Identification of vessels encapsulating tumor clusters in solitary hepatocellular carcinoma via imaging biomarkers in preoperative contrast-enhanced magnetic resonance imaging
Introduction
With progressively increasing incidence, hepatocellular carcinoma (HCC) is now the most prevalent primary liver malignancy in the world (1). Although the diagnosis of HCC is improving, the estimation of prognosis remains unsatisfactory and complicated (2,3) due to HCC’s highly heterogeneous nature on the genetic, molecular, and histological levels (3,4). As the poor prognosis of HCC is mainly attributed to recurrence and metastasis and as angiogenesis is intimately related to the mechanisms of occurrence, progression, and metastasis (5), it is reasonable to speculate that angiogenesis could be used for estimating HCC prognosis.
Researchers investigating the metastatic mechanism of HCC have proposed various theories, among which epithelial-to-mesenchymal transition (EMT) is the most widely recognized (6). Recently, it has been reported that the vessels encapsulating tumor clusters (VETC) pattern is common in HCC and is formed by tumor cells. The newly discovered VETC vascular growth pattern promotes HCC metastasis in an EMT-independent manner, indicating that the presence of VETC facilitates HCC metastasis and recurrence (7-9). In addition to supplying oxygen and nutrients to support tumor growth, HCC tumor vessels also provide routes for metastasis (5). A study by Fang et al. indicated that VETC could be a predictor of sorafenib efficacy in patients with HCC (10). These findings point to the potential of the VETC pattern to better inform and aid in the prevention and reduction of metastatic HCC (7,10,11). However, the confirmation of VETC pattern can only be completed via invasive histopathological evaluation. Thus, the preoperative identification of VETC pattern via noninvasive means may be of considerable value in facilitating treatment decision-making, developing individual follow-up plans, and implementing prompt interventions.
Thus far, few studies have sought to assess the pretherapeutic characterization of VETC pattern in HCC. Although previous studies have reported that the radiological characteristics of computed tomography (CT) and magnetic resonance imaging (MRI) are independent predictors for VETC pattern (12-14), three-dimensional volumetric quantification of tumor tissue in multiparametric MRI for VETC assessment has not been examined. Moreover, the findings that have been published thus far have not yet been thoroughly validated or put into practical use, and further study in this field is still required. The Liver Imaging Reporting and Data System (LI-RADS) (15) can be used to standardize HCC diagnosis. Recent studies have found that characteristics in solid mass as interpreted by the 2018 LI-RADS, including mosaic architecture, corona enhancement, and fat sparing may be biomarkers for evaluating microvascular invasion, early recurrence, and survival in patients with HCC (16-19). However, there are no reports on the use of LI-RADS radiological features from multiparametric MRI for assessing VETC status in HCC.
Therefore, we conducted a study to clarify the value of three-dimensional tumor volumetric analysis, LI-RADS radiological features, and contrast-enhanced MRI-derived quantitative parameters for predicting VETC pattern in patients with solitary HCC. We present this article in accordance with the TRIPOD reporting checklist (available at https://qims.amegroups.com/article/view/10.21037/qims-24-315/rc).
Methods
Study design and patient population
In total, 126 patients confirmed by pathology as HCC who underwent abdominal contrast-enhanced 3-T MRI within two weeks before hepatectomy between December 2018 and January 2022 in Chongqing General Hospital were enrolled. This retrospective study was approved by the institutional review board of the Chongqing General Hospital (No. s2019-038-01) and conducted in accordance with the Declaration of Helsinki (as revised in 2013). Written informed consent was waived due to the retrospective nature of this research. Figure 1 presents the detailed procedures of patients’ enrollment. Solitary HCC was diagnosed via pathological examination, which included hematoxylin and eosin staining and cluster of differentiation 34 (CD34) immunostaining (7,10). Finally, 36 patients with pathologically diagnosed solitary HCC were enrolled. All preoperative clinical data and laboratory examination results of eligible patients were obtained from the electronic medical record system, including age, sex, history of cirrhosis, hepatitis B/C virus infection, Child-Pugh class, serum alpha-fetoprotein (AFP) level, alanine aminotransferase (ALT) level, aspartate aminotransferase (AST) level, gamma-glutamyl transferase (GGT) level, platelet count, total bilirubin (TBIL), and albumin level.
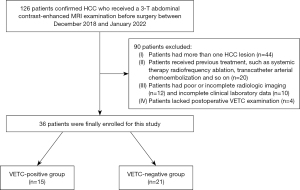
Histopathologic examination
All surgical specimens were independently reviewed by two experienced abdominal pathologist (H.Y. and Y.G., with 3 and 12 years of experience, respectively.) who were not informed of the clinical, laboratory, or MRI results. VETC assessment was independently performed by two experienced pathologists, with any inconsistencies being discussed to reach a consensus. Immunohistochemical staining was performed to assess VETC pattern. The confirmation of VETC pattern included microscopy-indicated presence of sinusoid-like vessels forming web-like networks and encapsulating the individual tumor clusters with an unequivocal and continuous lining of CD34-positive endothelium in the whole or part of the tumor (7). VETC-positive status was considered to be the presence of a visible VETC pattern in whole or part of the solitary HCC section, and group VETC-negative status was considered to be the lack of VETC pattern in whole or part of the solitary HCC section (7,9).
MR examination
Details on image acquisition parameters and scanners can be found in Appendix 1 and Table S1.
Three-dimensional tumor volumetric measurements
To evaluate the interexaminer reliability of volumetry, the tumor three-dimensional volumetric analysis was independently operated on the Vitrea workstation (Canon Medical Systems Corporation, Otawara, Japan) by two radiologists (J.Z. and Z.S., with 3 and 10 years of experience in abdominal MRI and volumetric image analysis, respectively) who were not informed of any clinical data, MRI data, or pathological outcomes. Total liver volume (TLV), tumor volume (TV), and necrosis tumor volume (NTV) were respectively quantified on the basis of portal venous phase (PVP) images, and quantification of enhancing tumor volume (ETV) was based on arterial phase (AP) images. The ETV was volumetrically quantified by gauging the enhancing lesions of HCC, which were defined as the presence of enhancement on the AP images. NTV was quantified by measuring the necrotic lesions of HCC, which were defined as the absence of enhancement on all contrast-enhanced images corresponding to a T2-weighted imaging (T2WI) high signal intensity (SI). The specific segmentation of TLV was performed as follows: first, the PVP data of the solitary HCC were imported into Vitrea software in Digital Imaging and Communications in Medicine format. Second, the organ task was selected in the segmentation anatomy module of the Vitrea software. Third, the region of interest (ROI) with liver edge at each layer of images was automatically identified by clicking on liver parenchyma. Fourth, the observer semiautomatically adjusted the ROI of each slice to improve the accuracy of ROI of the TLV, and then each ROI was automatically merged via Vitrea software into a volume of interest (VOI). Finally, the VOI was added to another module of the Vitrea software, and the TLV was displayed by selecting “show volumes task in another module”. TV, NTV, and ETV were obtained by the same separation method described above. When TV measurements were performed, the peritumor vasculature was avoided. In addition, the following parameters were calculated: NTV percentage (NTVP), defined as the ratio of the NTV to the TV; ETV percentage (ETVP), defined as the ratio of the ETV to the TV; the tumor burden (TB), defined as the ratio of the TV to the TLV; the tumor necrosis burden (TNB), defined as the ratio of the NTV to the TLV; and enhancing tumor burden (ETB), defined as the ratio of the ETV to the TLV.
LI-RADS radiological features analysis
Diagnostic imaging characteristics of each solitary HCC lesion defined in the 2018 LI-RADS were analyzed by two independent radiologists (W.S. and Z.L., with 4 and 13 years of experience in abdominal MRI, respectively) who were not informed of the imaging report or the clinical, laboratory, and pathologic results (15). The diagnostic imaging characteristics consisted of major LI-RADS features, including nonrim AP hyperenhancement, nonperipheral washout, and enhancing capsule, and LI-RADS ancillary features including nonenhancing capsule, nodule-in-nodule architecture, mosaic architecture, blood products in mass, fat in mass, restricted diffusion, mild-to-moderate T2 hyperintensity, corona enhancement, fat sparing in solid mass, and iron sparing in solid mass. In addition, LI-RADS categories were also determined for each solitary HCC lesion according to the 2018 LI-RADS (15). Inconsistencies between the two observers were resolved via appealing to a third radiologist (Z.T., with 18 years of experience in abdominal MRI).
Quantitative parameters assessment
The assessment of quantitative parameters were independently performed by two radiologists (Q.L. and X.R., with 3 and 8 years of experience in abdominal MRI, respectively) on the basis of previous studies (14,20). The SI of tumor was gauged by drawing the ROI on AP phase and PVP phase. The ROI size was manually sketched on the largest cross-section of tumor to cover almost the whole solitary HCC area and avoid peripheral portions and necrotic and cystic areas as much as possible. The average gray level of the ROI represented the SI of tumor. Delta tumor washout was defined as the subtraction of the tumor SI in the AP phase and PVP phase. Meanwhile, the SI of the unaffected liver parenchyma was used to calculate the tumor-to-liver SI ratio on AP images (AP-TLSI). The ROI area (≥50 mm2) of the unaffected liver parenchyma was selected and was close to the ROI area of the lesion as much as possible, with artifacts and large vessels being excluded as much as possible. The minimum, mean, and maximum apparent diffusion coefficient (ADC) values were calculated by two b values (0 and 800 sec/mm2) with a monoexponential fit that was obtained by sketching a ROI on the ADC map at the same level of the largest diameter of the tumor in the axial plane diffusion-weighted images, with necrotic region, cystic regions, and vascular and biliary tract structures being avoided. Finally, the mean value of the parametric values generated by the two radiologists were calculated to obtain the final results.
Statistical analysis
Interexaminer reliability for the measurement of volume was evaluated with intraclass correlation coefficients (ICCs) and categorized as follows: poor agreement, 0–0.49; moderate agreement, 0.50–0.74; good agreement, 0.75–0.89; and excellent agreement, 0.90–1 (21). A two-sided P value <0.05 was considered to indicate a statistically significant difference. Continuous variables are presented as the mean ± standard deviations or as the median and interquartile range. Categorical variables are represented as numbers and percentages. The two-sample t-test or Mann-Whitney test was used to analyze continuous variables. Categorical variables were analyzed with chi-square test or Fisher exact test. Logistic regression analysis was applied to ascertain independent determinants of VETC pattern in solitary HCC. Variables with P<0.05 in univariate analysis were incorporated into the multivariate analysis. A prediction model was derived from multivariate logistic regression analysis, based on which a visual nomogram was constructed. The discrimination capability of the model was quantified by the area under the receiver operating characteristic curve (AUROC). The goodness of fit of the model was evaluated via the calibration curve. Decision curve analysis (DCA) was used to assess the clinical practicability of the model. The bootstrap resampling method (500 repetitions) was applied to perform internal validation on the model. The optimal cutoff threshold was identified by maximizing the Youden index based on nomogram score, and values of sensitivity and specificity at the optimal threshold were calculated. Patients were reclassified by the optimal cutoff threshold, and the accuracy was calculated. All analyses were conducted in R v. 3.6.0 (The R Foundation of Statistical Computing; http://www.Rproject.org).
Results
Patient characteristics and reliability of TV measurements
In total, 36 patients with solitary HCC (30 males and 6 females, with an average age of 60±11.53 years) were included in our study and assigned into the VETC-positive group (15 cases) or VETC-negative group (21 cases). The VETC-positive group contained 14 males and 1 female (average age 57.93±12.01 years), with 13 cases of hepatitis B and no case of hepatitis C. The VETC-negative group contained16 males and 5 females (average age 61.48±11.24 years), with 17 cases of hepatitis B and 2 cases of hepatitis C. No statistically significant difference in the clinical characteristics or laboratory indicators was found between the two groups (P>0.05) (Table 1). The interexaminer reproducibility of TLV, TV, NTV, and ETV were calculated, and the ICCs of these indexes were 0.882, 0.860, 0.832, and 0.810, respectively, indicating good reproducibility.
Table 1
Characteristic | Total (n=36) | VETC negative (n=21) | VETC positive (n=15) | P |
---|---|---|---|---|
Sex, n [%] | 0.367 | |||
Female | 6 [17] | 5 [24] | 1 [7] | |
Male | 30 [83] | 16 [76] | 14 [93] | |
Age (years), mean ± SD | 60±11.53 | 61.48±11.24 | 57.93±12.01 | 0.378 |
Diameter (mm), n [%] | 0.123 | |||
≤50 | 21 [58] | 15 [71] | 6 [40] | |
>50 | 15 [42] | 6 [29] | 9 [60] | |
AFP (ng/mL), median (Q1, Q3) | 39.06 (5.67, 1,128.65) | 13.59 (5.8, 105.8) | 268.4 (17.41, 1,210) | 0.172 |
Hepatitis B virus infection, n [%] | 0.650 | |||
Absent | 6 [17] | 4 [19] | 2 [13] | |
Present | 30 [83] | 17 [81] | 13 [87] | |
Hepatitis C virus infection, n [%] | 0.500 | |||
Absent | 34 [94] | 19 [90] | 15 [100] | |
Present | 2 [6] | 2 [10] | 0 [0] | |
Cirrhosis, n [%] | 0.864 | |||
Absent | 21 [58] | 12 [57] | 9 [60] | |
Present | 15 [42] | 9 [43] | 6 [40] | |
ALT (U/L), median (Q1, Q3) | 39.5 (23.5, 59) | 45 (30.07, 62) | 30 (20.5, 45) | 0.086 |
AST (U/L), median (Q1, Q3) | 38.5 (28.25, 69.5) | 42 (34.5, 71) | 29 (25, 51) | 0.153 |
GGT (U/L), median (Q1, Q3) | 115.1 (37.75, 189.5) | 120 (49, 197) | 72 (33.5, 174.5) | 0.564 |
TBIL (μmol/L), median (Q1, Q3) | 13.05 (8.15, 20) | 11.5 (8.3, 18.5) | 17.8 (9.5, 20.9) | 0.460 |
Albumin (g/L), mean ± SD | 39.55±4.18 | 38.61±4.19 | 40.87±3.94 | 0.109 |
Platelet count (109/L), median (Q1, Q3) | 160 (106, 191) | 142 (107, 186) | 169 (121.5, 218) | 0.386 |
Child-Pugh, n [%] | 0.626 | |||
A | 32 [89] | 18 [86] | 14 [93] | |
B | 4 [11] | 3 [14] | 1 [7] | |
TLV (cm3), median (Q1, Q3) | 1,303.59 (1,108.44, 1,571.24) | 1,228.94 (958.3, 1,406.97) | 1,494.95 (1,316.56, 1,821.26) | 0.010* |
TV (cm3), median (Q1, Q3) | 24.84 (10.38, 223.64) | 17.47 (9.49, 57.06) | 55.1 (20.3, 446.55) | 0.033* |
NTV (cm3), median (Q1, Q3) | 2.27 (0.8, 33.05) | 2.3 (0.59, 32.69) | 2.24 (1, 34.78) | 0.553 |
ETV (cm3), median (Q1, Q3) | 7.24 (2.43, 38.91) | 6.17 (2.01, 10.61) | 20.39 (4.86, 80.48) | 0.109 |
NTVP (%), median (Q1, Q3) | 8.7 (5.19, 26.46) | 7.67 (4.85, 9.33) | 33.74 (13.26, 47.17) | 0.004* |
ETVP (%), median (Q1, Q3) | 29.02 (8.3, 63.21) | 37.39 (10.3, 65.58) | 21.85 (5.83, 38.26) | 0.170 |
TB (%), median (Q1, Q3) | 2.03 (1.11, 16.07) | 1.69 (0.77, 6.07) | 5.96 (1.44, 24.49) | 0.062 |
ETB (%), median (Q1, Q3) | 0.63 (0.18, 3.62) | 0.57 (0.18, 0.91) | 0.85 (0.4, 5.65) | 0.238 |
TNB (%), median (Q1, Q3) | 0.16 (0.07, 2.95) | 0.1 (0.04, 0.26) | 1.53 (0.11, 12.46) | 0.012* |
Delta tumor washout, mean ± SD | −5.86±42.53 | −13.86±46.7 | 5.33±34.31 | 0.164 |
Minimum ADC (×10−3 mm2/s), mean ± SD | 0.63±0.24 | 0.65±0.25 | 0.6±0.22 | 0.566 |
Mean ADC (×10−3 mm2/s), mean ± SD | 1.13±0.17 | 1.15±0.17 | 1.11±0.16 | 0.495 |
Maximum ADC (×10−3 mm2/s), mean ± SD | 1.8±0.41 | 1.78±0.45 | 1.83±0.37 | 0.714 |
AP-TLSI (%), mean ± SD | 1.25±0.39 | 1.06±0.31 | 1.39±0.38 | 0.007* |
Nonrim AP hyperenhancement, n [%] | 0.845 | |||
Absent | 9 [25] | 5 [24] | 4 [27] | |
Present | 27 [75] | 16 [76] | 11 [73] | |
Nonperipheral washout, n [%] | 0.559 | |||
Absent | 3 [8] | 1 [5] | 2 [13] | |
Present | 33 [92] | 20 [95] | 13 [87] | |
Enhancing capsule, n [%] | 0.729 | |||
Absent | 11 [31] | 7 [33] | 4 [27] | |
Present | 25 [69] | 14 [67] | 11 [73] | |
Nonenhancing capsule, n [%] | 0.650 | |||
Absent | 30 [83] | 17 [81] | 13 [87] | |
Present | 6 [17] | 4 [19] | 2 [13] | |
Nodule-in-nodule architecture, n [%] | 0.009* | |||
Absent | 20 [56] | 16 [76] | 4 [27] | |
Present | 16 [44] | 5 [24] | 11 [73] | |
Mosaic architecture, n [%] | 0.003* | |||
Absent | 19 [53] | 16 [76] | 3 [20] | |
Present | 17 [47] | 5 [24] | 12 [80] | |
Blood products in mass, n [%] | 0.559 | |||
Absent | 33 [92] | 20 [95] | 13 [87] | |
Present | 3 [8] | 1 [5] | 2 [13] | |
Fat in mass, n [%] | 0.125 | |||
Absent | 32 [89] | 17 [81] | 15 [100] | |
Present | 4 [11] | 4 [19] | 0 [0] | |
Restricted diffusion, n [%] | NA | |||
Present | 36 [100] | 21 [100] | 15 [100] | |
Mild/moderate T2 hyperintensity, n [%] | 0.391 | |||
Absent | 1 [3] | 1 [5] | 0 [0] | |
Present | 35 [97] | 20 [95] | 15 [100] | |
Corona enhancement, n [%] | 0.900 | |||
Absent | 26 [72] | 15 [71] | 11 [73] | |
Present | 10 [28] | 6 [29] | 4 [27] | |
Fat sparing in solid mass, n [%] | 0.729 | |||
Absent | 25 [69] | 14 [67] | 11 [73] | |
Present | 11 [31] | 7 [33] | 4 [27] | |
Iron sparing in solid mass, n [%] | 0.806 | |||
Absent | 2 [6] | 1 [5] | 1 [7] | |
Present | 34 [94] | 20 [95] | 14 [93] | |
LI-RADS category, n [%] | 0.973 | |||
3 | 3 [8] | 2 [10] | 1 [7] | |
4 | 18 [50] | 10 [48] | 8 [53] | |
5 | 12 [33] | 7 [33] | 5 [33] | |
M | 3 [8] | 2 [10] | 1 [7] |
*, P values less than 0.05 were considered statistically significant. VETC, vessels encapsulating tumor clusters; HCC, hepatocellular carcinomas; SD, standard deviation; AFP, alpha-fetoprotein; Q1, quartile 1; Q3, quartile 3; ALT, alanine aminotransferase; AST, aspartate aminotransferase; GGT, gamma-glutamyl transferase; TBIL, total bilirubin; TLV, total liver volume; TV, tumor volume; NTV, necrosis tumor volume; ETV, enhancing tumor volumes; NTVP, necrosis tumor volumetric percentage; ETVP, enhancing tumor volumetric percentage; TB, tumor burden; ETB, enhancing tumor burden; TNB, tumor necrosis burden; ADC, apparent diffusion coefficient; AP-TLSI, tumor-to-liver signal intensity ratio on arterial phase images; AP, arterial phase; LI-RADS, Liver Imaging Reporting and Data System.
Characteristics of VETC-positive and VETC-negative solitary HCC
There were statistically significant differences between the VETC-positive and negative-group for the volumetric parameters of TLV (P=0.010), TV (P=0.033), NTVP (P=0.004), and TNB (P=0.012). The VETC-positive solitary HCCs had a higher TLV, TV, NTVP, and TNB. The median values of TLV, TV, NTVP, TNB were 1,494.95 cm3, 55.1 cm3, 33.74%, and 1.53% in the VETC-positive group, respectively. The median values of TLV, TV, NTVP, and TNB were 1,228.94 cm3, 17.47 cm3, 7.67%, and 0.1% in the VETC-negative group, respectively. For the LI-RADS radiological features, nodule-in-nodule architecture was more frequent in the VETC positive-group (11/15, 73%) than in the VETC-negative group (5/21, 24%) (P=0.009). Mosaic architecture was more common in the VETC-positive group (12/15, 80%) than in the VETC-negative group (5/21, 24%) (P=0.003). There was no statistical difference in remaining LI-RADS radiological features between the VETC-positive group and VETC-negative group (Table 1). Intergroup quantitative parameters analysis showed that VETC-positive solitary HCCs had a significantly higher AP-TLSI value (mean value 1.39%) than did the VETC-negative solitary HCCs (mean value 1.06%) (P=0.007). The comparisons of tumor volumetric parameters, LI-RADS radiological features, and quantitative parameters between two groups are summarized in Table 1.
Predictors for VETC pattern identified by logistic regression analysis
The predictors for VETC pattern according to logistic regression analysis are summarized in Table 2. The results of univariate logistic analysis indicated that the TLV [odds ratio (OR) =1.002; P=0.010], TV (OR =1.004; P=0.033), NTVP (OR =1.115; P=0.004), TNB (OR =1.376; P=0.012), AP-TLSI (OR =1.933; P=0.007), nodule in nodule architecture (OR =8.8, P=0.009), and mosaic architecture (OR =12.8; P=0.003) were significantly correlated with VETC status. However, the results of multivariate logistic analysis indicated that NTVP [OR =1.16; 95% confidence interval (CI): 1.019–1.32; P=0.024] (Figure 2), AP-TLSI (OR =1.993, 95% CI: 1.494–2; P=0.023) (Figure 3), and nodule-in-nodule architecture (OR =17.426; 95% CI: 1.1–275.945, P=0.042) (Figure 4) were independent predictors of VETC status.
Table 2
Parameter | Univariate analysis | Multivariate analysis | |||
---|---|---|---|---|---|
OR (95% CI) | P | OR (95% CI) | P | ||
TLV | 1.002 (1–1.004) | 0.010 | – | – | |
TV | 1.004 (0.999–1.008) | 0.033 | – | – | |
NTVP | 1.115 (1.034–1.202) | 0.004 | 1.16 (1.019–1.32) | 0.024* | |
TNB | 1.376 (0.924–2.051) | 0.012 | – | – | |
AP-TLSI | 1.933 (1.390–1.993) | 0.007 | 1.993 (1.494–2) | 0.023* | |
Nodule-in-nodule architecture | 8.8 (1.92–40.336) | 0.009 | 17.426 (1.1–275.945) | 0.042* | |
Mosaic architecture | 12.8 (2.545–64.372) | 0.003 | – | – |
*, P values less than 0.05 were considered statistically significant. VETC, vessels encapsulating tumor clusters; OR, odds ratio; CI, confidence interval; TLV, total liver volume; TV, tumor volume; NTVP, necrosis tumor volumetric percentage; TNB, tumor necrosis burden; AP-TLSI, tumor-to-liver signal intensity ratio on arterial phase images.
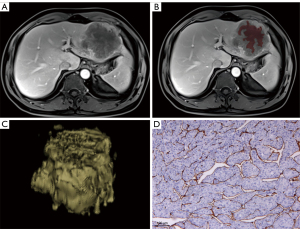
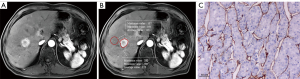
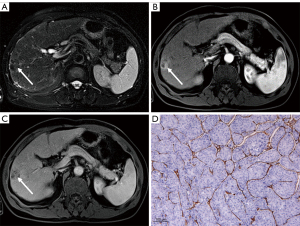
Nomogram construction and evaluation
A multivariate logistic regression model for predicting VETC status was constructed from the independent predictors (Figure 5). The area under the curve (AUC) of this model was 0.942 (95% CI: 0.872–1). The bootstrap resampling method (500 repetitions) was used to perform internal validation on the nomogram, yielding a concordance index of 0.943 (95% CI: 0.874–1). The optimal cutoff threshold on the basis of nomogram score was calculated to be 62.105, and the corresponding sensitivity and specificity were 1.000 and 0.733, respectively. Each patient with solitary HCC and nomogram score higher than the threshold value was assigned to the VETC-positive group, while those with a score lower than the threshold value were assigned to the VETC-negative group. All included patients with solitary HCC were reclassified as per the optimal cutoff, which resulted in an accuracy of 88.89% (Table S2). In addition, a calibration plot was used to visually characterize the agreement between predictions and observations. In the results, the actual and corrected line was closer to the ideal line (Figure 6A). The decision curve of the nomogram is shown in Figure 6B. In DCA, when the threshold probability was higher than 0, the net benefit of the nomogram for VETC status prediction in solitary HCC was greater than that of the two extreme conditions.
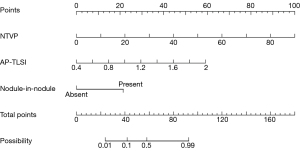
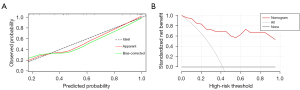
Discussion
In this study, models constructed for preoperative prediction of VETC in solitary HCC were combined with tumor three-dimensional volumetric data, LI-RADS radiological features, and quantitative parameters extracted from contrast-enhanced MRI. The results suggested that NTVP, AP-TLSI, and nodule-in-nodule architecture were independent variables for the prediction of VETC-positive solitary HCC. The visual nomogram displayed good diagnostic efficacy, higher accuracy, good correspondence between actual observations and nomogram prediction and potential clinical benefits in distinguishing the VETC pattern in patients with solitary HCC. In our study, 83% of the patients enrolled had hepatitis B, which could be attributed to patient source, considering all the patients originated from China, where the occurrence of HCC is intimately correlated with the development of hepatitis and liver cirrhosis (22).
A recent study of 170 patients conducted by Feng et al. reported that intratumor necrosis in CT was independently associated with VETC pattern in HCC (13). Our findings demonstrated NTVP derived from contrast-enhanced MRI to be an independent marker of VETC status in patients with solitary HCC and that VETC-positive solitary HCCs had a much higher NTVP. The likely reason for this is that angiogenic activation is characteristic of VETC-positive HCC and promotes its growth (23,24) and that the increased distance between the tumor tissue and the vascular supply could result in hypoxia (25,26). Moreover, the VETC pattern is associated with a local low blood supply due to the compression of the peripheral vascular space (7), and this, along with angiogenesis, hypoxia, and an inadequate blood supply, can lead to substantial necrosis, ultimately resulting in a higher NTVP in VETC-positive HCC. MRI provides multiparametric data and enables high soft-tissue contrast. Thus MRI can measure intratumor necrosis much more accurately than can CT. Hence, our research results could be considered fairly comprehensive. The univariate analyses revealed that TLV, TV, and TNB were associated with VETC-positive solitary HCCs. Nevertheless, in the multivariate analysis, the variables were not found to be significantly different, which could be due to the interaction effects and the small sample size.
Our study indicated that AP-TLSI was an independent predictive factor for VETC-positive status in solitary HCCs, which corresponded to the findings reported by Fan et al. (14). The AP-TLSI values in VETC-positive solitary HCCs were higher than those in VETC-negative solitary HCCs. HCCs presenting AP hyperenhancement may be associated with a decrease in normal vascularity and an increase in neoangiogenesis and sinusoidal capillarization. The AP hyperenhancement might represent the heterogeneity of tumor vascularity and tumor cell proliferation. VETC is a noncapillary network of HCC vessels that is prone to opening into larger vessel and thus manifests as hyperenhancement in the AP (7). Moreover, HCC has an abundant microvasculature, and intratumoral microvessel density is correlated with VETC pattern, possibly because the increase in vascularity can provide suitable environmental conditions for VETC (27). Therefore, a higher SI in the AP (with reference to liver background) was more frequently found in VETC-positive HCCs in our study.
Previous studies have shown that LI-RADS radiological features can provide prognostic information for HCC (16-19). Our study demonstrated that nodule-in-nodule architecture was as an independent predictor for VETC-positive solitary HCCs. Nodule-in-nodule architecture is the existence of a smaller inner nodule with a larger outer nodule that can be distinguished in imaging findings (15). In principle, the inner nodule exhibits characteristics of the progressed HCC, while the outer nodule possesses features of early HCC or a dysplastic nodule (28). In this study, nodule-in-nodule architecture was more commonly found in the VETC-positive group. One possible explanation for this is that hypervascularity of the inner nodule at the early stage of HCC is more likely to develop into VETC and dedifferentiation (8,29). Additionally, mosaic architecture was significantly associated with VETC status in the univariate analyses; however, in the multivariate analysis, this variable was not significant. Mosaic architecture is known as a marker of tumor heterogeneity, and the internal nodules may vary according to in biologic behavior; for instance, a more prominent mosaic architecture tends to indicate vascular invasion (28), which may explain the association with VETC status found in our study to some extent. Other studies (8,13,14) have reported that serum AFP level, AST level, and tumor size larger than 5 cm are significant independent variables for the prediction of VETC-positive HCC. In our study, however, no significant difference was found between these factors and VETC pattern, which may be due to the heterogeneity of the study populations.
Ultimately, our nomogram had good diagnostic performance, with an AUC of 0.942 and a predicted accuracy of 88.89%. Fan et al. (14,30) established two models for predicting VETC-positive HCC, with AUCs of 0.885 and 0.844, respectively. The model in our study yielded a relatively better performance. This could be because NTVP with greater weighting in our model was a larger contributor to the nomogram. Previous studies have reported that intratumor necrosis, AP hyperenhancement, and VETC pattern are independent indicators for poor prognosis and that nodule-in-nodule architecture is associated with advanced HCC (8,31,32). In addition, other research indicates that anatomic hepatectomy could reduce early recurrence in patients with VETC-positive HCC and that VETC-positive HCC may benefit from adjuvant transcatheter arterial chemoembolization (TACE) or sorafenib therapy (5,10,33). Consequently, for patients with high-risk solitary HCC and VETC-positive status as indicated by our nomogram, we suggest first-line treatment with nonanatomical hepatectomy should be performed with great caution and that anatomic hepatectomy and that postoperative adjuvant therapies should be used as for alternative treatment and short-term monitoring.
It is also necessary to discuss several limitations in our study. First, intermediate- and advanced-stage HCC constitute a large proportion of HCC cases in China due to the prevalence of hepatitis and liver cirrhosis. These particular patients with HCC tend to be more suited for TACE or systemic therapy, which can lead to small samples. Moreover, selection bias was inevitable due to the retrospective, single-institution design; inadequate sample size; and lack of external assessment. Second, postoperative survival data were not available due to poor patient compliance. Therefore, a multicenter study with a larger sample size and a full complement of prognostic data that can address these limitations as much as possible is needed to verify and optimize these preliminary results. Third, our study used extracellular contrast media. Thus, hepatobiliary-phase radiological characteristics were not assessed. However, these features may be relevant to determining real-the world clinical value and generalizability, as hepatocyte-specific contrast agent is expensive and not regularly used in primary institutions. Fourth, intrahepatic vascular structures were included at TLV measurements, as they are typically visualized as a whole. Finally, radiomics, deep learning, and artificial intelligence approaches derived from medical images have the potential to enhance predictive analytics, and our step future studies should incorporate these approaches.
Conclusions
Although we conducted a small-sample exploratory study with preliminary results, the nomogram we developed to preoperatively predict VETC-positive status in patients with solitary HCC achieved a good diagnostic performance and classification accuracy. These findings support the potential of applying LI-RADS MR features and measuring three-dimensional TV for the preoperative assessment of HCC invasiveness.
Acknowledgments
Funding: This work was supported by
Footnote
Reporting Checklist: The authors have completed the TRIPOD reporting checklist. Available at https://qims.amegroups.com/article/view/10.21037/qims-24-315/rc
Conflicts of Interest: All authors have completed the ICMJE uniform disclosure form (available at https://qims.amegroups.com/article/view/10.21037/qims-24-315/coif). The authors have no conflicts of interest to declare.
Ethical Statement: The authors are accountable for all aspects of the work in ensuring that questions related to the accuracy or integrity of any part of the work are appropriately investigated and resolved. This study was conducted in accordance with the Declaration of Helsinki (as revised in 2013) and was approved by the Institutional Review Board of Chongqing General Hospital (No. s2019-038-01). Written informed consent was waived due to the retrospective nature of the research.
Open Access Statement: This is an Open Access article distributed in accordance with the Creative Commons Attribution-NonCommercial-NoDerivs 4.0 International License (CC BY-NC-ND 4.0), which permits the non-commercial replication and distribution of the article with the strict proviso that no changes or edits are made and the original work is properly cited (including links to both the formal publication through the relevant DOI and the license). See: https://creativecommons.org/licenses/by-nc-nd/4.0/.
References
- Sung H, Ferlay J, Siegel RL, Laversanne M, Soerjomataram I, Jemal A, Bray F. Global Cancer Statistics 2020: GLOBOCAN Estimates of Incidence and Mortality Worldwide for 36 Cancers in 185 Countries. CA Cancer J Clin 2021;71:209-49. [Crossref] [PubMed]
- Yao LQ, Chen ZL, Feng ZH, Diao YK, Li C, Sun HY, Zhong JH, Chen TH, Gu WM, Zhou YH, Zhang WG, Wang H, Zeng YY, Wu H, Wang MD, Xu XF, Pawlik TM, Lau WY, Shen F, Yang T. Clinical Features of Recurrence After Hepatic Resection for Early-Stage Hepatocellular Carcinoma and Long-Term Survival Outcomes of Patients with Recurrence: A Multi-institutional Analysis. Ann Surg Oncol 2022; Epub ahead of print. [Crossref] [PubMed]
- Marrero JA, Kulik LM, Sirlin CB, Zhu AX, Finn RS, Abecassis MM, Roberts LR, Heimbach JK. Diagnosis, Staging, and Management of Hepatocellular Carcinoma: 2018 Practice Guidance by the American Association for the Study of Liver Diseases. Hepatology 2018;68:723-50. [Crossref] [PubMed]
- Cancer Genome Atlas Research Network. Electronic address: wheeler@bcm.edu; Cancer Genome Atlas Research Network. Comprehensive and Integrative Genomic Characterization of Hepatocellular Carcinoma. Cell 2017;169:1327-1341.e23. [Crossref]
- Liu K, Dennis C, Prince DS, Marsh-Wakefield F, Santhakumar C, Gamble JR, Strasser SI, McCaughan GW. Vessels that encapsulate tumour clusters vascular pattern in hepatocellular carcinoma. JHEP Rep 2023;5:100792. [Crossref] [PubMed]
- Jou J, Diehl AM. Epithelial-mesenchymal transitions and hepatocarcinogenesis. J Clin Invest 2010;120:1031-4. [Crossref] [PubMed]
- Fang JH, Zhou HC, Zhang C, Shang LR, Zhang L, Xu J, Zheng L, Yuan Y, Guo RP, Jia WH, Yun JP, Chen MS, Zhang Y, Zhuang SM. A novel vascular pattern promotes metastasis of hepatocellular carcinoma in an epithelial-mesenchymal transition-independent manner. Hepatology 2015;62:452-65. [Crossref] [PubMed]
- Renne SL, Woo HY, Allegra S, Rudini N, Yano H, Donadon M, Viganò L, Akiba J, Lee HS, Rhee H, Park YN, Roncalli M, Di Tommaso L. Vessels Encapsulating Tumor Clusters (VETC) Is a Powerful Predictor of Aggressive Hepatocellular Carcinoma. Hepatology 2020;71:183-95. [Crossref] [PubMed]
- Ding T, Xu J, Zhang Y, Guo RP, Wu WC, Zhang SD, Qian CN, Zheng L. Endothelium-coated tumor clusters are associated with poor prognosis and micrometastasis of hepatocellular carcinoma after resection. Cancer 2011;117:4878-89. [Crossref] [PubMed]
- Fang JH, Xu L, Shang LR, Pan CZ, Ding J, Tang YQ, Liu H, Liu CX, Zheng JL, Zhang YJ, Zhou ZG, Xu J, Zheng L, Chen MS, Zhuang SM. Vessels That Encapsulate Tumor Clusters (VETC) Pattern Is a Predictor of Sorafenib Benefit in Patients with Hepatocellular Carcinoma. Hepatology 2019;70:824-39. [Crossref] [PubMed]
- Ao J, Chiba T, Kanzaki H, Kanayama K, Shibata S, Kurosugi A, et al. Serum Angiopoietin 2 acts as a diagnostic and prognostic biomarker in hepatocellular carcinoma. J Cancer 2021;12:2694-701. [Crossref] [PubMed]
- Yu Y, Fan Y, Wang X, Zhu M, Hu M, Shi C, Hu C. Gd-EOB-DTPA-enhanced MRI radiomics to predict vessels encapsulating tumor clusters (VETC) and patient prognosis in hepatocellular carcinoma. Eur Radiol 2022;32:959-70. [Crossref] [PubMed]
- Feng Z, Li H, Zhao H, Jiang Y, Liu Q, Chen Q, Wang W, Rong P. Preoperative CT for Characterization of Aggressive Macrotrabecular-Massive Subtype and Vessels That Encapsulate Tumor Clusters Pattern in Hepatocellular Carcinoma. Radiology 2021;300:219-29. [Crossref] [PubMed]
- Fan Y, Yu Y, Hu M, Wang X, Du M, Guo L, Hu C. Imaging features based on Gd-EOB-DTPA-enhanced MRI for predicting vessels encapsulating tumor clusters (VETC) in patients with hepatocellular carcinoma. Br J Radiol 2021;94:20200950. [Crossref] [PubMed]
- Chernyak V, Fowler KJ, Kamaya A, Kielar AZ, Elsayes KM, Bashir MR, Kono Y, Do RK, Mitchell DG, Singal AG, Tang A, Sirlin CB. Liver Imaging Reporting and Data System (LI-RADS) Version 2018: Imaging of Hepatocellular Carcinoma in At-Risk Patients. Radiology 2018;289:816-30. [Crossref] [PubMed]
- Song W, Chen Q, Guo D, Jiang C. Preoperative estimation of the survival of patients with unresectable hepatocellular carcinoma achieving complete response after conventional transcatheter arterial chemoembolization: assessments of clinical and LI-RADS MR features. Radiol Med 2022;127:939-49. [Crossref] [PubMed]
- Wei H, Jiang H, Zheng T, Zhang Z, Yang C, Ye Z, Duan T, Song B. LI-RADS category 5 hepatocellular carcinoma: preoperative gadoxetic acid-enhanced MRI for early recurrence risk stratification after curative resection. Eur Radiol 2021;31:2289-302. [Crossref] [PubMed]
- Wei H, Jiang H, Liu X, Qin Y, Zheng T, Liu S, Zhang X, Song B. Can LI-RADS imaging features at gadoxetic acid-enhanced MRI predict aggressive features on pathology of single hepatocellular carcinoma? Eur J Radiol 2020;132:109312. [Crossref] [PubMed]
- Chen J, Zhou J, Kuang S, Zhang Y, Xie S, He B, Deng Y, Yang H, Shan Q, Wu J, Sirlin CB, Wang J. Liver Imaging Reporting and Data System Category 5: MRI Predictors of Microvascular Invasion and Recurrence After Hepatectomy for Hepatocellular Carcinoma. AJR Am J Roentgenol 2019;213:821-30. [Crossref] [PubMed]
- Choi SY, Kim SH, Park CK, Min JH, Lee JE, Choi YH, Lee BR. Imaging Features of Gadoxetic Acid-enhanced and Diffusion-weighted MR Imaging for Identifying Cytokeratin 19-positive Hepatocellular Carcinoma: A Retrospective Observational Study. Radiology 2018;286:897-908. [Crossref] [PubMed]
- Koo TK, Li MY. A Guideline of Selecting and Reporting Intraclass Correlation Coefficients for Reliability Research. J Chiropr Med 2016;15:155-63. [Crossref] [PubMed]
- Sankar K, Gong J, Osipov A, Miles SA, Kosari K, Nissen NN, Hendifar AE, Koltsova EK, Yang JD. Recent advances in the management of hepatocellular carcinoma. Clin Mol Hepatol 2024;30:1-15. [Crossref] [PubMed]
- Calderaro J, Meunier L, Nguyen CT, Boubaya M, Caruso S, Luciani A, et al. ESM1 as a Marker of Macrotrabecular-Massive Hepatocellular Carcinoma. Clin Cancer Res 2019;25:5859-65. [Crossref] [PubMed]
- Calderaro J, Couchy G, Imbeaud S, Amaddeo G, Letouzé E, Blanc JF, Laurent C, Hajji Y, Azoulay D, Bioulac-Sage P, Nault JC, Zucman-Rossi J. Histological subtypes of hepatocellular carcinoma are related to gene mutations and molecular tumour classification. J Hepatol 2017;67:727-38. [Crossref] [PubMed]
- Sin SQ, Mohan CD, Goh RMW, You M, Nayak SC, Chen L, Sethi G, Rangappa KS, Wang L. Hypoxia signaling in hepatocellular carcinoma: Challenges and therapeutic opportunities. Cancer Metastasis Rev 2023;42:741-64. [Crossref] [PubMed]
- Villa E, Critelli R, Lei B, Marzocchi G, Cammà C, Giannelli G, et al. Neoangiogenesis-related genes are hallmarks of fast-growing hepatocellular carcinomas and worst survival. Results from a prospective study. Gut 2016;65:861-9. [Crossref] [PubMed]
- Huang CW, Lin SE, Huang SF, Yu MC, Tang JH, Tsai CN, Hsu HY. The Vessels That Encapsulate Tumor Clusters (VETC) Pattern Is a Poor Prognosis Factor in Patients with Hepatocellular Carcinoma: An Analysis of Microvessel Density. Cancers (Basel) 2022.
- Chernyak V, Tang A, Flusberg M, Papadatos D, Bijan B, Kono Y, Santillan C. LI-RADS(®) ancillary features on CT and MRI. Abdom Radiol (NY) 2018;43:82-100. [Crossref] [PubMed]
- Xing F, Ma Q, Lu J, Zhu W, Du S, Jiang J, Zhang T, Xing W. Nodule-in-nodule architecture of hepatocellular carcinomas: enhancement patterns in the hepatobiliary phase and pathological features. Abdom Radiol (NY) 2024; Epub ahead of print. [Crossref] [PubMed]
- Fan Y, Yu Y, Wang X, Hu M, Du M, Guo L, Sun S, Hu C. Texture Analysis Based on Gd-EOB-DTPA-Enhanced MRI for Identifying Vessels Encapsulating Tumor Clusters (VETC)-Positive Hepatocellular Carcinoma. J Hepatocell Carcinoma 2021;8:349-59. [Crossref] [PubMed]
- Cheng J, Li X, Wang L, Chen F, Li Y, Zuo G, Pei M, Zhang H, Yu L, Liu C, Wang J, Han Q, Cai P, Li X. Evaluation and Prognostication of Gd-EOB-DTPA MRI and CT in Patients With Macrotrabecular-Massive Hepatocellular Carcinoma. J Magn Reson Imaging 2024;59:2071-81. [Crossref] [PubMed]
- Wang L, Feng B, Li D, Liang M, Wang S, Wang S, Ma X, Zhao X. Risk stratification of solitary hepatocellular carcinoma ≤ 5 cm without microvascular invasion: prognostic values of MR imaging features based on LI-RADS and clinical parameters. Eur Radiol 2023;33:3592-603. [Crossref] [PubMed]
- Wang JH, Li XS, Tang HS, Fang RY, Song JJ, Feng YL, Guan TP, Ruan Q, Wang J, Cui SZ. Vessels that encapsulate tumor clusters (VETC) pattern predicts the efficacy of adjuvant TACE in hepatocellular carcinoma. J Cancer Res Clin Oncol 2023;149:4163-72. [Crossref] [PubMed]