Comparison of computer-aided quantitative measurement and physician visual assessment in the evaluation of intracranial atherosclerotic stenosis: a vessel wall magnetic resonance imaging study
Introduction
Intracranial atherosclerosis stenosis (ICAS) is a primary cause of ischemic stroke in China (1). Ischemic stroke in China is characterized by a high incidence (1,700/100,000), high mortality rate (11.9%), and high recurrence rate (10–17%) (2). In the Asian population, particularly among Chinese individuals, the middle cerebral artery (MCA) and basilar artery (BA) demonstrate a higher propensity for atherosclerosis involvement compared to the intracranial carotid artery (3). Accurately assessing ICAS through imaging techniques is paramount in guiding therapeutic interventions and prognostic stratification. The application of vessel wall magnetic resonance imaging (VWMRI) has transitioned the imaging assessment of intracranial atherosclerotic stenosis from evaluating lumen stenosis to assessing vessel wall structure and plaque stability (4). Digital subtraction angiography (DSA) provides detailed information about the anatomy, severity of the stenosis, and collateral circulation of the intracranial arteries (5). Its superior spatial resolution establishes DSA as the reference standard for luminal stenosis evaluation. However, DSA is an imaging technology based on vascular lumen, which is unsuitable for evaluating vessel wall characteristics (5). The VWMRI is currently recognized as a reliable method for evaluating intracranial vessel walls in vivo (6,7). By providing insights into the underlying pathophysiological mechanisms of ischemic stroke, VWMRI offers a comprehensive approach to ICAS evaluation, encompassing stenosis severity, plaque stability, and vessel wall structure characteristics (8). Therefore, the accuracy of VWMRI assessment of intracranial atherosclerotic stenosis is crucial for the diagnosis and treatment of ischemic stroke.
With the advancement of scientific technology, the application of computer-aided quantitative measurement (CAQM) and artificial intelligence in imaging assessment is increasing (9-11). However, radiologists rely primarily on visual assessment as the primary evaluation method in current imaging diagnostics. Although radiologists can quickly obtain imaging information and make comprehensive diagnoses through visual assessment combined with a medical history and clinical presentation, visual assessment heavily depends on individual experience, leading to subjectivity and uncertainty. In contrast, CAQM can provide objective and precise data by quantitatively analyzing imaging data, reducing subjective errors, and is particularly important for the acceptable assessment of diseases (12,13). A previous study had shown inconsistencies between visual assessment by radiologists and quantitative measurement in coronary angiography, resulting in excessive utilization of medical resources (14). Therefore, we hypothesize that there may be differences between physician visual assessment (PVA) and CAQM in the evaluation of intracranial atherosclerotic stenosis using VWMRI. This study compares PVA and CAQM for the diagnosis of ICAS. The objective is to determine the most suitable imaging assessment method, improve the accuracy of VWMRI diagnosis, and offer a dependable imaging reference for clinical diagnosis and treatment of ischemic stroke. We present this article in accordance with the STROBE reporting checklist (available at https://qims.amegroups.com/article/view/10.21037/qims-24-788/rc).
Methods
Patient recruitment
This retrospective study consecutively enrolled patients diagnosed with ICAS, as confirmed by imaging examinations at the Fourth Affiliated Hospital of China Medical University from December 2018 to December 2023. The clinical data of patients (including age, gender, history of hypertension, diabetes, dyslipidemia, smoking, alcohol consumption, and DSA measurement results) were collected. The study was conducted in accordance with the Declaration of Helsinki (as revised in 2013). The study was approved by the Ethics Committee of the Fourth Affiliated Hospital of China Medical University (No. EC-2024-KS-020), and individual consent for this retrospective analysis was waived.
Inclusion criteria: (I) confirmed ICAS (MCA and/or BA) by imaging examinations; (II) patients who had previously undergone VWMRI; (III) patients with complete clinically relevant medical history.
Exclusion criteria: (I) vascular stenosis caused by non-atherosclerotic lesions confirmed by imaging examinations (e.g., Moyamoya disease, arterial dissection, vertebrobasilar dolichoectasia, vasculitis, etc.); (II) poor image quality of VWMRI unsuitable for evaluation; (III) patients who underwent intracranial arterial angioplasty and/or stent implantation; (IV) complete occlusion of intracranial arteries.
VWMRI scanning protocol and image quality assessment
VWMRI was performed using a 3.0T MRI (GE Medical Systems Discovery MR750, GE Healthcare, USA) with an 8-channel head coil, and the scan parameters are shown in Table 1. The scanning scope included the diseased vessels of the MCA BA. The image quality was graded by a radiologist (Y.W., with eight years of experience in VWMRI diagnosis). The grading was based on the visualization of the vessel wall, lumen, and plaques (15), divided into four levels (levels 1–2: poor image quality and undiagnosable; levels 3–4: good image quality for diagnostic interpretation). Images of level 3 or 4 were included in the study.
Table 1
Sequences | TR (ms) | TE (ms) | Flip angle | Slice thickness (mm) | FOV (mm) | NEX | Locs per slab | Matrix | Acquisition time |
---|---|---|---|---|---|---|---|---|---|
3D TOF-MRA | 23 | 2.5 | 20 | 1.4 | 220 | 3 | 32 | 320×256 | 4 min 1 s |
3D CUBE T1WI | 1,140 | 14 | – | 1 | 180 | 1 | 80 | 320×228 | 4 min 10 s |
3D CUBE T2WI | 1,800 | 60 | – | 1 | 180 | 1 | 80 | 320×228 | 4 min 4 s |
2D FSE T2WI | 4,000 | 42 | 125 | 2 | 130 | 4 | 16 | 256×224 | 3 min 45 s |
2D FSE PDWI | 2,500 | 60 | 125 | 2 | 130 | 4 | 16 | 256×224 | 3 min 45 s |
TR, repetition time; TE, echo time; FOV, field of view; NEX, number of excitations; Locs per slab, total number of locations (slices) generated from a slab; TOF-MRA, time of flight magnetic resonance angiography; CUBE, variable-flip-angle turbo-spin-echo; T1WI, T1-weighted images; T2WI, T2-weighted images; FSE, fast spin echo; PDWI, proton-weighted images.
Definitions and measurement standards for imaging characteristics of VWMRI
Stenosis severity
PVA categorizes stenosis severity into mild, moderate, and severe based on luminal diameter (stenosis rate <50%, 50–69%, and >70%, respectively) (16). CAQM employs the normalized wall index (NWI) to evaluate stenosis severity, calculated as NWI = (vessel wall area − lumen area)/vessel wall area × 100%, referencing stenosis rate grading standards (17).
Vessel wall remodeling
PVA assessment identifies negative remodeling as vessel shrinkage and compensatory vessel enlargement as positive remodeling; otherwise, it is considered no remodeling. CAQM utilizes the remodeling ratio (RR) to determine vessel wall remodeling type, which is calculated as RR =vessel area at stenosis/adjacent normal vessel area. RR between 0.95 and 1.05 indicates no remodeling, RR <0.95 indicates negative remodeling, and RR >1.05 indicates positive remodeling (17).
Vessel wall thickening pattern
PVA defines eccentric wall thickening when localized thickening is observed at the narrowest point of the vessel cross-section. Otherwise, it is considered concentric thickening. CAQM employs the eccentricity index (EI) to evaluate the wall thickening pattern, calculated as EI = (maximum wall thickness − minimum wall thickness)/maximum wall thickness; eccentric wall thickening is determined if the EI ≥0.5, otherwise considered concentric wall thickening (17).
Fibrous cap structure and lipid core proportion of plaques
PVA subjectively evaluates fibrous cap thickness consistency and lipid core size. By delineating the regions of interest (ROIs) of plaque and its different components (such as lipid core, calcification, and hemorrhage), CAQM can automatically calculate the areas of different ROIs and determine the maximum and minimum thicknesses of the fibrous cap. CAQM defines plaques with a lipid core ratio (lipid core area/plaque area ×100%) greater than 40% as having a large lipid core (18,19). CAQM calculates the ratio of the minimum fibrous cap thickness to the maximum fibrous cap thickness. The fibrous cap thickness is uniform if this ratio is more significant than 0.5 (19-21).
Plaque enhancement degree
PVA categorizes enhancement based on pre- and post-contrast signal intensity differences: no difference = degree 0, lower signal than pituitary stalk = degree 1, higher signal=degree 2 (22). By outlining the ROIs of plaques and brain parenchyma at the same level (with the same area as plaques) pre- and post-contrast enhancement, CAQM can automatically measure the signal intensity of these ROIs. Enhancement ratio = [signal intensity of plaque (post-contrast)/signal intensity of gray matter (post-contrast)]/[signal intensity of plaque (pre-contrast)/signal intensity of gray matter (pre-contrast)], with ratios ≤1.1, (1.1, 1.5), and ≥1.5, indicating enhancement degrees 0, 1, and 2, respectively (23).
The process of VWMRI assessment
PVA
Original images were directly imported into the Picture Archiving and Communication System, enabling physicians to conduct image assessments and diagnoses based on their expertise.
CAQM
The images were analyzed and measured using the Vessel Mass (Leiden University Medical Center, Leiden, the Netherlands) software (Figure 1). The software imported original data, automatically/semi-automatically identified and delineated vessels and lumens, and reconstructed cross-sectional vessel images to improve accuracy. ROI, such as fibrous cap and lipid core, were labeled automatically/semi-automatically, and relevant values were generated for physicians to reference in image assessments and diagnoses.
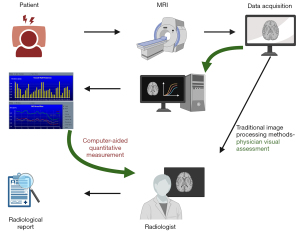
Two radiologists (Y.D. and F.L., each with three and four years of experience in VWMRI diagnosis), respectively, independently and simultaneously conducted CAQM and PVA on all images following the evaluation mentioned above criteria and operational procedures. Four weeks later, one of the radiologists (Y.D.) repeated the evaluation process according to relevant standards. Clinical information was concealed during the assessment process, and the time taken for diagnosis using each assessment method was recorded separately (Figure 1, the time records of the two methods were from the beginning of data acquisition to the radiological report generation.). Stenosis degree, vessel wall remodeling, and wall thickening pattern were assessed on 3D CUBE T1WI images, while fibrous cap structure and lipid core ratio were evaluated on 2D FSE T2WI or 3D CUBE T2WI images. Plaque enhancement pattern was assessed on 3D CUBE T1WI and CE-3D CUBE T1WI images. The evaluation results from one radiologist (Y.D.) were utilized for further statistical analysis.
Statistics
All data underwent statistical analysis using MedCalc software (MedCalc v22.0.19; MedCalc Software Ltd, Ostend, Belgium). Continuous variables were presented as mean ± standard deviation, while categorical variables were expressed as numerical values (percentages). The Kolmogorov-Smirnov test was used to determine whether the continuous variables were normally distributed. Consistency between assessment methods, both interobserver and intraobserver, was assessed using Cohen’s kappa coefficient (K) and intraclass correlation coefficient (ICC). K values <0.4 indicated poor agreement, 0.40–0.59 moderate agreement, 0.60–0.80 good agreement, and >0.80 excellent agreement (24). ICC >0.75 indicated good consistency, 0.40≤ ICC ≤0.75 indicated fair consistency, and ICC <0.4 represented poor consistency (25). The disparities and concordance between the two assessment methods for VWMRI of ICAS were scrutinized using paired sample t-tests, Wilcoxon signed-rank tests, and Cohen’s kappa coefficient analysis. DSA served as the reference standard, and area under the curve (AUC) was utilized to evaluate the diagnostic performance of the assessment methods for luminal stenosis severity. A two-sided level of P<0.05 was considered indicative of statistical significance.
Results
Patient clinical information
Between December 2018 and December 2023, 762 patients were collected according to the inclusion criteria. Of these, 173 patients were excluded. Ultimately, 589 patients were included in the study (Figure 2). Detailed clinical information of the patients can be found in Table 2.
Table 2
Characteristics | MCA (n=343) | BA (n=246) |
---|---|---|
Sex, n (%) | ||
Male | 225 (65.60) | 152 (61.79) |
Female | 118 (34.40) | 94 (38.21) |
Age (years), mean ± SD | 59.57±10.59 | 62.37±11.23 |
Hypertension, n (%) | 236 (68.80) | 170 (69.11) |
Diabetes, n (%) | 144 (41.98) | 106 (43.09) |
Dyslipidemia, n (%) | 195 (56.85) | 134 (54.47) |
Smoking (ever), n (%) | 159 (46.36) | 115 (46.75) |
Alcoholism (ever), n (%) | 89 (25.95) | 64 (26.02) |
MCA, middle cerebral artery; BA, basilar artery.
Comparison of PVA and CAQM
The two assessment methods show good intra- and inter-observer consistency in evaluating the imaging features of VWMRI, respectively (Table 3). The assessment time for PVA was significantly shorter than that for CAQM (12.02±3.63 vs. 20.48±6.50 min).
Table 3
Characteristic (n=589) | CAQM (ICC, 95% CI) | PVA (K, 95% CI) | |||
---|---|---|---|---|---|
Intraobserver | Interobserver | Intraobserver | Interobserver | ||
Stenosis severity | 0.92 (0.91–0.93) | 0.90 (0.88–0.98) | 0.91 (0.84–0.96) | 0.71 (0.63–0.79) | |
Vessel wall remodeling | 0.87 (0.84–0.90) | 0.83 (0.77–0.89) | 0.84 (0.80–0.89) | 0.79 (0.77–0.85) | |
Wall thickening pattern | 0.95 (0.93–0.98) | 0.88 (0.84–0.93) | 0.85 (0.81–0.90) | 0.81 (0.72–0.91) | |
Fibrous cap structure | 0.93 (0.92–0.94) | 0.87 (0.82–0.92) | 0.79 (0.75–0.85) | 0.74 (0.65–0.84) | |
Lipid core ratio | 0.91 (0.88–0.93) | 0.90 (0.85–0.94) | 0.87 (0.82–0.93) | 0.90 (0.84–0.96) | |
Plaque enhancement degree* | 0.94 (0.93–0.95) | 0.81 (0.77–0.85) | 0.88 (0.84–0.90) | 0.81 (0.72–0.89) |
*, n=113. ICC, intraclass correlation coefficient; K, kappa value; CAQM, computer-aided quantitative measurement; CI, confidence interval; PVA, physician visual assessment.
Statistical differences (P<0.05) were observed in the assessment results of luminal stenosis severity between the two assessment methods (Figure 3). Compared to CAQM, PVA underestimated the degree of luminal stenosis (Table 4). When compared to the DSA (severe stenosis 262 cases, non-severe stenosis 14 cases), CAQM and PVA demonstrated sensitivities and specificities for the diagnosis of severe luminal stenosis of 98.1%/88.9% and 78.6%/71.4%, respectively, with corresponding AUCs (Figure 4) of 0.883 (P<0.05, 95% CI: 0.76–1.01) and 0.802 (P<0.05, 95% CI: 0.61–0.94). In evaluating vessel wall imaging characteristics in VWMRI, there were no significant statistical differences between the two methods in assessing vessel wall thickening patterns (P=0.12/0.39) and lipid core proportion of plaques (P=0.65/0.27) (Table 4). However, significant statistical differences (P<0.05) were observed in evaluating vessel wall remodeling (Figure 5) and fibrous cap structure (Figure 6). While there were no statistical differences in the assessment results of plaque enhancement degree in the MCA and BA by the two evaluation methods (n=77/36, P=0.08/0.21), there were statistical differences in the overall enhancement degree of intracranial arterial plaques (n=113, P=0.03).
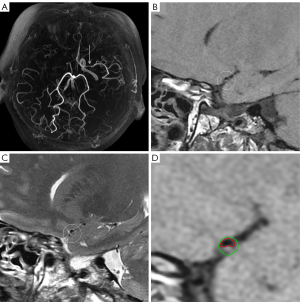
Table 4
Characteristic | Stenosis of MCA (n=343) | Stenosis of BA (n=246) | |||||
---|---|---|---|---|---|---|---|
CAQM | PVA | P | CAQM | PVA | P | ||
Stenosis severity, n (%) | 0.001 | 0.001 | |||||
Mild stenosis | 4 (1.17) | 21 (6.12) | 2 (0.81) | 11 (4.47) | |||
Moderate stenosis | 53 (15.45) | 71 (20.70) | 10 (4.07) | 32 (13.01) | |||
Severe stenosis | 286 (83.38) | 251 (73.18) | 234 (95.12) | 203 (82.52) | |||
Vessel wall remodeling, n (%) | 0.001 | 0.001 | |||||
Negative remodeling | 189 (55.10) | 116 (33.82) | 117 (47.56) | 55 (22.36) | |||
No remodeling | 80 (23.32) | 215 (62.68) | 71 (28.86) | 147 (59.76) | |||
Positive remodeling | 74 (21.57) | 12 (3.50) | 58 (23.58) | 44 (17.89) | |||
Wall thickening pattern, n (%) | 0.12 | 0.39 | |||||
Eccentric thickening | 302 (88.05) | 291 (84.84) | 163 (66.26) | 154 (62.60) | |||
Centripetal thickening | 41 (11.95) | 52 (15.16) | 83 (33.74) | 92 (37.40) | |||
Fibrous cap characteristics*, n (%) | 0.001 | 0.002 | |||||
Irregular | 93 (27.11) | 54 (15.74) | 78 (31.71) | 73 (29.67) | |||
Uniform | 80 (23.32) | 119 (34.69) | 65 (26.42) | 70 (28.46) | |||
Lipid core ratio*, n (%) | 0.65 | 0.27 | |||||
≤40% | 170 (49.56) | 171 (49.85) | 139 (56.50) | 136 (55.28) | |||
>40% | 3 (0.87) | 2 (0.58) | 4 (1.63) | 7 (2.85) | |||
Plaque enhancement degree#, n (%) | 0.08 | 0.21 | |||||
Level 0 | 3 (3.90) | 10 (12.99) | 3 (8.33) | 8 (22.22) | |||
Level 1 | 28 (36.36) | 26 (33.77) | 13 (36.11) | 9 (25.00) | |||
Level 2 | 46 (59.74) | 41 (53.25) | 20 (55.56) | 19 (52.78) |
*, the cases with unclear visualization of the fibrous cap or lipid core were excluded. #, MCA (n=77), BA (n=36). PVA, physician visual assessment; CAQM, computer-aided quantitative measurement; MCA, middle cerebral artery; BA, basilar artery.
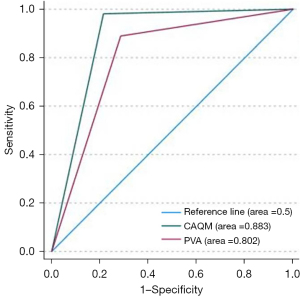
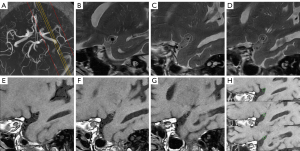
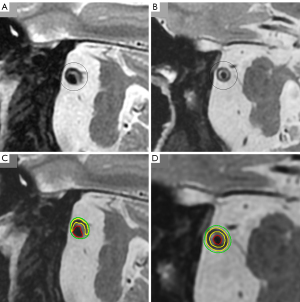
Using Cohen’s kappa coefficient, the consistency of the two methods in assessing atherosclerotic stenosis of the MCA and BA was compared. Notably, high levels of agreement were observed in the evaluation of vessel wall thickening patterns [K=0.67 (0.57–0.81) and 0.85 (0.76–0.92)] and the proportion of plaque lipid cores [K=0.97 (0.95–0.99) and 0.94 (0.91–0.98)]. However, poorer consistency was observed in the assessment of luminal stenosis severity [K=0.37 (0.27–0.47) and 0.15 (0.02–0.27)], vessel wall remodeling [K=0.06 (−0.011 to 0.12) and 0.06 (−0.02 to 0.14)], fibrous cap structure [K=0.59 (0.53–0.66) and 0.58 (0.50–0.66)], and plaque enhancement degree [K=0.13 (−0.05 to 0.32) and 0.16 (−0.06 to 0.39)].
Discussion
This study compared the differences and consistencies between PVA and CAQM in diagnosing ICAS in 589 cases using VWMRI. While numerous studies have employed VWMRI to evaluate the imaging characteristics of ICAS, to our knowledge, this is the first study to compare the disparities in VWMRI features of ICAS obtained from different assessment methods. Our findings indicate that although PVA is faster than CAQM, there are significant differences between the two methods regarding stenosis degree, vessel wall remodeling, plaque fibrous cap structure, and plaque enhancement degree. This research provides valuable insights for selecting the most suitable assessment methods to achieve more accurate results in VWMRI.
The severity of stenosis is a crucial assessment parameter in evaluating ICAS by VWMRI, as differences in stenosis degree may imply varied treatment options. A previous study suggested that ICAS with higher degrees of stenosis may benefit from intravascular stenting procedures (26). In our study, we observed that compared to CAQM, PVA underestimated the severity of ICAS stenosis when referenced against DSA. This disparity may stem from the PVA relying on luminal diameter ratios, while CAQM is based on the NWI to evaluate stenosis degree. Multiple studies have reported that NWI offers a more accurate reflection of the stenosis degree, as it is an independent imaging feature associated with recurrent stroke (27,28). NWI takes into account the impact of vessel wall remodeling on stenosis degree. Positive remodeling leading to compensatory vessel dilatation may result in underestimation of actual luminal stenosis severity when relying solely on luminal diameter assessment.
Vessel wall remodeling is a common phenomenon in atherosclerotic lesions. Prior studies have shown that positive remodeling in intracranial atherosclerotic lesions is associated with an increased incidence of ischemic stroke (29,30). Our study observed that PVA predominantly identified no-remodeling in ICAS, with fewer positive and negative remodeling instances than CAQM. The anatomical characteristics of intracranial arteries primarily account for the differences between the two assessment methods. Due to the tortuous course of intracranial arteries, VWMRI often struggles to obtain perfect cross-sectional images, leading to deviation in the visual assessment of vessel cross-sections. In contrast, CAQM utilizes three-dimensional curved planar reconstruction techniques to generate standardized cross-sectional images of tortuous vessels, compensating for this limitation.
Plaque enhancement is another key indicator used by VWMRI to assess ICAS. Given the extensive research correlating plaque enhancement with the occurrence, recurrence, and prognosis of ischemic stroke (31-33), it is crucial to assess its degree accurately. Although the differences between PVA and CAQM in this study’s assessment of plaque enhancement were not particularly significant, the concordance of results between these two assessment methods was relatively poor. CAQM involves calculating the plaque’s signal enhancement ratio before and after contrast injection to define and quantitatively analyze plaque enhancement. Given the clinical context, we hypothesize that the results of CAQM may better reflect the actual degree of plaque enhancement in the real world. Given our study’s relatively small sample size, further research with an expanded sample size is warranted to provide more precise evidence to support this hypothesis.
In our study, the focus on plaque fibrous cap structure is primarily on its uniformity, as inhomogeneity of the fibrous cap indicates a potentially increased risk of plaque rupture (34). Accurate delineation of the fibrous cap on VWMRI is often challenging. The minute size of the fibrous cap poses a significant challenge for radiologists to visually assess it, especially in the marginal regions of the cap. CAQM reduces subjective errors by quantifying fibrous cap parameters (mean, standard deviation, maximum and minimum values).
The analysis of ICAS by VWMRI encompasses the assessment parameters mentioned in this study and includes plaque distribution and plaque signal characteristics [such as intra-plaque hemorrhage (IPH)]. The distribution of plaques and whether they involve perforating arteries are relevant factors, with plaques occurring on the superior wall of the MCA being more likely to involve lenticulostriate arteries (35). However, current methods for assessing plaque distribution rely on visual assessment based on physician expertise, as CAQM struggles to quantify plaque distribution effectively. Some studies have indicated that IPH is one of the indicators of plaque instability and is independently associated with symptomatic stroke (23,36). IPH is generally defined as a signal intensity within the plaque exceeding 1.5 times that of adjacent brain tissue (37). However, due to our study’s limited number of IPH cases, further statistical analysis was not conducted to analyze the differences between PVA and CAQM in assessing intraplaque hemorrhage.
The use of CAQM in evaluating ICAS requires more time than PVA. The additional time is mainly spent correcting the automatic recognition of the quantitative measurement software and manually outlining the ROIs. It is important to note that manual outlining of ROIs can introduce errors. However, the use of advanced artificial intelligence technologies has the potential to improve the accuracy of the software’s automatic recognition, reduce human errors, and significantly shorten the evaluation time for quantitative measurements. However, the complex anatomical structure of intracranial arteries and the intricate characteristics of lesion signals pose significant challenges to the application of AI in intracranial arterial magnetic resonance vessel wall imaging. At the same time, PVA relies on their diagnostic experience and clinical judgment, which can be improved by accumulating clinical cases and feedback. CAQM, with its objective quantitative indicators, serves as a crucial tool to compensate for any lack of diagnostic experience among physicians.
There are some limitations in this study. It was conducted as a single-center retrospective study, which limited the number of patients included. Furthermore, the visual assessment by physicians was based on the software and hardware available at the tertiary comprehensive medical center where the researchers were located, as well as the diagnostic experience of the physicians, without stratification based on their experience. Future studies could benefit from a multi-center approach, a larger sample size, and a stratified evaluation by multiple physicians to investigate the subject further. Additionally, this study only examined the differences between PVA and CAQM in ICAS without comparing them in non-atherosclerotic conditions such as moyamoya disease, arterial dissection, and vasculitis. Furthermore, although the zero interpolation padding technique used in GE MRI can enhance the resolution of 3D sequence images to 0.4 mm × 0.4 mm × 0.4 mm (isotropic voxels), 2D sequence images cannot achieve such high resolution. Consequently, this limitation in spatial resolution may affect the evaluation of vessel walls in VWMRI imaging.
Conclusions
This study has revealed disparities in the assessment results between two different assessment methods in VWMRI of ICAS. We recommend the utilization of CAQM as the preferred assessment method for evaluating stenosis degree, vessel wall remodeling, and fibrous cap characteristics. However, we suggest adopting PVA to assess wall thickening patterns and lipid core ratio to expedite the radiological diagnosis process. CAQM may be a superior assessment method for evaluating plaque enhancement degrees. However, further research with an expanded sample size is still necessary to validate this hypothesis.
Acknowledgments
Funding: The project was funded by
Footnote
Reporting Checklist: The authors have completed the STROBE reporting checklist. Available at https://qims.amegroups.com/article/view/10.21037/qims-24-788/rc
Conflicts of Interest: All authors have completed the ICMJE uniform disclosure form (available at https://qims.amegroups.com/article/view/10.21037/qims-24-788/coif). Yejun Wu reports that he received funding from the Natural Science Foundation of Liaoning Province (No. 2019-ZD-0762). The other authors have no conflicts of interest to declare.
Ethical Statement: The authors are accountable for all aspects of the work in ensuring that questions related to the accuracy or integrity of any part of the work are appropriately investigated and resolved. The study was conducted in accordance with the Declaration of Helsinki (as revised in 2013). The study was approved by the Ethics Committee of the Fourth Affiliated Hospital of China Medical University (No. EC-2024-KS-020), and individual consent for this retrospective analysis was waived.
Open Access Statement: This is an Open Access article distributed in accordance with the Creative Commons Attribution-NonCommercial-NoDerivs 4.0 International License (CC BY-NC-ND 4.0), which permits the non-commercial replication and distribution of the article with the strict proviso that no changes or edits are made and the original work is properly cited (including links to both the formal publication through the relevant DOI and the license). See: https://creativecommons.org/licenses/by-nc-nd/4.0/.
References
- Saini V, Guada L, Yavagal DR. Global Epidemiology of Stroke and Access to Acute Ischemic Stroke Interventions. Neurology 2021;97:S6-S16. [Crossref] [PubMed]
- Pan Y, Li Z, Li J, Jin A, Lin J, Jing J, Li H, Meng X, Wang Y, Wang Y. Residual Risk and Its Risk Factors for Ischemic Stroke with Adherence to Guideline-Based Secondary Stroke Prevention. J Stroke 2021;23:51-60. [Crossref] [PubMed]
- Banerjee C, Chimowitz MI. Stroke Caused by Atherosclerosis of the Major Intracranial Arteries. Circ Res 2017;120:502-13. [Crossref] [PubMed]
- Qiao Y, Steinman DA, Qin Q, Etesami M, Schär M, Astor BC, Wasserman BA. Intracranial arterial wall imaging using three-dimensional high isotropic resolution black blood MRI at 3.0 Tesla. J Magn Reson Imaging 2011;34:22-30. [Crossref] [PubMed]
- Sanchez S, Mossa-Basha M, Anagnostakou V, Liebeskind DS, Samaniego EA. Comprehensive imaging analysis of intracranial atherosclerosis. J Neurointerv Surg 2024;jnis-2023-020622. Epub ahead of print. [Crossref]
- Mandell DM, Mossa-Basha M, Qiao Y, Hess CP, Hui F, Matouk C, Johnson MH, Daemen MJ, Vossough A, Edjlali M, Saloner D, Ansari SA, Wasserman BA, Mikulis DJVessel Wall Imaging Study Group of the American Society of Neuroradiology. Intracranial Vessel Wall MRI: Principles and Expert Consensus Recommendations of the American Society of Neuroradiology. AJNR Am J Neuroradiol 2017;38:218-29. [Crossref] [PubMed]
- Vranic JE, Hartman JB, Mossa-Basha M. High-Resolution Magnetic Resonance Vessel Wall Imaging for the Evaluation of Intracranial Vascular Pathology. Neuroimaging Clin N Am 2021;31:223-33. [Crossref] [PubMed]
- Mossa-Basha M, Alexander M, Gaddikeri S, Yuan C, Gandhi D. Vessel wall imaging for intracranial vascular disease evaluation. J Neurointerv Surg 2016;8:1154-9. [Crossref] [PubMed]
- Wolff L, Uniken Venema SM, Luijten SPR, Hofmeijer J, Martens JM, Bernsen MLE, van Es ACGM, van Doormaal PJ, Dippel DWJ, van Zwam W, van Walsum T, van der Lugt A. Diagnostic performance of an algorithm for automated collateral scoring on computed tomography angiography. Eur Radiol 2022;32:5711-8. [Crossref] [PubMed]
- Lang S, Hoelter P, Schmidt MA, Mrochen A, Kuramatsu J, Kaethner C, Roser P, Kowarschik M, Doerfler A. Accuracy of Dose-Saving Artificial-Intelligence-Based 3D Angiography (3DA) for Grading of Intracranial Artery Stenoses: Preliminary Findings. Diagnostics (Basel) 2023;13:712. [Crossref] [PubMed]
- Qiu J, Tan G, Lin Y, Guan J, Dai Z, Wang F, Zhuang C, Wilman AH, Huang H, Cao Z, Tang Y, Jia Y, Li Y, Zhou T, Wu R. Automated detection of intracranial artery stenosis and occlusion in magnetic resonance angiography: A preliminary study based on deep learning. Magn Reson Imaging 2022;94:105-11. [Crossref] [PubMed]
- Groen AM, Kraan R, Amirkhan SF, Daams JG, Maas M. A systematic review on the use of explainability in deep learning systems for computer aided diagnosis in radiology: Limited use of explainable AI? Eur J Radiol 2022;157:110592. [Crossref] [PubMed]
- Hosny A, Parmar C, Quackenbush J, Schwartz LH, Aerts HJWL. Artificial intelligence in radiology. Nat Rev Cancer 2018;18:500-10. [Crossref] [PubMed]
- Zhang H, Mu L, Hu S, Nallamothu BK, Lansky AJ, Xu B, et al. Comparison of Physician Visual Assessment With Quantitative Coronary Angiography in Assessment of Stenosis Severity in China. JAMA Intern Med 2018;178:239-47. [Crossref] [PubMed]
- Li F, Wang Y, Du Y, Hu T, Wu Y. Correlation of the middle cerebral artery atherosclerotic plaque characteristics with ischemic stroke recurrence: a vessel wall magnetic resonance imaging study. Aging (Albany NY) 2023;15:7844-52. [Crossref] [PubMed]
- Samuels OB, Joseph GJ, Lynn MJ, Smith HA, Chimowitz MI. A standardized method for measuring intracranial arterial stenosis. AJNR Am J Neuroradiol 2000;21:643-6.
- Li F, Wang Y, Hu T, Wu Y. Application and interpretation of vessel wall magnetic resonance imaging for intracranial atherosclerosis: a narrative review. Ann Transl Med 2022;10:714. [Crossref] [PubMed]
- Chen XY, Wong KS, Lam WW, Zhao HL, Ng HK. Middle cerebral artery atherosclerosis: histological comparison between plaques associated with and not associated with infarct in a postmortem study. Cerebrovasc Dis 2008;25:74-80. [Crossref] [PubMed]
- Jiang Y, Peng W, Tian B, Zhu C, Chen L, Wang X, Liu Q, Wang Y, Xiang Z, Degnan AJ, Teng Z, Saloner D, Lu J. Identification and Quantitative Assessment of Different Components of Intracranial Atherosclerotic Plaque by Ex Vivo 3T High-Resolution Multicontrast MRI. AJNR Am J Neuroradiol 2017;38:1716-22. [Crossref] [PubMed]
- Redgrave JN, Gallagher P, Lovett JK, Rothwell PM. Critical cap thickness and rupture in symptomatic carotid plaques: the oxford plaque study. Stroke 2008;39:1722-9. [Crossref] [PubMed]
- Virmani R, Kolodgie FD, Burke AP, Farb A, Schwartz SM. Lessons from sudden coronary death: a comprehensive morphological classification scheme for atherosclerotic lesions. Arterioscler Thromb Vasc Biol 2000;20:1262-75. [Crossref] [PubMed]
- Lu Y, Ye MF, Zhao JJ, Diao SS, Li T, Ding DX, Zhang LL, Yao FR, Kong Y, Xu Z. Gadolinium enhancement of atherosclerotic plaque in the intracranial artery. Neurol Res 2021;43:1040-9. [Crossref] [PubMed]
- Shi Z, Li J, Zhao M, Peng W, Meddings Z, Jiang T, Liu Q, Teng Z, Lu J. Quantitative Histogram Analysis on Intracranial Atherosclerotic Plaques: A High-Resolution Magnetic Resonance Imaging Study. Stroke 2020;51:2161-9. [Crossref] [PubMed]
- McHugh ML. Interrater reliability: the kappa statistic. Biochem Med (Zagreb) 2012;22:276-82.
- Shen ZZ, Ren SJ, Wu RR, Su CQ, Ge S, Hong XN, Lu SS. Temporal changes in plaque characteristics after treatment and their relationship with stroke recurrence: a quantitative study using magnetic resonance imaging. Quant Imaging Med Surg 2022;12:4559-69. [Crossref] [PubMed]
- Yoon NK, Awad AW, Kalani MY, Taussky P, Park MS. Stent technology in ischemic stroke. Neurosurg Focus 2017;42:E11. [Crossref] [PubMed]
- Harteveld AA, Denswil NP, Van Hecke W, Kuijf HJ, Vink A, Spliet WGM, Daemen MJ, Luijten PR, Zwanenburg JJM, Hendrikse J, van der Kolk AG. Ex vivo vessel wall thickness measurements of the human circle of Willis using 7T MRI. Atherosclerosis 2018;273:106-14. [Crossref] [PubMed]
- Mattay RR, Saucedo JF, Lehman VT, Xiao J, Obusez EC, Raymond SB, Fan Z, Song JW. Current Clinical Applications of Intracranial Vessel Wall MR Imaging. Semin Ultrasound CT MR 2021;42:463-73. [Crossref] [PubMed]
- Song JW, Pavlou A, Xiao J, Kasner SE, Fan Z, Messé SR. Vessel Wall Magnetic Resonance Imaging Biomarkers of Symptomatic Intracranial Atherosclerosis: A Meta-Analysis. Stroke 2021;52:193-202. [Crossref] [PubMed]
- Huo R, Liu Y, Xu H, Li J, Xin R, Xing Z, Deng S, Wang T, Yuan H, Zhao X. Associations between carotid atherosclerotic plaque characteristics determined by magnetic resonance imaging and improvement of cognition in patients undergoing carotid endarterectomy. Quant Imaging Med Surg 2022;12:2891-903. [Crossref] [PubMed]
- Song X, Zhao X, Liebeskind DS, Wang L, Xu W, Xu Y, Hou D, Zheng Z, Wu J. Incremental value of plaque enhancement in predicting stroke recurrence in symptomatic intracranial atherosclerosis. Neuroradiology 2020;62:1123-31. [Crossref] [PubMed]
- Gupta A, Baradaran H, Al-Dasuqi K, Knight-Greenfield A, Giambrone AE, Delgado D, Wright D, Teng Z, Min JK, Navi BB, Iadecola C, Kamel H. Gadolinium Enhancement in Intracranial Atherosclerotic Plaque and Ischemic Stroke: A Systematic Review and Meta-Analysis. J Am Heart Assoc 2016;5:e003816. [Crossref] [PubMed]
- Gómez-Vicente B, Hernández-Pérez M, Martínez-Velasco E, Rodríguez-Velasco M, Munuera J, Rubiera M, Vert C, Dorado L, de Lera M, Calleja AI, Cortijo E, Agulla J, López-Cancio E, Arenillas JF. Intracranial atherosclerotic plaque enhancement and long-term risk of future strokes: A prospective, longitudinal study. J Neuroimaging 2023;33:289-301. [Crossref] [PubMed]
- Wang Y, Liu X, Wu X, Degnan AJ, Malhotra A, Zhu C. Culprit intracranial plaque without substantial stenosis in acute ischemic stroke on vessel wall MRI: A systematic review. Atherosclerosis 2019;287:112-21. [Crossref] [PubMed]
- Yu YN, Li ML, Xu YY, Meng Y, Trieu H, Villablanca JP, Gao S, Feng F, Liebeskind DS, Xu WH. Middle cerebral artery geometric features are associated with plaque distribution and stroke. Neurology 2018;91:e1760-9. [Crossref] [PubMed]
- Zhu C, Tian X, Degnan AJ, Shi Z, Zhang X, Chen L, Teng Z, Saloner D, Lu J, Liu Q. Clinical Significance of Intraplaque Hemorrhage in Low- and High-Grade Basilar Artery Stenosis on High-Resolution MRI. AJNR Am J Neuroradiol 2018;39:1286-92. [Crossref] [PubMed]
- de Havenon A, Mossa-Basha M, Shah L, Kim SE, Park M, Parker D, McNally JS. High-resolution vessel wall MRI for the evaluation of intracranial atherosclerotic disease. Neuroradiology 2017;59:1193-202. [Crossref] [PubMed]