Comparison of single shot and multishot diffusion-weighted imaging in 5-T magnetic resonance imaging for brain disease diagnosis
Introduction
Brain diseases are one of the most common causes of death in humans, and cerebrovascular disease ranks the third leading cause of death among Chinese residents (1). Other brain diseases, such as intracranial tumors, brain abscesses, leukoencephalopathy, brain infectious lesions, can also cause serious damage to the brain. Early diagnosis of these diseases has a substantial impact on prognosis and treatment. Magnetic resonance imaging (MRI) is a noninvasive technique that can provide detailed information of brain anatomy and functions. Distinct from conventional structural MRI, diffusion-weighted imaging (DWI) plays an important role in the early detection of a variety of brain diseases, such as acute cerebral infarction (ACI), brain abscess, and intracranial tumors (2,3). Moreover, DWI can obtain critical quantitative measurements such as apparent diffusion coefficient (ADC) in various other brain pathologies, which can help to establish a specific diagnosis and management approach for central nervous system disorders (4). Based on the mechanism of the random Brownian motion of water molecules, DWI provides qualitative and quantitative information of the water in biological tissues (5). In traditional methods, DWI uses a single-shot echo-planar imaging (ssEPI) sequence for data collection. In an ssEPI sequence, all lines of the k-space are acquired within one excitation, which has a high sampling efficacy and is insensitive to motion. However, ssEPI is susceptible to B0 inhomogeneity and eddy currents, which can cause severe distortions and susceptibility artifacts (6). Furthermore, due to the long echo train, the signal intensity across the readout direction of the k-space varies greatly and is weaker at the beginning and the end; thus, images suffer from T2* blurring (7,8). Multishot echo-planar imaging (msEPI) has been designed to address the shortcomings of ssEPI (9). The msEPI technique collects k-space data in multiple excitations, which reduces distortion and blurring due to the short effective echo spacing and echo train length. The msEPI technique is sensitive to patient motion during the implementation of diffusion encoding gradients between shots, resulting in phase errors that can lead to severe artifacts (10,11). To mitigate these motion effects, an additional navigator is typically added at the end of data acquisition in the msEPI sequence.
Previous studies have shown that at 3 T, the image distortion is greatly improved by msEPI DWI as compared to ssEPI DWI (12-14). With the emergence of ultrahigh magnetic field strength MRI systems, the image quality of DWI has been further improved. A comparative study was conducted on breast DWI at 7 and 3 T, and it found that using higher spatial resolution in msEPI DWI at 7 T is feasible (15). Another study reported that compared to ssEPI at 7 T, msEPI reduced image blurring and distortions while improving the subjective image quality (16). Other research has demonstrated that an msEPI sequence with two-dimensional (2D) navigator correction at 7 T—whether it is readout- or phase-segmented—can provide substantial improvement in image quality in terms through reducing distortions and artifacts (17,18). However, the application of 7-T MRI in clinical settings is still limited due to field inhomogeneity in body scans and the high cost of scanners. Moreover, under ultrahigh magnetic field strength, the specific absorption rate (SAR) in the body increases (19), which may cause physical discomfort. Recently, the ability of 5-T MRI to provide neuroanatomical details with similar image quality to that of 7-T MRI has been demonstrated (20). Furthermore, 5-T MRI produces less radio frequency inhomogeneity than does 7 T (21). Under an intermediate field strength, a 5-T scanner can be used to image the entire body with good visualization and maintain a clinically acceptable SAR. Although a few studies have been conducted regarding the advantages of msEPI DWI at 3 T and 7 T, its performance at 5 T has not been investigated thus far.
This study thus compared the subjective image quality and quantitative indices of ssEPI DWI and msEPI DWI in a wide spectrum of brain lesions at 5 T and assessed the feasibility of msEPI in detecting specific diffusion abnormalities. We present this article in accordance with the STARD reporting checklist (available at https://qims.amegroups.com/article/view/10.21037/qims-24-118/rc).
Methods
Participants
This study retrospectively reviewed the records of consecutive patients with suspected brain diseases who underwent 5-T magnetic resonance examination at the First Affiliated Hospital of University of Science and Technology of China from August 2023 to September 2023. The inclusion criteria were completion of (I) T2-weighted fluid-attenuated inversion recovery (T2-FLAIR), ssEPI, and msEPI DWI scans in the same session and (II) no history of cranial surgery. Meanwhile, the exclusion criterion was severe motion artifacts in the scanned image. Ultimately, 107 patients (49.1±16.7 years; 55 males and 52 females) were enrolled, with a total of 43 obvious lesions being observed on MRI among 42 of these patients (Figure S1). Brain lesions were subsequently diagnosed by histological biopsy or MRI findings with reference to clinical history, with the distribution of diagnoses being as follows: 8 ACI lesions, 5 chronic cerebral infarction lesions, 12 low-grade gliomas (LGGs), 5 high-grade gliomas, 5 meningiomas, 2 brain abscesses, 2 cases of metastases, 1 central nervous system cell tumor, 1 case of intracranial infection, 1 solitary fibroma, and 1 case of hematoma resorption. The remaining 65 patients had no obvious lesions on brain MRI. This study was conducted in accordance with the Declaration of Helsinki (as revised in 2013) and was approved by the Ethics Review Committee of the First Affiliated Hospital of University of Science and Technology of China (approval No. 2023-RE-319). All patients were compliant with the MRI scans and signed an informed consent form prior to examination.
Data acquisition and sequence details
All head MRI examinations were performed on a 5-T scanner (uMR Jupiter, United Imaging Healthcare, Shanghai, China) with a maximum gradient strength of 120 mT/m and gradient slew rate of 200 T/m/s. For signal reception, a commercial dual-channel transmission and 48-channel receive head volume coil (United Imaging Healthcare) were applied. Scan sequences included axial T2-FLAIR, conventional ssEPI DWI, and msEPI DWI (number of excitations =5). For both DWI sequences, two b values were applied (0 and 1,000 s/mm2), and the ADC map was automatically generated on the workstation (uWS-MR, United Imaging Healthcare). The scanning parameters are shown in Table 1. It should be noted that the same bandwidth in the phase-encoding direction was applied for ssEPI and msEPI DWI. However, in msEPI, as each segment is sampled with a uniform sampling interval, Δky, in the phase-encoding direction in an interleaved manner, the effective Δky should be divided by the number of shots. Therefore, the effective bandwidth in the phase-encoding direction of msEPI is five times that of ssEPI, and thus the effective echo spacing is shorter in msEPI. As the echo spacing is larger than the dwell time, the distortion is particularly severe along the phase-encoding direction (22). When the effective echo spacing decreases, the distortion is reduced.
Table 1
MRI parameter | ssEPI DWI | msEPI DWI | T2-FLAIR |
---|---|---|---|
FOV (phase × readout) (mm2) | 220×230 | 220×230 | 220×230 |
Number of slices | 20 | 20 | 20 |
Slice thickness/gap (mm) | 5/1.5 | 5/1.5 | 5/1.5 |
TR/TE (ms) | 3,609/75.6 | 3,700/73.0 | 9,000/134.6 |
Matrix size | 245×256 | 245×256 | 245×256 |
Acquired voxel size (mm3) | 0.9×0.9×5 | 0.9×0.9×5 | 0.9×0.9×5 |
Reconstruction voxel size (mm3) | 0.45×0.45×5 | 0.45×0.45×5 | 0.45×0.45×5 |
Number of shots | 1 | 5 | n.a. |
Echo train length | 92 | 49 | 37 |
PI acceleration factor | 2 | 1 | 2 |
Partial Fourier factor | 75% | 100% | 100% |
B values [averages] (s/mm2) | 0 [4], 1,000 [10] | 0 [1], 1,000 [2] | n.a. |
Number of diffusion directions | 3 | 3 | n.a. |
Bandwidth in the phase-encoding direction (Hz/pixel) | 1,500 | 1,500 | 260 |
2D navigator TE (ms) | n.a. | 137.5 | n.a. |
2D navigator FOV (mm2) | n.a. | 220×230 | n.a. |
2D navigator resolution (mm2) | n.a. | 6.9×7.2 | n.a. |
2D navigator matrix (for k-space center sampling) | n.a. | 32×32 | n.a. |
Acquisition time | 2 min 18 s | 2 min 26 s | 1 min 48 s |
ssEPI, single-shot echo-planar imaging; DWI, diffusion-weighted imaging; msEPI, multishot echo-planar imaging; T2-FLAIR, T2-weighted fluid-attenuated inversion recovery; MRI, magnetic resonance imaging; FOV, field of view; TR, repetition time; TE, echo time; PI, parallel imaging; 2D, two-dimensional; n.a., not applicable.
In this study, we used a phase-segmented msEPI sequence with a 2D navigator echo for the shot-to-shot motion correction. The k-space was divided into five segments along the phase-encoding direction in an interleaved manner, and each segment was sampled following an excitation. The diffusion preparation was composed of three gradients, with two applied in opposite directions on one side of the 180° pulse, and a third applied on the other side (Figure 1). In this manner, the rephasing gradient was split into two lobes, and thus the duration of the third diffusion gradient was reduced to yield shorter echo times (TEs) for high-resolution imaging. Moreover, the duration of the diffusion gradients could be adjusted to null the eddy current (23). After the diffusion preparation, the imaging data were collected, and an additional 2D navigator echo recorded the varying phase. The image field of view (FOV) of the navigator was identical to the image FOV for imaging while the navigator covered the k-space center with a matrix size of 32×32. For each shot, navigator data were zero-padded in the k-space to the same size as imaging matrix and Fourier-transformed to the image domain. For the imaging data, a parallel imaging (PI) technique was applied to reconstruct the image. Subsequently, the image from each shot was phase-corrected with the built-in algorithm in the scanner, which was similar to that described previously (24). After phase correction, images from each shot were transformed to the frequency domain, combined to form a fully sampled k-space, and reconstructed to artifact-free diffusion-weighted images.
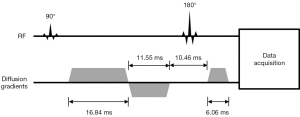
Image analysis
Qualitative analysis
Two observers (associate chief physicians with 6- and 5-year work experience in MRI, respectively) who were blind to patients’ information and diagnosis graded ssEPI and msEPI DWI image quality according to a four-point scale (Table 2) (25). Each observer assessed the whole image set including all slices and both b-0 and b-1,000 s/mm2 images for each patient. The two observers were blind to the type of DWI sequence, and ssEPI and msEPI DWI images were organized in a random order. A total of four aspects were evaluated: anatomical distortion, edge sharpness, susceptibility artifact, and overall image quality.
Table 2
Score | Anatomical distortion | Edge clarity | Susceptibility artifact | Overall image quality |
---|---|---|---|---|
1 | Serious | Very poor | Serious | Bad |
2 | Moderate | Poor | Moderate | Fair |
3 | Mild | Moderate | Mild | Good |
4 | None | Good | None | Excellent |
Quantitative analysis
All quantitative analyses were conducted on a workstation (uMR-WS) by two radiologists (associate chief physicians with 6 and 5 years of work experience in MRI, respectively) who reached a consensus.
- For each enrolled patient, T2-FLAIR image was considered the gold standard for brain structural reference. We used the same matrix size, voxel size, and FOV for both DWI sequences and T2-FLAIR. During the examination, the T2-FLAIR was scanned first, and the location of the FOV of T2-FLAIR was copied to and applied for both DWI sequences. Distortions were quantified through measurement of the displacement between DWI b-1,000 s/mm2 and T2-FLAIR at specific brain regions and assessment of brainstem diameter differences (25,26). In EPI images, distortions are caused by magnetic field inhomogeneity (22). At air-tissue interfaces, magnetic field inhomogeneity is more prevalent and introduces heavier geometric distortions. Thus, we examined the peak distortion displacement in the prefrontal lobe, anterior temporal lobe, posterior temporal lobe, brain diameter, and brainstem diameter (Figure 2). A line aligned with the brain structure was drawn as the reference line on the T2-FLAIR image and copied to the same site on the DWI image; next, on the DWI image, the peak displacement between the distorted brain structure and the reference line was measured. Brain parenchyma and brainstem diameters along the anterior-posterior direction were measured, and the differences between DWI and T2-FLAIR were calculated.
- For the 42 patients (43 lesions) diagnosed with brain disease, signal-to-noise ratio (SNR) of the lesion, SNR of the normal tissue, and contrast-to-noise ratio (CNR) were calculated. Ellipse-shaped regions of interest (ROIs) with areas of 5–10 mm2 were placed on the lesion and contralateral healthy white matter and drawn synchronously on DWI b-1,000 s/mm2 images and ADC maps (Figure 3). The mean signal intensity, the standard deviation (SD) of the signal, and the mean ADC value of the ROI were quantified automatically on the workstation.
The SNR and CNR were calculated by the following formulas (27): - To further evaluate the diagnostic performance of ssEPI and msEPI, patients with LGG (n=12) and patients with ACI (n=8) were examined. The ability of ADC values derived from ssEPI and msEPI to distinguish lesions from contralateral normal tissues was evaluated.
- To further evaluate the SNR and ADC at different brain regions, 20 participants were randomly selected for measurement. On ssEPI and msEPI DWI b-1,000 s/mm2 images and ADC maps, ellipse-shaped ROIs with as large as possible areas were placed at the thalamus and corpus callosum. The SNR was calculated according to
Eq. [2], and the mean ADC values within the ROI were recorded.
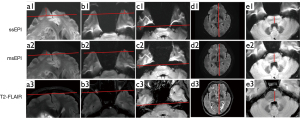
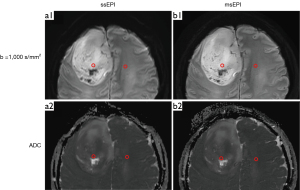
Statistical analysis
A normality distribution test was performed on image quality scores (Table S1). The Wilcoxon test was used to compare image quality scores, and the interclass correlation coefficient (ICC) was used to assess the consistency of the scores between the two readers (ICC <0.20 indicates poor consistency; 0.20–0.39, fair consistency; 0.40–0.59, moderate consistency; 0.60–0.79, strong consistency; and 0.80–1.0, very strong consistency) (28). The paired t-test was used to compare the differences in distortion length, SNR, CNR, and ADC values between ssEPI and msEPI as well as the ADC values between lesions and contralateral healthy white matter. Pearson correlation coefficients and Bland-Altman analysis were used to assess the correlation of ADC values from the two DWI sequences. The receiver operating characteristic (ROC) curve was plotted and the area under the curve (AUC) was calculated to analyze the diagnostic efficacy of the ADC value in differentiating LGGs from their contralateral normal tissues. The DeLong test was performed to compare the AUCs of ssEPI and msEPI DWI. A two-sided P value <0.05 was considered statistically significant.
Results
Qualitative analysis
Image quality scores of msEPI were significantly higher than those of ssEPI in terms of anatomical distortion, edge clarity, susceptibility artifacts, and overall image quality for both readers (two-sided P<0.001) (Table 3). Representative DWI images of typical patients are displayed in Figures 4-6. The image quality evaluations from the two readers were found to have moderate-to-strong consistency for ssEPI (ICCs ranging from 0.478 to 0.882; two-sided P<0.001) and very strong consistency for msEPI (ICCs ranging from 0.947 to 1; two-sided P<0.001) (Table 3).
Table 3
Grading index | Anatomical distortion | Susceptibility artifact | Edge clarity | Overall image quality |
---|---|---|---|---|
Image quality score comparison | ||||
Reader 1 | ||||
ssEPI | 1.94±0.30 | 2.50±0.50 | 2.06±0.23 | 2.02±0.14 |
msEPI | 3.36±0.48 | 3.00±0.00 | 3.42±0.50 | 3.48±0.50 |
Z | −9.241 | −7.28 | −9.119 | −9.276 |
P value | <0.001 | <0.001 | <0.001 | <0.001 |
Reader 2 | ||||
ssEPI | 1.90±0.36 | 2.38±0.51 | 2.02±0.14 | 2.01±0.10 |
msEPI | 3.37±0.49 | 3.00±0.00 | 3.42±0.50 | 3.46±0.50 |
Z | −9.214 | −8.004 | −9.201 | −9.279 |
P value | <0.001 | <0.001 | <0.001 | <0.001 |
Interobserver agreement | ||||
ssEPI | ||||
ICC | 0.478 | 0.882 | 0.662 | 0.798 |
P value | <0.001 | <0.001 | <0.001 | <0.001 |
msEPI | ||||
ICC | 0.947 | 1.000 | 0.960 | 0.961 |
P value | <0.001 | n.a. | <0.001 | <0.001 |
Data are presented as the mean ± SD. ssEPI, single-shot echo-planar imaging; DWI, diffusion-weighted imaging; msEPI, multishot echo-planar imaging; ICC, interclass correlation coefficient; n.a., not applicable because the rating scores were 3 for all the participants for both readers; SD, standard deviation.
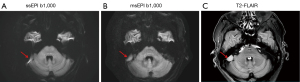
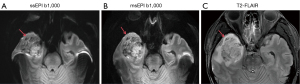
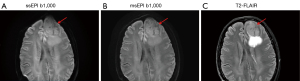
Distortion displacement
The peak distortion displacements of msEPI in the prefrontal lobe, anterior temporal lobe, posterior temporal lobe, brain diameter, and brainstem diameter were significantly lower than those of ssEPI (two-sided P values <0.001) (Table 4).
Table 4
Brain lobes | ssEPI | msEPI | P value |
---|---|---|---|
Frontal lobe | 15.4±2.3 | 6.1±1.3 | <0.001 |
Anterior temporal lobe | 7.2±1.9 | 4.2±0.9 | <0.001 |
Posterior temporal lobe | 8.4±2.1 | 4.9±1.5 | <0.001 |
Brain diameter | 12.6±3.3 | 4.2±1.5 | <0.001 |
Brainstem diameter | 1.9±1.5 | 0.8±0.6 | <0.001 |
Data are presented as means ± SD. ssEPI, single-shot echo-planar imaging; DWI, diffusion-weighted imaging; msEPI, multishot echo-planar imaging; SD, standard deviation.
Comparisons of SNR and CNR
In the analysis of 43 lesions in 42 patients with a wide spectrum of brain diseases, there were no significant differences between msEPI and ssEPI in terms of lesion SNR (two-sided P=0.848), normal-appearing white matter SNR (two-sided P=0.601), or CNR (two-sided P=0.638) (Figure 7, Table S2).
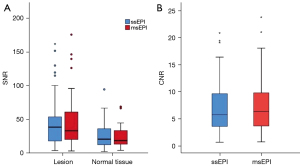
Comparison of ADC values
There was no significant difference in ADC values between ssEPI and msEPI in lesions (two-sided P=0.216) or normal white tissues (two-sided P=0.408) (Table 5). A strong correlation was found between ADC results from ssEPI and msEPI for both lesions (r=0.97; two-sided P<0.001) and contralateral normal tissues (r=0.91; two-sided P<0.001) (Figure 8). In the Bland-Altman plots, most data lay within the mean difference ±1.96 SD (Figure 9).
Table 5
Tissue type | ssEPI | msEPI | P value |
---|---|---|---|
Lesion ADC (×10−6 mm2/s) | 1,036.9±542.0 | 1,066.6±602.2 | 0.216 |
Normal tissue ADC (×10−6 mm2/s) | 877.3±216.7 | 898.8±340.0 | 0.408 |
Data are presented as the mean ± SD. ADC, apparent diffusion coefficient; ssEPI, single-shot echo-planar imaging; msEPI, multishot echo-planar imaging; DWI, diffusion-weighted imaging; SD, standard deviation.
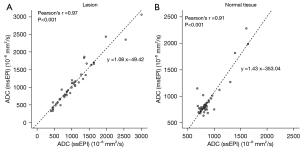
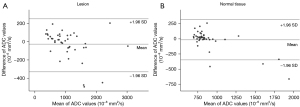
Diagnostic performance
In the analysis of 12 LGG and 8 ACI lesions, the ADC values of LGGs were significantly higher than those of contralateral normal tissues (ssEPI: two-sided P=0.003; msEPI: two-sided P=0.001), while there was a significant ADC decrease for ACI lesions as compared to contralateral white matter for both ssEPI and msEPI (ssEPI: two-sided P<0.001; msEPI: two-sided P<0.001) (Table 6).
Table 6
Sequence | Low-grade glioma | Acute cerebral infarction | |||||
---|---|---|---|---|---|---|---|
Lesion ADC (×10−6 mm2/s) |
Normal tissue ADC (×10−6 mm2/s) |
P value | Lesion ADC (×10−6 mm2/s) |
Normal tissue ADC (×10−6 mm2/s) |
P value | ||
ssEPI | 1,119.9±273.1 | 805.1±73.9 | 0.003 | 603.9±273.2 | 888.9±212.0 | <0.001 | |
msEPI | 1,196.2±355.6 | 757.3±98.0 | 0.001 | 538.0±281.2 | 905.0±188.9 | <0.001 |
Data are presented as the mean ± SD. ADC, apparent diffusion coefficient; ssEPI, single-shot echo-planar imaging; msEPI, multishot echo-planar imaging; DWI, diffusion-weighted imaging; SD, standard deviation.
In the ROC analysis for the 12 LGG lesions, the AUC was 0.934 for ssEPI [95% confidence interval (CI): 0.84–1.00; two-sided P<0.001] and 0.944 for msEPI (95% CI 0.86–1.00; two-sided P<0.001) (Figure 10). The DeLong test showed there was no significant difference in the AUCs between ssEPI and msEPI DWI (two-sided P=0.833). The ADC cutoff with highest Youden index in ssEPI was 841.9×10−6 mm2/s, with a sensitivity of 91.7% and a specificity of 83.3%, while the ADC cutoff in msEPI was 819.0×10−6 mm2/s, with a sensitivity of 91.7% and a specificity of 91.7%.
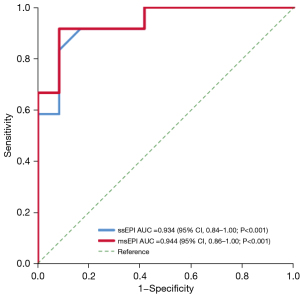
SNR and ADC values of thalamus and corpus callosum
In the analysis of 20 randomly selected participants, there was no significant difference between ssEPI and msEPI DWI in terms of SNR for the thalamus (two-sided P=0.329) and corpus callosum (two-sided P=0.532) (Table S3). There was also no significant difference between ssEPI and msEPI DWI in terms of ADC values for the thalamus (two-sided P=0.590) and corpus callosum (two-sided P=0.922) (Table S4).
Discussion
In this study, we analyzed brain images of 107 patients obtained with a 5-T ultra-high magnetic field using ssEPI and msEPI sequences. In the qualitative assessment, our study showed that subjective scores for msEPI were significantly higher than those of ssEPI in terms of anatomical distortion, edge clarity, susceptibility artifacts, and overall image quality. Moreover, the quantitative evaluation results showed that the msEPI significantly improved image distortion. msEPI reduced image distortion and allowed higher spatial resolution compared to ssEPI sequences, which is consistent with previous studies of MRI of the brain at 3 T (12,14,29,30).
Due to ssEPI typically producing large echo spacing in the phase-encoding direction, the distortion of images with EPI acquisition is inevitable. Geometric distortions may occur with extreme susceptibility variations along the phase-encoding direction at locations such as tissue-air interfaces. In addition, ssEPI has a longer readout duration compared to that of free induction decay, inducing image blurring and limiting spatial resolution (11,31). In our study, we used a phase-segmented msEPI sequence that collects k-space data with multiple excitations, which decreases effective echo spacing and echo train length. Thus, the accumulation of signals along the phase-encoding direction, distortions, and blurring are reduced. To overcome the motion-induced phase errors from shot to shot, an additional navigator is commonly used in msEPI (32,33). In our study, the 2D navigator acquired a second echo train after the acquisition of the image data, minimizing artifacts and thus improving image quality.
The diagnosis, progression management, and treatment of brain diseases benefit from early detection and accurate segmentation of diffusion abnormalities. With the development of deep learning (DL), several neural network models have emerged for brain segmentation such as VoxResNet, RAAGR2-Net, M-net, Bu-net, Mindcontrol, and Transbts (34-38). Theses automated segmentation models are more efficient than are manual segmentation and well-adapted to large-scale studies. Nevertheless, segmenting DWI images still faces certain challenges. Conventional ssEPI DWI is affected by image distortions due to field inhomogeneity, which introduces segmentation errors. Our study found that distortions were alleviated in msEPI DWI as compared to ssEPI DWI, which suggests msEPI is a promising tool for facilitating the segmentation of diffusion abnormalities with DL networks.
In addition to msEPI, which acquires different subsets of k-space data in a consistent phase-encoding direction, other acquisition techniques are also helpful for distortion correction. For example, blip-up blip-down acquisition (39) is a widely used technique both in ssEPI and msEPI DWI. The blip-up and blip-down method applies two acquisitions with opposite phase-encoding directions and estimates the field inhomogeneity through an iterative algorithm. In the joint reconstruction of two acquisitions, the field map is translated into the displacement map, and the displacement map is used to correct images. The differences between two images are calculated to estimate a more accurate field map until the sum of squared differences between two acquisitions reaches a minimum. This method achieves distortion-free imaging without substantially extending the scanning time. Therefore, the combination of msEPI and the blip-up blip-down acquisition technique may help further reduce image distortions in DWI.
In the analyses of SNR and CNR, considering the lesion heterogeneity, we used the SD of the signal intensity in the normal-appearing tissue ROI to represent the noise. To avoid including different tissues (e.g., white matter and gray matter), we used small-size ROIs (26,40). If large-size ROIs had been applied, different tissues would have introduced higher signal intensity variance and might have affected the accuracy of the calculation of SNR. There was no significant difference in SNR and CNR between the msEPI and ssEPI sequences. In previous studies, the SNR comparison results between msEPI and ssEPI images were not consistent. For instance, Morelli et al. compared ssEPI and msEPI in brain DWI and found that the SNR of msEPI was lower than that of ssEPI (41). However, the results obtained by Byeon et al. showed no significant difference between ssEPI and msEPI DWI (42). This may because there are many parameters that affect the SNR, such as the reconstruction filters applied, the TE and repetition time (TR), patient movement, partial volume effects, and magnetic field strength. The scanning scheme under which both the ssEPI and msEPI sequences were acquired with a clinically durable time (about 2 min 20 sec) in this study, parameters were fine-tuned and optimized based on the clinical performance. The same resolution and k-space filter, along with similar TR/TE and scanning durations, was applied to ssEPI and msEPI DWI, while the acceleration and the number of averages were different. In ssEPI DWI, PI acceleration and partial Fourier techniques were employed, which impaired the SNR and CNR; however, the number of averages was higher in ssEPI DWI than in msEPI for both b values and could compensate for the issues in SNR and CNR. Thus, this may be the possible reason that no significant difference between msEPI and ssEPI sequences in terms of SNR and CNR was found.
We further discovered that ADC values derived from ssEPI and msEPI were strongly correlated and showed no significant difference. The ADC values of LGG lesions were significantly higher than those of contralateral normal tissues, while the ADC values of ACI lesions were significantly lower than those of contralateral normal tissues. For ACI, the ADC value decreases due to intracranial cytotoxic edema narrowing the intercellular space and restricting the movement of water molecules (43). However, the necrotic cystic transformation area of the LGG is dominated by serous necrosis, and its viscosity is relatively reduced, thus increasing the ADC value (44).
msEPI has been applied to the brain with good results at lower magnetic fields (13,15). This suggests that msEPI DWI is conducive to the diagnosis of craniocerebral diseases. In addition, the diagnostic efficacy analysis in this study suggests that msEPI has a competitive diagnostic performance for LGG detection relative to ssEPI, which further confirms that msEPI DWI is a promising alternative to ssEPI DWI for the diagnosis of brain tumors especially at ultrahigh fields such as 5 T.
There are several limitations to this study that should be addressed. First, only a small number of patients for each specific brain disease were included, and only patients with brain lesions instead of all enrolled participants were analyzed in the SNR comparison. Moreover, the ROC analysis included only 12 cases of LGG, and the ability of ADC values to detect other brain diseases was not examined. A greater number patients with different brain diseases should be included in future studies to further verify the conclusion that msEPI is superior to ssEPI in the diagnosis of various brain lesions. Second, the distortion analysis was completed manually, and subjective errors were inevitable. Distortion is nonuniform across the image plane and slices; however, only the peak distortion at specific brain regions was measured. An automatic method for measuring image distortion in different directions should be implemented in the future for a more objective and comprehensive quantification of EPI image qualities. Third, different imaging parameters used between ssEPI and msEPI might have influenced the assessment of SNR and CNR. The application of PI results in SNR loss, and the SNR of PI images is calculated by dividing the SNR of the unaccelerated image by the square root of the acceleration factor and the geometric factor (g factor). The g factor represents the spatial nonuniformity of the noise, which was difficult to quantify in this study. All participants in this study were patients, and the methods for evaluating the g factor, as discussed by Breuer et al., are not clinically practical due to extra noise-only scanning (45). The purpose of the SNR analysis in this study was to compare the SNR between ssEPI and msEPI at the same anatomical location. Therefore, we attempted to minimize the influence of the spatially nonuniform noise by placing ROIs at the same locations for both DWI sequences. In future research, phantoms or healthy volunteers should be involved to estimate the noise nonuniformity of the PI image. Additionally, the PI acceleration factor is two in ssEPI (vs. one in msEPI) and impairs SNR and CNR by a factor of , while the number of averages is higher in ssEPI and improves SNR and CNR. Moreover, there were slight variations in TR, TE, and the scanning duration between ssEPI and msEPI. Consistent imaging parameters should be used for ssEPI and msEPI in subsequent studies to enhance the credibility of the findings.
Conclusions
This is the first study to compare performance of ssEPI and msEPI in terms of image quality at 5 T. msEPI DWI significantly reduced image distortion as compared to ssEPI while producing relatively similar ADC values across a wide spectrum of brain diseases. These results indicate that msEPI is a valuable alternative to ssEPI DWI in the diagnosis of various brain diseases in an ultrahigh magnetic field.
Acknowledgments
We would like to thank all the participants who contributed to our research.
Funding: None.
Footnote
Reporting Checklist: The authors have completed the STARD reporting checklist. Available at https://qims.amegroups.com/article/view/10.21037/qims-24-118/rc
Conflicts of Interest: All authors have completed the ICMJE uniform disclosure form (available at https://qims.amegroups.com/article/view/10.21037/qims-24-118/coif). R.T. and X.S. were employees of United Imaging Healthcare throughout their involvement in the study. The other authors have no conflicts of interest to declare.
Ethical Statement: The authors are accountable for all aspects of the work in ensuring that questions related to the accuracy or integrity of any part of the work are appropriately investigated and resolved. This study was conducted in accordance with the Declaration of Helsinki (as revised in 2013) and was approved by the Ethics Review Committee of the First Affiliated Hospital of University of Science and Technology of China (approval No. 2023-RE-319). Informed consent was obtained from all participants involved in the study.
Open Access Statement: This is an Open Access article distributed in accordance with the Creative Commons Attribution-NonCommercial-NoDerivs 4.0 International License (CC BY-NC-ND 4.0), which permits the non-commercial replication and distribution of the article with the strict proviso that no changes or edits are made and the original work is properly cited (including links to both the formal publication through the relevant DOI and the license). See: https://creativecommons.org/licenses/by-nc-nd/4.0/.
References
- Report on Cardiovascular Health and Diseases in China 2021: An Updated Summary. Biomed Environ Sci 2022;35:573-603. [Crossref] [PubMed]
- Engelter ST, Wetzel SG, Bonati LH, Fluri F, Lyrer PA. The clinical significance of diffusion-weighted MR imaging in stroke and TIA patients. Swiss Med Wkly 2008;138:729-40. [Crossref] [PubMed]
- Dmytriw AA, Sawlani V, Shankar J. Diffusion-Weighted Imaging of the Brain: Beyond Stroke. Can Assoc Radiol J 2017;68:131-46. [Crossref] [PubMed]
- Gaddamanugu S, Shafaat O, Sotoudeh H, Sarrami AH, Rezaei A, Saadatpour Z, Singhal A. Clinical applications of diffusion-weighted sequence in brain imaging: beyond stroke. Neuroradiology 2022;64:15-30. [Crossref] [PubMed]
- Bley TA, Wieben O, Uhl M. Diffusion-weighted MR imaging in musculoskeletal radiology: applications in trauma, tumors, and inflammation. Magn Reson Imaging Clin N Am 2009;17:263-75. [Crossref] [PubMed]
- Le Bihan D, Poupon C, Amadon A, Lethimonnier F. Artifacts and pitfalls in diffusion MRI. J Magn Reson Imaging 2006;24:478-88. [Crossref] [PubMed]
- Holdsworth SJ, O'Halloran R, Setsompop K. The quest for high spatial resolution diffusion-weighted imaging of the human brain in vivo. NMR Biomed 2019;32:e4056. [Crossref] [PubMed]
- Ma L, Lian S, Liu H, Meng T, Zeng W, Zhong R, Zhong L, Xie C. Diagnostic performance of synthetic magnetic resonance imaging in the prognostic evaluation of rectal cancer. Quant Imaging Med Surg 2022;12:3580-91. [Crossref] [PubMed]
- Wu W, Miller KL. Image formation in diffusion MRI: A review of recent technical developments. J Magn Reson Imaging 2017;46:646-62. [Crossref] [PubMed]
- Khoo MM, Tyler PA, Saifuddin A, Padhani AR. Diffusion-weighted imaging (DWI) in musculoskeletal MRI: a critical review. Skeletal Radiol 2011;40:665-81. [Crossref] [PubMed]
- Bammer R. Basic principles of diffusion-weighted imaging. Eur J Radiol 2003;45:169-84. [Crossref] [PubMed]
- Johansson J, Lagerstrand K, Ivarsson L, Svensson PA, Hebelka H, Maier SE. Brain diffusion MRI with multiplexed sensitivity encoding for reduced distortion in a pediatric patient population. Magn Reson Imaging 2022;87:97-103. [Crossref] [PubMed]
- Konar AS, Fung M, Paudyal R, Oh JH, Mazaheri Y, Hatzoglou V, Shukla-Dave A. Diffusion-Weighted Echo Planar Imaging using MUltiplexed Sensitivity Encoding and Reverse Polarity Gradient in Head and Neck Cancer: An Initial Study. Tomography 2020;6:231-40. [Crossref] [PubMed]
- Chen X, Zhang Y, Cao Y, Sun R, Huang P, Xu Y, Wang W, Feng Q, Xiao J, Yi J, Li Y, Dai J. A feasible study on using multiplexed sensitivity-encoding to reduce geometric distortion in diffusion-weighted echo planar imaging. Magn Reson Imaging 2018;54:153-9. [Crossref] [PubMed]
- Gruber S, Minarikova L, Pinker K, Zaric O, Chmelik M, Strasser B, Baltzer P, Helbich T, Trattnig S, Bogner W. Diffusion-weighted imaging of breast tumours at 3 Tesla and 7 Tesla: a comparison. Eur Radiol 2016;26:1466-73. [Crossref] [PubMed]
- Bogner W, Pinker K, Zaric O, Baltzer P, Minarikova L, Porter D, Bago-Horvath Z, Dubsky P, Helbich TH, Trattnig S, Gruber S. Bilateral diffusion-weighted MR imaging of breast tumors with submillimeter resolution using readout-segmented echo-planar imaging at 7 T. Radiology 2015;274:74-84. [Crossref] [PubMed]
- Jeong HK, Gore JC, Anderson AW. High-resolution human diffusion tensor imaging using 2-D navigated multishot SENSE EPI at 7 T. Magn Reson Med 2013;69:793-802. [Crossref] [PubMed]
- Heidemann RM, Porter DA, Anwander A, Feiweier T, Heberlein K, Knösche TR, Turner R. Diffusion imaging in humans at 7T using readout-segmented EPI and GRAPPA. Magn Reson Med 2010;64:9-14. [Crossref] [PubMed]
- Fiedler TM, Ladd ME, Bitz AK. SAR Simulations & Safety. Neuroimage 2018;168:33-58. [Crossref] [PubMed]
- Shi Z, Zhao X, Zhu S, Miao X, Zhang Y, Han S, Wang B, Zhang B, Ye X, Dai Y, Chen C, Rao S, Lin J, Zeng M, Wang H. Time-of-Flight Intracranial MRA at 3 T versus 5 T versus 7 T: Visualization of Distal Small Cerebral Arteries. Radiology 2023;306:207-17. [Crossref] [PubMed]
- Wei Z, Chen Q, Han S, Zhang S, Zhang N, Zhang L, Wang H, He Q, Cao P, Zhang X, Liang D, Liu X, Li Y, Zheng H. 5T magnetic resonance imaging: radio frequency hardware and initial brain imaging. Quant Imaging Med Surg 2023;13:3222-40. [Crossref] [PubMed]
- Zeng H, Constable RT. Image distortion correction in EPI: comparison of field mapping with point spread function mapping. Magn Reson Med 2002;48:137-46. [Crossref] [PubMed]
- Finsterbusch J. Eddy-current compensated diffusion weighting with a single refocusing RF pulse. Magn Reson Med 2009;61:748-54. [Crossref] [PubMed]
- Atkinson D, Counsell S, Hajnal JV, Batchelor PG, Hill DL, Larkman DJ. Nonlinear phase correction of navigated multi-coil diffusion images. Magn Reson Med 2006;56:1135-9. [Crossref] [PubMed]
- Okuchi S, Fushimi Y, Yoshida K, Nakajima S, Sakata A, Hinoda T, Otani S, Sagawa H, Zhou K, Yamao Y, Okawa M, Nakamoto Y. Comparison of TGSE-BLADE DWI, RESOLVE DWI, and SS-EPI DWI in healthy volunteers and patients after cerebral aneurysm clipping. Sci Rep 2022;12:17689. [Crossref] [PubMed]
- Gumeler E, Parlak S, Yazici G, Karabulut E, Kiratli H, Oguz KK. Single shot echo planar imaging (ssEPI) vs single shot turbo spin echo (ssTSE) DWI of the orbit in patients with ocular melanoma. Br J Radiol 2021;94:20200825. [Crossref] [PubMed]
- Vranic JE, Cross NM, Wang Y, Hippe DS, de Weerdt E, Mossa-Basha M. Compressed Sensing-Sensitivity Encoding (CS-SENSE) Accelerated Brain Imaging: Reduced Scan Time without Reduced Image Quality. AJNR Am J Neuroradiol 2019;40:92-8. [Crossref] [PubMed]
- Donner A, Eliasziw M, Shoukri M. Review of inference procedures for the interclass correlation coefficient with emphasis on applications to family studies. Genet Epidemiol 1998;15:627-46. [Crossref] [PubMed]
- Holdsworth SJ, Yeom K, Skare S, Gentles AJ, Barnes PD, Bammer R. Clinical application of readout-segmented- echo-planar imaging for diffusion-weighted imaging in pediatric brain. AJNR Am J Neuroradiol 2011;32:1274-9. [Crossref] [PubMed]
- Yeom KW, Holdsworth SJ, Van AT, Iv M, Skare S, Lober RM, Bammer R. Comparison of readout-segmented echo-planar imaging (EPI) and single-shot EPI in clinical application of diffusion-weighted imaging of the pediatric brain. AJR Am J Roentgenol 2013;200:W437-43. [Crossref] [PubMed]
- Dietrich O, Biffar A, Baur-Melnyk A, Reiser MF. Technical aspects of MR diffusion imaging of the body. Eur J Radiol 2010;76:314-22. [Crossref] [PubMed]
- Ma X, Zhang Z, Dai E, Guo H. Improved multi-shot diffusion imaging using GRAPPA with a compact kernel. Neuroimage 2016;138:88-99. [Crossref] [PubMed]
- Liu W, Zhao X, Ma Y, Tang X, Gao JH. DWI using navigated interleaved multishot EPI with realigned GRAPPA reconstruction. Magn Reson Med 2016;75:280-6. [Crossref] [PubMed]
- Chen H, Dou Q, Yu L, Qin J, Heng PA. VoxResNet: Deep voxelwise residual networks for brain segmentation from 3D MR images. Neuroimage 2018;170:446-55. [Crossref] [PubMed]
- Rehman MU, Ryu J, Nizami IF, Chong KT. RAAGR2-Net: A brain tumor segmentation network using parallel processing of multiple spatial frames. Comput Biol Med 2023;152:106426. [Crossref] [PubMed]
- Mehta R, Sivaswamy J. M-net: A convolutional neural network for deep brain structure segmentation. 2017 IEEE 14th International Symposium on Biomedical Imaging (ISBI 2017), Melbourne, VIC, Australia, 2017:437-40.
- Keshavan A, Datta E. M McDonough I, Madan CR, Jordan K, Henry RG. Mindcontrol: A web application for brain segmentation quality control. Neuroimage 2018;170:365-72. [Crossref] [PubMed]
- Lin J, Lin J, Lu C, Chen H, Lin H, Zhao B, Shi Z, Qiu B, Pan X, Xu Z, Huang B, Liang C, Han G, Liu Z, Han C. CKD-TransBTS: Clinical Knowledge-Driven Hybrid Transformer With Modality-Correlated Cross-Attention for Brain Tumor Segmentation. IEEE Trans Med Imaging 2023;42:2451-61. [Crossref] [PubMed]
- Andersson JL, Skare S, Ashburner J. How to correct susceptibility distortions in spin-echo echo-planar images: application to diffusion tensor imaging. Neuroimage 2003;20:870-88. [Crossref] [PubMed]
- Sheng Y, Hong R, Sha Y, Zhang Z, Zhou K, Fu C. Performance of TGSE BLADE DWI compared with RESOLVE DWI in the diagnosis of cholesteatoma. BMC Med Imaging 2020;20:40. [Crossref] [PubMed]
- Morelli J, Porter D, Ai F, Gerdes C, Saettele M, Feiweier T, Padua A, Dix J, Marra M, Rangaswamy R, Runge V. Clinical evaluation of single-shot and readout-segmented diffusion-weighted imaging in stroke patients at 3 T. Acta Radiol 2013;54:299-306. [Crossref] [PubMed]
- Byeon J, Kim JY, Cho AH. Readout-segmented echo-planar imaging in diffusion-weighted MR imaging of acute infarction of the brainstem and posterior fossa: comparison of single-shot echo-planar diffusion-weighted sequences. Clin Imaging 2015;39:765-9. [Crossref] [PubMed]
- Jin D, Su X, Jin Y, Gu Y, Yang J, Wang Q, Wang Y, Shi D, Xu L. Diagnostic value of MRI perfusion-weighted imaging and diffusion-weighted imaging parameters in cerebral apoplexy. Am J Transl Res 2023;15:1097-106.
- Yanaka K, Shirai S, Kimura H, Kamezaki T, Matsumura A, Nose T. Clinical application of diffusion-weighted magnetic resonance imaging to intracranial disorders. Neurol Med Chir (Tokyo) 1995;35:648-54. [Crossref] [PubMed]
- Breuer FA, Kannengiesser SA, Blaimer M, Seiberlich N, Jakob PM, Griswold MA. General formulation for quantitative G-factor calculation in GRAPPA reconstructions. Magn Reson Med 2009;62:739-46. [Crossref] [PubMed]