Tumour-pleura relationship on computed tomography (CT) provides effective risk stratification for peripheral pulmonary nodules with Lung Imaging Reporting and Data System (Lung-RADS) score of 4X
Introduction
Low-dose computed tomography (LDCT) is the primary screening method for early detection of lung cancer and can reduce mortality in certain high-risk populations (1,2). To standardize the image reporting of pulmonary nodules and management of pulmonary nodule patients, the American College of Radiology developed the Lung Imaging Reporting and Data System (Lung-RADS) based on the characteristics of pulmonary nodules (such as size, attenuation, and growth pattern) to stratify the risk of lesions (3). Category 4X is a special category of lesions that exhibit additional features and imaging findings and represents the highest risk of malignant tumours, with a malignancy rate greater than 15% (4,5). In addition, most of the lung cancer detected by screening belong to pulmonary nodules with a Lung-RADS score of 4X, which makes these nodules highly clinically significant (6).
Among Lung-RADS 4X pulmonary nodules, the most common pathological type of benign pulmonary nodule is tuberculous granuloma, which is challenging to distinguish due to its similar imaging features to lung cancer (7). Accurate identification of the two can guide clinicians to make reasonable and effective treatment and prevent patients from receiving overtreatment or losing the optimal treatment time due to delayed treatment (8). Lung adenocarcinoma is the most common pathological type of peripheral lung cancer, and visceral pleural invasion (VPI) is an important prognostic factor and staging index (9). For patients with early-stage peripheral lung adenocarcinoma, the five-year survival rate is 86% for patients without VPI, compared with 57–70% for patients with VPI (10). In addition, in the presence of VPI, extensive surgery and adjuvant chemotherapy can significantly improve patient survival outcomes (11-13). Therefore, accurate differentiation of pathological types and VPI of Lung-RADS 4X peripheral pulmonary nodules before treatment can aid in stratification, and maximize the guarantee that patients receive reasonable and accurate treatment.
Several previous studies have reported that the relationship between peripheral lung cancer and the pleura plays an important role in further risk stratification of lesions (9,14). However, whether the relationship between peripheral pulmonary nodules of Lung-RADS 4X and the pleura can provide an important basis for classifying pulmonary nodules and VPI has not been explored by scholars. Therefore, the aim of this study was to confirm whether the relationship between lesion and pleura could provide valid risk stratification for Lung-RADS 4X peripheral pulmonary nodules. We present this article in accordance with the STROBE reporting checklist (available at https://qims.amegroups.com/article/view/10.21037/qims-24-530/rc).
Methods
Patients
This retrospective study was approved by the ethics committee of Lanzhou University Second Hospital (No. 2023A-745), and the requirement for informed consent was waived. The study was conducted in accordance with the Declaration of Helsinki (as revised in 2013). A total of 838 patients with pathologically confirmed diagnosis of tuberculous granuloma and lung adenocarcinoma who underwent computed tomography (CT) scanning from January 2019 to December 2023 were retrospectively enrolled in this study. The exclusion criteria were as follows: (I) Lung-RADS scores for pulmonary nodules of other categories except 4X; (II) central pulmonary nodules located in the segmental bronchi and above (main bronchi, lobar bronchi); (III) pulmonary nodules in contact with the pleura on CT images; (IV) patients with multiple pulmonary nodules; (V) patients who received preoperative anti-inflammatory, antituberculosis or antitumor therapy; (VI) incomplete clinical or imaging data; (VII) poor image quality not meeting the research requirements. Finally, a total of 482 patients were included in our study. The inclusion and exclusion criteria are shown in Figure 1. The Lung-RADS v2022 is used for the management of indeterminate pulmonary nodules. For pulmonary nodules with Lung-RADS score of 4X, the following is recommended: (I) diagnostic chest CT with or without contrast; (II) positron emission tomography (PET)/CT may be considered if there is a ≥8 mm (≥268 mm3) solid nodule or solid component; (III) tissue sampling; (IV) and/or referral for further clinical evaluation. Clinical data including gender, age and lung cancer tumour markers [carcinoembryonic antigen (CEA), neuron-specific enolase (NSE), cytokeratin fragment 19 (CYFRA21-1), pro-gastrin-releasing peptide (ProGR), and squamous cell carcinoma antigen (SCCA)] were collected.
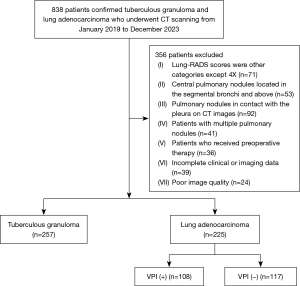
CT protocol
All patients underwent preoperative chest CT scanning using multi-slice spiral CT scanners (Discovery CT 750 HD and Revolution CT, GE Healthcare, USA; Somatom Force, Siemens Healthcare, Germany; iCT 256, Philips Healthcare, Amsterdam, USA). The patient was trained to breathe before the scan and was instructed to hold his or her breath as long as possible after inhalation during the scan. The patient was placed in the supine position on the CT scanning bed, head first, with arms straight and raised above the head. CT scanning range was as follows: thoracic entrance to the lower margin of diaphragm. The tube voltage was 120 kVp, the tube current range was 150–200 mm, the scanning layer thickness and scanning spacing were both 5 mm, and the reconstruction layer thickness and layer spacing were both 1.25 mm.
Image analysis
Two chest radiologists with 6 and 10 years of experience independently collected clinical data and evaluated the CT images without knowing the histopathological results. The following CT data were collected: (I) type of nodule (solid, subsolid and ground-glass nodule); (II) maximum diameter of the nodule; (III) maximum diameter of solid component; (IV) type of linear connection between lesion and pleura (single line or multiple lines); (V) length of the line connecting the lesion to the pleura (shortest line length measured on axial, sagittal or coronal images); (VI) type of adjacent pleura (interlobular pleura, peripheral pleura or both); (VII) presence of soft tissue components on the pleura side, and measurement of the maximum base width of soft tissue components on the pleura side if present (Figure 2). All CT images were observed on the lung and mediastinal windows. In case of disagreements during the analysis, the authors would discuss and come to a consensus.
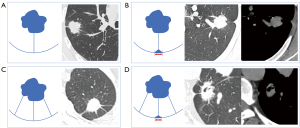
Statistical analysis
SPSS 26.0 (IBM Corp, USA), R software (version 4.2.6) and MedCalc software (version 4.2.6) were used for statistical analysis. Kappa and the intraclass correlation coefficient (ICC) were used to analyze the consistency between classifications and continuous variables obtained by two radiologists. The Shapiro-Wilk test was used to analyze the normality of continuous variables. Two-sample t-test and Mann-Whitney U test were used to compare the differences between the two groups of continuous variables. The Chi-square or Fisher’s exact probability test was used to compare the difference between the two groups of categorical variables. Multivariate logistic regression model was used to screen for statistically significant variables, a predictive model was developed and the model was visualized as a nomogram. ROC curves were used to evaluate the predictive efficiency of each independent predictor and the combined model.
Results
Patient characteristics
Table 1 summarizes the detailed information of the 482 patients with Lung-RADS 4X, 257 patients with tuberculous granuloma and 225 patients with lung adenocarcinoma were included in our study, where in lung adenocarcinoma included 108 patients with presence of VPI and 117 patients without VPI. This study included 282 males and 200 females with a mean age of 57.90±10.51 years. Gender and CEA level were significantly different between tuberculous granuloma and adenocarcinoma patients (P<0.001), while age and other tumour markers (NSE, CYFRA21-1, ProGR, and SCCA) were not significantly different between the two groups (P>0.05). In terms of VPI, only CEA was significantly different between the two groups (P=0.021).
Table 1
Variables | Tuberculous granuloma (n=257) | Lung adenocarcinoma (n=225) | Lung adenocarcinoma (n=225) | P1 | P2 | |
---|---|---|---|---|---|---|
VPI (+) (n=108) | VPI (−) (n=117) | |||||
Gender | <0.001 | 0.410 | ||||
Male | 178 (69.3) | 104 (46.2) | 53 (49.1) | 51 (43.6) | ||
Female | 79 (30.7) | 121 (53.8) | 55 (50.9) | 66 (56.4) | ||
Age (years) | 53.00 (45.00, 60.50) | 58.00 (52.50, 65.00) | 57.06±8.47 | 59.07±9.61 | <0.001 | 0.098 |
Tumor markers | ||||||
CEA | <0.001 | 0.021 | ||||
Positive | 22 (8.6) | 61 (27.1) | 38 (35.2) | 25 (21.4) | ||
Negative | 235 (91.4) | 164 (72.9) | 70 (64.8) | 92 (78.6) | ||
NSE | 0.273 | 0.402 | ||||
Positive | 23 (8.9) | 27 (12.0) | 15 (13.9) | 12 (10.3) | ||
Negative | 234 (91.1) | 198 (88.0) | 93 (86.1) | 105 (89.7) | ||
ProGRP | 0.949 | 0.142 | ||||
Positive | 52 (20.2) | 45 (20.0) | 26 (24.1) | 19 (16.2) | ||
Negative | 205 (79.8) | 180 (80.0) | 82 (75.9) | 98 (83.8) | ||
SCCA | 1.000 | 1.000 | ||||
Positive | 5 (1.9) | 4 (1.8) | 2 (1.9) | 2 (1.7) | ||
Negative | 252 (98.1) | 221 (98.2) | 106 (98.1) | 115 (98.3) | ||
CYFRA21-1 | 0.649 | 0.233 | ||||
Positive | 34 (13.2) | 33 (14.7) | 19 (17.6) | 14 (12.0) | ||
Negative | 223 (86.8) | 192 (85.3) | 89 (82.4) | 103 (88.0) | ||
N stage | – | 0.12 | ||||
N0 | – | – | 94 (87.0) | 109 (93.2) | ||
N1 | – | – | 14 (13.0) | 8 (6.8) | ||
M stage | – | 0.27 | ||||
M0 | – | – | 99 (91.7) | 112 (95.7) | ||
M1 | – | – | 9 (8.3) | 5 (4.3) |
Normally distributed variables were expressed as mean ± standard deviations and non-normally distributed variables as medians (Q1, Q3), categorical variables were expressed as count (percentage). CEA, carcinoembryonic antigen; NSE, neuron-specific enolase; ProGR, pro-gastrin-releasing peptide; SCCA, squamous cell carcinoma antigen; CYFRA21-1, cytokeratin fragment 19; VPI, visceral pleural invasion; P1, tuberculous granuloma vs. lung adenocarcinoma; P2, visceral pleural invasion positive vs. visceral pleural invasion negative.
Univariate analysis of conventional CT signs
The interobserver agreement between the two radiologists in analyzing the CT signs was good (κ=0.92–0.99; ICC =0.86–0.94). In the differentiation of tuberculous granuloma and adenocarcinoma with Lung-RADS 4X, there were significant differences in type of nodules, type of linear connection between lesion and pleura, presence of soft tissue components on the pleura side and the maximum base width of soft tissue components on the pleura side (P values were <0.001, 0.001, <0.001, and <0.001, respectively). There were no significant differences in the maximum diameter of nodules, the maximum diameter of solid components, length of the line connecting the lesion to the pleura, the type of adjacent pleura and CT signs of lung nodule itself (calcification, cavity, air bronchogram, lobulation sign, spiculation, bubblelike lucency, necrosis and CT value) between them (P>0.05) (Table 2).
Table 2
Variables | Tuberculous granuloma (n=257) | Lung adenocarcinoma (n=225) | Lung adenocarcinoma (n=225) | P1 | P2 | |
---|---|---|---|---|---|---|
VPI (+) (n=108) | VPI (−) (n=117) | |||||
Type of nodules | <0.001 | 0.005 | ||||
Solid | 243 (94.6) | 129 (57.3) | 71 (65.7) | 58 (49.6) | ||
Subsolid | 12 (4.7) | 85 (37.8) | 36 (33.3) | 49 (41.9) | ||
Ground-glass | 2 (0.8) | 11 (4.9) | 1 (0.9) | 10 (8.5) | ||
Maximum diameter of the nodule (mm) | 19.41 (16.54, 23.23) | 19.41 (17.37, 23.01) | 23.89±6.59 | 21.88±8.46 | 0.906 | 0.049 |
Calcification | 0.11 | 0.99 | ||||
Yes | 44 (17.1) | 27 (12.0) | 13 (12.0) | 14 (12.0) | ||
No | 213 (82.9) | 198 (88.0) | 95 (88.0) | 103 (88.0) | ||
Cavity | 0.84 | 0.28 | ||||
Yes | 36 (14.0) | 33 (14.7) | 13 (12.0) | 20 (17.1) | ||
No | 221 (86.0) | 192 (85.3) | 95 (88.0) | 97 (82.9) | ||
Air bronchogram | 0.51 | 0.43 | ||||
Yes | 52 (20.2) | 51 (22.7) | 22 (20.4) | 29 (24.8) | ||
No | 205 (79.8) | 174 (77.3) | 86 (79.6) | 88 (75.2) | ||
Lobulation sign | 0.26 | 0.09 | ||||
Yes | 117 (45.5) | 114 (50.7) | 61 (56.5) | 53 (45.3) | ||
No | 140 (54.5) | 111 (49.3) | 47 (43.5) | 64 (54.7) | ||
Spiculation | 0.07 | 0.33 | ||||
Yes | 103 (40.1) | 109 (48.4) | 56 (51.9) | 53 (45.3) | ||
No | 154 (59.9) | 101 (51.6) | 52 (48.1) | 64 (54.7) | ||
Bubblelike lucency | 0.66 | 0.36 | ||||
Yes | 101 (39.3) | 84 (37.3) | 37 (34.3) | 47 (40.2) | ||
No | 156 (60.7) | 141 (62.7) | 71 (65.7) | 70 (59.8) | ||
Necrosis | 0.49 | 0.37 | ||||
Yes | 29 (11.3) | 23 (10.2) | 9 (8.3) | 14 (12.0) | ||
No | 228 (88.7) | 202 (89.8) | 99 (91.7) | 103 (88.0) | ||
CT value (HU) | 37 (32, 43) | 36 (33, 42) | 37.72±7.13 | 36.06±5.97 | 0.13 | 0.07 |
Maximum diameter of solid component (mm) | 18.96 (16.05, 23.01) | 18.78 (12.00, 23.51) | 20.86 (15.86, 26.3) | 16.27 (10.28, 20.98) | 0.093 | <0.001 |
Type of linear connection | 0.001 | <0.001 | ||||
Single line | 37 (14.4) | 59 (26.2) | 16 (14.8) | 53 (45.3) | ||
Multiple line | 220 (85.6) | 166 (73.8) | 92 (85.2) | 64 (54.7) | ||
Length of the line (mm) | 7.63 (4.95, 10.47) | 6.87 (4.88, 10.21) | 6.87 (4.88, 10.21) | 7.63 (4.95, 10.47) | 0.082 | 0.599 |
Type of adjacent pleura | 0.166 | 0.036 | ||||
Interlobular pleura | 12 (4.7) | 15 (6.7) | 8 (7.4) | 7 (6.0) | ||
Peripheral pleura | 225 (87.5) | 183 (81.3) | 81 (75.0) | 102 (87.2) | ||
Both | 20 (7.8) | 27 (12.0) | 19 (17.6) | 8 (6.8) | ||
Soft tissue on the pleura side | <0.001 | 0.001 | ||||
Positive | 237 (92.2) | 181 (80.4) | 97 (89.8) | 84 (71.8) | ||
Negative | 20 (7.8) | 44 (19.6) | 11 (10.2) | 33 (28.2) | ||
Maximum base width of soft tissue (mm) | 4.83 (3.22, 7.10) | 3.39 (2.23, 5.45) | 4.31 (3.02, 6.25) | 2.62 (0.00, 4.45) | <0.001 | <0.001 |
Normally distributed variables were expressed as mean ± standard deviations and non-normally distributed variables as medians (Q1, Q3), categorical variables were expressed as count (percentage). VPI, visceral pleural invasion; P1, tuberculous granuloma vs. lung adenocarcinoma; P2, visceral pleural invasion positive vs. visceral pleural invasion negative; CT, computed tomography; HU, Hounsfield unit; Maximum base width of soft tissue, maximum base width of soft tissue components on the pleura side; Type of linear connection, type of linear connection between lesion and pleura; Length of the line, length of the line connecting the lesion to the pleura; Soft tissue on the pleura side, presence of soft tissue components on the pleura side.
In predicting VPI, there were statistically significant differences in the type of nodule, the maximum diameter of nodule, the maximum diameter of solid component, type of linear connection between lesion and pleura, type of adjacent pleura, presence of soft tissue components on the pleura side and the maximum base width of soft tissue components on the pleura side between them (P values were 0.005, 0.049, <0.001, <0.001, 0.036, 0.001 and <0.001, respectively), but there was no significant difference in length of the line connecting the lesion to the pleura and CT signs of lung nodule itself (calcification, cavity, air bronchogram, lobulation sign, spiculation, bubblelike lucency, necrosis and CT value) between the two (P>0.05) (Table 2).
In addition, type of nodule, type of linear connection between lesion and pleura, presence of soft tissue components on the pleura side and the maximum base width of soft tissue components on the pleura side were statistically different both in the classification of pulmonary nodules and VPI (P<0.05).
Multivariate logistic regression analysis
Multivariate logistic regression analysis revealed that gender [odds ratio (OR) =0.392; P<0.001], CEA level (OR =8.331; P<0.001), type of nodule (OR =13.551 and 7.478; P<0.001 and P=0.016) and the maximum base width of soft tissue components on the pleura side (OR =0.857; P=0.005) were independent risk factors for distinguishing lung adenocarcinoma from tuberculous granuloma. The variance inflation factor for the above six potential predictors ranged from 1.005–1.089, indicating the absence of multicollinearity. A nomogram was constructed using the six predictors above to provide a visual measure of outcomes (Table 3, Figure 3A).
Table 3
Variables | Multivariable | P | |
---|---|---|---|
OR | 95% CI | ||
Gender | |||
Male (vs. female) | 0.392 | 0.247–0.620 | <0.001 |
CEA | |||
Positive (vs. negative) | 8.331 | 4.486–15.472 | <0.001 |
Type of nodules | |||
Subsolid (vs. solid) | 13.551 | 6.871–26.727 | <0.001 |
Ground-glass (vs. solid) | 7.478 | 1.450–38.555 | 0.016 |
Type of linear connection | |||
Multiple (vs. single) | 0.627 | 0.358–1.099 | 0.103 |
Soft tissue on the pleura side | |||
Positive (vs. negative) | 1.08 | 0.444–2.630 | 0.865 |
Maximum base width of soft tissue (mm) | 0.857 | 0.769–0.954 | 0.005 |
OR, odds ratio; CI, confidence interval; CEA, carcinoembryonic antigen; Type of linear connection, type of linear connection between lesion and pleura; Soft tissue on the pleura side, presence of soft tissue components on the pleura side; Maximum base width of soft tissue, maximum base width of soft tissue components on the pleura side.
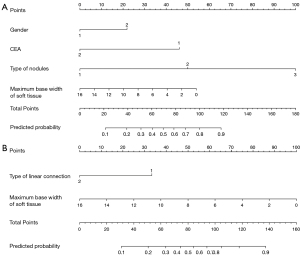
Multivariate logistic regression analysis was used to identify two independent predictors with variance inflation factors of 1.142 and 2.053. Multivariate analysis revealed that the type of linear connection between lesion and pleura (OR =3.936; P<0.001) and the maximum base width of soft tissue components on the pleura side (OR =1.359; P=0.001) were independently associated with VPI. A nomogram was constructed to visualize the results (Table 4, Figure 3B).
Table 4
Variables | Multivariable | P | |
---|---|---|---|
OR | 95% CI | ||
CEA | |||
Positive (vs. negative) | 1.362 | 0.691–2.686 | 0.374 |
Type of nodules | |||
Subsolid (vs. solid) | 0.839 | 0.407–1.728 | 0.633 |
Ground-glass (vs. solid) | 0.305 | 0.028–3.342 | 0.331 |
Maximum diameter of the nodule | 0.977 | 0.928–1.028 | 0.374 |
Maximum diameter of solid component (mm) | 1.010 | 0.977–1.044 | 0.570 |
Type of linear connection | |||
Multiple (vs. single) | 3.936 | 1.926–8.044 | <0.001 |
Type of adjacent pleura | |||
Peripheral (vs. interlobular) | 0.425 | 0.121–1.490 | 0.181 |
Both (vs. interlobular) | 0.912 | 0.198–4.208 | 0.907 |
Soft tissue on the pleura side | |||
Positive (vs. negative) | 1.827 | 0.580–5.755 | 0.303 |
Maximum base width of soft tissue (mm) | 1.359 | 1.135–1.628 | 0.001 |
OR, odds ratio; CI, confidence interval; CEA, carcinoembryonic antigen; Type of linear connection, type of linear connection between lesion and pleura; Soft tissue on the pleura side, presence of soft tissue components on the pleura side; Maximum base width of soft tissue, maximum base width of soft tissue components on the pleura side.
Diagnostic performance evaluation
ROC curve analysis showed that the area under the curve (AUC) of gender and CEA in differentiating adenocarcinoma from tuberculous granuloma was 61.52% [95% confidence interval (CI): 56.47–66.56%] and 60.30% (95% CI: 55.20–65.41%), respectively. The AUC of type of nodules and the maximum base width of soft tissue components on the pleura side were 68.58% (95% CI: 63.70–73.45%) and 65.14% (95% CI: 60.26–70.03%), respectively. When the maximum base width of soft tissue components on the pleura side was 3.16, the sensitivity and specificity were 45.78% (95% CI: 39.1–52.5%) and 78.21% (95% CI: 72.7–83.1%), respectively. A logistic regression combined model was established for the above statistically significant variables, and the AUC was 82.60% (95% CI: 78.85–86.35%%) (Table 5, Figure 4).
Table 5
Variables | AUC (95% CI), % | P value | YI | Cut-off | Sensitivity (95% CI), % |
Specificity (95% CI), % |
PLR (95% CI) |
NLR (95% CI) |
---|---|---|---|---|---|---|---|---|
Gender | 61.52 (56.47–66.56) | <0.001 | 0.23 | – | 53.78 (47.0–60.4) | 69.26 (63.2–74.8) | 1.75 (1.4–2.2) | 0.67 (0.6–0.8) |
CEA | 60.30 (55.20–65.41) | <0.001 | 0.21 | – | 28.00 (22.2–34.4) | 96.21 (88.7–95.5) | 3.79 (2.3–6.1) | 0.78 (0.7–0.8) |
Type of nodules | 68.58 (63.70–73.45) | <0.001 | 0.37 | – | 42.67 (36.1–49.4) | 94.55 (91.0–97.0) | 7.83 (4.6–13.3) | 0.61 (0.5–0.7) |
Maximum base width of soft tissue (mm) | 65.14 (60.26–70.03) | <0.001 | 0.24 | 3.16 | 45.78 (39.1–52.5) | 78.21 (72.7–83.1) | 2.10 (1.6–2.8) | 0.69 (0.6–0.8) |
Combined | 82.60 (78.85–86.35) | <0.001 | 0.54 | 0.46 | 70.67 (64.2–76.5) | 83.27 (78.1–87.6) | 4.22 (3.2–5.6) | 0.35 (0.3–0.4) |
AUC, area under the curve; CI, confidence interval; YI, Youden Index; PLR, positive likelihood ratio; NLR, negative likelihood ratio; CEA, carcinoembryonic antigen; Maximum base width of soft tissue, maximum base width of soft tissue components on the pleura side.
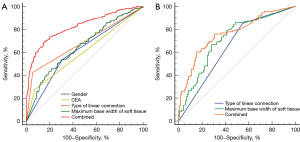
The AUC of the type of linear connection between lesion and pleura and the maximum base width of soft tissue components on the pleura side for predicting VPI were 65.24% (95% CI: 58.07–72.41%) and 71.31% (95% CI: 64.53–78.09%), respectively. When the maximum base width of soft tissue components on the pleura side was 3.51, the sensitivity and specificity were 66.67% (95% CI: 56.9–75.4%) and 71.79% (95% CI: 62.7–79.7%), respectively. A Logistic regression combined model was established with the AUC of 76.10% (95% CI: 69.83–82.38%) (Table 6, Figure 4).
Table 6
Variables | AUC (95% CI), % | P value | YI | Cut-off | Sensitivity (95% CI), % |
Specificity (95% CI), % |
PLR (95% CI) |
NLR (95% CI) |
---|---|---|---|---|---|---|---|---|
Type of linear connection | 65.24 (58.07–72.41) | <0.001 | 0.30 | – | 85.19 (77.1–91.3) | 45.30 (36.1–54.8) | 1.56 (1.3–1.9) | 0.33 (0.2–0.5) |
Maximum base width of soft tissue (mm) | 71.31 (64.53–78.09) | <0.001 | 0.38 | 3.51 | 66.67 (56.9–75.4) | 71.79 (62.7–79.7) | 2.36 (1.7–3.2) | 0.46 (0.3–0.6) |
Combined | 76.10 (69.83–82.38) | <0.001 | 0.45 | 0.50 | 75.93 (66.7–83.6) | 69.23 (60.0–77.4) | 2.47 (1.8–3.3) | 0.35 (0.2–0.5) |
AUC, area under the curve; CI, confidence interval; YI, Youden Index; PLR, positive likelihood ratio; NLR, negative likelihood ratio; Maximum base width of soft tissue, maximum base width of soft tissue components on the pleura side.
Discussion
In this study, we first assessed the classification of Lung-RADS 4X peripheral pulmonary nodules using clinical factors and the tumour-pleura relationship, and then further evaluated VPI in patients with Lung-RADS 4X peripheral lung adenocarcinoma using the above clinical and imaging factors. Our study found that gender, CEA, type of nodule, and the maximum base width of soft tissue components on the pleura side were independent risk factors for distinguishing lung adenocarcinoma from tuberculous granuloma. In addition, the type of linear connection between lesion and pleura and the maximum base width of soft tissue components on the pleura side were independent predictors of VPI in patients with peripheral lung adenocarcinoma. ROC curve analysis revealed that the combined model based on the above independent predictors demonstrated good predictive performance in predicting the classification and VPI of Lung-RADS 4X peripheral pulmonary nodules (AUC were 82.60% and 76.10%, respectively).
CEA is a reproducible and widely used tumour marker. Previous studies have shown that CEA enhances intercellular adhesion and promotes tumour formation in a variety of tumour cells, and is widely used in the detection of a variety of tumours, especially in the diagnosis and prognosis of lung cancer (15-17). The results of this study showed that CEA was significantly different in the classification of pulmonary nodules and VPI, and was an independent predictor of tuberculous granuloma and lung adenocarcinoma. Several researchers have shown that CEA levels are greater in lung cancer patients than those in patients with tuberculous granuloma, especially those with lung adenocarcinoma (8,18). Moreover, Zhu et al. (19) and Fujibayashi et al. (20) reported that CEA was a good marker for predicting VPI in non-small cell lung cancer. This is consistent with our findings.
The results of our study suggest that type of nodule and the maximum base width of soft tissue components on the pleura side are independent risk factors for distinguishing Lung-RADS 4X lung adenocarcinoma from tuberculous granuloma. In terms of type of nodules, although both can present as solid, subsolid or ground-glass nodules, tuberculous granuloma is more often solid, whereas lung adenocarcinoma is more often subsolid and ground-glass nodules. Henschke et al. (21) reported that patients with subsolid nodules (63%) had a higher risk of malignancy, followed by those with ground-glass nodules (18%) and solid nodules (7%). Kim et al. (22) also showed that approximately 75% of ground-glass nodules were lung adenocarcinoma. The presence of a soft tissue component at the pleural side correlates with the type of peripheral lung nodule, and lung adenocarcinomas are due to the growth of tumour cells towards the pleura, thus filling the cavity between the depressed visceral pleura and the wall pleura. Tuberculous granuloma is more likely to involve the pleura, causing exudation and fibrin deposition (23). Therefore, we hypothesized that tuberculous granuloma is more likely to cause pleural adhesion thickening than lung adenocarcinoma, resulting in more extensive formation of soft tissue components on the pleural side. However, in order to confirm our results, further studies in a wider population are needed.
VPI is one of the key determinants of pathological staging of lung cancer and is associated with occult lymph node metastasis and local recurrence (24,25). The results of this study showed that the type of linear connection between lesion and pleura and the maximum base width of soft tissue components on the pleura side were independent predictors of VPI in Lung-RADS 4X peripheral lung adenocarcinoma. The linear connection between lesions and pleura is thought to be thickened interlobular interval extending from the surface of the nodule to the surface of the pleura, and may be related to local oedema, the spread of tumour cells in and out of the lymphatic vessels, inflammatory cells or fibrosis (9). Therefore, the likelihood of VPI is greater for multilinear connections. This is consistent with the findings of Yang et al. (26) and Hsu et al. (9). We hypothesized that the maximum base width of soft tissue components on the pleura side was related to the amount of tumour cells entering the interpleural space between the visceral and mural layers, and that the more aggressive the tumour was, the more extensive the soft tissue formation on the pleural side was, the greater the soft tissue base width will be.
In this study, although some variables were significantly different between the two groups in the univariate analysis, they were not significantly different in multivariate logistic regression. Such as those predicting VPI including type of nodules, maximum diameter of the nodule, maximum diameter of solid component, type of adjacent pleura and presence of soft tissue components on the pleura side. This is because multivariate logistic regression analysis balances the effects of confounding factors and allows screening for objective factors that predict the type of pulmonary nodule and VPI.
This study has several limitations: First, as a single-center retrospective study, we only selected patients who underwent surgery for the evaluation of VPI, which is inevitable for potential selection bias. Second, due to the low percentage of other pathological types such as fungal infection, inflammatory lesions, squamous cell lung cancer, and small cell lung cancer among Lung-RADS 4X peripheral pulmonary nodules, they were not included in this study, and in the future, we need to include multi-center and more pathological types for research to obtain more extensive and accurate conclusions.
Conclusions
In conclusion, for Lung-RADS 4X peripheral pulmonary nodules, the type of nodules and the maximum base width of soft tissue components on the pleura side were found to be independent risk factors for distinguishing lung adenocarcinoma from tuberculous granuloma, and the type of linear connection between lesion and pleura and the maximum base width of soft tissue components on the pleura side were independently associated with VPI. These easy-to-use quantitative variables will help to provide effective risk stratification of Lung-RADS 4X peripheral pulmonary nodules.
Acknowledgments
Funding: This study was supported by grants from
Footnote
Reporting Checklist: The authors have completed the STROBE reporting checklist. Available at https://qims.amegroups.com/article/view/10.21037/qims-24-530/rc
Conflicts of Interest: All authors have completed the ICMJE uniform disclosure form (available at https://qims.amegroups.com/article/view/10.21037/qims-24-530/coif). All the authors report that the study was supported by grants from the Natural Science Foundation of China (No. 82371914), Gansu Provincial Natural Science Foundation of China (No. 21JR7RA427), and the Cuiying Scientific and Technological Innovation Program of Lanzhou University Second Hospital (No. CY2021-QN-B08). The authors have no other conflicts of interest to declare.
Ethical Statement: The authors are accountable for all aspects of the work in ensuring that questions related to the accuracy or integrity of any part of the work are appropriately investigated and resolved. The study was conducted in accordance with the Declaration of Helsinki (as revised in 2013). The study was approved by the ethics committee of Lanzhou University Second Hospital (No. 2023A-745), and the requirement for informed consent was waived for this retrospective study.
Open Access Statement: This is an Open Access article distributed in accordance with the Creative Commons Attribution-NonCommercial-NoDerivs 4.0 International License (CC BY-NC-ND 4.0), which permits the non-commercial replication and distribution of the article with the strict proviso that no changes or edits are made and the original work is properly cited (including links to both the formal publication through the relevant DOI and the license). See: https://creativecommons.org/licenses/by-nc-nd/4.0/.
References
- de Koning HJ, van der Aalst CM, de Jong PA, Scholten ET, Nackaerts K, Heuvelmans MA, et al. Reduced Lung-Cancer Mortality with Volume CT Screening in a Randomized Trial. N Engl J Med 2020;382:503-13. [Crossref] [PubMed]
- Kastner J, Hossain R, Jeudy J, Dako F, Mehta V, Dalal S, Dharaiya E, White C. Lung-RADS Version 1.0 versus Lung-RADS Version 1.1: Comparison of Categories Using Nodules from the National Lung Screening Trial. Radiology 2021;300:199-206. [Crossref] [PubMed]
- Shu J, Wen D, Xu Z, Meng X, Zhang Z, Lin S, Zheng M. Improved interobserver agreement on nodule type and Lung-RADS classification of subsolid nodules using computer-aided solid component measurement. Eur J Radiol 2022;152:110339. [Crossref] [PubMed]
- Chung K, Jacobs C, Scholten ET, Goo JM, Prosch H, Sverzellati N, Ciompi F, Mets OM, Gerke PK, Prokop M, van Ginneken B, Schaefer-Prokop CM. Lung-RADS Category 4X: Does It Improve Prediction of Malignancy in Subsolid Nodules? Radiology 2017;284:264-71. [Crossref] [PubMed]
- Dyer SC, Bartholmai BJ, Koo CW. Implications of the updated Lung CT Screening Reporting and Data System (Lung-RADS version 1.1) for lung cancer screening. J Thorac Dis 2020;12:6966-77. [Crossref] [PubMed]
- Kim H, Goo JM, Kim TJ, Kim HY, Gu G, Gil B, et al. Effectiveness of radiologist training in improving reader agreement for Lung-RADS 4X categorization. Eur Radiol 2021;31:8147-59. [Crossref] [PubMed]
- Khorrami M, Bera K, Thawani R, Rajiah P, Gupta A, Fu P, Linden P, Pennell N, Jacono F, Gilkeson RC, Velcheti V, Madabhushi A. Distinguishing granulomas from adenocarcinomas by integrating stable and discriminating radiomic features on non-contrast computed tomography scans. Eur J Cancer 2021;148:146-58. [Crossref] [PubMed]
- Jia H, Zhang L, Wang B. The Value of Combination Analysis of Tumor Biomarkers for Early Differentiating Diagnosis of Lung Cancer and Pulmonary Tuberculosis. Ann Clin Lab Sci 2019;49:645-9. [PubMed]
- Hsu JS, Han IT, Tsai TH, Lin SF, Jaw TS, Liu GC, Chou SH, Chong IW, Chen CY. Pleural Tags on CT Scans to Predict Visceral Pleural Invasion of Non-Small Cell Lung Cancer That Does Not Abut the Pleura. Radiology 2016;279:590-6. [Crossref] [PubMed]
- Oyama M, Miyagi Maeshima A, Tochigi N, Tsuta K, Kawachi R, Sakurai H, Watanabe S, Asamura H, Tsuda H. Prognostic impact of pleural invasion in 1488 patients with surgically resected non-small cell lung carcinoma. Jpn J Clin Oncol 2013;43:540-6. [Crossref] [PubMed]
- Wo Y, Zhao Y, Qiu T, Li S, Wang Y, Lu T, Qin Y, Song G, Miao S, Sun X, Liu A, Kong D, Dong Y, Leng X, Du W, Jiao W. Impact of visceral pleural invasion on the association of extent of lymphadenectomy and survival in stage I non-small cell lung cancer. Cancer Med 2019;8:669-78. [Crossref] [PubMed]
- Ren J, Ren J, Wang K, Xu Y, Zhu M, Ren T, Guo Z, Li R, Huang J, Tan Q. The location of visceral pleural invasion in stage IB patients with non-small cell lung cancer: Comparison and prognosis. Eur J Surg Oncol 2023;49:950-7. [Crossref] [PubMed]
- Yu Y, Huang R, Wang P, Wang S, Ling X, Zhang P, Yu J, Wang J, Xiao J, Wang Z. Sublobectomy versus lobectomy for long-term survival outcomes of early-stage non-small cell lung cancer with a tumor size ≤2 cm accompanied by visceral pleural invasion: a SEER population-based study. J Thorac Dis 2020;12:592-604. [Crossref] [PubMed]
- Zhang C, Wang L, Cai X, Li M, Sun D, Wang P. Tumour-pleura relationship on CT is a risk factor for occult lymph node metastasis in peripheral clinical stage IA solid adenocarcinoma. Eur Radiol 2023;33:3083-91. [Crossref] [PubMed]
- Burotto M, Thomas A, Subramaniam D, Giaccone G, Rajan A. Biomarkers in early-stage non-small-cell lung cancer: current concepts and future directions. J Thorac Oncol 2014;9:1609-17. [Crossref] [PubMed]
- Grunnet M, Sorensen JB. Carcinoembryonic antigen (CEA) as tumor marker in lung cancer. Lung Cancer 2012;76:138-43. [Crossref] [PubMed]
- Matsuoka K, Sumitomo S, Nakashima N, Nakajima D, Misaki N. Prognostic value of carcinoembryonic antigen and CYFRA21-1 in patients with pathological stage I non-small cell lung cancer. Eur J Cardiothorac Surg 2007;32:435-9. [Crossref] [PubMed]
- Jia Y, Xiao X, Sun Q, Jiang H. CT spectral parameters and serum tumour markers to differentiate histological types of cancer histology. Clin Radiol 2018;73:1033-40. [Crossref] [PubMed]
- Zhu K, Chen L, He C, Lang Y, Kong X, Qu C, Xu S. Prediction of Pleural Invasion in Challenging Non-Small-Cell Lung Cancer Patients Using Serum and Imaging Markers. Dis Markers 2020;2020:6430459. [Crossref] [PubMed]
- Fujibayashi Y, Ogawa H, Kitazume M, Nishikubo M, Nishioka Y, Kimura K, Tane S, Kitamura Y, Nishio W. Pleural invasion, epidermal growth factor receptor mutation and carcinoembryonic antigen level affect pleural lavage cytology-positive status in non-small-cell lung cancer. Eur J Cardiothorac Surg 2021;59:791-8. [Crossref] [PubMed]
- Henschke CI, Yankelevitz DF, Mirtcheva R, McGuinness G, McCauley D, Miettinen OS. ELCAP Group. CT screening for lung cancer: frequency and significance of part-solid and nonsolid nodules. AJR Am J Roentgenol 2002;178:1053-7. [Crossref] [PubMed]
- Kim HY, Shim YM, Lee KS, Han J, Yi CA, Kim YK. Persistent pulmonary nodular ground-glass opacity at thin-section CT: histopathologic comparisons. Radiology 2007;245:267-75. [Crossref] [PubMed]
- Tan H, Wang Y, Jiang Y, Li H, You T, Fu T, Peng J, Tan Y, Lu R, Peng B, Huang W, Xiong F. A study on the differential of solid lung adenocarcinoma and tuberculous granuloma nodules in CT images by Radiomics machine learning. Sci Rep 2023;13:5853. [Crossref] [PubMed]
- Goldstraw P, Chansky K, Crowley J, Rami-Porta R, Asamura H, Eberhardt WE, Nicholson AG, Groome P, Mitchell A, Bolejack VInternational Association for the Study of Lung Cancer Staging and Prognostic Factors Committee, Advisory Boards, and Participating Institutions. International Association for the Study of Lung Cancer Staging and Prognostic Factors Committee Advisory Boards and Participating Institutions. The IASLC Lung Cancer Staging Project: Proposals for Revision of the TNM Stage Groupings in the Forthcoming (Eighth) Edition of the TNM Classification for Lung Cancer. J Thorac Oncol 2016;11:39-51. [Crossref] [PubMed]
- Jiang L, Liang W, Shen J, Chen X, Shi X, He J, Yang C, He J. The impact of visceral pleural invasion in node-negative non-small cell lung cancer: a systematic review and meta-analysis. Chest 2015;148:903-11. [Crossref] [PubMed]
- Yang Y, Xie Z, Hu H, Yang G, Zhu X, Yang D, Niu Z, Mao G, Shao M, Wang J. Using CT imaging features to predict visceral pleural invasion of non-small-cell lung cancer. Clin Radiol 2023;78:e909-17. [Crossref] [PubMed]