital of Nanjing Medical University, Nanjing, China. Informed consent was obtained from the participants’ parents or guardians. The enrollment process of participants is shown in Figure 1.
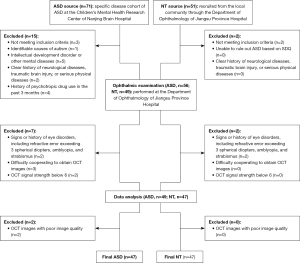
Inclusion criteria for the ASD group comprised: (I) a confirmed diagnosis of ASD based on DSM-5 (1) criteria was made by two deputy chief physicians or above in the Department of Child and Adolescent Psychiatry of Nanjing Brain Hospital; (II) age ranging from 7 to 13 years; (III) full-scale intelligence quotient (IQ) of Wechsler Intelligence Scale for Children, Fourth Edition (WISC-IV) (31) measurement ≥70; (IV) right-handedness; (V) the child and their parent/guardian agreed to participate in this study. Exclusion criteria for the ASD group included: (I) identifiable causes of autism; (II) intellectual development disorder or other mental diseases ; (III) a clear history of neurological diseases, traumatic brain injury, or serious physical diseases; (IV) a history of any psychotropic drugs in the past 3 months; (V) signs or history of eye disorders, including a refractive error exceeding 3 spherical diopters, amblyopia, and strabismus; (VI) difficulties in cooperating for obtaining high-quality OCT images without artifacts; and (VII) OCT signal strength below 6.
Inclusion criteria for the NT group comprised: (I) age ranging from 7 to 13 years; (II) the child and their parent/guardian agreed to participate in this study. Exclusion criteria for the NT group included: (I) the inability to rule out ASD based on Strengths and Difficulties Questionnaire (32); (II) a clear history of neurological diseases, traumatic brain injury, or serious physical diseases; (III) signs or history of eye disorders, including a refractive error exceeding 3 spherical diopters, amblyopia, and strabismus; (IV) difficulties in cooperating for obtaining high-quality OCT images without artifacts; and (V) OCT signal strength below 6.
All participants underwent a comprehensive ophthalmic examination, including autorefraction, intraocular pressure (IOP) measurement, slit lamp biomicroscopy, mydriasis funduscopy, OCT, conducted by experienced ophthalmologists. ASD participants underwent additional clinical psychological evaluations performed by professionally trained psychologists or psychiatrists in the Child Psychology Research Center of Nanjing Brain Hospital utilizing: (I) Autism Diagnostic Observation Schedule, Second Edition (ADOS-2) (33,34); (II) Autism Diagnoses Interview-Revised (ADI-R) (34,35); (III) the Chinese version of Social Responsiveness Scale (SRS) (36,37); and (IV) the Chinese version of WISC-IV (31).
OCT image acquisition and feature extraction
In this study, OCT images were acquired using a commercial 70-kHz SD-OCT system (RTVue XR Avanti, Optovue Inc., CA, USA) with a center wavelength of 840 nm, an acquisition rate of 70,000 A-scans per second, an axial resolution of 5 µm in tissue, and a lateral resolution of 11.25 µm. An OCT image centered on the disc with a field of view of 4.5×4.5 mm and a depth of approximately 1.92 mm was scanned. Each 3D OCT volume consisted of 400 B-scans, each of which included 400 A-scans with 640 pixels per A-scan, and each fixed location was scanned at least twice to ensure high-quality images (Figure 2A). Intraretinal layer thickness analysis encompassed different regions, including the global peripapillary region and 6 quadrantal subregions (Figure 2B): supero-temporal, supero-nasal, nasal, infero-nasal, infero-temporal, and temporal quadrants (ST, SN, N, IN, IT, and T). Intraretinal layer borders were automatically calculated by OCTExplorer software (v.3.8.0; The Iowa Institute for Biomedical Imaging, Iowa, USA) (38-40) and were utilized to measure the thickness of 10 retinal sublayers (Figure 2C) including the retinal nerve fiber layer (RNFL), ganglion cell layer (GCL), inner plexiform layer (IPL), inner nuclear layer (INL), outer plexiform layer (OPL), Henle fiber layer/outer nuclear layer/myoid zone (HOAM), ellipsoid zone (EZ), outer segment (OS), outer segment PR/RPE complex and subretinal virtual space (OPRS), and retinal pigment epithelium (RPE).
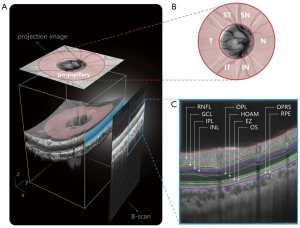
Statistical analysis
Statistical analysis was conducted using MATLAB (MathWorks, 2021). If data from both eyes of participants were available, the final parameters were averaged bilaterally. Otherwise, parameters from the included single eye were used. Data distribution was presented as the mean ± standard deviation (SD). The Shapiro-Wilk test was employed to assess the normality of values, while the two-sample F-test gauged homogeneity of variances. The unpaired t-test and Welch’s t-test were utilized for comparisons between continuous variables that satisfied normal distribution and those that only partially met the normal distribution, respectively. The Mann-Whitney U test was employed for comparisons where at least one group did not meet the normal distribution. The Chi-squared test was applied for non-continuous variables. Correlations between clinical parameters (total SRS scores and total IQ scores) and intraretinal layer thickness were determined using the Spearman correlation coefficient. A significance level of P<0.05 was considered statistically significant for the two-tailed test. Stepwise regression was employed to identify specific intraretinal layers that significantly contribute to the prediction of ASD clinical parameters. The thresholds selected were entry significance level less than 0.05 and removal significance level greater than 0.1.
Results
Participants
A total of 177 eyes from 94 participants were included in this study, including 47 ASD subjects (41 boys and 6 girls) and 47 age- and sex-matched NT subjects (mean age: 9.57±1.83 vs. 9.89±1.70 years). Twenty-three SRS scales of children with ASD were excluded due to parents’ irregular completion or completion by non-primary caregivers. Six children with ASD were uncooperative to give valid IQ and were excluded from IQ related statistics. Due to poor cooperation, 5 left eyes and 6 right eyes were excluded from the ASD group. Table 1 gives the demographics and clinical characteristics of included samples.
Table 1
Parameter | ASD (n=47) | NT (n=47) | P |
---|---|---|---|
Sex (male/female) | 41/6 | 41/6 | 1.000a |
Age (years) | 9.57±1.83 | 9.89±1.70 | 0.157b |
Laterality | 0.936a | ||
Left eye | 42 | 47 | |
Right eye | 41 | 47 | |
IQ | 98.34±22.91; NA =6 | – | – |
ADI-(SI+CO) | 30.10±4.60 | – | – |
ADI-Total | 37.49±4.98 | – | – |
ADOS-(S+C) | 13.34±4.45 | – | – |
ADOS-Total | 16.07±5.58 | – | – |
SRS-Aw | 16.38±2.95; NA =23 | – | – |
SRS-Cog | 29.33±7.94; NA =23 | – | – |
SRS-Com | 46.17±10.40; NA =23 | – | – |
SRS-Mo | 21.29±4.16; NA =23 | – | – |
SRS-Ma | 24.75±7.75; NA =23 | – | – |
SRS-Total | 137.83±29.70; NA =23 | – | – |
Data are presented as mean ± standard deviation or n. a, χ2 test for categorical variables; b, Mann-Whitney U test. NA indicates the number of unavailable data points. ASD, autism spectrum disorder; NT, neurotypical; IQ, score of full-scale intelligence quotient of the Chinese version of Wechsler Intelligence Scale for Children, Fourth Edition; ADI-(SI+CO), total score of Social Interaction and Communication Module in the Autism Diagnostic Interview-Revised; ADI-Total, total score of the Autism Diagnostic Interview-Revised; ADOS-(S+C), total score of combined social-communication domain in ADOS; ADOS-Total, total score of ADOS; ADOS, Autism Diagnostic Observation Schedule, Second Edition; SRS-Aw, score of the social awareness domain in SRS; SRS-Cog, score of social cognition domain in SRS; SRS-Com, score of social communication domain in SRS; SRS-Mo, score of social motivation domain in SRS; SRS-Ma, score of Autistic Mannerisms domain in SRS; SRS-Total, total score of SRS; SRS, Social Responsiveness Scale.
Intraretinal layer thickness differences between ASD and NT
Global thickness statistical analysis revealed that the thickness of GCL and INL in the ASD group was significantly higher compared to the control group (P=0.022 and P<0.001). Further quadrant analysis showed significant differences in the thickness of the IN, IT, and T quadrants of the GCL layer, as well as the N, IN, IT, and T quadrants of the INL layer, between the ASD and NT groups, with greater thickness observed in the ASD group as shown in Table 2.
Table 2
Layer | ASD | NT | P |
---|---|---|---|
RNFL | 130.53±16.36 | 127.11±13.43 | 0.106a |
GCL* | 14.15±1.30 | 13.71±1.67 | 0.022a |
ST | 11.86±2.23 | 11.68±2.01 | 0.700c |
SN | 12.89±2.83 | 12.73±2.62 | 0.774c |
N | 14.79±2.23 | 14.83±2.84 | 0.283a |
IN* | 12.48±1.76 | 11.86±2.11 | 0.035a |
IT* | 11.58±2.41 | 10.55±1.62 | 0.030a |
T* | 17.41±1.97 | 16.69±2.44 | 0.018a |
IPL | 29.23±1.51 | 28.96±1.22 | 0.360c |
INL* | 16.69±1.88 | 15.59±1.88 | <0.001a,** |
ST | 15.10±2.79 | 14.35±1.74 | 0.128b |
SN | 14.59±2.44 | 14.13±3.01 | 0.159a |
N* | 16.63±2.60 | 15.72±2.86 | 0.037a |
IN* | 15.06±2.88 | 13.84±2.40 | 0.006a |
IT* | 14.85±2.69 | 13.44±2.02 | 0.006c |
T* | 20.33±3.00 | 18.86±2.88 | 0.019c |
OPL | 25.26±1.21 | 24.95±1.18 | 0.215c |
HOAM | 59.06±4.75 | 60.26±7.45 | 0.344a |
EZ | 14.38±1.60 | 13.94±1.42 | 0.162c |
OS | 9.42±1.50 | 9.53±1.47 | 0.716c |
OPRS | 11.81±3.48 | 11.74±2.27 | 0.917b |
RPE | 20.53±0.94 | 20.52±0.55 | 0.211a |
Data are presented as mean ± standard deviation. a, Mann-Whitney U test; b, Welch’s t-test; c, Student’s t-test. *, significant differences; **, significant results after Bonferroni correction. ASD, autism spectrum disorder; NT, neurotypical; RNFL, retinal nerve fiber layer; GCL, ganglion cell layer; ST, supero-temporal quadrant; SN, supero-nasal quadrant; N, nasal quadrant; IN, infero-nasal quadrant; IT, infero-temporal quadrant; T, temporal quadrant; IPL, inner plexiform layer; INL, inner nuclear layer; OPL, outer plexiform; HOAM, Henle fiber layer/outer nuclear layer/myoid zone; EZ, ellipsoid zone; OS, outer segment; OPRS, outer segment PR/RPE complex and subretinal virtual space; PR, photoreceptor; RPE, retinal pigment epithelium.
Correlation between intraretinal thickness and clinical parameters
For the thickness of the GCL and INL, which showed intergroup differences, further analysis was conducted to explore their correlation with the social functioning quantified by SRS scale and cognitive level quantified by IQ based on WISC-IV. According to Spearman’s correlation analyses, there were no significant correlations observed between the subscale and total SRS scores and the thickness of the involved intraretinal layers or their respective sub-quadrants as shown in Table 3. The thickness from IN quadrant of INL showed significant correlation with IQ (r=0.381, P=0.014) as shown in Table 4.
Table 3
Layer thickness | SRS-Aw | SRS-Cog | SRS-Com | SRS-Mo | SRS-Ma | SRS-Total | |||||||||||
---|---|---|---|---|---|---|---|---|---|---|---|---|---|---|---|---|---|
r | P | r | P | r | P | r | P | r | P | r | P | ||||||
GCL | 0.018 | 0.935 | −0.116 | 0.591 | −0.156 | 0.467 | −0.273 | 0.197 | −0.149 | 0.488 | −0.128 | 0.551 | |||||
IN | −0.222 | 0.297 | −0.079 | 0.712 | −0.209 | 0.328 | 0.006 | 0.977 | −0.119 | 0.580 | −0.119 | 0.579 | |||||
IT | −0.045 | 0.834 | −0.008 | 0.969 | 0.019 | 0.931 | −0.257 | 0.225 | −0.033 | 0.878 | 0.005 | 0.981 | |||||
T | −0.060 | 0.782 | −0.051 | 0.814 | −0.157 | 0.463 | −0.123 | 0.568 | −0.096 | 0.657 | −0.045 | 0.834 | |||||
INL | −0.203 | 0.342 | −0.112 | 0.604 | −0.281 | 0.183 | −0.189 | 0.376 | −0.236 | 0.266 | −0.156 | 0.467 | |||||
N | −0.209 | 0.328 | −0.321 | 0.126 | −0.336 | 0.108 | 0.128 | 0.551 | −0.334 | 0.111 | −0.248 | 0.243 | |||||
IN | −0.439 | 0.032 | −0.057 | 0.793 | −0.189 | 0.377 | 0.034 | 0.874 | −0.174 | 0.417 | −0.098 | 0.649 | |||||
IT | 0.017 | 0.937 | −0.007 | 0.973 | −0.186 | 0.383 | −0.325 | 0.122 | −0.149 | 0.487 | −0.092 | 0.668 | |||||
T | 0.011 | 0.961 | −0.080 | 0.711 | −0.292 | 0.166 | −0.387 | 0.061 | −0.232 | 0.274 | −0.150 | 0.484 |
SRS, the Chinese version of Social Responsiveness Scale; SRS-Aw, score of the social awareness domain in SRS; SRS-Cog, score of social cognition domain in SRS; SRS-Com, score of social communication domain in SRS; SRS-Mo, score of social motivation domain in SRS; SRS-Ma, score of Autistic Mannerisms domain in SRS; SRS-Total, total score of SRS; GCL, ganglion cell layer; IN, infero-nasal quadrant; IT, infero-temporal quadrant; T, temporal quadrant; INL, inner nuclear layer; N, nasal quadrant.
Table 4
Layer thickness | IQ | |
---|---|---|
r | P | |
GCL | −0.167 | 0.297 |
IN | 0.093 | 0.564 |
IT | −0.083 | 0.605 |
T | −0.243 | 0.125 |
INL | 0.035 | 0.828 |
N | 0.270 | 0.088 |
IN* | 0.381 | 0.014 |
IT | −0.095 | 0.555 |
T | −0.153 | 0.340 |
*, significant differences. WISC-IV, Wechsler Intelligence Scale for Children, Fourth Edition; IQ, score of full-scale intelligence quotient of WISC-IV; GCL, ganglion cell layer; IN, infero-nasal quadrant; IT, infero-temporal quadrant; T, temporal quadrant; INL, inner nuclear layer; N, nasal quadrant.
Predictive potential of intraretinal thickness for ASD
Based on the results of the stepwise regression analysis, our aim was to determine which retinal layer thickness have the potential for effective prediction of social functioning (subscale and total SRS scores) and cognitive level (total IQ score) in individuals with ASD. With regard to the total SRS score, it was not possible to identify any specific retinal sublayer thickness that demonstrated effective predictive performance. However, in the case of the total IQ score, a significant positive correlation was observed between the global thickness of INL and the total IQ score as shown in Table 5. Although the overall R2 is not at a high level, this association exhibits statistical significance.
Table 5
Step | Model | β | SE | t | P | RMSE | R2 | Adjusted R2 |
---|---|---|---|---|---|---|---|---|
1 | (Intercept) | 31.613 | 30.620 | 1.032 | 0.308 | |||
INL | 3.986 | 1.818 | 2.193 | 0.034 | 21.900 | 0.110 | 0.087 |
β indicates the regression coefficient. IQ, score of full-scale intelligence quotient of WISC-IV; ASD, autism spectrum disorder; SE, standard error; RMSE, root mean square error; INL, the thickness of the inner nuclear layer; WISC-IV, Wechsler Intelligence Scale for Children, Fourth Edition.
Discussion
The retina serves as the site for photoelectric conversion and intricately regulates various enzymes and proteins. Consequently, it is highly susceptible to systemic changes and may act as an indicator for various diseases (17), offering a potential avenue for aiding in the diagnosis and understanding of the pathological mechanisms of ASD. This study substantiates this notion by revealing that, compared to the control group, individuals with ASD manifested thickening of the GCL and INL within the peripapillary global region and its quadrants. Additionally, we explored the relationship between the global and quadrant-specific thickness of GCL and INL and the social functioning (i.e., total SRS score) or cognitive level (i.e., total IQ score) of ASD children, exposing a significant correlation between the inferior-nasal INL thickness and IQ, and no statistically significant correlation between the selected thickness and SRS. Furthermore, we employed stepwise regression to discern the most influential predictors within the complex interplay of retinal features and clinical outcomes, finding that the global INL thickness fit well with IQ. However, none of the layer thicknesses demonstrated predictive capability for the SRS parameter. To the best of our knowledge, this study represents the first comprehensive exploration of all intraretinal layers within the peripapillary region of children with ASD.
Research gaps in assessing retinal changes in ASD
Despite the retina’s potential for ASD research, only a few studies employing OCT have explored retinal alterations associated with autism, with a predominant focus on the pRNFL in the investigations related to the retinal disc area. Emberti Gialloreti et al. (25) conducted a study comparing the pRNFL thickness in 24 individuals with ASD and 24 NT young adults (mean age: 23.4±6.0 years). Their findings revealed a thinning of the pRNFL in the nasal quadrant among the ASD group and established a negative correlation between pRNFL thickness in ASD individuals and verbal IQ. In contrast, García-Medina et al. (26) investigated pRNFL thickness in 27 ASD individuals (mean age: 13.7±3.0 years) compared to 27 NT controls, reporting a thickening in the temporal inferior, nasal inferior, and inferior quadrants of pRNFL in the ASD group. They further identified a positive correlation between pRNFL thickness in the temporal-inferior region of ASD individuals and both verbal and nonverbal IQ. However, a subsequent study by Garcia-Medina et al. (27) found no significant differences in pRNFL thickness between 13 ASD and 14 NT individuals (mean age: 16.6±3.0 years). Bozkurt et al. (28) extended the investigation to 40 ASD individuals and 40 control individuals (mean age: 9.4±1.6 years), discovering significantly lower pRNFL thickness in the temporal, temporal superior, nasal superior, temporal inferior, and global regions in ASD individuals, with no observed correlation between pRNFL thickness and the severity of ASD symptoms. Friedel et al. (29) conducted a comparative study involving 34 ASD individuals and 31 NT controls (mean age: 35±10 years) but did not observe any changes in pRNFL thickness in individuals with ASD. In a more recent study by Bağcı et al. (30) encompassing 41 ASD subjects, 38 healthy siblings, and 43 healthy controls (average age: 12, 13, and 12 years, with a range of age: 5–18, 4–18, and 7–17 years), no significant differences were found in pRNFL thickness among these groups.
In light of these varying findings, the assessment of intraretinal layer changes in individuals with ASD presently lacks a consensus. Firstly, retinal imaging in ASD is a relative new field, with ongoing studies yet to attain a sufficient scale of sample size. Secondly, the age distribution among participants in relevant studies exhibits noteworthy disparities, and there is a scarcity of longitudinal studies. Furthermore, ASD manifests considerable heterogeneity, and the existing sample sizes may not suffice for a more nuanced patient stratification, potentially resulting in disparate outcomes despite similar sample sizes. Additionally, the utilization of diverse imaging equipment may introduce notable biases. Considering these factors, we contend that the current body of research on disparities in retinal sublayers between individuals with ASD and their typically developing counterparts lacks substantial comparative relevance, even when their findings diverge.
Understanding retinal abnormalities in ASD: potential mechanisms
Retinal nuclear layers, such as GCL and INL, are recognized as a paradigm of GM. Extensive ASD research reveals structural changes in GM (41) as well as atypical visual processing (42). Here, we explore the mechanisms behind thickened retinal layers, focusing on well-established functional alterations in ASD. Notably, OCT research primarily shows structural changes. Thus, the discussion on potential mechanisms related to retinal alterations remains speculative, aiming at providing additional perspectives within the emerging field.
The excitatory/inhibitory (E/I) imbalance theory in autism highlights γ-aminobutyric acid (GABA) and glutamate roles (43,44). Immunohistochemistry studies show GABA and glutamate in GCL and INL, impacting retinal responsiveness (45). An ASD rat model study reported the GABA reduction and mGluR5 increase affect visual information processing (46). And the GABAergic system has been reported to modulate retinal function differently in autistic and typically developed individuals (20). Based on our findings, we hypothesize that GABA/glutamate imbalance may influence synaptic function, potentially leading to the thickening of specific intraretinal layers, such as INL and GCL.
Mitochondrial dysfunction is postulated to play a pivotal role in the pathophysiology of ASD (47-49), with ASD even being considered as part of a broader spectrum of mitochondrial diseases (50,51). Mitochondrial dysfunction affects adenosine triphosphate production and causing oxidative stress. Neuroimaging studies confirm altered glucose metabolism and increased oxidative stress in ASD brains (52-55). The retina’s complex electrophysiological processes require precise energy supply, and mitochondrial dysfunction may disrupt retinal homeostasis, potentially altering retinal structure.
Immune damage and neuroinflammation play key roles in ASD pathogenesis. Abnormal activation of microglia and astrocytes is linked to enhanced immune responses and synaptic regulation in ASD brains (56-62). Microglia and astrocytes distribute across multiple retinal sublayers (63), potentially contributing to the thickening of GCL and INL.
Intraretinal layer thickness as a potential biomarker for ASD
This study did not find a significant correlation or predictive relationship between retinal sublayer thickness in the ASD group and the severity of core ASD symptoms measured by the total SRS score. However, the limited association between retinal thickness and social functioning in ASD, alongside the variability in social impairments and potential biases from partial clinical data exclusions, suggests caution in interpreting these results. Despite this, the study hints at potential for using retinal layer thickness as an ASD biomarker, encouraging future research with larger, stratified samples to explore simpler diagnostic methods.
Interestingly, we observed a positive correlation between the infero-nasal INL thickness and the cognitive level measured by the total IQ score. Moreover, the overall INL thickness demonstrated potential for predicting cognitive functioning. This suggests a potential coupling between global or localized INL thickness and cognitive function. Previous research also highlights a higher prevalence of intellectual disabilities in ASD populations, underscoring the relevance of investigating INL thickness as a predictor of cognitive abilities in autism (64-68). These findings imply that INL thickness may hold research potential within the hierarchical context of cognitive impairments in autism, providing inspiration for future investigations.
Limitations & future directions
The retina of individuals with ASD remains an area with limited comprehensive research. To address the heterogeneity of ASD, it is imperative to conduct stratified research and longitudinal analysis based on an expanded sample size. In light of the potential for multiple comparison corrections to introduce false-negative results beyond the anticipated level (69), this study cautiously emphasizes exploration of unadjusted results while also presenting statistically corrected significant findings to uphold diverse interpretations of the results. Notably, the statistical significance in this paper was explored under the resolution of the OCT device, meaning differences below 5 µm were not taken into consideration.
Given the strong gender bias towards males in ASD, the number of female samples is relatively small in all known related studies. In our study, we equalized the gender ratio between the ASD and NT groups to potentially account for any gender-related differences. However, future research considering gender as a subgroup is worth exploration. Additionally, the integration of multimodal data is essential for a comprehensive exploration of the retina from various perspectives. For example, optical coherence tomography angiography (OCTA) technology is able to provide information on blood flow, which naturally register and complement with OCT. Furthermore, abnormalities in brain lateralization provide valuable insights into the neural basis and developmental mechanisms of autism, which is possibly projected to the differences in interocular asymmetry between autism and control groups, making it a pertinent area of interest for our future work.
Conclusions
In this study, we enlarged the range of intraretinal layers from only the RNFL to almost all the retinal sublayer of peripapillary region, compared to previous studies. The expanded sample size might mitigate the data randomness, enhancing the reliability of the experimental results to some extent. This study was conducted in 47 children aged 7 to 13 with ASD and a well-matched control group in terms of number, age, and gender. We found statistically significant thickening in global GCL and INL in ASD subjects, suggesting a structural retinal change in children with ASD. Furthermore, we performed quadrant-wise analysis and observed increased thickness in the GCL in the nasal, inferior-temporal, and temporal quadrants, while the INL showed thickening in the nasal, inferior-nasal, inferior-temporal, and temporal quadrants in ASD group. Meanwhile, the inferior-nasal thickness of INL demonstrated a correlation with the total IQ scores. The stepwise regression model revealed that the global thickness of INL exhibited effective fitting for the IQ scores in individuals with ASD. No significant correlation or predictive power was observed between the thickness of intraretinal layers and the total SRS scores. This study provides additional evidence for exploring the retina as a potential biomarker for ASD and may provide new directions for further investigation.
Acknowledgments
Funding: This study was supported by
Footnote
Reporting Checklist: The authors have completed the STROBE reporting checklist. Available at https://qims.amegroups.com/article/view/10.21037/qims-24-753/rc
Conflicts of Interest: All authors have completed the ICMJE uniform disclosure form (available at https://qims.amegroups.com/article/view/10.21037/qims-24-753/coif). The authors have no conflicts of interest to declare.
Ethical Statement: The authors are accountable for all aspects of the work in ensuring that questions related to the accuracy or integrity of any part of the work are appropriately investigated and resolved. The study was conducted in accordance with the Declaration of Helsinki (as revised in 2013). The study was approved by the Medical Ethics Committee of Nanjing Medical University (Approval ID: 2020-SR-363). Informed consent was obtained from the participants’ parents or guardians.
Open Access Statement: This is an Open Access article distributed in accordance with the Creative Commons Attribution-NonCommercial-NoDerivs 4.0 International License (CC BY-NC-ND 4.0), which permits the non-commercial replication and distribution of the article with the strict proviso that no changes or edits are made and the original work is properly cited (including links to both the formal publication through the relevant DOI and the license). See: https://creativecommons.org/licenses/by-nc-nd/4.0/.
References
- American Psychiatric Association. Diagnostic and Statistical Manual of Mental Disorders. 5th ed. 2013.
- Xu G, Strathearn L, Liu B, O’Brien M, Kopelman TG, Zhu J, Snetselaar LG, Bao W. Prevalence and Treatment Patterns of Autism Spectrum Disorder in the United States, 2016. JAMA Pediatr 2019;173:153-9. [Crossref] [PubMed]
- Saito M, Hirota T, Sakamoto Y, Adachi M, Takahashi M, Osato-Kaneda A, Kim YS, Leventhal B, Shui A, Kato S, Nakamura K. Prevalence and cumulative incidence of autism spectrum disorders and the patterns of co-occurring neurodevelopmental disorders in a total population sample of 5-year-old children. Mol Autism 2020;11:35. [Crossref] [PubMed]
- Talantseva OI, Romanova RS, Shurdova EM, Dolgorukova TA, Sologub PS, Titova OS, Kleeva DF, Grigorenko EL. The global prevalence of autism spectrum disorder: A three-level meta-analysis. Front Psychiatry 2023;14:1071181. [Crossref] [PubMed]
- Yanoff M, Sassani JW. Neural (Sensory) Retina. In: Ocular Pathology. 7th ed. 2015:351-425.
- Chan-Ling T. Glial, neuronal and vascular interactions in the mammalian retina. Prog Retin Eye Res 1994;13:357-89.
- Stone J, Dreher Z. Relationship between astrocytes, ganglion cells and vasculature of the retina. J Comp Neurol 1987;255:35-49. [Crossref] [PubMed]
- Wässle H, Koulen P, Brandstätter JH, Fletcher EL, Becker CM. Glycine and GABA receptors in the mammalian retina. Vision Res 1998;38:1411-30. [Crossref] [PubMed]
- Pourcho RG. Neurotransmitters in the retina. Curr Eye Res 1996;15:797-803. [Crossref] [PubMed]
- Kaur C, Foulds WS, Ling EA. Blood-retinal barrier in hypoxic ischaemic conditions: Basic concepts, clinical features and management. Prog Retin Eye Res 2008;27:622-47. [Crossref] [PubMed]
- Streilein JW. Ocular immune privilege: therapeutic opportunities from an experiment of nature. Nat Rev Immunol 2003;3:879-89. [Crossref] [PubMed]
- Szapiro J, Pakula H. Anatomical Studies of The Collateral Blood Supply To The Brain And The Retina. J Neurol Neurosurg Psychiatry 1963;26:414-7. [Crossref] [PubMed]
- Steuer H, Jaworski A, Elger B, Kaussmann M, Keldenich J, Schneider H, Stoll D, Schlosshauer B. Functional characterization and comparison of the outer blood-retina barrier and the blood-brain barrier. Invest Ophthalmol Vis Sci 2005;46:1047-53. [Crossref] [PubMed]
- Simmons DR, Robertson AE, McKay LS, Toal E, McAleer P, Pollick FE. Vision in autism spectrum disorders. Vision Res 2009;49:2705-39. [Crossref] [PubMed]
- Cheng N, Pagtalunan E, Abushaibah A, Naidu J, Stell WK, Rho JM, Sauvé Y. Atypical visual processing in a mouse model of autism. Sci Rep 2020;10:12390. [Crossref] [PubMed]
- Spiteri S, Crewther D. Neural Mechanisms of Visual Motion Anomalies in Autism: A Two-Decade Update and Novel Aetiology. Front Neurosci 2021;15:756841. [Crossref] [PubMed]
- Rasmussen R, Matsumoto A, Dahlstrup Sietam M, Yonehara K. A segregated cortical stream for retinal direction selectivity. Nat Commun 2020;11:831. [Crossref] [PubMed]
- Constable PA, Ritvo ER, Ritvo AR, Lee IO, McNair ML, Stahl D, Sowden J, Quinn S, Skuse DH, Thompson DA, McPartland JC. Light-Adapted Electroretinogram Differences in Autism Spectrum Disorder. J Autism Dev Disord 2020;50:2874-85. [Crossref] [PubMed]
- Manjur SM, Hossain MB, Constable PA, Thompson DA, Marmolejo-Ramos F, Lee IO, Skuse DH, Posada-Quintero HF. Detecting Autism Spectrum Disorder Using Spectral Analysis of Electroretinogram and Machine Learning: Preliminary results. Annu Int Conf IEEE Eng Med Biol Soc 2022;2022:3435-8. [Crossref] [PubMed]
- Huang Q, Ellis CL, Leo SM, Velthuis H, Pereira AC, Dimitrov M, Ponteduro FM, Wong NML, Daly E, Murphy DGM, Mahroo OA, McAlonan GM. Retinal GABAergic Alterations in Adults with Autism Spectrum Disorder. J Neurosci 2024;44:e1218232024. [Crossref] [PubMed]
- Apicella F, Costanzo V, Purpura G. Are early visual behavior impairments involved in the onset of autism spectrum disorders? Insights for early diagnosis and intervention. Eur J Pediatr 2020;179:225-34. [Crossref] [PubMed]
- Swanson EA, Izatt JA, Hee MR, Huang D, Lin CP, Schuman JS, Puliafito CA, Fujimoto JG. In vivo retinal imaging by optical coherence tomography. Opt Lett 1993;18:1864-6. [Crossref] [PubMed]
- Huang D, Swanson EA, Lin CP, Schuman JS, Stinson WG, Chang W, Hee MR, Flotte T, Gregory K, Puliafito CA. Optical coherence tomography. Science 1991;254:1178-81. [Crossref] [PubMed]
- Thomas D, Duguid G. Optical coherence tomography--a review of the principles and contemporary uses in retinal investigation. Eye (Lond) 2004;18:561-70. [Crossref] [PubMed]
- Emberti Gialloreti L, Pardini M, Benassi F, Marciano S, Amore M, Mutolo MG, Porfirio MC, Curatolo P. Reduction in retinal nerve fiber layer thickness in young adults with autism spectrum disorders. J Autism Dev Disord 2014;44:873-82. [Crossref] [PubMed]
- García-Medina JJ, García-Piñero M, Del-Río-Vellosillo M, Fares-Valdivia J, Ragel-Hernández AB, Martínez-Saura S, Cárcel-López MD, Zanon-Moreno V, Pinazo-Duran MD, Villegas-Pérez MP. Comparison of Foveal, Macular, and Peripapillary Intraretinal Thicknesses Between Autism Spectrum Disorder and Neurotypical Subjects. Invest Ophthalmol Vis Sci 2017;58:5819-26. [Crossref] [PubMed]
- Garcia-Medina JJ, Rubio-Velazquez E, Lopez-Bernal MD, Parraga-Muñoz D, Perez-Martinez A, Pinazo-Duran MD, Del-Rio-Vellosillo M. Optical Coherence Tomography Angiography of Macula and Optic Nerve in Autism Spectrum Disorder: A Pilot Study. J Clin Med 2020;9:3123. [Crossref] [PubMed]
- Bozkurt A, Say GN, Şahin B, Usta MB, Kalyoncu M, Koçak N, et al. Evaluation of retinal nerve fiber layer thickness in children with autism spectrum disorders. Res Autism Spectr Disord 2022;98:102050.
- Friedel EBN, Tebartz van Elst L, Schäfer M, Maier S, Runge K, Küchlin S, Reich M, Lagrèze WA, Kornmeier J, Ebert D, Endres D, Domschke K, Nickel K. Retinal Thinning in Adults with Autism Spectrum Disorder. J Autism Dev Disord 2024;54:1143-56. [Crossref] [PubMed]
- Bağcı KA, Çöp E, Memiş PN, Işık FD. Investigation of retinal layers thicknesses in autism spectrum disorder and comparison with healthy siblings and control group. Res Autism Spectr Disord 2023;108:102242.
- Zhang HC. The revision of WISC-IV Chinese version. Psychol Sci 2009;32:1177-9.
- Goodman R, Scott S. Comparing the Strengths and Difficulties Questionnaire and the Child Behavior Checklist: is small beautiful? J Abnorm Child Psychol 1999;27:17-24. [Crossref] [PubMed]
- Chojnicka I, Pisula E. Adaptation and Validation of the ADOS-2, Polish Version. Front Psychol 2017;8:1916. [Crossref] [PubMed]
- Lebersfeld JB, Swanson M, Clesi CD, O’Kelley SE. Systematic Review and Meta-Analysis of the Clinical Utility of the ADOS-2 and the ADI-R in Diagnosing Autism Spectrum Disorders in Children. J Autism Dev Disord 2021;51:4101-14. [Crossref] [PubMed]
- Saemundsen E, Magnússon P, Smári J, Sigurdardóttir S. Autism Diagnostic Interview-Revised and the Childhood Autism Rating Scale: convergence and discrepancy in diagnosing autism. J Autism Dev Disord 2003;33:319-28. [Crossref] [PubMed]
- Cen CQ, Liang YY, Chen QR, Chen KY, Deng HZ, Chen BY, Zou XB. Investigating the validation of the Chinese Mandarin version of the Social Responsiveness Scale in a Mainland China child population. BMC Psychiatry 2017;17:51. [Crossref] [PubMed]
- Aldridge FJ, Gibbs VM, Schmidhofer K, Williams M. Investigating the clinical usefulness of the Social Responsiveness Scale (SRS) in a tertiary level, autism spectrum disorder specific assessment clinic. J Autism Dev Disord 2012;42:294-300. [Crossref] [PubMed]
- Li K, Wu X, Chen DZ, Sonka M. Optimal surface segmentation in volumetric images--a graph-theoretic approach. IEEE Trans Pattern Anal Mach Intell 2006;28:119-34. [Crossref] [PubMed]
- Antony B, Abràmoff MD, Tang L, Ramdas WD, Vingerling JR, Jansonius NM, Lee K, Kwon YH, Sonka M, Garvin MK. Automated 3-D method for the correction of axial artifacts in spectral-domain optical coherence tomography images. Biomed Opt Express 2011;2:2403-16. [Crossref] [PubMed]
- Garvin MK, Abràmoff MD, Wu X, Russell SR, Burns TL, Sonka M. Automated 3-D intraretinal layer segmentation of macular spectral-domain optical coherence tomography images. IEEE Trans Med Imaging 2009;28:1436-47. [Crossref] [PubMed]
- Sun F, Chen Y, Gao Q, Zhao Z. Abnormal gray matter structure in children and adolescents with high-functioning autism spectrum disorder. Psychiatry Res Neuroimaging 2022;327:111564. [Crossref] [PubMed]
- Chung S, Son JW. Visual Perception in Autism Spectrum Disorder: A Review of Neuroimaging Studies. Soa Chongsonyon Chongsin Uihak 2020;31:105-20. [Crossref] [PubMed]
- Rubenstein JL, Merzenich MM. Model of autism: increased ratio of excitation/inhibition in key neural systems. Genes Brain Behav 2003;2:255-67. [Crossref] [PubMed]
- Uzunova G, Pallanti S, Hollander E. Excitatory/inhibitory imbalance in autism spectrum disorders: Implications for interventions and therapeutics. World J Biol Psychiatry 2016;17:174-86. [Crossref] [PubMed]
- Crooks J, Kolb H. Localization of GABA, glycine, glutamate and tyrosine hydroxylase in the human retina. J Comp Neurol 1992;315:287-302. [Crossref] [PubMed]
- Guimarães-Souza EM, Joselevitch C, Britto LRG, Chiavegatto S. Retinal alterations in a pre-clinical model of an autism spectrum disorder. Mol Autism 2019;10:19. [Crossref] [PubMed]
- Fillano JJ, Goldenthal MJ, Rhodes CH, Marín-García J. Mitochondrial dysfunction in patients with hypotonia, epilepsy, autism, and developmental delay: HEADD syndrome. J Child Neurol 2002;17:435-9. [Crossref] [PubMed]
- Filipek PA, Juranek J, Smith M, Mays LZ, Ramos ER, Bocian M, Masser-Frye D, Laulhere TM, Modahl C, Spence MA, Gargus JJ. Mitochondrial dysfunction in autistic patients with 15q inverted duplication. Ann Neurol 2003;53:801-4. [Crossref] [PubMed]
- Tsao CY, Mendell JR. Autistic disorder in 2 children with mitochondrial disorders. J Child Neurol 2007;22:1121-3. [Crossref] [PubMed]
- Palmieri L, Persico AM. Mitochondrial dysfunction in autism spectrum disorders: cause or effect? Biochim Biophys Acta 2010;1797:1130-7.
- Nissenkorn A, Zeharia A, Lev D, Watemberg N, Fattal-Valevski A, Barash V, Gutman A, Harel S, Lerman-Sagie T. Neurologic presentations of mitochondrial disorders. J Child Neurol 2000;15:44-8. [Crossref] [PubMed]
- Chauhan A, Chauhan V. Oxidative stress in autism. Pathophysiology 2006;13:171-81. [Crossref] [PubMed]
- Chauhan A, Chauhan V, Brown WT, Cohen I. Oxidative stress in autism: increased lipid peroxidation and reduced serum levels of ceruloplasmin and transferrin--the antioxidant proteins. Life Sci 2004;75:2539-49. [Crossref] [PubMed]
- Al-Gadani Y, El-Ansary A, Attas O, Al-Ayadhi L. Metabolic biomarkers related to oxidative stress and antioxidant status in Saudi autistic children. Clin Biochem 2009;42:1032-40. [Crossref] [PubMed]
- Zoroglu SS, Armutcu F, Ozen S, Gurel A, Sivasli E, Yetkin O, Meram I. Increased oxidative stress and altered activities of erythrocyte free radical scavenging enzymes in autism. Eur Arch Psychiatry Clin Neurosci 2004;254:143-7. [Crossref] [PubMed]
- Matta SM, Hill-Yardin EL, Crack PJ. The influence of neuroinflammation in Autism Spectrum Disorder. Brain Behav Immun 2019;79:75-90. [Crossref] [PubMed]
- Schafer DP, Lehrman EK, Kautzman AG, Koyama R, Mardinly AR, Yamasaki R, Ransohoff RM, Greenberg ME, Barres BA, Stevens B. Microglia sculpt postnatal neural circuits in an activity and complement-dependent manner. Neuron 2012;74:691-705. [Crossref] [PubMed]
- Carroll L, Braeutigam S, Dawes JM, Krsnik Z, Kostovic I, Coutinho E, Dewing JM, Horton CA, Gomez-Nicola D, Menassa DA. Autism Spectrum Disorders: Multiple Routes to, and Multiple Consequences of, Abnormal Synaptic Function and Connectivity. Neuroscientist 2021;27:10-29. [Crossref] [PubMed]
- Koyama R, Ikegaya Y. Microglia in the pathogenesis of autism spectrum disorders. Neurosci Res 2015;100:1-5. [Crossref] [PubMed]
- Elçi ME, Usta MB, Karabekiroğlu K. Role of Glia Cells in Autism Spectrum Disorders. Psikiyatr Güncel Yaklaşımlar 2023;15:577-88.
- Morgan JT, Chana G, Pardo CA, Achim C, Semendeferi K, Buckwalter J, Courchesne E, Everall IP. Microglial activation and increased microglial density observed in the dorsolateral prefrontal cortex in autism. Biol Psychiatry 2010;68:368-76. [Crossref] [PubMed]
- Tetreault NA, Hakeem AY, Jiang S, Williams BA, Allman E, Wold BJ, Allman JM. Microglia in the cerebral cortex in autism. J Autism Dev Disord 2012;42:2569-84. [Crossref] [PubMed]
- Silverman SM, Wong WT. Microglia in the Retina: Roles in Development, Maturity, and Disease. Annu Rev Vis Sci 2018;4:45-77. [Crossref] [PubMed]
- Charman T, Pickles A, Simonoff E, Chandler S, Loucas T, Baird G. IQ in children with autism spectrum disorders: data from the Special Needs and Autism Project (SNAP). Psychol Med 2011;41:619-27. [Crossref] [PubMed]
- Shaw SR, Jankowska AM. Pediatric Intellectual Disabilities at School Translating Research into Practice. Pediatric School Psychology. Springer, 2018.
- Maenner MJ, Shaw KA, Baio J, Washington A, Patrick M, et al. Prevalence of Autism Spectrum Disorder Among Children Aged 8 Years - Autism and Developmental Disabilities Monitoring Network, 11 Sites, United States, 2016. MMWR Surveill Summ 2020;69:1-12. [Crossref] [PubMed]
- Davis CH, Kim KY, Bushong EA, Mills EA, Boassa D, Shih T, Kinebuchi M, Phan S, Zhou Y, Bihlmeyer NA, Nguyen JV, Jin Y, Ellisman MH, Marsh-Armstrong N. Transcellular degradation of axonal mitochondria. Proc Natl Acad Sci U S A 2014;111:9633-8. [Crossref] [PubMed]
- Rodriguez JI, Kern JK. Evidence of microglial activation in autism and its possible role in brain underconnectivity. Neuron Glia Biol 2011;7:205-13. [Crossref] [PubMed]
- Rothman KJ. No adjustments are needed for multiple comparisons. Epidemiology 1990;1:43-6.