A deep learning algorithm for the detection of aortic dissection on non-contrast-enhanced computed tomography via the identification and segmentation of the true and false lumens of the aorta
Introduction
Acute aortic dissection represents a life-threatening and critical medical emergency, holding significant implications for human health. Characterized by its sudden onset, rapid progression, and the challenges it presents in diagnosis and treatment, acute aortic dissection is often misdiagnosed (1). Most patients with acute aortic dissection will have clinical manifestations such as severe chest and back pain or abdominal pain and an increased blood pressure gap between the upper and lower limbs. Nevertheless, certain patients may experience atypical symptoms, including mild chest pain or tightness, dizziness, and nausea (2). Chest pain is also one of the most common reasons for emergency room visits and may result from a wide array of diseases. Given this complexity, emergency physicians often favor chest computed tomography (CT) scans over routine aortic CT angiography (CTA) for initial evaluation (3,4).
Aortic CTA is extensively used owing to its high sensitivity and specificity and has increasingly been recognized as the gold standard for diagnosing aortic dissection and assessing prognosis (5). However, technological limitations and constrained rescue capabilities, coupled with the critical condition of most patients with aortic dissection and potential allergic reactions to contrast media, pose challenges for small hospitals in conducting emergency aortic CTA examinations. A critical concern is the unknown renal function status of emergency patients. Conducting CTA examination in patients with preexisting renal insufficiency risks exacerbating renal damage, potentially leading to renal failure (6,7). Chest CT scans are commonly employed in emergency settings due to their ease of operation and comparatively lower risks than those of CTA.
From an economic standpoint, a chest CT scan is approximately one-tenth the cost of a thoracoabdominal aortic CTA examination (8,9). However, current CT technology is limited in its ability to delineate aortic lesions, which may lead to missed diagnoses of aortic dissection, consequently delaying optimal treatment (10,11).
Although CTA can demonstrate clear vascular structures, it has some disadvantages (12). CTA is contraindicated in patients allergic to iodine, as most contrast agents are iodine-based. In addition, the use of contrast media requires an intravenous indwelling needle. This causes additional discomfort and may lead to associated complications, including damage to punctured vessels and skin damage from the extravasation of contrast material. Furthermore, contrast media are nephrotoxic, with an incidence of acute kidney injury (contrast nephropathy) as high as 12% following their use (13). This is mainly a problem in the older adult population, who either have reduced baseline renal function or chronic kidney disease, and in emergency patients with unclear renal function status. In these high-risk patients, there is a recognized risk of complete renal failure, which may lead to subsequent renal dialysis treatment (14,15).
Recent advancements in computer algorithms and hardware, along with the extensive expansion of medical data sets, have significantly enhanced the application of artificial intelligence (AI) in the medical domain (16,17). Several network models capable of synthesizing enhanced CT images based on noncontrast CT scans have been developed to aid clinical diagnosis (18,19). In this study, we developed a no new U-Net (nnU-Net) based deep learning network model that segments the true and false lumen of the aorta in non-contrast-enhanced computed tomography (NCE-CT) images, thereby determining the presence of aortic dissection.
As X-rays traverse a patient’s body, they are attenuated by the density of the tissues encountered. Variations in the physical density of different objects translate into differences in attenuation and subsequent radiodensity [measured in Hounsfield units (HU)] on the CT scan. The higher the attenuation is, the brighter the CT image (such as bone and calcifications); the lower the attenuation, the darker the CT image (such as air). Thus, the inherent contrast in the image arises from the differences in attenuation between adjacent tissues (20). Within the X-ray energy spectrum utilized in current medical CT scans (80–120 KeV), a subtle difference in the X-ray attenuation coefficient is expected between the aortic intima and blood. This distinction can be discerned through a comprehensive analysis of non-contrast-enhanced CT (NCE-CT) images. Consequently, we developed a deep learning algorithm to segment the potential true and false lumens in the aorta from NCE-CT scan images, thereby facilitating the determination of the presence of aortic dissection (AD). We present this article in accordance with the TRIPOD+AI reporting checklist (available at https://qims.amegroups.com/article/view/10.21037/qims-24-533/rc).
Methods
Patients
This retrospective cohort study was conducted in accordance with the Declaration of Helsinki (as revised in 2013) and was approved by the Institutional Review Boards (IRBs) of Fujian Provincial Hospital (IRB approval No. K2020-03-013), Beijing Anzhen Hospital (IRB No. 2024156x), and Xiangya Hospital (IRB No. 202008190). The requirement for individual consent was waived due to the retrospective nature of the analysis.
From May 2020 to May 2022, this retrospective study included 320 consecutive patients across three centers: Beijing Anzhen Hospital affiliated with Capital Medical University (100 cases), Fujian Provincial Hospital (156 cases), and Xiangya Hospital of Central South University (64 cases). All patients underwent simultaneous CE-CT examination and NCE-CT scans. The cohort consisted of 160 patients diagnosed with aortic dissection and 160 without aortic dissection. The exclusion criteria included patients who had undergone aortic dissection surgery and those with only noncontrast scans or exclusively CTA images. This study employed images from CT machines from a various manufacturers across the three hospitals.
Image data acquisition
NCE-CT and CE-CT images included the region from 3 cm above the aortic arch to the bifurcation level of the bilateral iliac arteries. Each patient underwent sequential NCE-CT and CE-CT scans with the same scan position, coverage, and parameters. The matrix size was 512×512, and the pixel size was 0.977×0.977 mm. The slice thickness varied, with a resolution ranging from 0.625 to 1.250 mm. CT images were acquired using GE HealthCare (Chicago, IL, USA), Philips (Amsterdam, the Netherlands), and Siemens Healthineers (Erlangen, Germany) devices operating at a kilovoltage peak range of 100–120.
Image preprocessing
This study adopted the following image preprocessing steps to ensure that the images used for training and testing were normalized.
- Image format conversion: all CT images initially in Digital Imaging and Communications in Medicine (DICOM) format were converted to nii format using ITK-SNAP software.
- Spatial scale standardization: the pixel spacing of the images in the x and y directions was 0.76 mm, and the spacing in the z direction was 1.25 mm.
- Scale determination method: images were sorted based on attributes in the training dataset, and the median value was used.
- Normalization: CT values ranged from −751 to 215 HU, with an average of 32.79 HU and a standard deviation of 94.28 HU. The normalized value calculation method was mean and standard deviation.
- Aorta localization and image cropping: the lung area location was used as a reference for aorta localization, and a central volume of 250×250 mm was used for depth cropping.
These preprocessing steps were performed to eliminate differences caused by different machines and imaging conditions, standardize the image data, and ensure consistency and comparability during training and testing.
In the training phase, a union bounding box encapsulating the true and false aortic lumens, as labeled, was established and subsequently expanded by 5 pixels in each direction: left, right, anterior, superior, and inferior. Padding with zeros was applied at the image boundary if necessary. In the inference phase, this procedure expedited the inference process and narrowed the inference scope. Alternatively, the entire volumetric data could be fed directly into the network model, omitting the volume of interest (VOI) extraction. The center in X and Y directions was determined by the lung lobe mask segmented from the lung area. From this central point, a 15-cm expansion was applied laterally and anteroposteriorly in the X and Y axes. The entire range was used in the Z-axis to delineate the bounding box, which defined the VOI for aortic segmentation inference. This method could ensure that only specific volume regions were focused on during the aortic segmentation process, thus improving computational efficiency and inference accuracy.
The application of these preprocessing methods had a considerable impact on the algorithm’s performance. The improvements in image quality facilitated easier analysis and recognition of features by the algorithm. Spatial rescaling and normalization played a crucial role in eliminating discrepancies in the images, leading to more stable and accurate image processing by the algorithm. Active localization and image cropping significantly reduced redundant information, enhancing computational efficiency and increasing the precision in the targeting of regions of interest, thereby boosting the algorithm’s recognition accuracy.
Algorithm development
Deep learning algorithms were developed using PyTorch version 1.8.2. This study employed the nnU-Net architecture for the convolutional neural network, which is an advanced iteration of the U-Net network. The predominant thickness of the image layers used in this study was 1.25 mm, with a maximum layer thickness maintained below 3.75 mm. Three-dimensional (3D) volume data were employed to train the algorithm in comprehensively using spatial information. Within the nnU-Net framework, 20% of the samples were randomly designated as the verification set. Owing to the random retention of 20% of the data for model evaluation during dataset preparation, training was confined to the default fold of 0, culminating in the derivation of the algorithm model. After these processes were completed, the algorithm model’s performance was rigorously evaluated.
The U-Net architecture serves as a foundational framework of the nnU-Net. The 3D full-resolution U-Net, a deep learning algorithm, excels in processing and analyzing volumetric data. This model employs U-Net’s design principles and extends them into a 3D context, enhancing its ability to capture intricate details within volumetric datasets. The primary objective of the 3D full-resolution U-Net is to produce high-fidelity mask images, thereby elevating both the accuracy and resolution of medical image analysis.
The 3D full-resolution U-Net is architecturally designed with an encoder-decoder structure, enabling a continuous computation of image features from detailed to abstract levels, thus facilitating a multiscale representation of features. The encoder and decoder components are finely tuned for efficient extraction of features and reconstruction of images in a 3D context. The network architecture of the 3D full-resolution U-net is primarily composed of three integral components: the encoder, the decoder, and the residual connection block (Figure 1). Upon receiving volumetric data as input, the model executes a series of 3D operations, encompassing convolution, maximum pooling, and upconvolution processes (21,22).
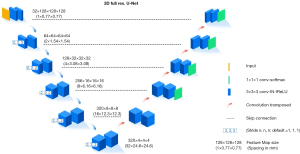
Establishment of the algorithm model
In this study, we tried to develop a deep learning-based algorithm for identifying the true and false lumens in the aorta on NCE-CT scans. The algorithm model was established as follows:
- In the initial dataset, the CE-CT images from 50 patients (comprising 25 AD) cases and 25 without AD] were annotated by radiologists to delineate true and false lumens via ITK-SNAP software, generating “Mask 1”.
- Through supervised learning and the incorporation of Mask 1 and enhanced image data, the 3D full-resolution U-net network developed “Algorithm 1”, which constructed masks for aortic true and false lumens from CE-CT images.
- Masks delineating the aortic true and false lumens, as identified by Algorithm 1, were overlaid on corresponding NCE-CT images. Notably, these visibly discernible masks often exhibited varying degrees of displacement relative to the NCE-CT image. The outlines of the aorta in NCE-CT images were used to modify these masks, resulting in the creation of “Mask 2” (Figure 2).
- Subsequently, “Algorithm 2” was generated using the 3D full-resolution U-net network, which employed supervised learning with Mask 2 and NCE-CT images to create masks for the aortic true and false lumens derived from NCE-CT images.
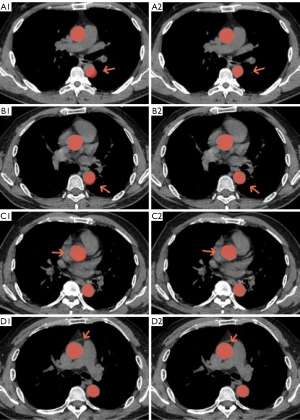
Algorithm 1 was engineered to streamline the labeling process, simplify the task, and enhance label accuracy. Subsequently, Mask 1, produced by this algorithm, was overlaid onto the corresponding NCE-CT aortic images. Mask 2, derived from NCE-CT images, could be acquired more rapidly and with greater precision, minimizing the need for annotation adjustments and thereby creating an efficient training supervision sample.
In the study’s initial phase, CE-CT images were manually annotated to develop Mask 1. Mask 1 was then adjusted on NCE-CT images to create Mask 2. Consequently, upon being trained and acquiring new data, Algorithm 1 generated Mask 1’ from the new CT images, which was then refined based on the CE-CT images to yield Mask 1. This was subsequently aligned with the NCE-CT images and modified to produce Mask 2.
The following steps involved adjusting Mask 2 in NCE-CT images. This process mainly consisted of using Mask 1 from CE-CT images to update and correct Mask 2 in NCE-CT images:
- Generation of the initial mask using the ITK-SNAP software. This involved the manual annotation of the aorta’s true and false lumen contours in CE-CT images, creating the initial Mask 1.
- Training of the initial algorithm. A supervised learning method was used to combine Mask 1 and enhanced image data, and the 3D full-resolution U-net network was used to train Algorithm 1. This algorithm could generate masks of the aorta’s true and false lumens from CE-CT images.
- Preliminary correction. The mask generated by Algorithm 1 was overlaid onto the corresponding NCE-CT images. These masks typically displayed varying degrees of displacement in the NCE-CT images. The aortic contours in NCE-CT images were used to adjust these mask, forming Mask 2.
- Training of the final algorithm. Mask 2 and NCE-CT images were used to train Algorithm 2 through supervised learning, generating masks of the aorta’s true and false lumens from NCE-CT images.
While the task of adjusting masks in nondissection aortic regions is relatively straightforward due to the regular aortic shape, areas with dissection pose unique challenges. The highly irregular shapes of the true and false lumens make transitioning from one mask to another difficult. For our study, this required detailed adjustments made via the annotation software, such as translation, additions, or edge smoothing, to better match the aortic contours in NCE-CT image.
This process was intended to improve accuracy through continuous algorithm updates, ultimately reducing misdiagnosis and the workload of manual annotations, thereby enhancing clinical diagnostic efficiency and patient care quality.
Figure 3 is a flowchart of a medical imaging study illustrating a complex process for evaluating aortic dissection using CT image processing.
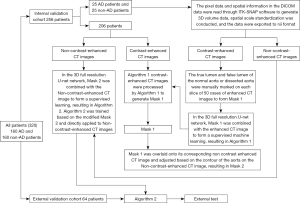
The process began with a sample of 320 patients divided into 160 patients with aortic dissection and 160 patients without aortic dissection. First, the these patients were divided into two groups: an internal validation cohort, comprising 256 patients (including the original 25 and 25 patients with and without aortic dissection, respectively), and an external validation cohort, comprising 64 patients.
In the internal validation cohort, the patient’s CT images were further categorized into CE-CT and NCE-CT images. The CE-CT images underwent further processing, including (I) the differentiation of the true and false lumens of the aortic wall on 50 manually labeled CT slices and (II) the conversion of the labeled images into a mask (Mask 1) through use of a 3D full-resolution U-Net (a deep learning model).
There were two critical algorithms in the process (Algorithm 1 and Algorithm 2): Algorithm 1 converted the CE-CT images into Mask 1; Algorithm 2 was used in a more complex advanced step that combined Mask 2 (which was generated by the 3D full-resolution U-Net network) with NCE-CT images for supervised learning, resulting in the final model learning outcomes.
Finally, the generated Mask 1 was overlaid onto its corresponding NE-CT images and adjusted according to the aortic contour on the NE-CT images to form Mask 2. This process was used for external testing to validate the method’s effectiveness.
Algorithm 2 (Figure 4), the focal point of this study, discerns between true and false aortic lumens in NCE-CT images. A dissection is identified when a false lumen is present and its volume exceeds 5 mL.
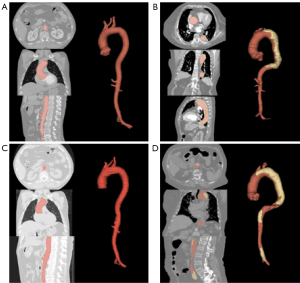
Furthermore, we conducted comparative analyses of the ability to diagnose aortic dissection from NCE-CT images between our developed model and three other models: pyramid attention (PA)-Net, UNetsub, and UNetplus (23-25).
- PA-Net: PA-Net is a network model that combines PA mechanisms and U-Net structures. It enhances the capture ability of details and global information through multiscale feature fusion and attention mechanisms, thereby improving the accuracy of image segmentation. PA-Net performs exceptionally well in medical image segmentation tasks, especially when dealing with images with complex structures.
- UNetsub: UNetsub is a variant of the traditional U-Net subnetwork structure and is focused on lightweight and efficiency improvements. It increases inference speed by reducing the number of model parameters and computations while maximally maintaining segmentation performance stability. UNetsub is typically used in scenarios requiring fast processing and deployment.
- UNetplus: UNetplus is an improved version of U-Net that further enhances segmentation performance by introducing multihop paths and dense skip connections. Its core principle involves improving the connection structure between the encoder and decoder, enhancing the fusion of multiscale features, reducing information loss, and improving the model’s representation capability. UNetplus has shown excellent performance in various medical image segmentation tasks.
The other essential information for the training of the other three models is listed below:
- The image preprocessing strategies were entirely consistent.
- For the training sampling strategy, foreground regions that accounted for at least 10% of the total voxel count in a patch size of 128×128×96 were randomly selected.
- The validation sampling strategy consisted of sliding window inference.
- Data augmentation included 20% random scaling from 0.8 to 1.3, a 20% random rotation from −15° to 15°, a 20% probability of elastic transformation, random blur, and Z normalization.
- The hyperparameters were as follows: first_out_channels, 16; learning rate, 1e−2; batch size, 4; epochs, 1,000; loss, cross-entropy + Dice; optimization algorithm, Adam; activation function, rectified linear unit (ReLU) for all layers except the final one, for which sigmoid was used.
Establishment of a volume threshold for the false lumen in dissection classification
Segmentation labels for the true and false aortic lumens underwent statistical analysis, with prediction being derived from NCE-CT images. The volume of the false lumen, as indicated in these labels, was quantified and assessed against dissection classification. A receiver operating characteristic (ROC) curve was employed to establish 5 mL as the volume threshold for dissection determination. The ROC curve data are listed in Table 1.
Table 1
Case | False positive rate | Recall (true positive rate) | Threshold |
---|---|---|---|
1 | 0.0 | 0.0 | Inf |
2 | 0.0 | 0.07272727 | 278.395477 |
3 | 0.0 | 0.09090909 | 269.327822 |
4 | 0.0 | 0.14545455 | 185.599574 |
5 | 0.0 | 0.16363636 | 170.596194 |
6 | 0.0 | 0.2 | 159.71359 |
7 | 0.0 | 0.23636364 | 139.058075 |
8 | 0.0 | 0.30909091 | 138.996856 |
9 | 0.0 | 0.32727273 | 134.703968 |
10 | 0.0 | 0.41818182 | 124.550177 |
11 | 0.0 | 0.45454545 | 120.420577 |
12 | 0.0 | 0.47272727 | 119.52183 |
13 | 0.0 | 0.54545455 | 93.1567159 |
14 | 0.0 | 0.56363636 | 88.3496475 |
15 | 0.0 | 0.61818182 | 81.2753556 |
16 | 0.0 | 0.69090909 | 54.8887402 |
17 | 0.0 | 0.72727273 | 15.4700055 |
18 | 0.0 | 0.76363636 | 9.97263304 |
19 | 0.0 | 0.78181818 | 6.44426137 |
20 | 0.0 | 0.81818182 | 5.17435455 |
21 | 0.13043478 | 0.81818182 | 4.13467421 |
22 | 0.13043478 | 0.83636364 | 0.615509033 |
23 | 0.2173913 | 0.83636364 | 0.236945688 |
24 | 1.0 | 1.0 | 0.0 |
ROC, receiver operating characteristic; inf, infinity.
Based on the current ROC curve data, the optimal threshold (threshold) based on Youden’s J statistic was 5.17435455 while that for the top-left corner was 5.17435455. Both methods yielded the same optimal threshold, 5.17435455 (rounded to 5 mL). This threshold performed the best in balancing the false-positive rate and recall (Figure 5).
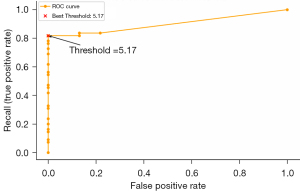
Statistical analysis
Statistical analyses were conducted using the Pandas 1.5.1 package in Python software version 3.8.16 (Wilmington, DE, USA). We assessed differences in clinical factors and CT findings between patients with and without dissection using independent t-tests or Mann-Whitney tests for continuous variables and the Fisher exact test or Chi-squared test for categorical variables. We calculated the differences in the accuracy, sensitivity, and specificity between the algorithm and each radiologist to evaluate the algorithm’s performance relative to the average radiologist. These differences were compared using a one-sample t-test (N=3). Furthermore, we used the McNemar test with Bonferroni correction to statistically examine the differences in accuracy, sensitivity, and specificity between the algorithm and each radiologist. During the validation of the aortic dissection assay, ROC analysis was employed to ascertain the area under the curve (AUC) for each unmasked and masked group. The two AUCs were compared via the DeLong method. A P value <0.05 denoted statistical difference.
Results
A total of 320 patients were included in this study, among whom 160 had aortic dissection (Stanford type A: n=75; Stanford type B: n=85) and 160 did not. The clinical factors and CT parameters of patients in the training set (n=206), validation set (n=50), and external test set (n=64) are listed in Table 2.
Table 2
Characteristic | Training set (n=206) | Validation set (n=50) | External test set (n=64) | ||||||||
---|---|---|---|---|---|---|---|---|---|---|---|
AD | Non-AD | P value | AD | Non-AD | P value | AD | Non-AD | P value | |||
Age (years) | 68.4±11.0 | 72.2±10.8 | <0.05 | 69.2±11.8 | 69.8±11.0 | <0.05 | 71.6±9.0 | 68.9±10.5 | <0.05 | ||
Gender | – | – | – | ||||||||
Male | 86 | 88 | 20 | 17 | 25 | 21 | |||||
Female | 17 | 15 | 5 | 8 | 7 | 11 | |||||
Chest or back pain | – | – | – | ||||||||
Yes | 92 | 98 | 23 | 21 | 29 | 18 | |||||
No | 11 | 5 | 2 | 4 | 3 | 14 | |||||
CT image parameters | |||||||||||
Slice thickness (mm) | 0.625 | 0.625 | – | 0.625 | 0.625 | – | 0.625 | 0.625 | – | ||
Stanford type | – | – | – | ||||||||
A | 49 | 0 | 11 | 0 | 15 | 0 | |||||
B | 54 | 0 | 14 | 0 | 17 | 0 |
Data are presented as mean ± standard deviation or number. AD, aortic dissection; CT, computed tomography.
Three radiologists evaluated the NCE-CT images in the internal test set and the external validation set to determine whether there was a dissection.
For the internal test set and external validation set, the Fleiss kappa coefficients for the three radiologists were 0.72 and 0.76, respectively, indicating moderate agreement. Our model demonstrated superior or equivalent accuracy to that of the three radiologists in the internal and external datasets, although the difference was not statistically significant. Our model exhibited higher sensitivity compared to all three radiologists. For the internal testing cohort, our model and radiologist 2 showed no significant difference in performance (P=0.04). Similarly, in the external validation cohort, no significant differences were observed between our model and the three radiologists (P>0.05). However, the specificity of our model was inferior to that of the radiologists. The diagnostic ability for detecting AD of the developed algorithm and radiologists is summarized in Table 3.
Table 3
Model/radiologist | Radiology experience (years) | Accuracy (95% CI), % | Sensitivity (95% CI), % | Specificity (95% CI), % |
---|---|---|---|---|
Internal validation cohort | ||||
3D full-resolution U-Net model | – | 93.8 (89.8–98.3) | 91.6 (86.7–95.8) | 95.6 (91.2–99.3) |
Radiologist 1 | 14 | 93.2 (88.4–97.2) | 90.3 (85.4–95.8) | 94.8 (90.3–99.1) |
Radiologist 2 | 22 | 83.5 (77.1–88.8) | 94.5 (89.8–99.3) | 82.9 (78.5–89.4) |
Radiologist 3 | 20 | 92.4 (88.7–96.8) | 90.2 (84.9–96.6) | 93.3 (90.8–97.8) |
External validation cohort | ||||
3D full-resolution U-Net model | – | 92.7 (89.0–96.6) | 90.3 (86.4–95.8) | 94.3 (88.8–96.1) |
Radiologist 1 | 14 | 94.2 (89.6–97.3) | 90.2 (84.9–95.8) | 96.6 (92.4–99.0) |
Radiologist 2 | 22 | 87.1 (81.4–94.2) | 87.6 (81.9–94.2) | 88.3 (82.4–95.6) |
Radiologist 3 | 20 | 88.5 (82.1–93.8) | 94.5 (89.0–99.3) | 83.9 (78.5–92.4) |
AD, aortic dissection; CI, confidence internal; 3D, three-dimensional.
The diagnostic ability in detecting aortic dissection of the proposed algorithm and that of the other models are listed in Table 4. Overall, the 3D full-resolution U-Net model performed well in all indicators, while the UNetsub model performed poorly. The PA-Net and UNetplus models’ overall performance was relatively similar; however, the UNetplus model had a slightly better accuracy and specificity.
Table 4
Model | Diagnostic ability | ||
---|---|---|---|
Accuracy (95% CI), % | Sensitivity (95% CI), % | Specificity (95% CI), % | |
3D full-resolution U-Net model | 93.8 (89.8–98.3) | 91.6 (86.7–95.8) | 95.6 (91.2–99.3) |
PA-Net model | 88.2 (80.6–91.4) | 90.1 (86.3–94.2) | 91.8 (88.3–95.1) |
UNetsub model | 83.6 (77.8–89.8) | 88.5 (80.8–91.3) | 82.3 (72.5–89.8) |
UNetplus model | 90.4 (86.7–95.8) | 89.2 (83.5–93.6) | 92.3 (87.8–95.8) |
AD, aortic dissection; CI, confidence internal; 3D, three-dimensional; PA, pyramid attention.
Discussion
Our algorithm is designed to segment the true and false lumens from NCE-CT images in patients with aortic dissection. Besides the visibly identifiable intimal patch in the aorta, NCE-CT images of some dissection patients may also exhibit characteristics such as uneven density between the true and false lumens, aortic dilation, displacement of calcified intimal patches, and occurrences of pericardial or pleural effusion (26). Deep learning algorithms can discern additional correlations between the paired NCE-CT and CE-CT images (10,27).
During the initial phases of our algorithm’s training, we used ITK-SNAP software to manually delineate both the aortic contours and the true and false lumen contours within the CE-CT images on a layer-by-layer basis to generate the training mask. This task proved to be exceedingly time-consuming and labor-intensive. Algorithm 1 was initially trained to generate the aortic mask in CE-CT images to enhance the speed and accuracy of annotation. This approach could significantly reduce the manual labor in annotating and modifying the CE-CT images. Furthermore, ongoing updates to Algorithm 1 strengthened the accuracy of the aortic mask in CE-CT images, thereby minimizing both time and annotation errors.
The developed algorithm demonstrated a sensitivity of 91.6% and a specificity of 95.6%, which was comparable to that of the radiologists. Despite a few limitations, such as the small sample size and the occurrence of some false positives, the algorithm’s overall performance in segmenting and detecting the true and false lumens appears promising. This indicates that the algorithm has high accuracy and reliability in identifying and segmenting the true and false lumens, which can effectively reduce misdiagnosis in clinical practice. The primary aim of this study was to develop an algorithm that can determine aortic dissection on NCE-CT images, providing a diagnostic reference for radiologists and clinicians. It offers an adequate alternative solution, particularly in scenarios where contrast agents are to be avoided.
The sensitivity and accuracy of our algorithm were marginally lower compared to those of the three radiologists, although these differences were not statistically significant. Conversely, our algorithm tended to have slightly higher specificity than that of the radiologists, yet this difference also lacked statistical significance. These differences in performance are acceptable, as the algorithm’s intended use is for the screening of aortic dissection, a task in which sensitivity takes precedence. Should the algorithm suggest the possibility of AD, additional evaluations, including aortic CTA, are recommended. Moreover, we developed the algorithm to distinguish between the true and false lumens within the aorta. Theoretically, a false lumen in the aorta indicates a dissection.
Table 3 shows that radiologists 2 and 3 had poorer diagnostic ability as compared to radiologist 1. This underscores the critical role that radiologists play in medical diagnosis, while acknowledging the potential for variation in their diagnostic abilities. However, it also presents a promising solution: the integration of high-performance automated diagnostic models with experienced radiologists. This approach holds the potential to significantly enhance diagnostic accuracy and consistency, offering improvements in diagnostic imaging.
A few images of normal aortas appeared as false positives, which might be due to the limitations of the algorithm or artifacts in the CT images. However, the falsely identified false lumen volumes were relatively small. To better address this issue, we selected a 5-mL threshold for false lumen volume based on an ROC curve to delineate aortic dissection classifications. This meant that if the false lumen volume exceeded 5 mL, an aortic dissection was indicated; otherwise, the algorithm may deem a false lumen result to be normal.
Despite the presence of false positives, the algorithm had a diagnostic performance similar to that of radiologists, with an accuracy of 93.8%, a sensitivity of 91.6%, and specificity of 95.6%. Therefore, the algorithm has high accuracy in identifying and diagnosing patients with aortic dissection and has the potential capacity to reduce misdiagnosis and improve patient outcomes. The findings of false positives should be further examined and resolved to minimize misdiagnosis and reduce stress in actual clinical use. Our model is highly sensitive to the division of the dataset, including both normal aortas and aortic dissections. The final test set is also completely independent of the training data, and a 1-fold cross-validation result is acceptable. Five-fold cross-validation may not significantly improve the model’s results.
A study from Osaka University reported the use of the relatively mature XceptionNet in diagnosing aortic dissection. They classified each NCE-CT image, and when nine consecutive slices were classified as aortic dissection, the patient was diagnosed with aortic dissection (10). In 2021, teams from Zhejiang University of Traditional Chinese Medicine and Guangzhou University of Traditional Chinese Medicine published their findings on aortic dissection identification from NCE-CT using radiomics-based methods. The diagnostic accuracy, sensitivity, and specificity were high (>85%) (28,29). More recently, the Peking Union Medical College Hospital team used a Gaussian Naive Bayes model for aortic dissection recognition. This model, which integrates aortic morphological and deep features, achieved positive results (30). Our work addresses limitations in other studies by offering a comprehensive, efficient, and accurate diagnostic tool for aortic dissection based on NCE-CT. It is distinct by virtue of its focus on true and false lumen segmentation and its comparable performance to that of experienced radiologists. We compared our model to PA-Net, UNetsub, and UNetplus algorithm and found that it had superior accuracy, sensitivity, and specificity. Future studies should focus on expanding sample sizes, ensuring external validation, and considering resource-efficient models to enhance practical applicability in various clinical settings.
Overall, radiologists recognize the potential benefits of AI in improving diagnostic accuracy, efficiency, and education. However, they also emphasize the importance of addressing ethical, legal, and practical challenges to ensure that AI tools enhance rather than disrupt their practice. Overall, the consensus is that AI will augment the capabilities of radiologists, making them more effective and efficient in their work.
Our study involved several limitations that should be addressed. First, the study sample size was small. Overfitting is a critical problem in machine learning, and it is more prominent when the number of training samples is small. Therefore, further use of external queues is needed for algorithm verification. Second, due to the retrospective design of this study, selection bias was inevitable. Finally, we did not examine classification types of aortic dissection such as acute versus chronic and Stanford A versus Stanford B. Since clinical decision-making is greatly affected by the type of aortic dissection, an algorithm that can differentiate between these types would be more helpful in clinical practice.
Conclusions
In conclusion, our proposed algorithm can distinguish the true and false lumen of aortic dissection, and its diagnostic performance is comparable to that of radiologists, which may reduce the missed diagnosis of dissection in clinical practice.
Acknowledgments
Funding: This work was supported by
Footnote
Reporting Checklist: The authors have completed the TRIPOD + AI reporting checklist. Available at https://qims.amegroups.com/article/view/10.21037/qims-24-533/rc
Conflicts of Interest: All authors have completed the ICMJE uniform disclosure form (available at https://qims.amegroups.com/article/view/10.21037/qims-24-533/coif). L.S. is an employee of Shanghai DeltaHealth Hospital (China). The other authors have no conflicts of interest to declare.
Ethical Statement: The authors are accountable for all aspects of the work in ensuring that questions related to the accuracy or integrity of any part of the work are appropriately investigated and resolved. This retrospective cohort study was conducted in accordance with the Declaration of Helsinki (as revised in 2013) and was approved by the Institutional Review Boards of Fujian Provincial Hospital (IRB No. K2020-03-013), Beijing Anzhen Hospital (IRB No. 2024156x), and Xiangya Hospital (IRB No. 202008190). The requirement for individual consent was waived due to the retrospective nature of the analysis.
Open Access Statement: This is an Open Access article distributed in accordance with the Creative Commons Attribution-NonCommercial-NoDerivs 4.0 International License (CC BY-NC-ND 4.0), which permits the non-commercial replication and distribution of the article with the strict proviso that no changes or edits are made and the original work is properly cited (including links to both the formal publication through the relevant DOI and the license). See: https://creativecommons.org/licenses/by-nc-nd/4.0/.
References
- Isselbacher EM, Preventza O, Hamilton Black J 3rd, Augoustides JG, Beck AW, Bolen MA, et al. 2022 ACC/AHA Guideline for the Diagnosis and Management of Aortic Disease: A Report of the American Heart Association/American College of Cardiology Joint Committee on Clinical Practice Guidelines. Circulation 2022;146:e334-482. [Crossref] [PubMed]
- Golledge J, Eagle KA. Acute aortic dissection. Lancet 2008;372:55-66. [Crossref] [PubMed]
- Kontos MC, de Lemos JA, Deitelzweig SB, Diercks DB, Gore MO, Hess EP, McCarthy CP, McCord JK, Musey PI Jr, Villines TC, Wright LJ. 2022 ACC Expert Consensus Decision Pathway on the Evaluation and Disposition of Acute Chest Pain in the Emergency Department: A Report of the American College of Cardiology Solution Set Oversight Committee. J Am Coll Cardiol 2022;80:1925-60. [Crossref] [PubMed]
- Salmasi MY, Al-Saadi N, Hartley P, Jarral OA, Raja S, Hussein M, Redhead J, Rosendahl U, Nienaber CA, Pepper JR, Oo AY, Athanasiou T. The risk of misdiagnosis in acute thoracic aortic dissection: a review of current guidelines. Heart 2020;106:885-91. [Crossref] [PubMed]
- Takaki JKT, Ford I, Yoon HC. Variation in CTA evaluation of ED patients suspected of aortic dissection. Emerg Radiol 2022;29:709-13. [Crossref] [PubMed]
- El-Abd YJ, Hagspiel KD. Review of Imaging With Focus on New Techniques in Aortic Dissection. Tech Vasc Interv Radiol 2021;24:100748. [Crossref] [PubMed]
- Yu YT, Ren XS, An YQ, Yin WH, Zhang J, Wang X, Lu B. Changes in the renal artery and renal volume and predictors of renal atrophy in patients with complicated type B aortic dissection after thoracic endovascular aortic repair. Quant Imaging Med Surg 2022;12:5198-208. [Crossref] [PubMed]
- Morello F, Santoro M, Fargion AT, Grifoni S, Nazerian P. Diagnosis and management of acute aortic syndromes in the emergency department. Intern Emerg Med 2021;16:171-81. [Crossref] [PubMed]
- Otani T, Ichiba T, Kashiwa K, Naito H. Potential of unenhanced computed tomography as a screening tool for acute aortic syndromes. Eur Heart J Acute Cardiovasc Care 2021;10:967-75. [Crossref] [PubMed]
- Hata A, Yanagawa M, Yamagata K, Suzuki Y, Kido S, Kawata A, Doi S, Yoshida Y, Miyata T, Tsubamoto M, Kikuchi N, Tomiyama N. Deep learning algorithm for detection of aortic dissection on non-contrast-enhanced CT. Eur Radiol 2021;31:1151-9. [Crossref] [PubMed]
- Panagiotopoulos N, Drüschler F, Simon M, Vogt FM, Wolfrum S, Desch S, Richardt D, Barkhausen J, Hunold P. Significance of an additional unenhanced scan in computed tomography angiography of patients with suspected acute aortic syndrome. World J Radiol 2018;10:150-61. [Crossref] [PubMed]
- Sun Z, Choo GH, Ng KH. Coronary CT angiography: current status and continuing challenges. Br J Radiol 2012;85:495-510. [Crossref] [PubMed]
- Su TH, Hsieh CH, Chan YL, Wong YC, Kuo CF, Li CH, Lee CC, Chen HY, Intravenous CT. Contrast Media and Acute Kidney Injury: A Multicenter Emergency Department-based Study. Radiology 2021;301:571-81. [Crossref] [PubMed]
- Rachoin JS, Wolfe Y, Patel S, Cerceo E. Contrast associated nephropathy after intravenous administration: what is the magnitude of the problem? Ren Fail 2021;43:1311-21. [Crossref] [PubMed]
- Kene M, Arasu VA, Mahapatra AK, Huang J, Reed ME. Acute Kidney Injury After CT in Emergency Patients with Chronic Kidney Disease: A Propensity Score-matched Analysis. West J Emerg Med 2021;22:614-22. [Crossref] [PubMed]
- Erickson BJ, Korfiatis P, Akkus Z, Kline TL. Machine Learning for Medical Imaging. Radiographics 2017;37:505-15. [Crossref] [PubMed]
- Niederer SA, Lumens J, Trayanova NA. Computational models in cardiology. Nat Rev Cardiol 2019;16:100-11. [Crossref] [PubMed]
- Huo D, Kou B, Zhou Z, Lv M. A machine learning model to classify aortic dissection patients in the early diagnosis phase. Sci Rep 2019;9:2701. [Crossref] [PubMed]
- Dey D, Slomka PJ, Leeson P, Comaniciu D, Shrestha S, Sengupta PP, Marwick TH. Artificial Intelligence in Cardiovascular Imaging: JACC State-of-the-Art Review. J Am Coll Cardiol 2019;73:1317-35. [Crossref] [PubMed]
- Osztrogonacz P, Berczeli M, Chinnadurai P, Chang SM, Shah DJ, Lumsden AB. Dynamic Imaging of Aortic Pathologies: Review of Clinical Applications and Imaging Protocols. Methodist Debakey Cardiovasc J 2023;19:4-14. [Crossref] [PubMed]
- Hahn LD, Baeumler K, Hsiao A. Artificial intelligence and machine learning in aortic disease. Curr Opin Cardiol 2021;36:695-703. [Crossref] [PubMed]
- Bashir M, Harky A. Artificial Intelligence in Aortic Surgery: The Rise of the Machine. Semin Thorac Cardiovasc Surg 2019;31:635-7. [Crossref] [PubMed]
- Liu F, Wang K, Liu D, Yang X, Tian J. Deep pyramid local attention neural network for cardiac structure segmentation in two-dimensional echocardiography. Med Image Anal 2021;67:101873. [Crossref] [PubMed]
- Punn NS, Agarwal S. Modality specific U-Net variants for biomedical image segmentation: a survey. Artif Intell Rev 2022;55:5845-89. [Crossref] [PubMed]
- Zhou Z, Siddiquee MMR, Tajbakhsh N, Liang J. UNet++: A Nested U-Net Architecture for Medical Image Segmentation. Deep Learn Med Image Anal Multimodal Learn Clin Decis Support (2018) 2018;11045:3-11. [Crossref] [PubMed]
- Ding Y, Zhang C, Wu W, Pu J, Zhao X, Zhang H, Zhao L, Schoenhagen P, Liu S, Ma X. A radiomics model based on aortic computed tomography angiography: the impact on predicting the prognosis of patients with aortic intramural hematoma (IMH). Quant Imaging Med Surg 2023;13:598-609. [Crossref] [PubMed]
- Polidori T, De Santis D, Rucci C, Tremamunno G, Piccinni G, Pugliese L, Zerunian M, Guido G, Pucciarelli F, Bracci B, Polici M, Laghi A, Caruso D. Radiomics applications in cardiac imaging: a comprehensive review. Radiol Med 2023;128:922-33. [Crossref] [PubMed]
- Guo Y, Chen X, Lin X, Chen L, Shu J, Pang P, Cheng J, Xu M, Sun Z. Non-contrast CT-based radiomic signature for screening thoracic aortic dissections: a multicenter study. Eur Radiol 2021;31:7067-76. [Crossref] [PubMed]
- Zhou Z, Yang J, Wang S, Li W, Xie L, Li Y, Zhang C. The diagnostic value of a non-contrast computed tomography scan-based radiomics model for acute aortic dissection. Medicine (Baltimore) 2021;100:e26212. [Crossref] [PubMed]
- Yi Y, Mao L, Wang C, Guo Y, Luo X, Jia D, Lei Y, Pan J, Li J, Li S, Li XL, Jin Z, Wang Y. Advanced Warning of Aortic Dissection on Non-Contrast CT: The Combination of Deep Learning and Morphological Characteristics. Front Cardiovasc Med 2021;8:762958. [Crossref] [PubMed]