Using artificial intelligence based imaging to predict lymph node metastasis in non-small cell lung cancer: a systematic review and meta-analysis
Introduction
Despite significant progress in the early detection and management of lung cancer, it has been the primary cause of cancer-related death worldwide for an extended period (1). The predominant histological subtype of lung cancer is non-small cell lung cancer (NSCLC), which comprises approximately 80–85% of all lung cancer cases (2). This subtype has a five-year survival rate of just 23.6% (2). Despite advances in clinical studies on the differential diagnosis, preoperative staging, efficacy evaluation, and prognosis prediction of NSCLC over the past decade, the prognosis of NSCLC patients remains poor This is primarily attributed to many patients having lymph node metastasis (LNM) or distant metastasis at the time of diagnosis. LNM is the predominant and consequential method of metastasis in patients with NSCLC. Approximately 16.6% of patients with tumor stage I (T1) NSCLC develop LNM (3). The survival rate beyond five years for NSCLC patients diagnosed with LNM ranges from 26% to 53% (4). In tumor-lymph node-metastasis (TNM) staging, node-staging (N-staging) plays a crucial role in the diagnosis, treatment strategy, and prognosis prediction of patients (5).
Existing approaches for evaluating LNM in lung cancer include clinical procedures, imaging techniques, and invasive medical examinations. LNM can be accurately assessed by imaging techniques such as ultrasonography, computed tomography (CT), magnetic resonance imaging (MRI), and positron emission tomography (PET)-CT. Lymph node biopsy and dissection are invasive techniques that provide direct access to lymph node tissue samples, enabling the confirmation of diagnosis and staging. However, despite the widespread use of these assessment techniques in patients diagnosed with lung cancer, each has its own inherent limitations. For example, imaging investigations are limited in their ability to identify micronodal metastasis and accurately evaluate the extent of metastasis. Further, in PET-CT scans, there is the potential of false positives when reactive non-metastatic lymph nodes exhibit fluorodeoxyglucose (FDG) concentrations (6). Despite their ability to provide conclusive outcomes, the efficacy of invasive testing is limited by its intrusive nature and the accompanying hazards. Thus, the development of novel and precise non-invasive assessment techniques has great clinical significance in the evaluation of LNM among patients diagnosed with lung cancer.
The advent of the digital age in recent years has resulted in a substantial increase in the volume of medical data. The wide variety of medical data has placed greater demands on data pre-processing, storage, analysis, and utilization. The continual advancement of AI technology is being deeply incorporated with clinical applications in lung cancer treatment (7). AI technology relies on extensive data training and deep-learning algorithms to autonomously extract information from medical pictures and execute precise analysis and decision making (8). AI algorithms are progressively becoming a significant part of the lung cancer imaging diagnosis. The primary components of AI include radiomics, machine learning, deep learning, and natural language processing procedures. Based on high-throughput automated data analysis, imageomics technology can automatically, accurately and quantitatively extract the image features of the region of interest, mine the changes of tissues, cells, proteins and nucleic acids implicit in the images through a large amount of data, analyze the pathophysiology, genetics and metabolism, signal transduction and other states of the region of interest. Consequently, it enables the accurate determination of tumor differentiation and staging and the prediction of tumor behavior (9).
Various machine-learning algorithms, such as logistic regression, support vector machines (SVMs), artificial neural networks, Bayesian, K-neighborhood algorithms, decision trees, and random forests (RFs), can be used to autonomously detect patient clinical variables and indicators, analyze patient medical data, and provide valuable insights that aid in the process of diagnostic and therapeutic decision making for specific cases. Machine-learning methods now have substantial clinical significance in the screening, diagnosis, and prognosis of lung cancer. Deep learning, which includes convolutional neural networks (CNNs), recurrent neural networks, generative adversarial networks, and transformers, represents a distinct category within the field of machine learning. These models employ deep networks to acquire knowledge iteratively and approximate intricate models, enabling robust functionalities. AI technology can extract abundant information from the imaging data of patients with lung cancer, enabling clinicians to precisely evaluate the presence and progression of tumors. Using deep learning and neural network technology, AI can autonomously detect lung cancer related lesion characteristics, including the form, size, and edge properties of the mass. This helps clinicians to promptly identify and diagnose a patient’s condition (10). Further, AI algorithms have been shown to have notable consistency and impartiality, enabling them to effectively make automated judgements using extensive training data and algorithmic models. This capability serves to reduce diagnostic disparities among medical practitioners (11). Unlike conventional human interpretation techniques, AI algorithms can provide automated image analysis and diagnosis. This leads to a significant reduction in the time required for diagnosis and an enhancement in lung cancer’s early detection rate and treatment efficacy (12). Urso et al. (13) have shown that imaging genomics and AI enable the detection of characteristics in medical images that are often imperceptible to the human eye. These features have significant promise for staging, prognosis, and biological assessment applications.
Recently, several scholars have developed imaging-based AI models to predict LNM (14-24). The accurate preoperative prediction of lymph node status facilitates the selection of lung segmentectomy or wedge resection for early-stage patients with negative lymph nodes. Notably, lymph node status cannot be assessed during wedge resection. Conversely, knowledge of patients’ lymph node metastatic status could help to stratify patients and inform treatment decisions. Lobectomy and radical lymph node dissection could improve the survival of high-risk patients. While less invasive procedures, such as sub-lobar resection or wedge resection, could improve the quality of life of low-risk patients. In addition, for patients planning to undergo radiation therapy, accurate mediastinal staging can help oncologists designate irradiation fields and reduce the risk of treatment failure due to occult LNM. Both NSCLC and small cell lung cancer can be evaluated by TNM reports, which can inform decisions about treatment strategies. Therefore, the accurate prediction of N-staging in the TNM report can provide clinicians with additional information for the selection of treatment strategies such as surgery, chemotherapy, and radiation therapy. Due to the limited number and diversity of image features, developing more reliable methods based on imaging to accurately predict LNM in NSCLC would be an essential advancement in diagnostic technology.
Several studies have examined the use of AI in predicting LNM in lung cancer; however, systematic assessments evaluating the predictive accuracy of AI-based imaging data are limited. This study sought to conduct a systematic review and meta-analysis of existing research data on the use of AI algorithm-assisted imaging for the evaluation of LNM in lung cancer. More specifically, this study sought to examine the diagnostic accuracy, sensitivity, and specificity of this imaging technique. By using AI algorithms, practitioners could enhance their understanding of and ability to predict LNM in lung cancer patients, which in turn could enhance the precision of early detection and the reliability of treatment decision making, ultimately leading to improved treatment outcomes and survival rates for lung cancer patients. We present this article in accordance with the PRISMA-DTA reporting checklist (25) (available at https://qims.amegroups.com/article/view/10.21037/qims-24-664/rc).
Methods
We conducted a systematic review and meta-analysis of diagnostic test accuracy studies. This study has been registered in the International Prospective Registry for Systematic Evaluation (PROSPERO) (ID: CRD42024516017).
Search strategies and literature screening
We conducted an extensive literature search to retrieve relevant articles. We searched the PubMed (Medline), Embase, Cochrane Library, and Web of Science databases to retrieve relevant articles published up to January 31, 2024. We used a combination of keywords, including subject headings (MeSH)/Emtree glossaries combined with free words, to search the titles and abstracts of articles. The following keywords were used: “non-small cell lung cancer”, “lymph node metastasis”, “artificial intelligence”, “deep learning”, “machine learning”, and “radiomics”. For more information on the search keywords used for each database, please see the table available at https://cdn.amegroups.cn/static/public/qims-24-664-1.xlsx. Searches were limited to English-language publications; however, there were no restrictions on the year of publication, age, or location of participants. Additionally, we manually searched the reference lists of the relevant studies and meta-analyses to ensure we identified any potentially relevant research articles. Two researchers independently selected studies based on pre-specified inclusion and exclusion criteria, and imported the literature into Endnote X9.3.1 (Clarivate Analytics, US) for management. Duplicate articles or ineligible studies were excluded. We identified studies that met the inclusion criteria by screening the titles, abstracts, and full text of all the articles.
Inclusion and exclusion criteria
After removing the duplicate articles, the remaining articles were reviewed to identify studies that met the following include criteria: (I) population: the study included patients with pathologically confirmed NSCLC (including adenocarcinoma, squamous cell carcinoma, and large cell carcinoma); (II) intervention: the study sought to predict LNM using an AI algorithm based on quantitative imaging data; (III) outcome: the study used the area under the curve (AUC) as the primary outcome metric, and sensitivity, specificity, and the positive likelihood ratio (PLR), and the negative likelihood ratio (NLR) as the secondary outcome metrics; and (IV) study design: the study was an observational study (retrospective or prospective), randomized or non-randomized controlled trial. Articles were excluded from the meta-analysis if they met any of the following criteria: (I) repeated studies with similar data; (II) were related to an animal study, case report, literature review, or conference abstract; and/or (III) were published in languages other than English. The above inclusion and exclusion criteria were employed to ensure the quality and reliability of the studies, and minimize potential biases and errors. Two researchers performed the data extraction independently, and a third researcher was consulted if any differences of opinion arose.
Data extraction and quality assessment
The following data were extracted from each of the included studies: (I) surname of the first author; (II) year of publication; (III) origin(s) of the patients; (IV) type of study; (V) number and average age of patients; (VI) metastasis/non-metastasis status; (VII) use of the gold standard for LNM (yes/no); (VIII) method of validation of the model; (IX) diagnostic endpoints; (X) type of imaging data; (XI) AI algorithms used to construct the model; and (XII) AUC, and other parameters. Four-cell tabulated data (2×2) were collected, including the true positive, true negative, false positive, and false negative. When comparing the diagnostic performance of different algorithms for the same sample, the algorithm that produced the best results was selected. If there were no sensitivities or specificities in a study, the Engauge Digitizer (version 12.1, Mark Mitchell) was used to calculate the sensitivities and specificities at the maximum of the Youden Index based on the receiver operating characteristic (ROC) curves from the article. If there were more than two models for the same group of patients in a study, the model with the higher AUC value was included in our meta-analysis.
The quality of the included studies was assessed by two reviewers using the Quality Assessment of Diagnostic Accuracy Studies-2 (QUADAS-2) (26). This tool assessed the “risk of bias” of the studies across the following four key domains: patient selection; index test; reference standard; flow and timing. The applicability of the first three domains was also assessed using a rating of “high”, “low”, or “unclear”. For individual studies, the risk of bias for each domain was assessed as “high”, “low”, or “unclear”. The evaluation was performed using Revman 5.3 (Cochrane Collaboration, UK).
Statistical analysis
Stata 14.2 (StataCorp LP, College Station, TX, USA) was used for the data analysis. Due to the significant heterogeneity of this study, we combined the relevant diagnostic accuracy indicators, including sensitivity, specificity, diagnostic odds ratio (DOR), NLR, and PLR, using a bivariate random-effects model. The model’s AUC was calculated using the summary receiver operating characteristic (SROC). A threshold effect test was conducted using Meta-disc version 1.4 (Hospital Ramon y Cajal and Complutense University of Madrid, ESP). The presence or absence of a threshold effect was determined by calculating the Spearman’s correlation coefficient between the logarithm of sensitivity and the logarithm of (1-specificity). A strong positive correlation indicated the presence of a threshold effect. The heterogeneity of the results of the included studies was assessed using Cochran’s Q test, combined with I2 statistics. By adding covariates to the model, meta-regression and subgroup analyses based on country (China or other), sample size, data source (single-center or multi-center), imaging modality (CT or PET/CT), model validation modality (train-test split or cross-validation), and the algorithm used to construct the model [least absolute shrinkage and selection operator (LASSO), SVM, or neural network were performed to identify the sources of heterogeneity]. The likelihood of publication bias was assessed using funnel plots, and the stability of the results was assessed by sensitivity analyses. Clinical utility was evaluated using Fagan plots, which provided the pretest probability of lymphatic metastasis when calculating the post-test probability. The combined effect value of multiple studies was statistically significant if P≤0.05.
Results
Literature search results
Figure 1 summarizes the results of the literature search and screening of relevant studies. Initially, 304 articles were retrieved from the searched databases; that is, PubMed [41], Embase [62], Cochrane Library [0], and Web of Science [201]. Using software and manually removing duplicate records, 55 duplicate articles were successfully eliminated. Following the application of the inclusion/exclusion criteria, 11 articles (14-24) were selected for inclusion in the meta-analysis.
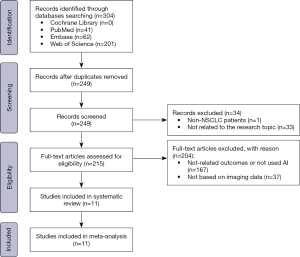
Basic characteristics of the included literature
Tables 1 and 2 detail the characteristics of each eligible study. All the included studies were published between 2020 and 2024. The study sample sizes ranged from 71 to 1,325 patients with a mean age of 58 to 74 years; four studies did not mention patient age. Almost all of these studies were retrospective in nature, and most were single-center studies; only three were multi-center studies. For the model validation, all of the studies used internal validation, including cross-validation and randomized division of the dataset. Of the 11 eligible studies, 9 used CT, and 2 used PET/CT to train their algorithms. Different AI algorithms were used for modeling, with common algorithms including SVMs, RFs, neural networks, LASSO regression, and extreme gradient boosting (XGBoost). All the studies diagnosed LNM based on a pathologic examination of the surgical resection sample.
Table 1
Study | Design | Data source | Country | Population | Number of patients | Metastasis/non-metastasis | Age (years) | Validation method |
---|---|---|---|---|---|---|---|---|
Tian, 2024 (14) | Retrospective | Multi-center | China | Patients with solid-predominantly invasive lung adenocarcinoma | 1,325 | 478/847 | 59.9±9.2 (training); 60.0±9.7 (validation); 59.4±10.6 (test) | Train-test split |
Shimada, 2023 (15) | Retrospective | Single center | Japan | Patients with non-small cell lung cancer | 720 | 83/637 | 66±10 (training); 67±10 (validation) |
Train-test split |
Ma, 2023 (16) | Retrospective | Single center | China | Patients with pathologically confirmed lung adenocarcinoma | 720 | 124/596 | 60.0 [52.5–64.0] [training]; 59.0 [53.0–66.0] [validation]; 58.5 [51.3–66.0] [test] |
Train-test split |
Zhang, 2023 (17) | Retrospective | Single center | China | Patients with lung cancer | 140 | 67/73 | 60.26±8.93 (training); 59.80±9.91 (test) |
Train-test split |
Wang, 2023 (18) | Retrospective | Single center | China | Patients with non-small cell lung cancer | 462 | 176/286 | 63.77±9.13 | Cross-validation |
Hu, 2023 (19) | Retrospective | Single center | China | Patients with non-small cell lung cancer | 681 | 92/589 | 61.22±9.51 | Cross-validation |
Yin, 2021 (20) | Retrospective | Single center | China | Patients with lung cancer | 71 | 20/51 | 61±8 | Train-test split |
Zhao, 2021 (21) | Retrospective | Multi-center | China | Patients with lung adenocarcinoma | 554 | 146/408 | NR | Cross-validation |
Geng, 2022 (22) | Retrospective | Single center | China | Patients with non-small cell lung cancer | 82 | 48/34 | NR | Train-test split |
Zhao, 2020 (23) | Retrospective | Multi-center | China | patients with lung adenocarcinoma | 501 | 133/368 | NR | Cross-validation |
Wu, 2020 (24) | Retrospective | Single center | China | Patients with non-small cell lung cancer | 1,102 | 116/986 | 58 [51–65] | Train-test split |
Age is expressed as the mean ± standard deviation or median [range]. NR, not reported.
Table 2
Study | AI algorithm | Image | Reference standard | Outcome definition | AUC | Accuracy | Specificity | Sensitivity |
---|---|---|---|---|---|---|---|---|
Tian, 2024 (14) | SVM, ResNet, DenseNet, Swin Transformer | CT | Surgical resection | LNM | 0.84 | 0.78 | 0.72 | 0.83 |
Shimada, 2023 (15) | CNN | CT | Surgical resection | LNM | 0.93 | 0.68 | 0.68 | 0.7 |
Ma, 2023 (16) | Swin Transformer | CT | Surgical resection | LNM | 0.97 | NR | 0.94 | 0.91 |
Zhang, 2023 (17) | LASSO regression, CNN, transfer learning | CT | Surgical resection | LNM | 0.95 | 0.85 | 0.84 | 0.85 |
Wang, 2023 (18) | LASSO regression, MLP, LightGBM, SVM, GBDT, XGBoost |
PET/CT | Surgical resection | LNM | 0.94 | 0.93 | 0.88 | 0.97 |
Hu, 2023 (19) | LR, SVM, ResNet,DenseNet, EfficientNet, ViT, Swin transform, MHGF, RF, GBDT, MLP, DensePriNet, 3M-CN, SR-CorRinE | CT | Surgical resection | LNM | 0.81 | NR | NR | NR |
Yin, 2021 (20) | SVM, RF, KNN | PET/CT | Surgical resection | LNM | 0.94 | 0.92 | NR | NR |
Zhao, 2021 (21) | 3M-CN | CT | Surgical resection | LNM | 0.95 | NR | 0.93 | 0.88 |
Geng, 2022 (22) | ANN, LASSO regression | CT | Surgical resection | LNM | 0.86 | 0.98 | 0.77 | 0.81 |
Zhao, 2020 (23) | CNN, LASSO regression | CT | Surgical resection | LNM | 0.93 | 0.88 | 0.88 | 0.86 |
Wu, 2020 (24) | AdaBoost, ANN, DT, GBDT, LR, MNB, RFC, XGBoost | CT | Surgical resection | LNM | 0.89 | NR | 0.71 | 0.93 |
AI, artificial intelligence; AUC, area under the curve; SVM, support vector machine; CT, computed tomography; LNM, lymph node metastasis; CNN, convolutional neural network; NR, not reported; LASSO, least absolute shrinkage and selection operator; MLP, multilayer perceptron; LightGBM, light gradient boosting machine; GBDT, gradient boosting decision tree; XGBoost, extreme gradient boosting; LR, logistic regression; ViT, vision transformer; MHGF, multi-modal heterogeneous graph forest; RF, random forest; 3M-CN, 3D multi-scale, multi-task, multi-label classification network; SR-CorRinE, core-ring residual estimation with size-related damper block; KNN, k-nearest neighbor; PET, positron emission tomography; ANN, artificial neural network; AdaBoost, adaptive boosting; DT, decision tree; MNB, multinomial naive bayes; RFC, random forest classifier.
Quality assessment
QUADAS-2 was used to examine the risk of bias and applicability issues of the included studies (Figure 2). In relation to patient selection, the overall risk of bias was low; only two studies were rated as having a high risk of bias because they did not avoid inappropriate exclusions. In relation to the “diagnostic tests to be evaluated”, four studies were rated as having an unclear risk of bias because they did not mention the timing of the interpretation of the results and the determination of thresholds. In relation to the gold standard, case process, and progression, almost all of the studies were rated as having a low risk of bias. Overall, the quality of the included articles was almost acceptable according to the QUADAS-2 results.
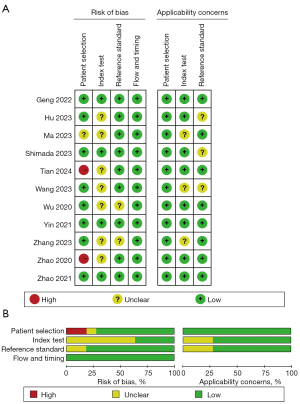
A meta-analysis of the accuracy of AI-based imaging models for predicting LNM
The forest plots and combined results of the meta-analysis for predicting LNM in patients with NSCLC using an AI-based imaging model are shown in Figures 3-5. The diagnostic threshold analysis showed no significant threshold effect (Spearman correlation coefficient =−0.409, P=0.212). The pooled sensitivity of the model was 0.87 [95% confidence interval (CI): 0.80–0.91; P<0.001; I2=90.80%], the specificity was 0.85 (95% CI: 0.78–0.89; P<0.001; I2=97.61%), the PLR was 5.64 (95% CI: 3.88–8.21; I2=96.58%), the NLR was 0.15 (95% CI: 0.10–0.24; I2=93.28%), the DOR was 36.51 (95% CI: 17.57–75.87), and the diagnostic score was 3.60 (95% CI: 2.87–4.33). The AUC based on the pooled ROC (SROC) curve was 0.92 (95% CI: 0.90–0.94; Figure 6). The I2 values for the sensitivity, specificity, PLR, and NLR indicated high heterogeneity. To further examine the source of heterogeneity, we performed a meta-regression analysis. The results showed that the following factors were significantly associated with study heterogeneity in the meta-regression model: imaging modality (CT or PET/CT) (P value =0.03), and the use of a neural network algorithm to construct the model (P value =0.01) (Table 3).
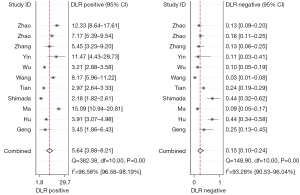
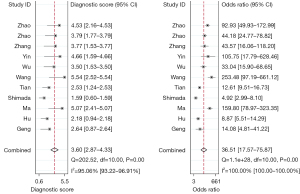
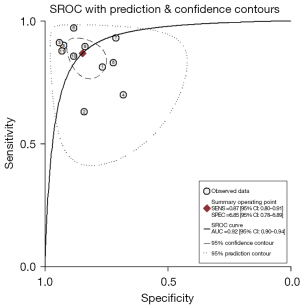
Table 3
Variable | n | Sensitivity (95% CI) | P1 | Specificity (95% CI) | P2 | Joint model analysis | ||
---|---|---|---|---|---|---|---|---|
LRT Chi2 | P value | I2 (%) | ||||||
Country | 0.41 | 0.56 | 3.71 | 0.16 | 46 | |||
China | 10 | 0.98 (0.83–0.93) | 0.86 (0.81–0.91) | |||||
Others | 1 | 0.70 (0.42–0.98) | 0.68 (0.43–0.93) | |||||
Sample size (numbers) | 0.12 | 0.07 | 0.05 | 0.98 | 0 | |||
>150 | 8 | 0.87 (0.80–0.93) | 0.84 (0.78–0.91) | |||||
≤150 | 3 | 0.88 (0.77–0.99) | 0.81 (0.74–0.96) | |||||
Data source | 0.00 | 0.08 | 0.32 | 0.85 | 0 | |||
Multi-center | 3 | 0.86 (0.77–0.95) | 0.86 (0.77–0.95) | |||||
Single center | 8 | 0.89 (0.84–0.95) | 0.84 (0.77–0.90) | |||||
Imaging mode | 0.00 | 0.02 | 6.89 | 0.03 | 71 | |||
CT | 9 | 0.84 (0.79–0.90) | 0.83 (0.77–0.89) | |||||
PET/CT | 2 | 0.96 (0.92–1.00) | 0.90 (0.81–0.99) | |||||
Validation methods | 0.10 | 0.09 | 2.44 | 0.29 | 18 | |||
Train-test split | 7 | 0.86 (0.80–0.92) | 0.79 (0.71–0.87) | |||||
Cross-validation | 4 | 0.91 (0.86–0.97) | 0.90 (0.84–0.96) | |||||
AI algorithm | ||||||||
NN | 8 | 0.83 (0.77–0.89) | 0.00 | 0.81 (0.75–0.87) | 0.00 | 8.57 | 0.01 | 77 |
LASSO regression | 4 | 0.90 (0.84–0.97) | 0.19 | 0.85 (0.76–0.94) | 0.03 | 1.22 | 0.54 | 0 |
SVM | 4 | 0.87 (0.78–0.96) | 0.07 | 0.85 (0.76–0.94) | 0.03 | 0.00 | 1.00 | 0 |
Others | 8 | 0.88 (0.83–0.94) | 0.33 | 0.84 (0.78–0.91) | 0.03 | 1.24 | 0.54 | 0 |
CI, confidence interval; LRT, likelihood ratio test; CT, computed tomography; PET, positron emission tomography; AI, artificial intelligence; NN, neural network; LASSO, least absolute shrinkage and selection operator; SVM, support vector machine.
Based on the subgroup analyses, we found that models constructed from sample size data from a single center had a higher sensitivity (89% vs. 86%, P value =0.00), and models built based on CT images had less sensitivity and specificity than those based on PET/CT images (84% vs. 96%, P value =0.00; 83% vs. 90%, P value =0.02). In addition, the sensitivity (83% vs. 94%, P value =0.00) and specificity (81% vs. 92%, P value =0.00) of studies using neural networks for model construction were lower than those using other methods [i.e., algorithms other than neural networks, such as SVM, gradient boosting decision tree (GBDT), XGBoost, multilayer perceptron (MLP), and multinomial naive Bayes (MNB)]. The specificity of the studies that used LASSO and SVM for model construction was higher than that of the studies that used other methods (i.e., algorithms other than LASSO and SVM, such as GBDT, XGBoost, MLP, and MNB) for model construction (85% vs. 84%, P value =0.03). The specificity of the studies that did not use other methods (i.e., methods other than neural networks, LASSO, and SVM) to construct the model was also relatively high (85% vs. 84%, P value =0.03) for other methods (e.g., GBDT, XGBoost, MLP, and MNB).
We performed a publication bias analysis of the included studies (Figure 7). The funnel plot asymmetry test showed no significant publication bias for the included studies (P=0.58). When conducting the meta-analysis, we also performed a sensitivity analysis (Figure 8), which showed that the point estimate of the combined effect size fell between the 95% CIs of the total combined effect size after deleting a study, indicating the stability of the findings.
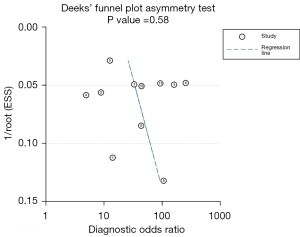
Studies have shown (27) that the pre-detection probability of the Fagan plot usually depends on the prevalence of the disease. Thus, we searched the related literature and summarized the estimates. The estimates showed that the probability of LNM in NSCLC was about 19.4%; thus, we set the pre-detection probability as 19.4%. Using the AI-based imaging model, the post-test probability increased from 19% to 58% when the pretest was positive with a PLR of 6. However, when the pretest was negative, the post-test probability decreased to 4% with a NLR of 0.15 (Figure 9). Thus, when the predictive model results were positive, it increased the likelihood of LNM (increasing the post-test probability from 19% to 58%), and when the predictive model results were negative, it provided evidence against the occurrence of LNM (decreasing the post-test probability from 19% to 4%). These findings suggest that AI models are helpful in clinical practice.
Discussion
AI and the value of the results of this meta-analysis
AI has become widely used in medical imaging, which has led to the active prediction of LNM in different malignancies using radiomics and deep-learning models. The primary objective of this study was to conduct a meta-analysis on the use of AI in medical imaging to predict the spread of cancer cells to the lymph nodes in patients with NSCLC. We thoroughly examined multiple recent studies to evaluate the efficacy of AI algorithms in this domain. Our analysis of these studies indicated that AI-based imaging has high predictive accuracy and reliability, particularly in predicting LNM in patients with NSCLC. AI algorithms can assist clinicians to accurately predict the occurrence of LNM in patients with NSCLC. This is essential for directing the creation of personalized treatment regimens and the selection of suitable treatment modalities.
In addition, the use of AI algorithms in image interpretation effectively minimizes the number of unnecessary puncture biopsies and surgical resections, which in turn, reduces the physical and psychological strain placed on patients while enhancing their treatment outcomes and overall quality of life. Unnecessary lymph node dissection can be avoided if lymph node metastatic status can be accurately predicted preoperatively. Lymph node status is first diagnosed by radiologic techniques (CT or PET/CT); however, this conventional technique may not accurately reflect lymph node status, as it is challenging to detect microscopic disease with conventional imaging features alone. Moreover, current image-based preoperative assessments of lymph node status largely depend on the subjective judgment of radiologists, and can be both time-consuming and inaccurate. Further, incorrect assessments of lymph node status can lead to under- or over-treatment and increase the risk of recurrence and postoperative complications (28). Therefore, developing an effective tool to diagnose lymph node status accurately is essential, as it could ensure better clinical treatment for lung adenocarcinoma patients. Our results and findings provide a powerful tool for therapeutic decision-making and prognostic assessment of NSCLC patients.
Comparison of different imaging modalities in the prediction of LNM
This was the first meta-analysis to assess the diagnostic efficacy of imaging modalities (such as CT and PET/CT) in predicting the spread of cancer cells to lymph nodes in patients with lung cancer using AI algorithms. Previous research has investigated the application of imaging techniques in detecting the spread of lung cancer to lymph nodes. However, only a few studies have thoroughly combined and assessed this study area of the application of imaging techniques to detect the spread of lung cancer to the lymph nodes. Previous research has largely sought to evaluate the spread of lung cancer to the lymph nodes using CT scans. Currently, CT is the most widely used imaging method and is extensively employed to evaluate the health of lymph nodes before surgery. CT is crucial in the regular diagnosis, analysis, and assessment of treatment effectiveness for lung cancer due to its exceptional spatial resolution. The clinical information provided by CT images, including information about the shape, size, and orientation of nodes, plays a crucial role in disease diagnosis and prognosis prediction (29,30). Multiple research studies have successfully used AI-based CT images to predict the occurrence of lung cancer LNM (14-17,19,21-24). Typically, conventional CT scans identify metastatic lymph nodes based on their physical characteristics, such as a short diameter greater than 10 mm and a short-to-long diameter ratio. CT is ineffective in diagnosing or ruling out metastatic lymph nodes when the mass is enlarged because of micrometastases or inflammatory lesions (31).
Compared with CT, PET/CT and dual-energy CT have unique advantages and disadvantages in assessing LNM in lung cancer patients. First, PET/CT characterizes the metabolic and anatomical features of lesions. It is also more reliable than conventional CT morphometric indices in the staging of lung cancer lymph nodes (32). However, PET/CT does not avoid invasive lymph node sampling to confirm metastasis and does not exclude LNM in patients without metabolically active lymph nodes, and it still has a high false-positive rate in detecting malignancies in normal-sized lymph nodes and in excluding malignancies in patients with comorbid inflammatory or infectious diseases. A meta-analysis by Seol et al. (33) reported that the pooled specificity of PET/CT was 0.65, and the false-positive rate was approximately 35%.
Recently, spectral CT, as a novel and convenient imaging modality, has been shown to be valuable in the preoperative assessment of LNM and can provide additional quantitative metrics through analytical tools (34). This multiparametric quantitative technique extends the capabilities of conventional CT to enable real-time, isotropic, homologous, simultaneous, accurate material separation and quantitative analysis, and had may be able to reduce contrast, radiation dose, or suppressing artifacts (35). The results of a meta-analysis of 11 studies (comprising 1,290 patients) by Wang et al. (36) reported that dual-energy CT in predicting LNM of lung cancer had a combined AUC greater than 0.8.
Performance of AI models
AI techniques are non-intrusive diagnostic tools that offer clinicians novel perspectives in disease management, particularly in oncology. Consequently, an increasing number of studies have examined the suitability of AI in many types of cancer, including gastrointestinal, pulmonary, neurological, and breast cancer. Liu et al. (37) conducted a systematic review and statistical analysis of 16 studies involving 4,764 patients diagnosed with breast cancer, CT, and MRI scans, and showed that the AI algorithms had high diagnostic precision in predicting the occurrence of LNM in breast cancer patients. The sensitivity, specificity and AUC of the summarized MRI scans were 0.85, 0.81, and 0.85, respectively. While the sensitivity, specificity, and AUC of the summarized CT images were 0.88, 0.80, and 0.91, respectively. Bedrikovetski et al. (38) performed a meta-analysis to evaluate the precision of AI in diagnosing LNM in colorectal cancer using CT/MRI, and reported an AUC of 0.917, which indicated that the performance of AI was considerably superior to that of radiologists. Hakkak Moghadam Torbati et al. (40) based on machine learning and a texture analysis of [18F] FDG PET/CT images to predict distant metastasis in NSCLC patients, showing an AUC of 0.63 and an accuracy of 74.4%. Thus, we believe that AI algorithms can enhance the precision and reliability of predicting LNM in lung cancer through image analysis, thereby addressing the inherent constraints of imaging.
In this meta-analysis, we analyzed an imaging model built based on AI algorithms, and the model’s sensitivity, specificity, and AUC were approximately 0.87, 0.85, and 0.92, respectively, indicating good performance. The confirmation of metastatic lymph node pathology, which was used as the reference standard for inclusion in the study, was determined postoperatively. However, in practice, if treatments need to be adjusted based on lymph node status, it would be better to determine that status before surgery. Imaging models based on AI algorithms have excellent predictive capabilities and can predict positive LNM results in patients preoperatively without unnecessary invasive interventions. In addition, the 84% specificity allowed us to determine with reasonable certainty that patients with positive model predictions of LNM required optimized treatment. Thus, the imaging models based on AI algorithms had good diagnostic performance.
Analysis of sources of heterogeneity
In our analyses, we found a high degree of heterogeneity between studies. We explored the possible sources of heterogeneity using subgroup analyses and meta-regression. The Spearman correlation coefficient was not a source of heterogeneity. However, differences between different imaging modalities and different algorithms could have been sources of heterogeneity. The results suggest that PET/CT (2/11) imaging modalities are more sensitive than CT imaging modalities, but most studies used CT imaging modalities (9/11) rather than PET/CT. In relation to the type of AI algorithms used for modeling, in the studies included in our meta-analysis, neural networks were the most commonly used AI algorithms.Neural networks as a special class of machine learning, by building deep networks continuously learn and approximate real complex models, that can acquire global and local image information directly from the nucleus,to achieve powerful learning capabilities and flexible diagnostic functions (7). Tian et al. (14,15,17,19,21-24) used a neural network classifier to predict LNM, and reported a pooled AUC of 0.89 (Figure 10A), which indicates fair overall performance; however, this algorithm had relatively low sensitivity and characterization compared to other algorithms. LASSO is a linear regression method that uses L1 regularization, which allows for feature sparsity and selection by making the learned weights of certain features zero. In this meta-analysis, LASSO had a higher specificity than the other algorithms, and the sensitivity was relatively high but not statistically significant, with a pooled AUC of 0.89 (Figure 10B).
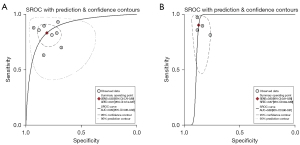
Other algorithms include the SVM, XGBoost, and RF. The SVM is one of the most popular classification techniques, is an excellent algorithm for modeling misspecification, and can effectively handle high-dimensional data (39). Hakkak Moghadam Torbati et al. (40) compared six machine-learning methods to select the best-trained model for predicting distant metastasis in NSCLC patients. The SVM and decision tree methods had the highest prediction accuracy for the whole set of variables. XGBoost is an optimized distributed gradient boosting library that is efficient, flexible, and portable. RFs are composed of many trees, but they are differentiated by randomly selecting variables to reduce the correlation between the fitted trees (41). However, the data for these algorithms are not sufficient, and the literature we have included revealed little about the calibration of the AI based imaging model, the purpose of calibration is to bring the model closer to reality in order to maintain the accuracy of its predictions. Therefore, in the future, it will be necessary to judge the accuracy of model predictions based on larger sample sizes and calibration of the joined models. Moreover, multiple polynomial models should be used in clinical routines to improve the diagnostic performance of the models.
The results of the meta-analysis are encouraging. With a pooled sensitivity of approximately 0.88, almost 90% of patients with LNM will be detected. However, this also implies that LNM may be undetected in 12 out of every 100 patients, which could negatively affect the overall survival of the 12% of patients with positive LNM. Thus, it is important to recognize that AI may not be suitable for routine clinical examinations, particularly when patient survival is used as the primary measure of a model’s predictive power.
Limitations of this study
Our meta-analysis had several limitations. First, almost all of the 11 studies included in this meta-analysis were retrospective, and such studies are prone to confounding bias and selection bias. Our ultimate goal was to apply the developed imaging model based on AI algorithms to improve prognosis prediction. On this basis, our model and estimation results should be generalizable to practice. However, most of the included studies used internal model validation, which is more prone to overestimation and lacks generalizability. Therefore, more prospective studies and more external validation studies need to be conducted to assess the model’s performance on unseen data before its application in the clinic. Second, only 11 original research articles met the selection criteria, as few studies have sought to predict LNM in lung cancer patients. We could only retrieve limited data from the specific studies, and the limited number of studies made it challenging to compare the results. Third, the classification performance of algorithms vary; therefore, we chose the highest-performing algorithm, which led to heterogeneity. However, this is common in meta-analyses of imaging-based profound learning studies (38,42-45). In addition, only six of the models in the results of all the studies included in this meta-analysis provided prediction CIs, and the rest did not assess the variability (or margin of error) of the predictions, resulting in incomplete data for assessing model performance. Fourth, only three studies had patients from multiple centers; recruiting patients from single centers also limited the generalizability and reproducibility of the results. Moreover, as almost all of the included studies (10/11) were from China, regional bias should be considered, and it could reduce the generalizability of the AI model outside China. Finally, the predictive performance of the algorithm may vary for different types of lung cancer LNMs (e.g., micro metastases and occult metastases), which must also be carefully considered.
Conclusions
Our results indicate that AI algorithm-based imaging accurately predicts LNM in NSCLC patients. Our findings provide new ideas and methods for the diagnosis and treatment of lung cancer patients. However, the high heterogeneity of the studies suggests that uniform AI guidelines are needed for upcoming studies. It is recommended that prospective studies with large sample sizes at multiple centers be conducted to help update predictive the models and make them compatible with new data. This will improve the performance of imaging models based on AI algorithms. Future studies should seek to explore and optimize the use of AI in diagnosing and treating lung cancer patients, enhancing its clinical value and potential.
Acknowledgments
Funding: This study was supported by grants from
Footnote
Reporting Checklist: The authors have completed the PRISMA-DTA reporting checklist. Available at https://qims.amegroups.com/article/view/10.21037/qims-24-664/rc
Conflicts of Interest: All authors have completed the ICMJE uniform disclosure form (available at https://qims.amegroups.com/article/view/10.21037/qims-24-664/coif). The authors have no conflicts of interest to declare.
Ethical Statement: The authors are accountable for all aspects of the work in ensuring that questions related to the accuracy or integrity of any part of the work are appropriately investigated and resolved.
Open Access Statement: This is an Open Access article distributed in accordance with the Creative Commons Attribution-NonCommercial-NoDerivs 4.0 International License (CC BY-NC-ND 4.0), which permits the non-commercial replication and distribution of the article with the strict proviso that no changes or edits are made and the original work is properly cited (including links to both the formal publication through the relevant DOI and the license). See: https://creativecommons.org/licenses/by-nc-nd/4.0/.
References
- Siegel RL, Miller KD, Wagle NS, Jemal A. Cancer statistics, 2023. CA Cancer J Clin 2023;73:17-48. [Crossref] [PubMed]
- Nasim F, Sabath BF, Eapen GA. Lung Cancer. Med Clin North Am 2019;103:463-73. [Crossref] [PubMed]
- Chen B, Wang X, Yu X, Xia WJ, Zhao H, Li XF, Liu LX, Liu Y, Hu J, Fu XN, Li Y, Xu YJ, Liu DR, Yang HY, Xu L, Jiang F. Lymph node metastasis in Chinese patients with clinical T1 non-small cell lung cancer: A multicenter real-world observational study. Thorac Cancer 2019;10:533-42. [Crossref] [PubMed]
- Song H, Yoon SH, Kim J, Kim J, Lee KW, Lee W, Lee S, Kim K, Lee CT, Chung JH, Lee KH. Application of N Descriptors Proposed by the International Association for the Study of Lung Cancer in Clinical Staging. Radiology 2021;300:450-7. [Crossref] [PubMed]
- Feng SH, Yang ST. The new 8th TNM staging system of lung cancer and its potential imaging interpretation pitfalls and limitations with CT image demonstrations. Diagn Interv Radiol 2019;25:270-9. [Crossref] [PubMed]
- Culverwell AD, Scarsbrook AF, Chowdhury FU. False-positive uptake on 2-[18F]-fluoro-2-deoxy-D-glucose (FDG) positron-emission tomography/computed tomography (PET/CT) in oncological imaging. Clin Radiol 2011;66:366-82.
- Huang S, Yang J, Shen N, Xu Q, Zhao Q. Artificial intelligence in lung cancer diagnosis and prognosis: Current application and future perspective. Semin Cancer Biol 2023;89:30-7. [Crossref] [PubMed]
- Lipkova J, Chen RJ, Chen B, Lu MY, Barbieri M, Shao D, Vaidya AJ, Chen C, Zhuang L, Williamson DFK, Shaban M, Chen TY, Mahmood F. Artificial intelligence for multimodal data integration in oncology. Cancer Cell 2022;40:1095-110. [Crossref] [PubMed]
- Gillies RJ, Kinahan PE, Hricak H. Radiomics: Images Are More than Pictures, They Are Data. Radiology 2016;278:563-77. [Crossref] [PubMed]
- Pei Q, Luo Y, Chen Y, Li J, Xie D, Ye T. Artificial intelligence in clinical applications for lung cancer: diagnosis, treatment and prognosis. Clin Chem Lab Med 2022;60:1974-83. [Crossref] [PubMed]
- Jiang Y, Yang M, Wang S, Li X, Sun Y. Emerging role of deep learning-based artificial intelligence in tumor pathology. Cancer Commun (Lond) 2020;40:154-66. [Crossref] [PubMed]
- Chauvie S, De Maggi A, Baralis I, Dalmasso F, Berchialla P, Priotto R, Violino P, Mazza F, Melloni G, Grosso M. SOS Study team. Artificial intelligence and radiomics enhance the positive predictive value of digital chest tomosynthesis for lung cancer detection within SOS clinical trial. Eur Radiol 2020;30:4134-40. [Crossref] [PubMed]
- Urso L, Manco L, Castello A, Evangelista L, Guidi G, Castellani M, Florimonte L, Cittanti C, Turra A, Panareo S. PET-Derived Radiomics and Artificial Intelligence in Breast Cancer: A Systematic Review. Int J Mol Sci 2022;23:13409. [Crossref] [PubMed]
- Tian W, Yan Q, Huang X, Feng R, Shan F, Geng D, Zhang Z. Predicting occult lymph node metastasis in solid-predominantly invasive lung adenocarcinoma across multiple centers using radiomics-deep learning fusion model. Cancer Imaging 2024;24:8. [Crossref] [PubMed]
- Shimada Y, Kudo Y, Maehara S, Fukuta K, Masuno R, Park J, Ikeda N. Artificial intelligence-based radiomics for the prediction of nodal metastasis in early-stage lung cancer. Sci Rep 2023;13:1028. [Crossref] [PubMed]
- Ma X, Xia L, Chen J, Wan W, Zhou W. Development and validation of a deep learning signature for predicting lymph node metastasis in lung adenocarcinoma: comparison with radiomics signature and clinical-semantic model. Eur Radiol 2023;33:1949-62. [Crossref] [PubMed]
- Zhang H, Liao M, Guo Q, Chen J, Wang S, Liu S, Xiao F. Predicting N2 lymph node metastasis in presurgical stage I-II non-small cell lung cancer using multiview radiomics and deep learning method. Med Phys 2023;50:2049-60. [Crossref] [PubMed]
- Wang M, Liu L, Dai Q, Jin M, Huang G. Developing a primary tumor and lymph node 18F-FDG PET/CT-clinical (TLPC) model to predict lymph node metastasis of resectable T2-4 NSCLC. J Cancer Res Clin Oncol 2023;149:247-61. [Crossref] [PubMed]
- Hu D, Li S, Wu N, Lu X. A Multi-modal Heterogeneous Graph Forest to Predict Lymph Node Metastasis of Non-small Cell Lung Cancer. IEEE J Biomed Health Inform 2023; Epub ahead of print. [Crossref]
- Yin G, Song Y, Li X, Zhu L, Su Q, Dai D, Xu W. Prediction of mediastinal lymph node metastasis based on (18)F-FDG PET/CT imaging using support vector machine in non-small cell lung cancer. Eur Radiol 2021;31:3983-92. [Crossref] [PubMed]
- Zhao X, Wang X, Xia W, Zhang R, Jian J, Zhang J, Zhu Y, Tang Y, Li Z, Liu S, Gao X. 3D multi-scale, multi-task, and multi-label deep learning for prediction of lymph node metastasis in T1 lung adenocarcinoma patients’ CT images. Comput Med Imaging Graph 2021;93:101987. [Crossref] [PubMed]
- Geng M, Geng M, Wei R, Chen M. Artificial intelligence neural network analysis and application of CT imaging features to predict lymph node metastasis in non-small cell lung cancer. J Thorac Dis 2022;14:4384-94. [Crossref] [PubMed]
- Zhao X, Wang X, Xia W, Li Q, Zhou L, Li Q, Zhang R, Cai J, Jian J, Fan L, Wang W, Bai H, Li Z, Xiao Y, Tang Y, Gao X, Liu S. A cross-modal 3D deep learning for accurate lymph node metastasis prediction in clinical stage T1 lung adenocarcinoma. Lung Cancer 2020;145:10-7. [Crossref] [PubMed]
- Wu Y, Liu J, Han C, Liu X, Chong Y, Wang Z, Gong L, Zhang J, Gao X, Guo C, Liang N, Li S. Preoperative Prediction of Lymph Node Metastasis in Patients With Early-T-Stage Non-small Cell Lung Cancer by Machine Learning Algorithms. Front Oncol 2020;10:743. [Crossref] [PubMed]
- Salameh JP, Bossuyt PM, McGrath TA, Thombs BD, Hyde CJ, Macaskill P, et al. Preferred reporting items for systematic review and meta-analysis of diagnostic test accuracy studies (PRISMA-DTA): explanation, elaboration, and checklist. BMJ 2020;370:m2632. [Crossref] [PubMed]
- Whiting PF, Rutjes AW, Westwood ME, Mallett S, Deeks JJ, Reitsma JB, Leeflang MM, Sterne JA, Bossuyt PM. QUADAS-2 Group. QUADAS-2: a revised tool for the quality assessment of diagnostic accuracy studies. Ann Intern Med 2011;155:529-36. [Crossref] [PubMed]
- Caraguel CG, Vanderstichel R. The two-step Fagan’s nomogram: ad hoc interpretation of a diagnostic test result without calculation. Evid Based Med 2013;18:125-8. [Crossref] [PubMed]
- Prenzel KL, Mönig SP, Sinning JM, Baldus SE, Brochhagen HG, Schneider PM, Hölscher AH. Lymph node size and metastatic infiltration in non-small cell lung cancer. Chest 2003;123:463-7. [Crossref] [PubMed]
- Wei SH, Zhang JM, Shi B, Gao F, Zhang ZX, Qian LT. The value of CT radiomics features to predict visceral pleural invasion in ≤3 cm peripheral type early non-small cell lung cancer. J Xray Sci Technol 2022;30:1115-26. [Crossref] [PubMed]
- Xie P, Zhang Y, He L. Prognostic Evaluation of CT Imaging Big Data-Assisted Arterial Chemoembolization Combined with (125)I Seed Implantation for Non-Small-Cell Lung Cancer. Comput Math Methods Med 2022;2022:3472982. [Crossref] [PubMed]
- Hoeijmakers F, Heineman DJ, Daniels JM, Beck N, Tollenaar RAEM, Wouters MWJM, Marang-van de Mheen PJ, Schreurs WHMDT Study Group. Variation Between Multidisciplinary Tumor Boards in Clinical Staging and Treatment Recommendations for Patients With Locally Advanced Non-small Cell Lung Cancer. Chest 2020;158:2675-87. [Crossref] [PubMed]
- Lee SW, Kim SJ. Is Delayed Image of 18F-FDG PET/CT Necessary for Mediastinal Lymph Node Staging in Non-Small Cell Lung Cancer Patients? Clin Nucl Med 2022;47:414-21. [Crossref] [PubMed]
- Seol HY, Kim YS, Kim SJ. Predictive Value of 18F-Fluorodeoxyglucose Positron Emission Tomography or Positron Emission Tomography/Computed Tomography for Assessment of Occult Lymph Node Metastasis in Non-Small Cell Lung Cancer. Oncology 2021;99:96-104.
- van Elmpt W, Landry G, Das M, Verhaegen F. Dual energy CT in radiotherapy: Current applications and future outlook. Radiother Oncol 2016;119:137-44. [Crossref] [PubMed]
- Yeh BM, FitzGerald PF, Edic PM, Lambert JW, Colborn RE, Marino ME, Evans PM, Roberts JC, Wang ZJ, Wong MJ, Bonitatibus PJ Jr. Opportunities for new CT contrast agents to maximize the diagnostic potential of emerging spectral CT technologies. Adv Drug Deliv Rev 2017;113:201-22. [Crossref] [PubMed]
- Wang T, Fan Z, Zou L, Hou Y. Can quantitative parameters of spectral computed tomography predict lymphatic metastasis in lung cancer? a systematic review and meta-analysis. Radiother Oncol 2023;183:109643. [Crossref] [PubMed]
- Liu CJ, Zhang L, Sun Y, Geng L, Wang R, Shi KM, Wan JX. Application of CT and MRI images based on an artificial intelligence algorithm for predicting lymph node metastasis in breast cancer patients: a meta-analysis. BMC Cancer 2023;23:1134. [Crossref] [PubMed]
- Bedrikovetski S, Dudi-Venkata NN, Kroon HM, Seow W, Vather R, Carneiro G, Moore JW, Sammour T. Artificial intelligence for pre-operative lymph node staging in colorectal cancer: a systematic review and meta-analysis. BMC Cancer 2021;21:1058. [Crossref] [PubMed]
- Stark GF, Hart GR, Nartowt BJ, Deng J. Predicting breast cancer risk using personal health data and machine learning models. PLoS One 2019;14:e0226765. [Crossref] [PubMed]
- Hakkak Moghadam Torbati A, Pellegrino S, Fonti R, Morra R, De Placido S, Del Vecchio S. Machine Learning and Texture Analysis of [18F]FDG PET/CT Images for the Prediction of Distant Metastases in Non-Small-Cell Lung Cancer Patients. Biomedicines 2024;12:472.
- Breiman L. Random Forests. Machine Learning 2001;45:5-32. [Crossref] [PubMed]
- Karabacak M, Ozkara BB, Mordag S, Bisdas S. Deep learning for prediction of isocitrate dehydrogenase mutation in gliomas: a critical approach, systematic review and meta-analysis of the diagnostic test performance using a Bayesian approach. Quant Imaging Med Surg 2022;12:4033-46. [Crossref] [PubMed]
- Aggarwal R, Sounderajah V, Martin G, Ting DSW, Karthikesalingam A, King D, Ashrafian H, Darzi A. Diagnostic accuracy of deep learning in medical imaging: a systematic review and meta-analysis. NPJ Digit Med 2021;4:65. [Crossref] [PubMed]
- Decharatanachart P, Chaiteerakij R, Tiyarattanachai T, Treeprasertsuk S. Application of artificial intelligence in chronic liver diseases: a systematic review and meta-analysis. BMC Gastroenterol 2021;21:10. [Crossref] [PubMed]
- Kim HY, Cho SJ, Sunwoo L, Baik SH, Bae YJ, Choi BS, Jung C, Kim JH. Classification of true progression after radiotherapy of brain metastasis on MRI using artificial intelligence: a systematic review and meta-analysis. Neurooncol Adv 2021;3:vdab080. [Crossref] [PubMed]