Support vector machine classification of irritable bowel syndrome patients based on whole-brain resting-state functional connectivity features
Introduction
Irritable bowel syndrome (IBS) is a common functional gastrointestinal disease, often accompanied by changes in bowel habits and stool characteristics (1). The pathogenic factors are various and pathophysiological mechanism of IBS are unknown, and lack of biomarker for structural or biochemical abnormality. In general, the pathogenesis of IBS is related to symptoms, such as gut microbiota disorder, gastrointestinal motility imbalance, genetic factors, diet, and psychological state. In recent years, studies have shown that the microbiota-gut-brain axis (MGBA) has a significant effect on the pathogenesis of IBS (2). The MGBA is composed of bodily fluids produced by gut microbiota and their products, intestinal tissue, and endocrine and immune systems, as well as the vagus and spinal nerves (3), which work together to regulate gut microbiota and the brain through various pathways. Due to the diverse and non-specific symptoms of IBS, it can easily be confused with other gastrointestinal disorders, such as Crohn’s disease, which is a clinically chronic inflammatory bowel disease that has been shown to be closely related to brain-gut axis dysfunction (4). A series of examinations and tests are required to exclude other diseases during the diagnostic process. However, these standards rely on patient self-reporting and may be influenced by their ability to understand and describe their symptoms. Therefore, neuroimaging research on IBS patients will assist in further understanding the corresponding pathogenesis of IBS.
At present, neuroimaging studies related to IBS include magnetic resonance imaging (MRI), and positron emission tomography (PET) studies (5,6). Resting-state functional magnetic resonance imaging (rs-fMRI), based on the contrast mechanism of blood oxygenation level dependence (BOLD), identifies brain functional states by observing brain signals in the resting state (7). Unlike most previous studies on task-state functional magnetic resonance imaging (fMRI), rs-fMRI studies are simple and do not require excessive patient cooperation (8). Chen et al. (9) measured altered spontaneous brain activity and functional connectivity (FC) in patients with diarrhea-predominant irritable bowel syndrome (IBS-D) using rs-fMRI, they found that abnormal spontaneous activity and FC dysregulation in brain regions related to pain regulation and emotional arousal in the prefrontal-limbic-midbrain circuit and somatosensory processing. Rs-fMRI is considered a reliable and direct method for measuring brain activity.
Machine learning is a scientific technology that uses data learning to help machines learn patterns from existing complex data with good generalizability. This technology has gained significant traction across various domains, due to its potential to automate decision-making and predictive tasks with high accuracy. Supervised learning can train algorithms by using labeled datasets to classify or accurately predict results (10,11), thereby better distinguishing between IBS patients and healthy subjects. By leveraging labeled datasets comprising clinical and demographic information, these algorithms can be trained to identify subtle patterns and differences that may not be apparent to human clinicians.
In summary, this study aimed to identify the neural network information of IBS patients and healthy controls (HCs) through whole-brain FC calculations based on regions of interest (ROIs), train classification models using supervised learning, construct better classification prediction models, and analyze the weights of neural network information classification to identify important regions in the human central nervous system that can be used to distinguish IBS patients from HCs to improve the diagnostic efficiency of IBS in clinical practice. We present this article in accordance with the TRIPOD+AI reporting checklist (available at https://qims.amegroups.com/article/view/10.21037/qims-24-892/rc).
Methods
Subjects
This study was conducted in accordance with the Declaration of Helsinki (as revised in 2013). This study was approved by the Ethics Committee of the First Affiliated Hospital of Shantou University Medical College (No. B-2022-020). All the participants gave written informed consent.
The study included two groups of subjects: patients with IBS, and HCs. Data were collected from January 2020 to January 2024. The IBS patients were recruited from the Department of Gastroenterology of the First Affiliated Hospital of Shantou University Medical College. To be eligible for inclusion in this study, the patients had to meet the following inclusion criteria (Figure 1): (I) have been diagnosed with IBS based on the Rome IV diagnostic criteria (12); (II) be aged between 18 and 60 years, have at least six years of education, be right-handed, and have normal/corrected-to-normal vision; and (III) have complete clinical data, neuropsychological scale assessment data, and MRI data available. Patients were excluded from the study if they met any of the following exclusion criteria: (I) had a history of organic gastrointestinal diseases, such as inflammatory bowel disease, or colon polyps, or had undergone gastrointestinal surgery; (II) had a history of central nervous system diseases or any diseases that may affect cognitive impairment; (III) had a history of neuropsychiatric diseases, drug or alcohol abuse or dependence; and/or (IV) had MRI contraindications. Initially, a total of 92 patients with IBS were included in the study, among whom, three were excluded for incomplete MRI data collection, as they were unable to cooperate with the MRI scans, and one was excluded due to their head motion angle exceeding 2° during the rs-fMRI data preprocessing analysis. Thus, ultimately, 88 patients were included in the final analysis. A detailed flow diagram of the patients included and excluded in study is shown in Figure 1. The HC group comprised 88 age-, sex- and education-matched HCs.
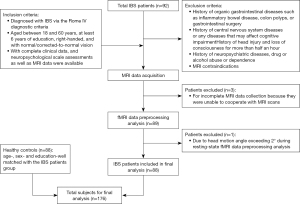
Questionnaires
The Montreal Cognitive Assessment (MoCA) (13) is a scale tool revised and published by Nasreddine et al. in 2004 for screening for mild cognitive impairment. It is an extension of the Mini-Mental State Examination and can more sensitively evaluate the overall cognitive function of participants. A total score below 26 and above 19 for a subject indicates the possibility of mild cognitive impairment, while a score below 19 indicates a higher likelihood of dementia. The participants in this study completed the Chinese version of the MoCA scale (14), which had been translated and revised by Peking Union Medical College Hospital in the same testing location and time limit.
MRI examination
The MRI scanning equipment used in this study was the Signa HDxt 1.5T superconducting MRI scanner (GE, the United States). The MRI scanning was completed by experienced MRI technicians at the First Affiliated Hospital of Shantou University Medical College and reviewed by professional MRI diagnostic physicians to exclude intracranial organic lesions in the subjects. T1-weighted imaging (T1WI) uses three-dimensional fast gradient-echo sequence technology to obtain fine anatomical images. The imaging parameters were as follows: echo time: 1.6 ms; repetition time: 5.1 ms; field of view: 256 mm × 256 mm; flip angle: 10°; and slice thickness: 1.4 mm. The fMRI scanning uses gradient echo-echo planar imaging (15), which can effectively improve the sensitivity and temporal resolution of blood oxygen saturation. The imaging parameters were as follows: echo time: 45 ms; repetition time: 3,000 ms; field of view: 250 mm × 200 mm; flip angle: 90°; and slice thickness: 6 mm.
fMRI data preprocessing
The fMRI data were processed using the MATLAB (MathWorks, Inc., Natick, MA, USA) platform and the GRETNA toolbox (16) (http://www.nitrc.org/projects/gretna/). All the selected MRI scans were exported in Digital Imaging and Communications in Medicine format and then changed to Neuroimaging Informatics Technology Initiative format. Next, the images were preprocessed for correction as follows: (I) slice timing; (II) realignment; (III) normalization; (IV) spatially smoothing; (V) temporally detrending; (VI) regression; (VII) filtering. After preprocessing, the automated anatomical labeling (AAL) template was applied to partition the entire brain into 90 ROIs (17), from which the average BOLD signal time series were extracted and used to conduct the Pearson correlation analysis one by one. To ensure the FC values followed a more normal distribution, we further processed the data using the Fisher-z transform.
Machine learning
In this study, machine learning models based on linear discriminant analysis (LDA), logistic regression (LR), K-nearest neighbor (KNN), and support vector machine (SVM) models accomplished by MATLAB were used to classify the IBS patients and HCs. These algorithms are commonly used in supervised learning to map the relationship between input and output to predict or classify new unlabeled data. In this study, we employed the LIBSVM (18) library to train and test our SVM models. We extracted the FC matrix containing all the ROIs as data features and labeled each piece of data based on supervised learning to complete dataset partitioning. The data features were normalized to maintain consistency, and the optimal parameters of the model were determined using a leave-one-out cross validation method. At the same time, we used the F-score for feature filtering to remove highly correlated or unimportant features, thereby improving the generalizability of the models. Each subset was used once as a validation set, while the remaining subsets were used for training. This approach not only reduces the risk of overfitting, but also provides a more reliable estimate of the performance of the models across different data segments. Moreover, it ensures that every data point is involved in both training and validation, thereby enhancing the robustness of evaluation. The accuracy, sensitivity, specificity, the area under the curve (AUC), and the 95% confidence interval (CI) of the AUC were used to assess the model’s performance for classification. We employed the DeLong test to compare the AUCs of the different models, thereby identifying the machine-learning model with superior performance. Subsequently, we used a permutation test to assess whether the model’s performance was significantly better than random guessing. In this process, the dataset labels were randomly shuffled 1,000 times, and the models were trained using the permuted data. The performance of the baseline model was then compared to the distribution of performances obtained from the permuted data. If the baseline model’s performance was significantly better than that of the permuted models, it indicated that the model had indeed captured patterns in the data rather than random phenomenon. Finally, the weights of important neuroimaging features were calculated to search for areas that had a significant effect on model establishment. Brain regions with higher weights were displayed through BrainNet Viewer (19) (www.nitrc.org/projects/bnv). The entire process is shown in Figure 2.
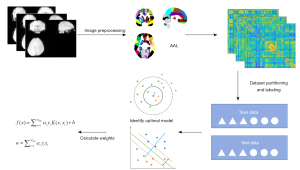
Statistical analysis
The data in this study were analyzed using statistical software SPSS (Windows, version 26.0). General demographic data and the MoCA scale scores of the IBS and HCs groups were used as econometric data. Therefore, variables that conformed to a normal distribution were subjected to two independent sample t-tests, while variables that did not conform to a normal distribution were subjected to the Mann-Whitney U test for the statistical analysis. Continuous variables are presented as the mean ± standard deviation. All the tests were two-sided, and a P value <0.05 indicated that the difference was statistically significant.
Results
Demographics and cognitive neuropsychological characteristics
The demographics and cognitive neuropsychological characteristics of the IBS patients and HCs are shown in Table 1. No significant differences were found between the two groups in terms of gender, age, or education level (P>0.05). The average MoCA total score of the IBS patient group was 25.80±2.58 (mean ± standard deviation), which was significantly lower than that of the HCs group (P<0.001).
Table 1
Protocols | IBS patients (n=88) | HCs (n=88) | P value |
---|---|---|---|
Gender (male/female) | 60/28 | 60/28 | 1.000 |
Age (years) | 32.35±9.02 | 32.44±9.04 | 0.947 |
Education (years) | 13.31±3.03 | 13.98±2.69 | 0.122 |
MoCA score | 25.80±2.58 | 27.43±1.87 | <0.001* |
Continuous variables are expressed as the mean ± standard deviation. *, P<0.001. IBS, irritable bowel syndrome; HCs, healthy controls; MoCA, Montreal Cognitive Assessment.
Model filtering
We plotted receiver operating characteristic (ROC) curves for different classification models to evaluate their performance (Figure 3). The classification accuracy of the LDA, LR, KNN, and SVM supervised learning models was 58.52%, 59.09%, 63.64%, and 75.00%, respectively. The AUC values were 0.5802 (95% CI: 0.4599–0.7005), 0.5887 (95% CI: 0.4692–0.7081), 0.6351 (95% CI: 0.5209–0.7492), and 0.7788 (95% CI: 0.6861–0.8715), respectively. The SVM model had significantly higher classification accuracy and performed significantly better than the other models (P<0.05). The significance of the performance indicators of the model, when randomly rearranging labels, was evaluated using permutation testing, and the calculated result was P<0.01.
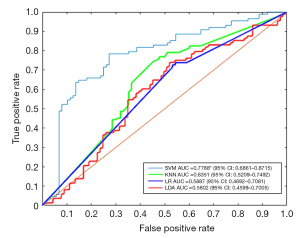
Weights of the machine-learning model outputs
We obtained the weight coefficient matrix of the support vector from the SVM model with the highest classification accuracy (Figure 4). According to the arrangement of the absolute weight values, we found that the important areas for model classification decisions included the Rolandic operculum (ROL) to the anterior cingulate gyrus (ACG), the calcarine sulcus (CAL) to the triangular part of the inferior frontal gyrus (IFG), the gyrus rectus (REC) to the inferior occipital gyrus (IOG), the lingual gyrus (LING) to the putamen (PUT), and the IOG to the angular gyrus (ANG). Among them, the classification weights of the ROL-ACG, CAL-IFG, and REC-IOG were positive, while the others were negative (Table 2, Figure 5).
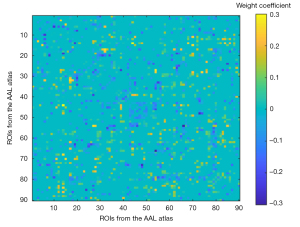
Table 2
FC brain regions | Weight direction (+/−) | Weight |
---|---|---|
ROL to ACG | + | 0.308960392 |
CAL to IFG | + | 0.273598007 |
REC to IOG | + | 0.271181849 |
LING to PUT | − | 0.277666647 |
IOG to ANG | − | 0.256528215 |
SVM, support vector machine; FC, functional connectivity; ROL, Rolandic operculum; ACG, anterior cingulate gyrus; CAL, calcarine sulcus; IFG, triangular part of the inferior frontal gyrus; REC, gyrus rectus; IOG, inferior occipital gyrus; LING, lingual gyrus; PUT, putamen; ANG, angular gyrus.
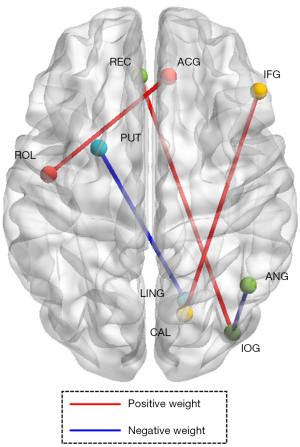
Discussion
The present study used the MoCA scale to evaluate the overall cognitive function of IBS patients. The analysis results indicated that the IBS patients exhibited mild cognitive impairment, which is consistent with the reports of previous studies (20-22). To further explore the neuroimaging characteristics and changes in the neural networks of IBS patients, this study focused on the static FC status of the whole brain in the IBS patients, using the correlations between different regions in the brain as features in an attempt to effectively distinguish between IBS patients and HCs from the perspective of neuroimaging.
Rs-fMRI is a technique that can measure the FC patterns of the brain in a resting state, helping to reveal the relationships and activity patterns between brain networks. A previous study found abnormal changes in brain regions related to emotion, cognitive assessment, pain perception, and visceral perception in IBS patients by analyzing the regional homogeneity of spontaneous brain activity in patients (23). In addition, another study found that IBS patients have brain regions with changes in the amplitude of low-frequency fluctuation (ALFF) values, and found a correlation between the ALFF values and disease duration, as well as increased functional connections between the cingulate and frontal cortex (24). FC reflects the functional network structure of the brain by measuring the synchronicity between different brain regions, and provides clues for disease diagnosis and research (25), which is a very important research direction in rs-fMRI. We constructed a whole brain FC matrix in which each element represents the correlation between two specific brain regions to comprehensively and intuitively describe the functional collaborations between different brain regions in a resting state. By comparing IBS patients with HCs, the FC matrix was able to identify changes in the brain functional network under disease conditions and provide sufficient neuroimaging features for subsequent machine learning.
Machine-learning technology can use a large amount of neuroimaging data to establish models for the diagnosis and classification of brain diseases, thereby improving the efficacy of disease diagnosis. Currently, research has used rs-FC of the habenula-dorsolateral prefrontal cortex, habenula-thalamus, and habenula-periaqueductal grey for SVM-based classification machine learning, with an independent subject queue validation accuracy of 65.2% (26). The present study found that the SVM is currently the best model for using whole brain rs-FC to distinguish IBS from HCs, with an accuracy of 75%, and its results were validated by permutation tests. The sensitivity of 62.7% reported in the literature suggests that the Rome IV criteria may fail to diagnose a significant number of true IBS cases. However, its high specificity of 97.1% indicates that the positive diagnoses are highly reliable (27). Therefore, we believe that machine-learning models optimized through hyperparameter tuning could help to reduce missed diagnoses and improve diagnostic efficacy for IBS patients. As a commonly used supervised learning algorithm for classification and regression analysis, the SVM aims to find the optimal hyperplane in the feature space (18,28). It can efficiently classify in high-dimensional space and has good robustness against outliers. Due to the lack of specific biomarkers that can accurately diagnose IBS, we believe that brain rs-FC has significant potential as a future neuroimaging biomarker by using SVM for classification.
After identifying the current optimal model SVM, we extracted the weight coefficients from the model’s decision function to understand which brain regions had a greater effect on classification decisions. To a certain extent, weight coefficients reflect the importance of each feature to the classification task, and features with larger absolute values have greater influence in classification decision making. The sign of the weight can indicate a positive or negative relationship between the corresponding feature and the decision. Positive weights indicate that as the feature increases, the decision function value increases, while negative weights indicate the opposite. We found that some regions have a profound effect on the construction of classification models, and these regions are likely important brain regions that differ between IBS patients and healthy populations.
We found from the weight matrix that the ROL-ACG is the FC region with the highest absolute weight coefficient. The ROL is mainly located between the frontal lobe, parietal lobe, and temporal lobe, and plays an important role in the cortical layer of the brain, including a portion of the motor cortex and sensory cortex (29). The ACG belongs to a part of the subcortical gray matter, and plays an important role in emotional regulation, especially in dealing with negative emotions and resolving emotional conflicts (30). The ROL may be related to emotional experience and expression, and participates in the neural circuit of emotional regulation through its connection with ACG (31). In addition, the ACG is an important component of pain perception and pain regulation, related to pain related emotional experiences and cognitive control (32). The ROL is also involved in pain perception processing, and the connection between the ROL-ACG may regulate pain perception and trigger emotional responses. As a functional gastrointestinal disease, previous understandings of IBS have emphasized the impact of emotional regulation and pain perception on IBS, both in terms of pathogenesis and treatment interventions (33). Most IBS patients will experience corresponding gastrointestinal symptoms when they feel uncomfortable and emotionally tense (34). Similarly, the REC is associated with the prefrontal cortex, which is an important area for emotional regulation and cognitive control. A previous study indicated that the IOG may also be involved in the process of emotional processing (35). The REC-IOG connection may be involved in regulating the integration and processing of emotional and cognitive information. The specific connectivity properties and roles between the CAL-IFG have not been fully explored, but in terms of two brain regions alone, some studies suggest that the CAL may also be involved in emotional processing, especially those related to emotional perception and regulation (36). At the same time, fMRI technology has been used to study neural networks in emotional regulation, and it has been found that the IFG plays an important role in emotional reevaluation and separation (37). Thus, we believe that the FC between CAL-IFG is also a key neural circuit for emotional cognitive work. Stress and emotions may affect intestinal motility, secretion, and sensation through the release of neurotransmitters, such as serotonin, dopamine, and adrenaline, exacerbating the clinical symptoms of IBS. Therefore, we believe that the SVM can use these brain regions associated with the pathogenesis of IBS to classify IBS patients and HCs. Machine-learning models are not only capable of identifying abnormal activity in these regions but can also further quantify their importance in the diagnosis of IBS.
In the superior classification models studied here, the FC of the LING-PUT and the IOG-ANG belong to regions with higher absolute negative weights, indicating that as the values of these features increase, the SVM model tends to classify the data into opposite categories. The specific functions of the neural circuits between the LING-PUT and IOG-ANG have not been explicitly detailed; however, from a functional perspective, both types of FC belong to brain regions involved in visuomotor information processing (38,39). To date, current scientific research has not clearly indicated any impairment of visual motor function in IBS patients. Therefore, our results suggest that abnormal fluctuations in these neural circuits can affect the misclassification of classification models. In the future development of IBS machine-learning models, it may be necessary to filter out FC features that are not closely related to the pathogenesis of IBS to improve the performance of classification models.
With the advancement of technology, artificial intelligence (AI) and advanced deep-learning techniques have gradually been applied in the field of neuroimaging. Deep-learning algorithms can more accurately identify and classify complex patterns of brain activity, assisting researchers to gain a deeper understanding of the brain’s operational principles (40). Further, the advantages of AI technology in large-scale data processing enable researchers to effectively handle and analyze extensive neuroimaging data, thereby discovering underlying patterns and trends (41). However, the SVM model used in our study typically performed better than deep-learning methods on small datasets. Deep-learning models require substantial data for training, while SVMs demonstrate remarkable performance even with limited samples. Due to its use of the structural risk minimization principle rather than empirical risk minimization, SVMs exhibit a superior generalization capability in handling small-sample data, effectively mitigating overfitting. Conversely, deep-learning models necessitate extensive hyperparameter tuning, while SVM model construction primarily focuses on selecting appropriate kernel functions and adjusting regularization parameters, streamlining parameter adjustment and model selection. Although deep-learning techniques were not directly applied to model the fMRI data of IBS patients in this study, the extracted whole-brain FC matrix belongs to two-dimensional data, and the SVM can clearly display the feature data that plays a key role in the classification results
This study had several limitations. First, the sample size of this study was relatively limited, the machine-learning algorithms used may be affected by overfitting, and rs-FC ignores the dynamic changes in the brain. To validate our findings, further improve the relevant models, and ensure their generalizability across IBS patients, future multicenter studies with larger sample sizes need to be conducted using more complex algorithms and combining static and dynamic functional connections. Second, this study used the AAL atlas to segment fMRI data with a scanning layer thickness of 6 mm, which may have drawbacks such as low resolution and dependence on anatomical brain structures. We acknowledge the potential limitations of using a 1.5-T magnetic field, but we believe that our imaging protocol was robust, yielding meaningful results. Future research could employ fMRI sequences with thinner scanning layers and use the Schaefer atlas for segmentation to achieve a more refined and detailed analysis of brain functional zoning in IBS patients. In addition, in the current research model, the data preprocessing still requires a great deal time and significant effort to extract BOLD signal time series. In clinical practice, it is much more useful to deploy a model that works with the entire MRI than requiring doctors to extract BOLD signal time series. In future research, deep-learning techniques, such as convolutional neural networks, could be used to identify specific regions in images, ensuring the model’s performance in clinical evaluations. Third, this was a cross-sectional and machine learning study, long-term longitudinal studies could help exploring the changes in brain connectivity patterns and better understanding the disease development trajectory of IBS.
Conclusions
This study employed a supervised learning approach to determine that the optimal machine-learning model for effectively classifying IBS patients and HCs based on whole-brain rs-FC features is SVM. By extracting the weights of the SVM model, specific brain regions contributing more significantly to the predictions were identified. We further explored these critical brain regions, highlighting the substantial influence of FC between emotion-related brain regions on model construction, which is closely related to the pathogenesis of the disease. Our study provides new directions for the clinical diagnosis and personalized treatment of IBS in the future. Whole-brain rs-FCs, as a classification feature in machine learning, has potential to achieve precision medicine.
Acknowledgments
We would like to sincerely thank all the participants in this study and the assistance from the Department of Gastroenterology, the First Affiliated Hospital of Shantou University Medical College.
Funding: This work was supported by the grants from
Footnote
Reporting Checklist: The authors have completed the TRIPOD+AI reporting checklist (available at https://qims.amegroups.com/article/view/10.21037/qims-24-892/rc).
Conflicts of Interest: All authors have completed the ICMJE uniform disclosure form (available at https://qims.amegroups.com/article/view/10.21037/qims-24-892/coif). All authors report that this work was supported by the grants from the National Natural Science Foundation of China (grant numbers: 82274657 and 82004468); the Natural Science Foundation of Guangdong Province of China (grant number: 2024A1515010813); and the China Postdoctoral Science Foundation (grant number 2019M663021). The authors have no other conflicts of interest to declare.
Ethical Statement: The authors are accountable for all aspects of the work in ensuring that questions related to the accuracy or integrity of any part of the work are appropriately investigated and resolved. This study was conducted in accordance with the Declaration of Helsinki (as revised in 2013). This study was approved by the Ethics Committee of the First Affiliated Hospital of Shantou University Medical College (No. B-2022-020). All participants gave written informed consent.
Open Access Statement: This is an Open Access article distributed in accordance with the Creative Commons Attribution-NonCommercial-NoDerivs 4.0 International License (CC BY-NC-ND 4.0), which permits the non-commercial replication and distribution of the article with the strict proviso that no changes or edits are made and the original work is properly cited (including links to both the formal publication through the relevant DOI and the license). See: https://creativecommons.org/licenses/by-nc-nd/4.0/.
References
- Oka P, Parr H, Barberio B, Black CJ, Savarino EV, Ford AC. Global prevalence of irritable bowel syndrome according to Rome III or IV criteria: a systematic review and meta-analysis. Lancet Gastroenterol Hepatol 2020;5:908-17. [Crossref] [PubMed]
- Margolis KG, Cryan JF, Mayer EA. The Microbiota-Gut-Brain Axis: From Motility to Mood. Gastroenterology 2021;160:1486-501. [Crossref] [PubMed]
- Forsythe P, Kunze W, Bienenstock J. Moody microbes or fecal phrenology: what do we know about the microbiota-gut-brain axis? BMC Med 2016;14:58. [Crossref] [PubMed]
- Zhang S, Chen F, Wu J, Liu C, Yang G, Piao R, Geng B, Xu K, Liu P. Altered structural covariance and functional connectivity of the insula in patients with Crohn's disease. Quant Imaging Med Surg 2022;12:1020-36. [Crossref] [PubMed]
- Mertz H, Morgan V, Tanner G, Pickens D, Price R, Shyr Y, Kessler R. Regional cerebral activation in irritable bowel syndrome and control subjects with painful and nonpainful rectal distention. Gastroenterology 2000;118:842-8. [Crossref] [PubMed]
- Naliboff BD, Derbyshire SW, Munakata J, Berman S, Mandelkern M, Chang L, Mayer EA. Cerebral activation in patients with irritable bowel syndrome and control subjects during rectosigmoid stimulation. Psychosom Med 2001;63:365-75. [Crossref] [PubMed]
- Zang YF, He Y, Zhu CZ, Cao QJ, Sui MQ, Liang M, Tian LX, Jiang TZ, Wang YF. Altered baseline brain activity in children with ADHD revealed by resting-state functional MRI. Brain Dev 2007;29:83-91. [Crossref] [PubMed]
- Biswal B, Yetkin FZ, Haughton VM, Hyde JS. Functional connectivity in the motor cortex of resting human brain using echo-planar MRI. Magn Reson Med 1995;34:537-41. [Crossref] [PubMed]
- Chen XF, Guo Y, Lu XQ, Qi L, Xu KH, Chen Y, Li GX, Ding JP, Li J. Aberrant Intraregional Brain Activity and Functional Connectivity in Patients With Diarrhea-Predominant Irritable Bowel Syndrome. Front Neurosci 2021;15:721822. [Crossref] [PubMed]
- Cabitza F, Rasoini R, Gensini GF. Unintended Consequences of Machine Learning in Medicine. JAMA 2017;318:517-8. [Crossref] [PubMed]
- Forsting M. Machine Learning Will Change Medicine. J Nucl Med 2017;58:357-8. [Crossref] [PubMed]
- Drossman DA, Hasler WL. Rome IV-Functional GI Disorders: Disorders of Gut-Brain Interaction. Gastroenterology 2016;150:1257-61. [Crossref] [PubMed]
- Nasreddine ZS, Phillips NA, Bédirian V, Charbonneau S, Whitehead V, Collin I, Cummings JL, Chertkow H. The Montreal Cognitive Assessment, MoCA: a brief screening tool for mild cognitive impairment. J Am Geriatr Soc 2005;53:695-9. [Crossref] [PubMed]
- Yu J, Li J, Huang X. The Beijing version of the Montreal Cognitive Assessment as a brief screening tool for mild cognitive impairment: a community-based study. BMC Psychiatry 2012;12:156. [Crossref] [PubMed]
- Chang Y, Woo ST, Kim Y, Lee JJ, Song HJ, Lee HJ, Kim SH, Lee H, Kwon YJ, Ahn JH, Park SJ, Chung IS, Jeong KS. Pallidal index measured with three-dimensional T1-weighted gradient echo sequence is a good predictor of manganese exposure in welders. J Magn Reson Imaging 2010;31:1020-6. [Crossref] [PubMed]
- Wang J, Wang X, Xia M, Liao X, Evans A, He Y. GRETNA: a graph theoretical network analysis toolbox for imaging connectomics. Front Hum Neurosci 2015;9:386. [Crossref] [PubMed]
- Tzourio-Mazoyer N, Landeau B, Papathanassiou D, Crivello F, Etard O, Delcroix N, Mazoyer B, Joliot M. Automated anatomical labeling of activations in SPM using a macroscopic anatomical parcellation of the MNI MRI single-subject brain. Neuroimage 2002;15:273-89. [Crossref] [PubMed]
- Chang CC, Lin CJ. LIBSVM: a library for support vector machines. ACM transactions on intelligent systems and technology (TIST) 2011;2:1-27.
- Xia M, Wang J, He Y. BrainNet Viewer: a network visualization tool for human brain connectomics. PLoS One 2013;8:e68910. [Crossref] [PubMed]
- Aziz MNM, Kumar J, Muhammad Nawawi KN, Raja Ali RA, Mokhtar NM. Irritable Bowel Syndrome, Depression, and Neurodegeneration: A Bidirectional Communication from Gut to Brain. Nutrients 2021;31:3061. [Crossref] [PubMed]
- Labus JS, Hubbard CS, Bueller J, Ebrat B, Tillisch K, Chen M, Stains J, Dukes GE, Kelleher DL, Naliboff BD, Fanselow M, Mayer EA. Impaired emotional learning and involvement of the corticotropin-releasing factor signaling system in patients with irritable bowel syndrome. Gastroenterology 2013;145:1253-61.e1-3.
- Seminowicz DA, Labus JS, Bueller JA, Tillisch K, Naliboff BD, Bushnell MC, Mayer EA. Regional gray matter density changes in brains of patients with irritable bowel syndrome. Gastroenterology 2010;139:48-57.e2. [Crossref] [PubMed]
- Ke J, Qi R, Liu C, Xu Q, Wang F, Zhang L, Lu G. Abnormal regional homogeneity in patients with irritable bowel syndrome: A resting-state functional MRI study. Neurogastroenterol Motil 2015;27:1796-803. [Crossref] [PubMed]
- Ma X, Li S, Tian J, Jiang G, Wen H, Wang T, Fang J, Zhan W, Xu Y. Altered brain spontaneous activity and connectivity network in irritable bowel syndrome patients: A resting-state fMRI study. Clin Neurophysiol 2015;126:1190-7. [Crossref] [PubMed]
- Sporns O. Network attributes for segregation and integration in the human brain. Curr Opin Neurobiol 2013;23:162-71. [Crossref] [PubMed]
- Mao CP, Chen FR, Huo JH, Zhang L, Zhang GR, Zhang B, Zhou XQ. Altered resting-state functional connectivity and effective connectivity of the habenula in irritable bowel syndrome: A cross-sectional and machine learning study. Hum Brain Mapp 2020;41:3655-66. [Crossref] [PubMed]
- Palsson OS, Whitehead WE, van Tilburg MA, Chang L, Chey W, Crowell MD, Keefer L, Lembo AJ, Parkman HP, Rao SS, Sperber A, Spiegel B, Tack J, Vanner S, Walker LS, Whorwell P, Yang Y. Rome IV Diagnostic Questionnaires and Tables for Investigators and Clinicians. Gastroenterology 2016; Epub ahead of print. [Crossref]
- Cao LJ, Tay FH. Support vector machine with adaptive parameters in financial time series forecasting. IEEE Trans Neural Netw 2003;14:1506-18. [Crossref] [PubMed]
- Triarhou LC. Cytoarchitectonics of the Rolandic operculum: morphofunctional ponderings. Brain Struct Funct 2021;226:941-50. [Crossref] [PubMed]
- Bian XL, Qin C, Cai CY, Zhou Y, Tao Y, Lin YH, Wu HY, Chang L, Luo CX, Zhu DY. Anterior Cingulate Cortex to Ventral Hippocampus Circuit Mediates Contextual Fear Generalization. J Neurosci 2019;39:5728-39. [Crossref] [PubMed]
- Koelsch S, Fritz T, V, Cramon DY, Müller K, Friederici AD. Investigating emotion with music: an fMRI study. Hum Brain Mapp 2006;27:239-50. [Crossref] [PubMed]
- Bliss TV, Collingridge GL, Kaang BK, Zhuo M. Synaptic plasticity in the anterior cingulate cortex in acute and chronic pain. Nat Rev Neurosci 2016;17:485-96. [Crossref] [PubMed]
- Mayer EA, Naliboff BD, Craig AD. Neuroimaging of the brain-gut axis: from basic understanding to treatment of functional GI disorders. Gastroenterology 2006;131:1925-42. [Crossref] [PubMed]
- Drossman DA. Functional Gastrointestinal Disorders: History, Pathophysiology, Clinical Features and Rome IV. Gastroenterology 2016; Epub ahead of print. [Crossref]
- Picó-Pérez M, Radua J, Steward T, Menchón JM, Soriano-Mas C. Emotion regulation in mood and anxiety disorders: A meta-analysis of fMRI cognitive reappraisal studies. Prog Neuropsychopharmacol Biol Psychiatry 2017;79:96-104. [Crossref] [PubMed]
- Lindquist KA, Wager TD, Kober H, Bliss-Moreau E, Barrett LF. The brain basis of emotion: a meta-analytic review. Behav Brain Sci 2012;35:121-43. [Crossref] [PubMed]
- Kanske P, Heissler J, Schönfelder S, Bongers A, Wessa M. How to regulate emotion? Neural networks for reappraisal and distraction. Cereb Cortex 2011;21:1379-88. [Crossref] [PubMed]
- Saygin ZM, Osher DE, Norton ES, Youssoufian DA, Beach SD, Feather J, Gaab N, Gabrieli JD, Kanwisher N. Connectivity precedes function in the development of the visual word form area. Nat Neurosci 2016;19:1250-5. [Crossref] [PubMed]
- Grill-Spector K, Malach R. The human visual cortex. Annu Rev Neurosci 2004;27:649-77. [Crossref] [PubMed]
- Zhang X, Yao L, Wang X, Monaghan J, McAlpine D, Zhang Y. A survey on deep learning-based non-invasive brain signals: recent advances and new frontiers. J Neural Eng 2021;
- Chen ZS, Kulkarni PP, Galatzer-Levy IR, Bigio B, Nasca C, Zhang Y. Modern views of machine learning for precision psychiatry. Patterns (N Y) 2022;3:100602. [Crossref] [PubMed]