Quantitative assessment of renal steatosis in patients with type 2 diabetes mellitus using the iterative decomposition of water and fat with echo asymmetry and least squares estimation quantification sequence imaging: repeatability and clinical implications
Introduction
The prevalence of diabetes mellitus (DM) has significantly increased in recent decades and thus represents a global health crisis. DM has emerged as a serious and prevalent chronic disease, imposing severe life-threatening and disabling consequences. Projections from recent epidemiological studies suggest that the global DM population may surpass 700 million by 2045 (1). Notably, China has experienced one of the most substantial increases in DM prevalence worldwide and currently hosts the largest DM population (2). Type 2 diabetes mellitus (T2DM) accounts for >95% of patients with DM in China. The incidence and prevalence of DM, predominantly attributed to T2DM, have shown an upward trend (3). This surge in T2DM cases has significantly contributed to the increasing incidence of diabetic kidney disease (DKD), a frequent complication of T2DM. DKD has become a predominant cause of end-stage renal disease, representing nearly 50% of cases in developed nations (4). Although the precise pathogenesis of DKD in T2DM is still being investigated, and evidence suggests a correlation with abnormal renal fat depositions (5).
Ectopic fat deposition, extensively documented in multiple organs such as the liver, skeletal muscle, and heart, disrupts the cellular functions within these organs (6). When observed in the kidneys, this phenomenon, termed renal fat deposition or fatty kidney, has been associated with glomerular sclerosis, tubular damage, interstitial fibrosis, and ultimately, impaired renal function. The concept of fatty kidney was first introduced in 1883, and since then, evidence has grown linking it to DKD development (7). Although the pathogenic mechanisms connecting renal fat deposition to kidney disease remain incompletely understood, emerging evidence suggests that abnormal fat metabolism and accumulation in the kidneys are key factors in DKD development (5). Currently, direct kidney biopsy and immunohistochemical staining serve as the gold standard for assessing renal steatosis (7,8). However, this invasive approach carries a heightened risk of complications. Consequently, there is a growing need for noninvasive methods to detect kidney fat deposition, which can significantly influence the evaluation of therapies aimed at preventing DKD progression. Despite previous attempts using conventional radiologic imaging techniques [such as ultrasound, computed tomography (CT), and structural magnetic resonance imaging (MRI)] to evaluate renal fat deposition, the results obtained have been inconsistent. In addition, ultrasound, CT, and structural MRI are used to evaluate the fat thickness of the perirenal and renal sinus (8) but are not able to assess the fat deposition of renal parenchyma. In short, there is an urgent need for a noninvasive diagnostic tool capable of sequentially monitoring renal parenchymal fat accumulation in patients with T2DM (9). Therefore, the development of reliable, reproducible, and noninvasive methodologies is critically required to realize the dynamic assessment of DKD onset and renal parenchymal fat deposition monitoring.
Quantitative MRI techniques such as proton magnetic resonance spectroscopy (MRS) and complex-based chemical shift imaging-based MRI have recently been employed for the noninvasive assessment of renal parenchyma fat deposition, offering benefits such as absence of ionizing radiation, safety, high soft-tissue resolution, and repeatability (10). Initially designed as the noninvasive gold standard for diagnosing fatty liver disease (11,12), these techniques are now used to quantify fat content in the skeletal muscle, bone marrow, and pancreas (13-18). MRS has the ability to noninvasively quantify triglycerides based on tissue-specific metabolite spectra and is considered as the most precise noninvasive method for quantifying renal fat deposition (19). Nevertheless, this technique is time-consuming, technically demanding, and potentially erroneous, as it usually samples only a small segment of the kidney (7,20). Therefore, complex-based chemical shift imaging-based MRI such as two-point Dixon and three-point Dixon imaging, which can rapidly cover the entire kidney, have been devised. Furthermore, the spectral complexity of fat is increasingly becoming the preferred method for quantifying renal steatosis in clinical trials (21). Iterative decomposition of water and fat with echo asymmetry and least square estimation quantification (IDEAL-IQ) is a Dixon pulse multiple-echo sequence and a more accurate method for fat quantification than the three-point Dixon technique. This is due to its consideration of T2* effects and field inhomogeneity, which can provide a proton-density fat fraction (PDFF) measurement that is not affected by iron overload (17,22). Moreover, it is simple to operate and directly measures PDFF values in the region of interest (ROI). IDEAL-IQ seems suited to investigating the role of fatty kidney in T2DM. Therefore, our study aimed to evaluate the repeatability of renal IDEAL-IQ imaging in assessing renal fat deposition and to characterize the variations in renal parenchymal fat deposition in patients with T2DM patients. Our findings can crucially contribute to evaluating the effectiveness of new treatments targeting DKD. We present this article in accordance with the STROBE reporting checklist (available at https://qims.amegroups.com/article/view/10.21037/qims-24-330/rc).
Methods
Study design and patient population
The study was conducted in accordance with the Declaration of Helsinki (as revised in 2013), and the study protocol and design were approved by the Institutional Review Board of Guizhou Provincial People’s Hospital (approval No. KY 2022-02). This study enrolled two distinct groups: group A included healthy volunteers who participated in the repeatability analyses, whereas group B included patients diagnosed with T2DM who were recruited from the endocrinology and nephrology outpatient clinic. MRI examinations were conducted between October 01, 2021, and November 28, 2023, at Guizhou Provincial People’s Hospital. Only participants without contraindications to MRI, such as metallic devices or claustrophobia, were selected for the evaluation of renal parenchymal PDFF via the IDEAL-IQ technique. The detailed inclusion and exclusion criteria are summarized in Figure 1. Written informed consent was obtained from all the participants before the examination.
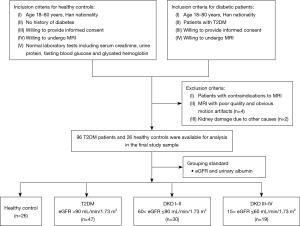
Laboratory measurements
During the initial visit, each participant was subjected to a comprehensive physical assessment, encompassing anthropometric measurements and blood collection. The following demographic and laboratory data were recorded: age; sex; height, weight, hip, and waist measurements; T2DM history; and fasting blood glucose, serum creatinine (Scr), albuminuria, and blood lipid levels. Scr and cystatin C was used to estimate glomerular filtration rate (eGFR) according to the Chronic Kidney Disease Epidemiology Collaboration formula (23). Based on their eGFR and albuminuria levels, patients were categorized into T2DM (eGFR >90 mL/min/1.73 m2), DKD stages I–II (60< eGFR ≤90 mL/min/1.73 m2), or DKD stages III–IV (15< eGFR ≤60 mL/min/1.73 m2). Laboratory analyses were performed in the central clinical chemistry laboratory of Guizhou Provincial People’s Hospital.
MRI protocols
All MRI examinations were performed on a 3.0-T MRI scanner (Discovery MR 750W, GE HealthCare, Chicago, IL, USA). Standard eight-channel abdomen surface receiver coils were applied with the participants in the supine position. Each exam included coronal and axial T2-weighted single-shot fast spin-echo sequences. Additionally, breath-hold axial IDEAL-IQ scans were acquired at end expiration. The IDEAL-IQ imaging parameters were as follows: repetition time, 6.0 ms; echo time, minimum; slice thickness, 5 mm; matrix size, 260×260×500; field of view, 420 mm × 336 mm; and acquisition time, 17 s. The images were processed to generate water-phase, fat-phase, and R2* and fat fraction maps. In healthy volunteers, examination differences were obtained by repeating the IDEAL-IQ measurement immediately after the initial scan without the repositioning of the participants or surface coil.
Image analysis
All image analyses were performed on anonymized data using a picture archiving and communication system (Advantage Workstation 4.6, GE HealthCare) by two experienced abdominal radiologists (C.T., 9 years of experience; Y.W., 3 years of experience) blinded to the demographic and clinical data except for the presence of benign renal lesions such as cysts. For renal parenchymal PDFF assessment, three central slices on the fat fraction maps were selected as ROIs for each side of the kidney. These ROIs were manually drawn along the edge of the kidney parenchyma, as illustrated in Figure 2. Care was taken to avoid areas potentially affected by artifacts, including contamination with renal sinus or perirenal fat, and benign renal lesions. The mean PDFF values were recorded for each renal side, and the overall mean renal parenchymal PDFF value was calculated by averaging all measurements from both sides. Segmentation of the cortex and medulla was not performed separately since no significant difference was found between the two structures.
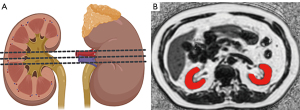
Statistical analysis
Statistical analysis was conducted using SPSS 26 software. Continuous variables that followed a normal distribution are presented as the mean ± standard deviation (SD), while those not adhering to a normal distribution presented as the median and interquartile range and were analyzed with the Kruskal-Wallis test. Meanwhile, categorical variables are presented as frequencies and were examined using the Chi-squared test. The intraclass correlation coefficient (ICC) was used to evaluate the consistency of the renal PDFF values measured by two radiologists. In evaluating the uniformity of renal fat quantification, the PDFF values of the right and left renal parenchyma were compared via a paired t-test. Pearson correlation coefficients were calculated to assess the agreement between the first and second measurements of renal parenchymal PDFF. Bland-Altman plots were visualized via scatterplots to illustrate the disparities, with reference lines at the mean difference and at the mean difference ±1.96 × SD of the differences (limits of agreement). The differences in clinical data and renal parenchymal PDFF values among the three groups were analyzed using one-way analysis of variance (ANOVA). Pearson correlation analysis was used to evaluate the associations between renal parenchymal PDFF and clinical indices. Receiver operating characteristic (ROC) curve analysis was used to assess the diagnostic performance of IDEAL-IQ for identifying healthy controls (HCs) and patients with T2DM, DKD–III, or DKD III–IV. The difference in performance was analyzed by comparing the corresponding areas under the curve (AUC). All analyses were two-tailed, and a P value <0.05 was considered statistically significant.
Results
Clinical and laboratory characteristics
Table 1 contains the demographic data of the participants. Among the initial patients, 6 were excluded due to the failure of IDEAL-IQ reconstruction owing to respiratory motion artifacts, resulting in a final cohort of 122 participants (71 males and 51 females). Table 1 also provides the characteristics of the patients, including age, gender, body mass index (BMI), waist-to-hip ratio (WHR), Scr, eGFR, and blood fat levels.
Table 1
Parameter | HC | T2DM | DKD I–II | DKD III–IV | F | P* |
---|---|---|---|---|---|---|
Gender (M/F) | 14/12 | 25/22 | 22/8 | 10/9 | 3.637† | 0.303 |
Age (years) | 53.58±1.77 | 53.13±1.36 | 57.73±1.53b | 61.42±2.14ab | 4.803* | 0.003 |
BMI (kg/m2) | 22.38±0.57 | 24.40±0.46a | 25.61±0.58 a | 25.73±0.92a | 5.829* | 0.001 |
WHR | 0.94±0.01 | 0.92±0.01 | 0.94±0.01 | 0.98±0.01abc | 5.908* | 0.001 |
Diabetes duration (years) | – | 6.72±0.87 | 9.77±0.85b | 11.84±1.70b | 5.840* | 0.004 |
Scr (mg/dL) | – | 61.71±1.97 | 77.90±2.21b | 153.75±13.17bc | 75.083* | <0.001 |
eGFR (mL/min/1.72 m2) | – | 108.26 (97.12, 130.59) | 88.50 (76.61, 105.08)b | 45.68 (36.63, 53.33)bc | 49.626‡ | <0.001 |
TG (mg/dL) | – | 1.43 (1.01, 2.33) | 1.95 (1.04, 2.55) | 2.42 (1.18, 4.56) | 4.708‡ | 0.095 |
TC (mg/dL) | – | 4.82±0.44 | 4.37±0.22 | 4.54±0.28 | 0.343* | 0.711 |
HDL-C (mg/dL) | – | 0.95 (0.81, 1.31) | 0.91 (0.75, 1.18) | 0.99 (0.76, 1.07) | 1.923‡ | 0.382 |
LDL-C (mg/dL) | – | 2.32 (1.85, 2.96) | 2.42 (1.54, 3.26) | 2.11 (1.47, 2.48) | 0.601‡ | 0.551 |
Data are presented as the number, mean ± standard deviation or median (first quartile, third quartile). *, least significant difference multiple pairwise comparison tests were used for statistically significant analysis of variance values. Means with different letters indicate groups that significantly differed; †, Chi-squared test; ‡, Kruskal-Wallis test. a, significant difference with HCs (P<0.05); b, significant difference with T2DM (P<0.05); c, significant difference with DKD I–II (P<0.05). BMI = weight (kg)/height (m)2. HC, healthy control; T2DM, type 2 diabetes mellitus; DKD, diabetic kidney disease; M, male; F, female; BMI, body mass index; WHR, waist-to-hip ratio; Scr, serum creatinine; eGFR, estimated glomerular filtration rate; TG, triglyceride; TC, total cholesterol; HDL-C, high-density lipoprotein cholesterol; LDL-C, low-density lipoprotein cholesterol.
Repeatability assessment
A total of 26 healthy participants (mean age 53.58±1.77 years; mean BMI 22.38±0.57 kg/m2; 14 males) underwent IDEAL-IQ for the assessment of repeatability. The renal parenchymal PDFF measurements in the second examination highly correlated with those from the first examination (r=0.81) (Figure 3A). Bland-Altman analysis for the examination measurements showed a mean difference of 0.004%, with the lower and upper limits of agreement being −0.22% and 0.23%, respectively (Figure 3B). The ICC of renal parenchyma PDFF values measured by two radiologists was 0.822 [95% confidence interval (CI): 0.774–0.861], which being higher than 0.75, indicated good repeatability.
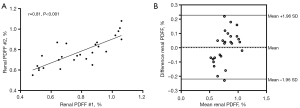
Renal parenchymal PDFF measured with IDEAL-IQ imaging
The renal parenchymal PDFF data for the study population are shown in Table 2 and Figure 4. There were no notable disparities observed in the PDFF values of the renal parenchyma between the two kidneys. However, statistically significant differences in renal parenchymal PDFF values were observed between the HCs, patients with T2DM, and those with different stages of DKD. These values were highest for DKD stage III–IV. Subsequent post hoc tests confirmed that there were significant differences between the HC and T2DM groups classified in different disease stages.
Table 2
Group | Left renal PDFF (%) | Right renal PDFF (%) | t | P# | Mean PDFF (%) |
---|---|---|---|---|---|
HC | 0.81±0.04 | 0.75±0.04 | −1.764 | 0.090 | 0.77±0.04 |
T2DM | 1.11±0.04a | 1.04±0.05a | −1.377 | 0.175 | 1.07±0.04a |
DKD I–II | 1.21±0.08a | 1.08±0.07a | −1.564 | 0.129 | 1.15±0.06a |
DKD III–IV | 1.44±0.06abc | 1.48±0.05abc | 0.598 | 0.557 | 1.46±0.04abc |
F | 18.052 | 21.239 | – | – | 28.251 |
P* | <0.001 | <0.001 | – | – | <0.001 |
Data are presented as mean ± standard deviation. #, paired t-test; *, least significant difference multiple pairwise comparison tests were used for statistically significant analysis of variance values. Means with different letters indicate groups that significantly differed. a, significant difference with HCs (P<0.05); b, significant difference with T2DM (P<0.05); c, significant difference with DKD I–II (P<0.05). PDFF, proton-density fat fraction; HC, healthy control; T2DM, type 2 diabetes mellitus; DKD, diabetic kidney disease.
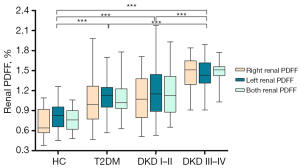
Correlation between renal parenchymal PDFF values and laboratory indices
Renal parenchymal PDFF value was negatively correlated with the eGFR (r=−0.437; P<0.001) and positively correlated with Scr level (r=0.421; P<0.001) (Figure 5). No statistically significant correlation was observed between the blood fat features (triglyceride, total cholesterol, high-density lipoprotein cholesterol, and low-density lipoprotein cholesterol) and renal parenchymal PDFF.
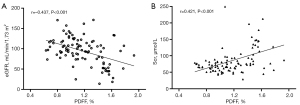
Renal IDEAL-IQ for identify renal injury in patients with T2DM
The ROC curves for IDEAL-IQ in identifying HCs and patients with T2DM and/or DKD are shown in Figure 6; Table 3 provides the corresponding threshold, sensitivity, specificity, AUC, and predictive values.
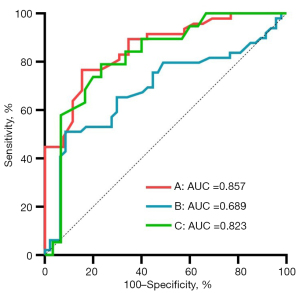
Table 3
Parameter | Threshold | AUC (95% CI) | P | Sensitivity, % | Specificity, % |
---|---|---|---|---|---|
HC and T2DM | 0.92 | 0.857 (0.77–0.94) | <0.001 | 76.6 | 84.62 |
T2DM and DKD | 1.36 | 0.689 (0.58–0.80) | <0.001 | 51.02 | 91.49 |
DKD I–II and DKD III–IV | 1.42 | 0.823 (0.70–0.94) | <0.001 | 78.95 | 76.67 |
IDEAL-IQ, iterative decomposition of water and fat with echo asymmetry and least squares estimation quantification; T2DM, type 2 diabetes mellitus; AUC, area under the curve; CI, confidence interval; HC, healthy control; DKD, diabetic kidney disease.
Discussion
In this study, we aimed to evaluate the repeatability and potential of IDEAL-IQ, a quantitative MRI technique, for the noninvasive quantification of renal fat deposition in patients with T2DM. Our findings revealed a substantial increase in renal parenchymal PDFF values in patients with T2DM when compared to healthy volunteers, indicating elevated renal fat deposition. Furthermore, we observed a positive correlation between the severity of DKD and increasing renal parenchymal fat deposition. These results emphasize the significant association between renal fat deposition and the progression of DKD in patients with T2DM.
Ensuring the consistency of a technique is crucial for the longitudinal monitoring of changes. When compared to MRS or the two-point Dixon method, the IDEAL-IQ technique is capable of acquiring PDFF images without the need for postprocessing and can autonomously compute the PDFF with a reduced scan and measurement duration. Meisamy et al. (24) documented the accuracy of IDEAL-IQ in hepatic applications, and Aoki et al. (25) confirmed its repeatability in pelvic imaging through repeated evaluations. However, no studies have yet demonstrated the value of IDEAL-IQ in the kidneys. Therefore, we performed validation and repeatability assessments of renal fat deposition measurements obtained using IDEAL-IQ. The results indicate a strong correlation between two repeatability examination measurements. To enhance interobserver agreement for renal PDFF measurements, we employed large and whole renal parenchymal ROIs. Additionally, multiple researchers were involved in image analysis, reflecting real-world scenarios, and reinforcing the robustness of our data analysis method. Furthermore, controlling for respiratory motion improved imaging quality and measurement repeatability. In addition considering the breathing motion artifact, it is necessary to account for the different resonance frequencies of water and fat, the inhomogeneity of the B0 and B1 fields, and the chemical shift differences (26), especially in patients with DKD and significant central adiposity. The quantitative IDEAL-IQ acquisition protocol used in this study, originally developed for liver fat quantification, demonstrated reliability in assessing renal fat content. Thus, noninvasive longitudinal measurements of renal parenchymal fat deposition using IDEAL-IQ may be suitable for monitoring changes in disease progression and treatment response.
Regarding the fat content of human kidneys, our study revealed an overall mean renal parenchymal PDFF value of 0.77%, which is consistent with the pathological findings regarding the inherently lower fat content in normal human kidneys, typically around 0.4–0.7% (27). Moreover, our results align with prior studies of volunteers measured by Dixon imaging, which reported PDFF values ranging from 0.12% to 2.52% (5,7,19,28). However, there were discrepancies between studies, indicating the existence of variations even within relatively homogeneous subject groups. This variability may be attributed to a combination of factors, including lower renal fat content, water-fat interference on the border of the kidney, technical differences such as field strength and acquisition methods, and variations in study populations (20). Therefore, further research into standardized scanning protocols and larger clinical and multiethnic population trials are warranted to better delineate the renal fat deposition.
In addition, we explored the application of IDEAL-IQ imaging in patients with T2DM. Our results demonstrated that IDEAL-IQ is a potential imaging tool for identifying early renal parenchymal damage in patients with T2DM. Research indicates that early-stage DKD is reversible (29), and thus the early diagnosis of kidney damage and the objective assessment damage severity are crucial for devising appropriate treatment strategies, improving prognosis, and reducing complications. Typically, DKD is diagnosed by the continuous detection of increased urinary albumin excretion (albuminuria), reduced eGFR, or other indicators of kidney damage (29). However, the accuracy, sensitivity, and specificity of these methods remain subject to debate. Therefore, invasive renal biopsy persists as the current gold standard for diagnosing DKD. However, this hampers our ability to predict the progression of the disease, thus necessitating the identification of novel biomarkers. We observed a significant increase in renal parenchymal PDFF values in patients with T2DM with normoalbuminuric and normal eGFR compared to HCs, which is in line with a previous retrospective study (27), thus supporting the potential of parenchymal PDFF to be an additional tool in assessing renal function in patients with T2DM. In addition, the application of IDEAL-IQ was supported by ROC curve analysis, which showed high specificity and a large AUC, suggesting the reliable distinction of patients with T2DM from HCs. Ectopic renal parenchyma fat deposition is related to lipotoxicity, inflammation, and fibrosis (30,31), which are responsible for DKD. Thus, accurate quantification of renal parenchyma fat content may be critical to investigating potential renal injury. Therefore, IDEAL-IQ may be particularly effective in detecting underlying pathologic injuries in the early stages of renal parenchymal damage in patients with T2DM with a normal eGFR.
We observed that IDEAL-IQ allowed for the quantification of changes in kidney fat deposition in T2DM and was able to detect small changes in renal parenchyma fat deposition across different stages of the DKD population, which is of substantial clinical relevance. In our study, higher renal parenchymal PDFF content was observed in patients with T2DM, and renal parenchymal fat deposition markedly increased as DKD worsened. These observations align with the histological and biochemical outcomes noted in prior human and animal research (32-34). Although renal parenchymal PDFF values remain relatively low in patients with T2DM and DKD, even minor increases in renal fat content hold significant pathophysiological implications (35). Our study demonstrated that increased renal parenchymal PDFF values are significantly associated with the severity of DKD in patients with T2DM, and similarly, previous studies reported fat accumulation and lipotoxicity in the kidneys of patients with T2DM (20,32). Renal fat deposition leads to inflammation, oxidative stress, and damage to renal vasculature, mesangial cells, the proximal tubule epithelium, and podocytes. These processes hinder interorgan communication and may contribute to the development and progression of DKD (36,37). Therefore, e renal fat deposition is a potential driver of DKD. Further studies are warranted to confirm the value of quantitative MRI in assessing the relationship between renal parenchyma fat deposition and renal perfusion, renal oxygenation, and renal fibrosis in the T2DM. Our study demonstrated that IDEAL-IQ has high diagnostic efficacy, sensitivity, and specificity in identifying the alteration of renal fat deposition in T2DM at different disease stages. Overall, accurately understanding the degree of fat accumulation in the human kidney may help to assess the severity of renal damage and therapeutic efficacy in T2DM. Consequently, IDEAL-IQ holds the potential to be a noninvasive biomarker of DKD.
Despite its strengths, our study had several limitations that need to be considered. First the inherently low concentration of renal parenchymal fat remains a challenge for IDEAL-IQ measurements. Additionally, better repeatability experiments should be performed after a certain interval, but due to compliance and ethical concerns, we only performed reproducibility scans immediately after the initial scan. Moreover, our cross-sectional design limited our ability to determine any causal relationships between renal parenchymal fat deposition and DKD. Follow-up longitudinal studies are thus essential for elucidating the longitudinal associations. Future investigations should consider regional differences in fat content, positional discrepancies in the cortex or medulla, and the influence of medical interventions, including glucose control, statins, and weight reduction.
Conclusions
Renal fat deposition is a potential driver of DKD. IDEAL-IQ is a promising, reproducible, reliable, and valuable technology for the assessment of renal fat deposition and may be valuable in identifying of the risk of DKD in patients with T2DM. In the future, this approach could facilitate risk assessment, personalized medicine, and the evaluation of damaged organs for patients with T2DM.
Acknowledgments
Funding: This study was supported by
Footnote
Reporting Checklist: The authors have completed the STROBE reporting checklist. Available at https://qims.amegroups.com/article/view/10.21037/qims-24-330/rc
Conflicts of Interest: All authors have completed the ICMJE uniform disclosure form (available at https://qims.amegroups.com/article/view/10.21037/qims-24-330/coif). Xianchun Zeng reports funding from National Natural Science Foundation of China (No. 82060314) and the Guizhou Science and Technology Project (No. QKHZC [2021] YB037). L.N. was an employee of GE HealthCare Magnetic Resonance Research throughout her involvement in the study. The other authors have no conflicts of interest to declare.
Ethical Statement: The authors are accountable for all aspects of the work in ensuring that questions related to the accuracy or integrity of any part of the work are appropriately investigated and resolved. This study was conducted in accordance with the Declaration of Helsinki (as revised in 2013), and the protocol and design were approved by the Institutional Review Board of Guizhou Provincial People’s Hospital (approval No. KY 2022-02). Written informed consent was obtained from all the participants before the examination.
Open Access Statement: This is an Open Access article distributed in accordance with the Creative Commons Attribution-NonCommercial-NoDerivs 4.0 International License (CC BY-NC-ND 4.0), which permits the non-commercial replication and distribution of the article with the strict proviso that no changes or edits are made and the original work is properly cited (including links to both the formal publication through the relevant DOI and the license). See: https://creativecommons.org/licenses/by-nc-nd/4.0/.
References
- Sun H, Saeedi P, Karuranga S, Pinkepank M, Ogurtsova K, Duncan BB, Stein C, Basit A, Chan JCN, Mbanya JC, Pavkov ME, Ramachandaran A, Wild SH, James S, Herman WH, Zhang P, Bommer C, Kuo S, Boyko EJ, Magliano DJ. IDF Diabetes Atlas: Global, regional and country-level diabetes prevalence estimates for 2021 and projections for 2045. Diabetes Res Clin Pract 2022;183:109119. [Crossref] [PubMed]
- Ma RCW. Epidemiology of diabetes and diabetic complications in China. Diabetologia 2018;61:1249-60. [Crossref] [PubMed]
- Jia W, Weng J, Zhu D, Ji L, Lu J, Zhou Z, et al. Standards of medical care for type 2 diabetes in China 2019. Diabetes Metab Res Rev 2019;35:e3158. [Crossref] [PubMed]
- Tuttle KR, Bakris GL, Bilous RW, Chiang JL, de Boer IH, Goldstein-Fuchs J, Hirsch IB, Kalantar-Zadeh K, Narva AS, Navaneethan SD, Neumiller JJ, Patel UD, Ratner RE, Whaley-Connell AT, Molitch ME. Diabetic kidney disease: a report from an ADA Consensus Conference. Am J Kidney Dis 2014;64:510-33. [Crossref] [PubMed]
- Jonker JT, de Heer P, Engelse MA, van Rossenberg EH, Klessens CQF, Baelde HJ, Bajema IM, Koopmans SJ, Coelho PG, Streefland TCM, Webb AG, Dekkers IA, Rabelink TJ, Rensen PCN, Lamb HJ, de Vries APJ. Metabolic imaging of fatty kidney in diabesity: validation and dietary intervention. Nephrol Dial Transplant 2018;33:224-30. [Crossref] [PubMed]
- Opazo-Ríos L, Mas S, Marín-Royo G, Mezzano S, Gómez-Guerrero C, Moreno JA, Egido J. Lipotoxicity and Diabetic Nephropathy: Novel Mechanistic Insights and Therapeutic Opportunities. Int J Mol Sci 2020;21:2632. [Crossref] [PubMed]
- Wang YC, Feng Y, Lu CQ, Ju S. Renal fat fraction and diffusion tensor imaging in patients with early-stage diabetic nephropathy. Eur Radiol 2018;28:3326-34. [Crossref] [PubMed]
- Hti Lar Seng NS, Lohana P, Chandra S, Jim B. The Fatty Kidney and Beyond: A Silent Epidemic. Am J Med 2023;136:965-74. [Crossref] [PubMed]
- Lin L, Dekkers IA, Lamb HJ. Fat accumulation around and within the kidney. Visceral and Ectopic Fat. Elsevier, 2023:131-47.
- Tamaki N, Ajmera V, Loomba R. Non-invasive methods for imaging hepatic steatosis and their clinical importance in NAFLD. Nat Rev Endocrinol 2022;18:55-66. [Crossref] [PubMed]
- Blukacz Ł, Nowak A, Wójtowicz M, Krawczyk A, Franik G, Madej P, Pluta D, Kowalczyk K, Żorniak M. Clinical Usefulness of Non-Invasive Metabolic-Associated Fatty Liver Disease Risk Assessment Methods in Patients with Full-Blown Polycystic Ovary Syndrome in Relation to the MRI Examination with the Ideal IQ Sequence. Biomedicines 2022;10:2193. [Crossref] [PubMed]
- Portillo-Sanchez P, Bril F, Maximos M, Lomonaco R, Biernacki D, Orsak B, Subbarayan S, Webb A, Hecht J, Cusi K. High Prevalence of Nonalcoholic Fatty Liver Disease in Patients With Type 2 Diabetes Mellitus and Normal Plasma Aminotransferase Levels. J Clin Endocrinol Metab 2015;100:2231-8. [Crossref] [PubMed]
- Liu J, Chen JD, Li P, Liao JW, Feng JX, Chen ZY, Cai ZY, Li W, Chen XJ, Su ZH, Lu H, Li SL, Ma YJ. Comprehensive assessment of osteoporosis in lumbar spine using compositional MR imaging of trabecular bone. Eur Radiol 2023;33:3995-4006. [Crossref] [PubMed]
- Soghomonian A, Dutour A, Kachenoura N, Thuny F, Lasbleiz A, Ancel P, Cristofari R, Jouve E, Simeoni U, Kober F, Bernard M, Gaborit B. Is increased myocardial triglyceride content associated with early changes in left ventricular function? A (1)H-MRS and MRI strain study. Front Endocrinol (Lausanne) 2023;14:1181452. [Crossref] [PubMed]
- Chen Y, Jiang Z, Long L, Miu Y, Zhang L, Zhong D, Tang Q. Magnetic resonance imaging: Proton density fat fraction for assessment of pancreatic fatty infiltration during progression of T2DM bama minipigs. J Magn Reson Imaging 2019;50:1905-13. [Crossref] [PubMed]
- Zheng CS, Wen HQ, Lin WS, Luo XW, Shen LS, Zhou X, Zou FY, Li QL, Hu HJ, Guo RM. Quantification of lumbar vertebral fat deposition: Correlation with menopausal status, non-alcoholic fatty liver disease and subcutaneous adipose tissue. Front Endocrinol (Lausanne) 2022;13:1099919. [Crossref] [PubMed]
- Ren W, Feng Y, Feng Y, Li J, Zhang C, Feng L, Cui L, Ran J. Relationship of liver fat content with systemic metabolism and chronic complications in patients with type 2 diabetes mellitus. Lipids Health Dis 2023;22:11. [Crossref] [PubMed]
- Yu X, Huang YH, Feng YZ, Cheng ZY, Cai XR. Well-controlled versus poorly controlled diabetes in patients with obesity: differences in MRI-evaluated pancreatic fat content. Quant Imaging Med Surg 2023;13:3496-507. [Crossref] [PubMed]
- Dekkers IA, de Heer P, Bizino MB, de Vries APJ, Lamb HJ. 1 H-MRS for the assessment of renal triglyceride content in humans at 3T: A primer and reproducibility study. J Magn Reson Imaging 2018;48:507-13. [Crossref] [PubMed]
- Dekkers IA, Bizino MB, Paiman EHM, Smit JW, Jazet IM, de Vries APJ, Lamb HJ. The Effect of Glycemic Control on Renal Triglyceride Content Assessed by Proton Spectroscopy in Patients With Type 2 Diabetes Mellitus: A Single-Center Parallel-Group Trial. J Ren Nutr 2021;31:611-9. [Crossref] [PubMed]
- Kim HJ, Cho HJ, Kim B, You MW, Lee JH, Huh J, Kim JK. Accuracy and precision of proton density fat fraction measurement across field strengths and scan intervals: A phantom and human study. J Magn Reson Imaging 2019;50:305-14. [Crossref] [PubMed]
- Eskreis-Winkler S, Corrias G, Monti S, Zheng J, Capanu M, Krebs S, Fung M, Reeder S, Mannelli L. IDEAL-IQ in an oncologic population: meeting the challenge of concomitant liver fat and liver iron. Cancer Imaging 2018;18:51. [Crossref] [PubMed]
- Inker LA, Tighiouart H, Adingwupu OM, Shlipak MG, Doria A, Estrella MM, Froissart M, Gudnason V, Grubb A, Kalil R, Mauer M, Rossing P, Seegmiller J, Coresh J, Levey AS. CKD-EPI and EKFC GFR Estimating Equations: Performance and Other Considerations for Selecting Equations for Implementation in Adults. J Am Soc Nephrol 2023;34:1953-64. [Crossref] [PubMed]
- Meisamy S, Hines CD, Hamilton G, Sirlin CB, McKenzie CA, Yu H, Brittain JH, Reeder SB. Quantification of hepatic steatosis with T1-independent, T2-corrected MR imaging with spectral modeling of fat: blinded comparison with MR spectroscopy. Radiology 2011;258:767-75. [Crossref] [PubMed]
- Aoki T, Yamaguchi S, Kinoshita S, Hayashida Y, Korogi Y. Quantification of bone marrow fat content using iterative decomposition of water and fat with echo asymmetry and least-squares estimation (IDEAL): reproducibility, site variation and correlation with age and menopause. Br J Radiol 2016;89:20150538. [Crossref] [PubMed]
- Ferreira PF, Gatehouse PD, Mohiaddin RH, Firmin DN. Cardiovascular magnetic resonance artefacts. J Cardiovasc Magn Reson 2013;15:41. [Crossref] [PubMed]
- Yokoo T, Clark HR, Pedrosa I, Yuan Q, Dimitrov I, Zhang Y, Lingvay I, Beg MS, Bobulescu IA. Quantification of renal steatosis in type II diabetes mellitus using dixon-based MRI. J Magn Reson Imaging 2016;44:1312-9. [Crossref] [PubMed]
- Shen Y, Xie L, Chen X, Mao L, Qin Y, Lan R, Yang S, Hu J, Li X, Ye H, Luo W, Gong L, Li Q, Mao Y, Wang Z. Renal fat fraction is significantly associated with the risk of chronic kidney disease in patients with type 2 diabetes. Front Endocrinol (Lausanne) 2022;13:995028. [Crossref] [PubMed]
- American Diabetes Association. 11. Microvascular Complications and Foot Care: Standards of Medical Care in Diabetes-2021. Diabetes Care 2021;44:S151-67. [Crossref] [PubMed]
- Mende C, Einhorn D. Fatty kidney disease: The importance of ectopic fat deposition and the potential value of imaging. J Diabetes 2022;14:73-8. [Crossref] [PubMed]
- Cheng JM, Luo WX, Pan J, Zhang JG, Zhou HY, Chen TW. Renal ectopic lipid deposition in rats with early-stage diabetic nephropathy evaluated by the MR mDixon-Quant technique: association with the expression of SREBP-1 and PPARα in renal tissue. Quant Imaging Med Surg 2023;13:4504-13. [Crossref] [PubMed]
- Herman-Edelstein M, Scherzer P, Tobar A, Levi M, Gafter U. Altered renal lipid metabolism and renal lipid accumulation in human diabetic nephropathy. J Lipid Res 2014;55:561-72. [Crossref] [PubMed]
- Kiss E, Kränzlin B, Wagenblaß K, Bonrouhi M, Thiery J, Gröne E, Nordström V, Teupser D, Gretz N, Malle E, Gröne HJ. Lipid droplet accumulation is associated with an increase in hyperglycemia-induced renal damage: prevention by liver X receptors. Am J Pathol 2013;182:727-41. [Crossref] [PubMed]
- Peng XG, Bai YY, Fang F, Wang XY, Mao H, Teng GJ, Ju S. Renal lipids and oxygenation in diabetic mice: noninvasive quantification with MR imaging. Radiology 2013;269:748-57. [Crossref] [PubMed]
- Bobulescu IA. Renal lipid metabolism and lipotoxicity. Curr Opin Nephrol Hypertens 2010;19:393-402. [Crossref] [PubMed]
- Kanbay M, Copur S, Demiray A, Sag AA, Covic A, Ortiz A, Tuttle KR. Fatty kidney: A possible future for chronic kidney disease research. Eur J Clin Invest 2022;52:e13748. [Crossref] [PubMed]
- Ruan XZ, Varghese Z, Moorhead JF. An update on the lipid nephrotoxicity hypothesis. Nat Rev Nephrol 2009;5:713-21. [Crossref] [PubMed]