The relationship between sarcopenia and metabolic parameters of 18F-fluorodeoxyglucose positron emission tomography/computed tomography (18F-FDG PET/CT) and the prognostic value of sarcopenia in early-stage non-small cell lung cancer
Introduction
Lung cancer stands as the most prevalent form of malignant tumor globally, with non-small cell lung cancer (NSCLC) emerging as the predominant pathological subtype, constituting approximately 80–85% of cases (1,2). Despite ongoing advancements in treatments and enhancements in patient care, the overall 5-year survival rate for lung cancer remains suboptimal at 15% (3). Even in cases of early-stage lung cancer, the 5-year survival rate post-surgery fails to surpass 75% (4). A more nuanced comprehension of prognostic factors can potentially unlock avenues for substantial improvements in survival outcomes among NSCLC patients.
18F-fluorodeoxyglucose positron emission tomography/computed tomography (18F-FDG PET/CT), an amalgamation of anatomic imaging and functional metabolic imaging, has become pervasive in the realm of lung cancer diagnosis, treatment, and post-treatment monitoring. PET/CT metabolic parameters provide an indirect assessment of tumor cell heterogeneity by quantifying the level of glucose metabolism within tumor cells. Numerous studies have substantiated the association between metabolic parameters and the overall prognosis of NSCLC, underscoring their significance as pivotal prognostic factors (5,6).
Clinicopathologic features, such as stages and pathologic subtypes, are recognized as common prognostic factors in NSCLC. In recent years, the advent of targeted therapy and immunotherapy has elevated the significance of molecular typing in both the diagnosis and treatment of lung cancer. Driver gene mutations, pivotal in altering the biological behavior of tumors, contribute to aspects of heterogeneity and aggressiveness (7), thus emerging as potential prognostic indicators. Furthermore, indicators of physical function, including body mass index (BMI) and sarcopenia, hold notable importance as clinicopathological features (8,9).
Sarcopenia, characterized by persistent loss of skeletal muscle mass (SMM), strength, and function (10), has gained prominence in the context of lung cancer. Its prevalence in the general population over the age of 70 hovers between 5% and 13% (11), but notably surges to approximately 50% in NSCLC patients (12). Timely recognition of sarcopenia, coupled with targeted interventions to enhance SMM and function, presents an avenue for potential improvement in prognostic outcomes (13). Presently, the measurement of the skeletal muscle area (SMA) at the third lumbar vertebrae through CT images, normalized to skeletal muscle index (SMI), serves as a diagnostic criterion for sarcopenia (14).
In light of these considerations, we conducted this study to explore the intricate relationship between sarcopenia and metabolic parameters. Additionally, we discussed the prognostic significance of sarcopenia in combination with metabolic parameters and patient characteristics in early-stage NSCLC patients. We present this article in accordance with the STROBE reporting checklist (available at https://qims.amegroups.com/article/view/10.21037/qims-24-852/rc).
Methods
Patients
This retrospective study involved the analysis of 187 patients diagnosed with NSCLC who underwent 18F-FDG PET/CT examinations at the First Affiliated Hospital of Soochow University between March 2019 and October 2023. The inclusion criteria were as follows: (I) surgically resected and histopathologically confirmed NSCLC; (II) early-stage NSCLC, including stage I or II (15); (III) availability of complete clinical history, immunohistochemical (IHC) results, and PET/CT images; (IV) no antitumor therapy prior to histopathological analysis and imaging; and (V) the absence of a previous history of malignant tumors other than lung cancer. The exclusion criteria were applied to patients with: (I) NSCLC pathologic subtypes other than squamous cell carcinoma (SCC) and adenocarcinomas (ADC); (II) discernible asymmetry in the shape of the psoas major muscle due to trauma, disease, or hereditary factors (Figure 1).
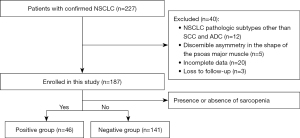
Among the 187 patients with early-stage NSCLC who underwent radical surgery, 41 received postoperative adjuvant chemotherapy. The retrospective analysis of clinicopathological features included age, gender, smoking history, history of diabetes, BMI, sarcopenia, tumor location, presence of cavity, burr characteristics, pathological subtype, tumor-node-metastasis (TNM) stage (according to the Eighth Edition of the TNM Staging System) (15), treatment modalities, cell proliferation antigen (Ki-67), epidermal growth factor receptor (EGFR), anaplastic lymphoma kinase (ALK), programmed death ligand-1 (PD-L1), psoas Hounsfield unit (HU), maximum standardized uptake value (SUVmax), metabolic tumor volume (MTV), total lesion glycolysis (TLG), SUVmax of the psoas major muscle (SUVmax-Muscle), progression-free survival (PFS), and overall survival (OS). Additionally, the study adhered to the principles of the Declaration of Helsinki (as revised in 2013) and obtained ethical approval from the institutional ethics committee of the First Affiliated Hospital of Soochow University (No. 2024-027) and the requirement for individual consent for this retrospective analysis was waived.
Detection of gene mutations
Histological classification of tumor surgical or biopsy specimens was performed using hematoxylin-eosin (H&E) staining. Detection of ALK fusion utilized the anti-ALK (D5F3) rabbit monoclonal antibody (ALK Ventana-D5F3 IHC; Ventana Medical Systems, Oro Valley, AZ, USA) (16); PD-L1 expression was assessed using the anti-PD-L1 (22C3) mouse monoclonal antibody (EnVision Flex, Dako Autostainer Link 48; Dako, Santa Clara, CA, USA) (17). DNA extraction from the samples and the amplification of specific nucleotide fragments containing EGFR gene fragments were achieved through polymerase chain reaction (PCR) technology. Subsequently, detection of EGFR gene mutation was carried out using real-time quantitative PCR (Cobas EGFR Mutation Test v2, Roche Molecular Systems; Pleasanton, CA, USA) (18).
18F-FDG PET/CT acquisition and analysis
Imaging was conducted using a PET/CT scanner (GE Healthcare, Chicago, IL, USA). Patients fasted for at least 6 hours prior to imaging, ensuring that blood glucose levels remained below 11 mmol/L. Subsequently, 18F-FDG was injected at a volume of 3.7–4.4 MBq/kg. Following injection, patients rested for 45–60 minutes. CT scans were conducted from the cranial vault to mid-thighs, with parameters set at an acquisition current of 120 mA, voltage of 140 kV, field of view of 700 mm, rotation speed of 0.8 seconds, and a slice thickness of 3.75 mm. PET imaging ensued, capturing 7–10 bed positions per patient, with 2–3 minutes allotted per bed position. The ordered subset expectation maximization (OSEM) algorithm facilitated the attenuation correction of PET images and their co-location with CT images.
Image analysis was executed at the Advantage Workstation (GE Healthcare, USA) by two nuclear medicine physicians holding an associate senior title or higher. The psoas major muscle and the primary tumor were delineated as regions of interest (ROIs). SUVmax and SUVmean represented the maximum and average values of the ROI, respectively. The European Association of Nuclear Medicine’s recommended MTV was determined using a 41% SUVmax cutoff value. TLG was computed by multiplying MTV by SUVmean (19).
Sarcopenia measurement
Semi-automatic outlining of the SMA at the third lumbar vertebra was performed on CT images obtained from PET/CT imaging, utilizing a threshold range of 29 HU to 150 HU (20). SMA was normalized to SMI (cm2/m2) based on the patient’s height. Sarcopenia was defined as SMI <32.50 cm2/m2 for females and SMI <44.77 cm2/m2 for males (21) (Figure 2). SMA measurements were conducted using Tomovision sliceOmatic (Version 5.0 Rev-9; TomoVision, Magog, Quebec, Canada). In addition, a BMI <18.5 indicated emaciation.
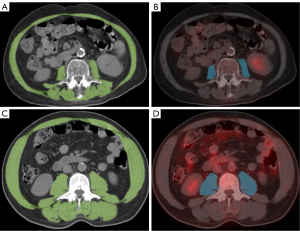
Statistical analysis
The Kolmogorov-Smirnov (KS) test was employed to assess the normality of the statistical data. Differences in categorical variables were assessed using the Chi-squared test, whereas differences in non-normally distributed continuous variables were analyzed with the Mann-Whitney U-test. Binary logistic regression analyzed the risk factors of sarcopenia. PFS was defined as the duration from treatment to progression or death, and OS was defined as the time from treatment to death.
Receiver operating characteristic (ROC) curve analysis was performed to determine the optimal threshold of metabolic parameters for predicting prognosis. Kaplan-Meier (KM) analysis and Cox proportional-hazards regression were employed for survival analysis. A significance threshold was set at P<0.05, and all P values were derived from two-sided tests. All statistical analyses were carried out using the statistical software SPSS 22.0 (IBM Corp., Armonk, NY, USA).
Results
Patient characteristics
In this study, 187 patients diagnosed with early-stage NSCLC were included, spanning an age range of 30 to 86 years, with a median age of 65 years. Among them, 66 (35.29%) were women, and 121 (64.71%) were men. Sarcopenia was diagnosed in 46 patients (24.60%), comprising 40 men and six women. The distribution of pathological subtypes revealed 136 cases (72.73%) of ADC and 51 cases (27.27%) of SCC. Additionally, 76 cases (40.64%) were classified as central NSCLC, whereas 111 cases (59.36%) were categorized as peripheral NSCLC. Furthermore, 96 patients (51.34%) tested positive for EGFR, 11 (5.88%) were positive for ALK, and 119 (63.64%) were positive for PD-L1. Diabetes was diagnosed in 26 patients (13.90%), and 16 patients (8.56%) exhibited a lower BMI, indicative of wasting. The staging of NSCLC patients revealed 92 cases (49.20%) at stage I and 95 cases (50.80%) at stage II (Table 1).
Table 1
Characteristics | Sarcopenia (n=46) | Non-sarcopenia (n=141) | c2 | P value |
---|---|---|---|---|
Age (<65/≥65 years) | 17 vs. 29 | 79 vs. 62 | 5.050 | 0.025* |
Gender (male/female) | 40 vs. 6 | 81 vs. 60 | 13.551 | <0.001* |
Diabetes (yes/no) | 7 vs. 39 | 19 vs. 122 | 0.088 | 0.767 |
BMI (<18.5/≥18.5 kg/m2) | 10 vs. 36 | 6 vs. 135 | 16.641 | <0.001* |
Location (center/periphery) | 23 vs. 23 | 53 vs. 88 | 2.215 | 0.137 |
Cavity (yes/no) | 21 vs. 25 | 49 vs. 92 | 1.760 | 0.185 |
Burr (yes/no) | 25 vs. 21 | 82 vs. 59 | 0.205 | 0.650 |
Pathology (ADC/SCC) | 31 vs. 15 | 105 vs. 36 | 0.876 | 0.349 |
Smoking (yes/no) | 28 vs. 18 | 93 vs. 48 | 0.393 | 0.531 |
Clinical stage (I/II) | 19 vs. 27 | 73 vs. 68 | 1.521 | 0.217 |
Ki-67 (<20%/≥20%) | 9 vs. 37 | 41 vs. 100 | 1.602 | 0.206 |
EGFR (positive/negative) | 22 vs. 24 | 74 vs. 67 | 0.301 | 0.583 |
ALK (positive/negative) | 1 vs. 45 | 10 vs. 131 | 1.515 | 0.218 |
PD-L1 (positive/negative) | 32 vs. 14 | 87 vs. 54 | 0.927 | 0.336 |
Psoas HU | 35.28 (14.68–50.14) | 37.97 (19.34–49.62) | – | 0.006* |
SUVmax | 5.31 (1.65–13.90) | 6.36 (0.55–17.76) | – | 0.103 |
MTV | 18.82 (2.74–241.27) | 21.02 (1.47–151.78) | – | 0.282 |
TLG | 60.33 (7.06–1,942.67) | 73.57 (0.84–1,132.26) | – | 0.162 |
SUVmax-Muscle | 0.84 (0.41–1.62) | 0.99 (0.53–1.73) | – | <0.001* |
Frequencies were reported for categorical variables and median (range) were reported for continuous variables. *, statistically significant. BMI, body mass index; ADC, adenocarcinoma; SCC, squamous cell carcinoma; Ki-67, cell proliferation antigen; EGFR, epidermal growth factor receptor; ALK, anaplastic lymphoma kinase; PD-L1, programmed death ligand-1; HU, hounsfield unit; SUVmax, maximum standard uptake value; MTV, metabolic tumor volume; TLG, total lesion glycolysis; SUVmax-Muscle, maximum standardized uptake value of the psoas major muscle.
Relationship between clinicopathologic features, metabolic parameters, and sarcopenia
Table 1 demonstrates significant differences between the sarcopenia and non-sarcopenia groups, encompassing age, gender, BMI, psoas HU, and SUVmax-Muscle (P<0.05 for all). Notably, the psoas HU and SUVmax-Muscle in the sarcopenia group were lower compared to the non-sarcopenia group (P<0.05 for all). No significant differences in other metabolic parameters, including SUVmax, MTV, and TLG, were observed between the two groups (P>0.05 for all).
The analysis identified gender [odds ratio (OR) =0.103; 95% confidence interval (CI): 0.042–0.249; P<0.001], BMI (OR =0.052; 95% CI: 0.019–0.148; P<0.001), psoas HU (OR =0.860; 95% CI: 0.812–0.910; P<0.001), and SUVmax-Muscle (OR =0.146; 95% CI: 0.053–0.403; P<0.001) were identified as independent predictors of sarcopenia (P<0.05 for all) (Table 2). A nomogram model incorporating these variables was constructed for the prediction of sarcopenia (Figure 3).
Table 2
Variable | OR | 95% CI | P value |
---|---|---|---|
Age (<65/≥65 years) | 1.145 | 0.620–2.117 | 0.665 |
Gender (male/female) | 0.103 | 0.042–0.249 | <0.001* |
BMI (<18.5/≥18.5 kg/m2) | 0.052 | 0.019–0.148 | <0.001* |
Psoas HU (high/low) | 0.860 | 0.812–0.910 | <0.001* |
SUVmax-Muscle (high/low) | 0.146 | 0.053–0.403 | <0.001* |
*, statistically significant. OR, odds ratio; CI, confidence interval; BMI, body mass index; HU, hounsfield unit; SUVmax-Muscle, maximum standardized uptake value of the psoas major muscle.
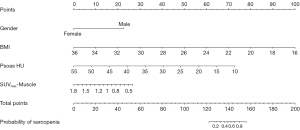
Survival analysis for early-stage NSCLC
Among the 187 early-stage NSCLC patients, 41 underwent postoperative adjuvant chemotherapy after radical surgery. Over a median follow-up duration of 36 months, 104 patients experienced tumor progression, resulting in 51 deaths. The 1-, 2-, and 3-year PFS rates were 71.1%, 50.8%, and 45.5%, respectively, whereas the 1-, 2-, and 3-year OS rates were 89.3%, 81.3%, and 75.9%, respectively. The median PFS and OS were 25 months and 36 months, respectively.
Univariate Cox regression analysis identified significant associations between gender, sarcopenia, clinical stage, adjuvant chemotherapy, and PFS (P<0.05 for all). Additionally, gender, sarcopenia, SUVmax, MTV, TLG, and PD-L1 were significantly associated with OS (P<0.05 for all) (Table 3, Figure 4).
Table 3
Variable | PFS | OS | |||||
---|---|---|---|---|---|---|---|
HR | 95% CI | P value | HR | 95% CI | P value | ||
Age (<65/≥65 years) | 0.990 | 0.680–1.441 | 0.958 | 1.288 | 0.697–2.380 | 0.418 | |
Gender (male/female) | 0.577 | 0.371–0.897 | 0.015* | 0.361 | 0.162–0.807 | 0.013* | |
Diabetes (yes/no) | 0.706 | 0.415–1.201 | 0.706 | 0.730 | 0.326–1.633 | 0.443 | |
BMI (<18.5/≥18.5 kg/m2) | 1.017 | 0.495–2.089 | 0.963 | 0.622 | 0.264–1.467 | 0.278 | |
Sarcopenia (yes/no) | 1.697 | 1.148–2.507 | 0.008* | 5.680 | 3.074–10.494 | <0.001* | |
Location (center/periphery) | 0.819 | 0.562–1.194 | 0.299 | 0.923 | 0.520–1.637 | 0.783 | |
Cavity (yes/no) | 1.172 | 0.782–1.757 | 0.441 | 0.947 | 0.518–1.733 | 0.861 | |
Burr (yes/no) | 1.331 | 0.902–1.965 | 0.150 | 1.161 | 0.626–2.151 | 0.635 | |
Pathology (ADC/SCC) | 1.484 | 1.007–2.185 | 0.053 | 1.488 | 0.794–2.790 | 0.214 | |
Smoking (yes/no) | 1.047 | 0.718–1.526 | 0.811 | 1.349 | 0.743–2.449 | 0.325 | |
Clinical stage (I/II) | 1.795 | 1.234–2.611 | 0.002* | 1.515 | 0.854–2.688 | 0.155 | |
Chemotherapy (yes/no) | 1.719 | 1.149–2.570 | 0.008* | 1.449 | 0.785–2.677 | 0.236 | |
Ki-67 (<20%/≥20%) | 1.346 | 0.792–2.288 | 0.272 | 2.422 | 0.869–6.754 | 0.091 | |
EGFR (positive/negative) | 1.103 | 0.758–1.605 | 0.608 | 0.774 | 0.432–1.387 | 0.389 | |
ALK (positive/negative) | 1.214 | 0.495–2.977 | 0.673 | 0.956 | 0.230–3.970 | 0.950 | |
PD-L1 (positive/negative) | 0.646 | 0.414–1.010 | 0.055 | 0.042 | 0.006–0.307 | 0.002* | |
SUVmax | 1.019 | 0.989–1.049 | 0.218 | 1.062 | 1.025–1.100 | 0.001* | |
MTV | 1.001 | 0.974–1.003 | 0.096 | 1.004 | 1.002–1.005 | <0.001* | |
TLG | 1.138 | 1.063–1.219 | 0.097 | 1.335 | 1.214–1.577 | <0.001* |
*, statistically significant. PFS, progression-free survival; OS, overall survival; NSCLC, non-small cell lung cancer; HR, hazard ratio; CI, confidence interval; BMI, body mass index; ADC, adenocarcinoma; SCC, squamous cell carcinoma; Ki-67, cell proliferation antigen; EGFR, epidermal growth factor receptor; ALK, anaplastic lymphoma kinase; PD-L1, programmed death ligand-1; SUVmax, maximum standard uptake value; MTV, metabolic tumor volume; TLG, total lesion glycolysis.
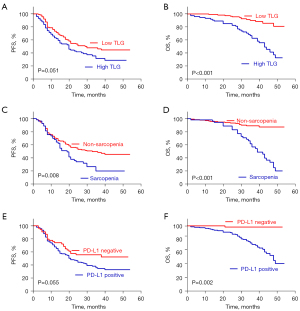
ROC curve analysis determined the optimal cut-off values of SUVmax, MTV, and TLG for predicting OS to be 6.2, 20.1, and 66.5, respectively. The area under the curve (AUC) for SUVmax was 0.691 (95% CI: 0.607–0.774; P<0.001), for MTV it was 0.709 (95% CI: 0.619–0.800; P<0.001), and for TLG it was 0.736 (95% CI: 0.647–0.824; P<0.001).
Factors that were statistically significant in the univariate Cox regression analysis were included as covariates in the multivariate analysis. The multivariate Cox regression analysis revealed that gender [hazard ratio (HR) =0.616; 95% CI: 0.390–0.973; P=0.038] and clinical stage (HR =1.637; 95% CI: 1.074–2.497; P=0.022) were independent prognostic factors for PFS. No significant differences in PFS were observed between those with and without sarcopenia or chemotherapy (P>0.05 for all).
For OS, sarcopenia (HR =3.323; 95% CI: 1.748–6.316; P<0.001), TLG (HR =2.499; 95% CI: 2.014–3.267; P=0.016), and PD-L1 (HR =0.093; 95% CI: 0.012–0.698; P=0.021) were established as independent prognostic factors, whereas gender, SUVmax, and MTV were not significant (P>0.05 for all) (Table 4).
Table 4
Variable | HR | 95% CI | P value |
---|---|---|---|
Multivariable Cox regression for PFS | |||
Gender (male/female) | 0.616 | 0.390–0.973 | 0.038* |
Sarcopenia (yes/no) | 1.426 | 0.948–2.145 | 0.089 |
Clinical stage (I/II) | 1.637 | 1.074–2.497 | 0.022* |
Chemotherapy (yes/no) | 1.289 | 0.816–2.038 | 0.276 |
Multivariable Cox regression for OS: entering MTV | |||
Gender (male/female) | 0.688 | 0.296–1.601 | 0.386 |
Sarcopenia (yes/no) | 3.470 | 1.822–6.607 | <0.001* |
PD-L1 (positive/negative) | 0.078 | 0.010–0.575 | 0.012* |
SUVmax | 1.021 | 0.973–1.071 | 0.393 |
MTV | 1.002 | 1.000–1.004 | 0.115 |
Multivariable Cox regression for OS: entering TLG | |||
Gender (male/female) | 0.710 | 0.307–1.642 | 0.424 |
Sarcopenia (yes/no) | 3.323 | 1.748–6.316 | <0.001* |
PD-L1 (positive/negative) | 0.093 | 0.012–0.698 | 0.021* |
SUVmax | 1.001 | 0.948–1.054 | 0.990 |
TLG | 2.499 | 2.014–3.267 | 0.016* |
*, statistically significant. PFS, progression-free survival; OS, overall survival; NSCLC, non-small cell lung cancer; HR, hazard ratio; CI, confidence interval; MTV, metabolic tumor volume; PD-L1, programmed death ligand-1; SUVmax, maximum standard uptake value; TLG, total lesion glycolysis.
Risk stratification for early-stage NSCLC
Risk stratification for patients with early-stage NSCLC was performed based on prognostic survival analysis. Early-stage NSCLC patients were categorized into three groups according to TLG, sarcopenia, and PD-L1 expression. Patients with high TLG, sarcopenia, and positive PD-L1 expression were designated as the high-risk group, whereas those with low TLG, non-sarcopenia, and negative PD-L1 expression were classified as the low-risk group. The remaining patients comprised the intermediate-risk group.
The distribution of patients among the high-, intermediate-, and low-risk groups was 28, 121, and 38, respectively. KM analysis (Figure 5) revealed significant differences in OS among these groups, with the high-risk group exhibiting the poorest prognosis (P<0.05 for all). This risk stratification model, incorporating TLG, sarcopenia, and PD-L1 expression, offered valuable insights for personalized therapy and follow-up strategies in patients with early-stage NSCLC.
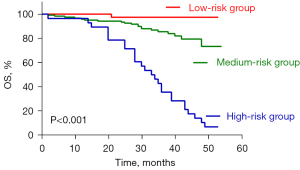
Discussion
Our study demonstrated significant associations between sarcopenia and male sex, low BMI, low psoas HU, and low SUVmax-Muscle. In early-stage NSCLC, independent predictors of OS included TLG, sarcopenia, and PD-L1 expression. Notably, patients with high TLG, sarcopenia, and PD-L1 positivity exhibited the poorest prognosis.
Several studies have consistently affirmed the association between high TLG and poor OS in NSCLC (5,6,22,23), which aligns with our current findings. However, two other studies have identified SUVmax as an independent predictor of poor OS rather than TLG (24,25). We postulated that the inclusion of TLG in the analysis might potentially yield positive results. SUVmax, being limited to the semi-quantitative analysis of FDG uptake in single voxels, may not adequately represent the overall biological metabolism level of tumors. Additionally, as a two-dimensional parameter, SUVmax fails to capture the tumor’s size or volume. In contrast, TLG, as a three-dimensional metabolic parameter combining tumor volume and metabolic information, offers a more comprehensive reflection of tumor burden and biological aggressiveness. We argue that TLG contributed to the nuanced stratification of patients at the same stage, facilitating personalized risk-adapted treatment strategies.
The prognostic role of sarcopenia in NSCLC patients remains a subject of controversy in the literature. Studies by Li et al. (22) and Hasenauer et al. (26), which included 344 and 401 NSCLC patients, respectively, have concluded that sarcopenia is not a predictor of OS. In contrast, studies by Yuan et al. (23) and Karaman et al. (27), incorporating 202 and 56 patients with advanced-stage NSCLC, respectively, have identified a correlation between sarcopenia and poor OS, aligning with our findings. The impact of sarcopenia on treatment-related toxicity is noteworthy, potentially resulting in prolonged post-treatment recovery or early termination of established treatment regimens. This effect may be attributed to alterations in the distribution of chemotherapeutic agents caused by reductions in skeletal muscle and adipose tissue. Such factors can elucidate the observed shortened PFS and OS associated with sarcopenia (28). Furthermore, the association between sarcopenia and low SUVmax-Muscle and low psoas HU, consistent with previous studies (22,23), suggested that intramuscular fat deposition in lung cancer patients may contribute to decreased muscle density and glucose metabolism, providing a plausible explanation for our findings.
In addition to TLG and sarcopenia, PD-L1 emerged as an independent predictor of OS in advanced-stage NSCLC, aligning with findings from other studies (29,30). PD-L1 expression is not only relevant for immunotherapy but also stands as a crucial prognostic factor. Based on our survival analysis results, we integrated TLG, PD-L1, and sarcopenia into a comprehensive risk stratification model for NSCLC prognosis. The model revealed that patients in the high-risk group had the poorest prognosis, followed by those in the intermediate-risk group, with the low-risk group exhibiting the best prognosis. A study by Yuan et al. (23), which used sarcopenia, stage, and TLG to construct a nomogram model predicting survival, mirrors our study protocol. This underscores the value of combining clinicopathologic features and metabolic parameters for a more precise prognostic analysis. Our model aided in identifying high-risk patients and tailoring personalized treatment plans. We advocated for the consideration of nutritional support and appropriate exercise alongside immunotherapy for patients with high TLG and sarcopenia to mitigate muscle loss.
Several limitations warrant consideration in our current study. Firstly, the measurement and calculation of the SMI were time-consuming and laborious, compounded by a lack of consensus on the measurement methods and the cut-off values defining sarcopenia. Secondly, according to the recommendations of the European Working Group on Sarcopenia in Older People (EWGSOP), sarcopenia encompasses not only a decrease in muscle mass but also a decline in muscle strength and physical performance (31). There is a lack of clinically feasible methods for strength assessment, which further complicates the evaluation process. Thirdly, incorporating additional clinicopathologic features is essential to enhance the precision of prognostic models. Expanding the scope of analyzed variables could offer a more comprehensive understanding of the factors influencing the prognosis of NSCLC patients. Finally, it is crucial to acknowledge that our study relied on a single-center retrospective analysis. To establish the reliability and generalizability of the developed model, external validation with data from other centers is imperative. A multi-center approach would help to validate the robustness and applicability of the prognostic model across diverse patient populations and clinical settings. Addressing these limitations in future research endeavors will contribute to the refinement and broader applicability of prognostic models for NSCLC.
Conclusions
The incidence of sarcopenia in NSCLC patients increased with decreasing SUVmax-Muscle and psoas HU. In early-stage NSCLC, TLG, sarcopenia, and PD-L1 expression emerged as independent prognostic factors for OS. The risk stratification model, incorporating TLG, sarcopenia, and PD-L1 expression, played a pivotal role in guiding personalized therapy and follow-up strategies. This comprehensive approach to risk assessment enhanced our ability to tailor interventions and monitoring protocols for early-stage NSCLC patients, contributing to more effective and individualized care.
Acknowledgments
Funding: This work was supported by
Footnote
Reporting Checklist: The authors have completed the STROBE reporting checklist. Available at https://qims.amegroups.com/article/view/10.21037/qims-24-852/rc
Conflicts of Interest: All authors have completed the ICMJE uniform disclosure form (available at https://qims.amegroups.com/article/view/10.21037/qims-24-852/coif). The authors have no conflicts of interest to declare.
Ethical Statement: The authors are accountable for all aspects of the work in ensuring that questions related to the accuracy or integrity of any part of the work are appropriately investigated and resolved. The study was conducted in accordance with the Declaration of Helsinki (as revised in 2013). The study was approved by ethics committee of the First Affiliated Hospital of Soochow University (No. 2024-027) and the requirement for individual consent for this retrospective analysis was waived.
Open Access Statement: This is an Open Access article distributed in accordance with the Creative Commons Attribution-NonCommercial-NoDerivs 4.0 International License (CC BY-NC-ND 4.0), which permits the non-commercial replication and distribution of the article with the strict proviso that no changes or edits are made and the original work is properly cited (including links to both the formal publication through the relevant DOI and the license). See: https://creativecommons.org/licenses/by-nc-nd/4.0/.
References
- Sung H, Ferlay J, Siegel RL, Laversanne M, Soerjomataram I, Jemal A, Bray F. Global Cancer Statistics 2020: GLOBOCAN Estimates of Incidence and Mortality Worldwide for 36 Cancers in 185 Countries. CA Cancer J Clin 2021;71:209-49. [Crossref] [PubMed]
- Bade BC, Dela Cruz CS. Lung Cancer 2020: Epidemiology, Etiology, and Prevention. Clin Chest Med 2020;41:1-24. [Crossref] [PubMed]
- Yang T, Xiong Y, Zeng Y, Wang Y, Zeng J, Liu J, Xu S, Li LS. Current status of immunotherapy for non-small cell lung cancer. Front Pharmacol 2022;13:989461. [Crossref] [PubMed]
- Ettinger DS, Wood DE, Aisner DL, Akerley W, Bauman JR, Bharat A, et al. Non-Small Cell Lung Cancer, Version 3.2022, NCCN Clinical Practice Guidelines in Oncology. J Natl Compr Canc Netw 2022;20:497-530. [Crossref] [PubMed]
- Agüloğlu N, Aksu A, Unat DS, Akyol M. The prognostic relationship of 18F-FDG PET/CT metabolic and volumetric parameters in metastatic ALK + NSCLC. Nucl Med Commun 2022;43:1217-24. [Crossref] [PubMed]
- Hashimoto K, Kaira K, Imai H, Mouri A, Shiono A, Miura Y, Yamaguchi O, Kobayashi K, Kagamu H, Kuji I. Prognostic Potential of Metabolic Activity on 18 F-FDG Accumulation in Advanced NSCLC Receiving Combining Chemotherapy Plus PD-1 Blockade. J Immunother 2022;45:349-57. [Crossref] [PubMed]
- Madeddu C, Donisi C, Liscia N, Lai E, Scartozzi M, Macciò A. EGFR-Mutated Non-Small Cell Lung Cancer and Resistance to Immunotherapy: Role of the Tumor Microenvironment. Int J Mol Sci 2022;23:6489. [Crossref] [PubMed]
- Tan Y, Duan T, Li B, Zhang B, Zhu Y, Yan K, Song J, Lv T, Yang J, Jiang L, Yang J, Wen T, Yan L. Sarcopenia defined by psoas muscle index independently predicts long-term survival after living donor liver transplantation in male recipients. Quant Imaging Med Surg 2022;12:215-28. [Crossref] [PubMed]
- van Heusden HC, Chargi N, Dankbaar JW, Smid EJ, de Bree R. Masseter muscle parameters can function as an alternative for skeletal muscle mass assessments on cross-sectional imaging at lumbar or cervical vertebral levels. Quant Imaging Med Surg 2022;12:15-27. [Crossref] [PubMed]
- Damluji AA, Alfaraidhy M, AlHajri N, Rohant NN, Kumar M, Al Malouf C, Bahrainy S, Ji Kwak M, Batchelor WB, Forman DE, Rich MW, Kirkpatrick J, Krishnaswami A, Alexander KP, Gerstenblith G, Cawthon P, deFilippi CR, Goyal P. Sarcopenia and Cardiovascular Diseases. Circulation 2023;147:1534-53. [Crossref] [PubMed]
- Boutin RD, Yao L, Canter RJ, Lenchik L. Sarcopenia: Current Concepts and Imaging Implications. AJR Am J Roentgenol 2015;205:W255-66. [Crossref] [PubMed]
- Suzuki Y, Okamoto T, Fujishita T, Katsura M, Akamine T, Takamori S, Morodomi Y, Tagawa T, Shoji F, Maehara Y. Clinical implications of sarcopenia in patients undergoing complete resection for early non-small cell lung cancer. Lung Cancer 2016;101:92-7. [Crossref] [PubMed]
- Tagliafico AS, Bignotti B, Torri L, Rossi F. Sarcopenia: how to measure, when and why. Radiol Med 2022;127:228-37. [Crossref] [PubMed]
- Vogele D, Otto S, Sollmann N, Haggenmüller B, Wolf D, Beer M, Schmidt SA. Sarcopenia - Definition, Radiological Diagnosis, Clinical Significance. Rofo 2023;195:393-405. [Crossref] [PubMed]
- Goldstraw P, Chansky K, Crowley J, Rami-Porta R, Asamura H, Eberhardt WE, Nicholson AG, Groome P, Mitchell A, Bolejack VInternational Association for the Study of Lung Cancer Staging and Prognostic Factors Committee, Advisory Boards, and Participating Institutions. International Association for the Study of Lung Cancer Staging and Prognostic Factors Committee Advisory Boards and Participating Institutions. The IASLC Lung Cancer Staging Project: Proposals for Revision of the TNM Stage Groupings in the Forthcoming (Eighth) Edition of the TNM Classification for Lung Cancer. J Thorac Oncol 2016;11:39-51. [Crossref] [PubMed]
- Taheri D, Zahavi DJ, Del Carmen Rodriguez M, Meliti A, Rezaee N, Yonescu R, Ricardo BF, Dolatkhah S, Ning Y, Bishop JA, Netto GJ, Sharma R. For staining of ALK protein, the novel D5F3 antibody demonstrates superior overall performance in terms of intensity and extent of staining in comparison to the currently used ALK1 antibody. Virchows Arch 2016;469:345-50. [Crossref] [PubMed]
- Roach C, Zhang N, Corigliano E, Jansson M, Toland G, Ponto G, Dolled-Filhart M, Emancipator K, Stanforth D, Kulangara K. Development of a Companion Diagnostic PD-L1 Immunohistochemistry Assay for Pembrolizumab Therapy in Non-Small-cell Lung Cancer. Appl Immunohistochem Mol Morphol 2016;24:392-7. [Crossref] [PubMed]
- Agüloğlu N, Aksu A, Akyol M, Katgı N, Doksöz TÇ. IMPORTANCE of PRETREATMENT 18F-FDG PET/CT TEXTURE ANALYSIS in PREDICTING EGFR and ALK MUTATION in PATIENTS with NON-SMALL CELL LUNG CANCER. Nuklearmedizin 2022;61:433-9. [Crossref] [PubMed]
- Boellaard R, Delgado-Bolton R, Oyen WJ, Giammarile F, Tatsch K, Eschner W, et al. FDG PET/CT: EANM procedure guidelines for tumour imaging: version 2.0. Eur J Nucl Med Mol Imaging 2015;42:328-54. [Crossref] [PubMed]
- Gomez-Perez S, McKeever L, Sheean P. Tutorial: A Step-by-Step Guide (Version 2.0) for Measuring Abdominal Circumference and Skeletal Muscle From a Single Cross-Sectional Computed-Tomography Image Using the National Institutes of Health ImageJ. JPEN J Parenter Enteral Nutr 2020;44:419-24. [Crossref] [PubMed]
- Zeng X, Shi ZW, Yu JJ, Wang LF, Luo YY, Jin SM, Zhang LY, Tan W, Shi PM, Yu H, Zhang CQ, Xie WF. Sarcopenia as a prognostic predictor of liver cirrhosis: a multicentre study in China. J Cachexia Sarcopenia Muscle 2021;12:1948-58. [Crossref] [PubMed]
- Li D, Tan X, Yuan H, Yao X, Sun X, He L, Jiang L. Usefulness of 18 F-FDG PET/computed tomography metabolic parameters in predicting sarcopenia and prognosis of treatment-naive patients with non-small cell lung cancer. Nucl Med Commun 2023;44:309-17. [Crossref] [PubMed]
- Yuan H, Tan X, Sun X, He L, Li D, Jiang L. Role of (18)F-FDG PET/CT and sarcopenia in untreated non-small cell lung cancer with advanced stage. Jpn J Radiol 2023;41:521-30. [Crossref] [PubMed]
- Kaira K, Shimizu K, Kitahara S, Yajima T, Atsumi J, Kosaka T, Ohtaki Y, Higuchi T, Oyama T, Asao T, Mogi A. 2-Deoxy-2-fluorine-18 fluoro-d-glucose uptake on positron emission tomography is associated with programmed death ligand-1 expression in patients with pulmonary adenocarcinoma. Eur J Cancer 2018;101:181-90. [Crossref] [PubMed]
- Kasahara N, Kaira K, Bao P, Higuchi T, Arisaka Y, Erkhem-Ochir B, Sunaga N, Ohtaki Y, Yajima T, Kosaka T, Oyama T, Yokobori T, Asao T, Nishiyama M, Tsushima Y, Kuwano H, Shimizu K, Mogi A. Correlation of tumor-related immunity with 18F-FDG-PET in pulmonary squamous-cell carcinoma. Lung Cancer 2018;119:71-7. [Crossref] [PubMed]
- Hasenauer A, Forster C, Hungerbühler J, Perentes JY, Abdelnour-Berchtold E, Koerfer J, Krueger T, Becce F, Gonzalez M. CT-Derived Sarcopenia and Outcomes after Thoracoscopic Pulmonary Resection for Non-Small Cell Lung Cancer. Cancers (Basel) 2023;15:790. [Crossref] [PubMed]
- Karaman E, Hursoy N, Goksel S. The Effect of Sarcopenia and Metabolic PET-CT Parameters on Survival in Locally Advanced Non-Small Cell Lung Carcinoma. Nutr Cancer 2023;75:286-95. [Crossref] [PubMed]
- Kim EY, Lee HY, Kim YS, Park I, Ahn HK, Cho EK, Jeong YM, Kim JH. Prognostic significance of cachexia score assessed by CT in male patients with small cell lung cancer. Eur J Cancer Care (Engl) 2018; [Crossref]
- Miyazawa T, Otsubo K, Sakai H, Kimura H, Chosokabe M, Morikawa K, Furuya N, Marushima H, Kojima K, Mineshita M, Koike J, Saji H. Combining PD-L1 Expression and Standardized Uptake Values in FDG-PET/CT Can Predict Prognosis in Patients With Resectable Non-Small-Cell Lung Cancer. Cancer Control 2021;28:10732748211038314. [Crossref] [PubMed]
- Zhang M, Wang D, Sun Q, Pu H, Wang Y, Zhao S, Wang Y, Zhang Q. Prognostic significance of PD-L1 expression and (18)F-FDG PET/CT in surgical pulmonary squamous cell carcinoma. Oncotarget 2017;8:51630-40. [Crossref] [PubMed]
- Cruz-Jentoft AJ, Bahat G, Bauer J, Boirie Y, Bruyère O, Cederholm T, Cooper C, Landi F, Rolland Y, Sayer AA, Schneider SM, Sieber CC, Topinkova E, Vandewoude M, Visser M, Zamboni M. Writing Group for the European Working Group on Sarcopenia in Older People 2 (EWGSOP2), and the Extended Group for EWGSOP2. Sarcopenia: revised European consensus on definition and diagnosis. Age Ageing 2019;48:601. [Crossref] [PubMed]