Association of liver multi-parameter quantitative metrics determined by dual-layer spectral detector computed tomography (SDCT) with coronary plaque scores
Introduction
Cardiovascular-related diseases are the leading cause of global increase in morbidity and mortality (1,2). Although primary and secondary prevention have been optimised in accordance with guidelines, some major adverse cardiovascular events (recurrent angina pectoris, acute myocardial infarction, severe arrhythmia, heart failure, and death due to coronary heart disease) continue to occur (3,4). The metabolic risk associated with cardiovascular disease has received increasing attention in clinical settings. Among the many cardiovascular metabolic risk factors, fat metabolism and inflammatory disorders caused by increased hepatic fat deposition are important contributors not only in the formation of atherosclerotic plaques but also in plaque rupture, triggering adverse cardiovascular events (5-11). Therefore, assessing the degree of hepatic steatosis is crucial for monitoring the occurrence and progression of coronary atherosclerosis and for the primary and secondary prevention of coronary artery disease (CAD).
Hsu et al. (12) analyzed the correlation between the degree of hepatic steatosis and coronary plaque characteristics. The study showed that hepatic steatosis is associated with the presence of non-calcified and mixed plaques in the coronary arteries, and the severity of coronary obstruction and the development of multi-vessel atherosclerosis are closely related to the severity of hepatic steatosis (13). However, previous studies generally have limitations in the precise and non-invasive diagnosis of hepatic steatosis. Traditional B-mode ultrasound assessment is subjective and exhibits limited sensitivity for the diagnosis of mild steatosis (14,15). The measurement of proton density fat fraction (PDFF) values based on magnetic resonance is not suitable for routine clinical screening and longitudinal assessment of hepatic steatosis (16). Currently, traditional computed tomography (CT) is widely used in clinical practice; however, its reliance on the attenuation imaging of mixed-energy X-rays restricts its capacity to identify material composition and diagnostic sensitivity (17). Dual-layer spectral detector computed tomography (DL-SDCT) is a new type of spectral CT technology that can provide virtual single-level imaging and effective atomic number imaging, enhancing the differentiation of tissue composition compared to conventional CT (18,19). Given that hepatic steatosis is an independent risk factor for cardiovascular diseases, precise detection of hepatic steatosis is expected to provide a new perspective for the prevention and treatment of CAD.
Coronary computed tomography angiography (CCTA) can be used to assess different stages of coronary atherosclerosis, from the detection of early subclinical disease to the evaluation of acute chest pain. In addition, CCTA can non-invasively quantify plaque burden, which is helpful for disease diagnosis, prognosis, and treatment (20). In recent years, the segmental involvement score (SIS) and the segmental stenosis score (SSS) have been used to evaluate the extent and severity of coronary artery plaques (21,22). However, there have been few previous studies on the relationship between hepatic steatosis and the overall plaque burden (23). Therefore, the purpose of this study was to evaluate the association between liver multiparameter metrics measured by spectral detector computed tomography (SDCT) and the severity and extent of coronary artery plaque assessed by CCTA plaque scores, achieving the screening and primary prevention of high-risk populations of coronary heart disease. We present this article in accordance with the STROBE reporting checklist (available at https://qims.amegroups.com/article/view/10.21037/qims-24-53/rc).
Methods
Study participants
Between October 2021 and August 2022, we assessed 2,421 patients suspected of CAD due to symptoms of chest tightness or pain who underwent CCTA at our hospital. Among these patients, 123 with a history of percutaneous coronary intervention or coronary artery bypass graft, 479 with incomplete clinical and laboratory data, 710 without abdominal spectral CT examination, 186 with a history of drinking and liver viral infection, and 279 with normal coronary arteries were excluded; 644 meeting the requirements were included in our study. The study was conducted in accordance with the Declaration of Helsinki (as revised in 2013). The study was approved by the Ethics Committee of Shengjing Hospital of China Medical University (No. 2022PS958K) and informed consent was taken from all individual participants. A flowchart of patient enrolment and study design is shown in Figure 1.
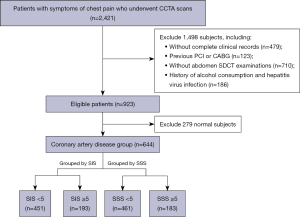
Information on the presence of cardiac risk factors for all subjects was compiled, drawing on their medical histories and case data retrieved from the hospital information system. Hypertension (HTN) was defined as a history of the disease or antihypertensive medicine consumption. Diabetes mellitus (DM) was defined as a previous diagnosis of DM or the use of hypoglycaemic medications. Current smoking or smoking cessation within 1 month of CCTA was defined as a history of smoking. Patients’ laboratory data, including total cholesterol (TC), triglyceride (TG), high-density lipoprotein (HDL), and low-density lipoprotein (LDL) levels, were measured within 1 month before and after CT scanning; if multiple measurements were taken, the laboratory data closest to the date of CCTA examination were considered.
Spectral CT scanning and post-processing
All scans were performed using a 64-slice dual-spectral detector CT scanner (IQon Spectral CT; Philips Healthcare, Best, Netherlands). Patients with a heart rate ≥70 bpm were administered an oral β-blocker (25–50 mg, metoprolol succinate sustained-release tablets, AstraZeneca, Sweden) to control heart rate prior to the examination. All sections from 1 cm below the bifurcation of the trachea to the base of the heart were scanned using the retrospective electrocardiogram (ECG)-gated axial scanning mode. The coronary CTA scan parameters were as follows: tube voltage, 120 kVp; tube current, automatic exposure control (dose right index =13); field of view, 220 mm; matrix, 512×512; tube rotation time, 0.27 s; detector collimation, 64×0.625 mm; slice thickness, 0.9 mm; and slice interval, 0.45 mm. Iohexol (350 mg/mL, GE Healthcare, Ireland) was used as the contrast agent (total amount of contrast agent = body weight × 0.8 mL/kg, injection flow rate (mL/s) = total amount (mL)/injection time (6 s), and then 20–30 mL of normal saline was injected at the same injection rate. Bolus Tracker contrast agent tracking technology was applied to the region of interest (ROI) in the ascending aorta at the pulmonary artery level, starting the scan 6 s after triggering after reaching a predetermined signal attenuation threshold of 90 Hounsfield units (HU). The average radiation dose for CCTA examination is 11.2 mSv.
The SDCT liver scan range for all upper abdominal CT scans spanned from the liver apex to 1 cm below the caudal end of the kidneys; this was performed with an inspiratory breath hold. Scanning parameters included a tube voltage of 120 kV; automatic tube current modulation technique [dose right index =22 reference (Ref): 162 mAs]; rotation time, 0.75 s; pitch, 1.172:1; and image thickness, 3.0 mm, the average radiation dose for a non-contrast SDCT scan of the upper abdomen is 4.5 mSv. The raw data were reconstructed using a spectral iterative reconstruction algorithm (Level-3) to generate spectral-based image datasets. These datasets comprised true conventional polychromatic (120 kVp) images as well as various spectral reconstructions such as virtual monoenergetic images (VMI; energy level at 40 keV), Z-effective (Zeff) images- are colour-coded based on the effective atomic number of tissues, and the slope of the energy spectrum curve of the liver tissue [λ HU, λ HU = (CT40 kev − CT70 kev)/30]. Circular ROIs in the right lobe of the liver, approximately 300 mm2 (deviation <10 mm2), were drawn while avoiding intrahepatic blood vessels, bile duct structures, focal liver lesions, and imaging artefacts. Two experienced radiologists (M.W. and R.B.) with 6 and 8 years of experience, respectively, measured the SDCT parameters while blinded to the clinical data. The final measurements were calculated as the mean values of the two observers after ensuring consistency. Figure 2 shows a multiparameter image from the spectral base image data package reconstructed from a patient’s liver SDCT images.
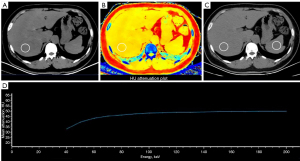
Group standard
We assessed each coronary artery using two coronary plaque scoring methods with CCTA: SSS and SIS (21,22). We defined the cut-off of CCTA scores based on data from a previous report: SSS ≥5 and SIS ≥5 (21). Figure 3 shows the SIS and SSS integration rules for each patient.
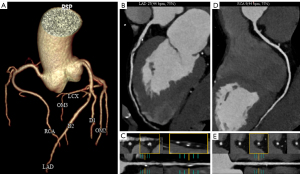
Statistical analysis
All statistical analyses were performed using the commercially available SPSS Statistics V 26.0 (IBM Corporate, New York, USA) software. Continuous variables were summarised as means ± standard deviation (SD) or median with the 25th and 75th percentiles of the interquartile range, as appropriate. Categorical variables were expressed as counts and percentages. The intraclass correlation coefficient (ICC) and Bland-Altman plots were calculated to assess inter-observer agreement for the two measurements, and the mean of the measured values was taken as statistical data for further analysis. The clinical characteristics of the subjects in the two groups were compared using a two-sample t-test or Mann-Whitney U-test. Categorical variables (e.g., sex) were compared using the Chi-squared test. Multivariate logistic regression models were used to assess the predictors of higher coronary plaque scores. Model 1 was adjusted for age, sex, smoking, HTN, hyperlipidaemia, and DM. Model 2 was adjusted for all the covariates in Model 1, including waist circumference, body mass index, and visceral adipose tissue (VAT)/subcutaneous adipose tissue (SAT). The strength of the associations is presented as odds ratios [ORs, 95% confidence interval (CI)]. Statistical significance was set at P<0.05.
Results
Demographic and clinical characteristics
A total of 644 consecutive participants participated in this study. Table 1 shows the characteristics and laboratory data of the enrolled patients. In the SIS and SSS groups, there were significant differences in age (P=0.011, <0.001, respectively), sex (P<0.001, <0.001, respectively), waist (P<0.001, 0.004, respectively), cholesterol levels (P=0.003, 0.001, respectively), HDL cholesterol levels (P<0.001, 0.003, respectively), and LDL cholesterol levels (P=0.008, 0.003, respectively). In the high SIS and SSS groups, the body mass index (BMI) was slightly higher than that in the low plaque burden group; however, the difference was significant in the SIS group (P=0.037), whereas it did not differ between the different SSS groups (P=0.228).
Table 1
Characteristics | SIS | SSS | |||||
---|---|---|---|---|---|---|---|
<5 (n=451) | ≥5 (n=193) | P | <5 (n=461) | ≥5 (n=183) | P | ||
Age (years) | 58.04±10.15 | 60.24±9.99 | 0.011 | 57.82±10.25 | 60.91±9.55 | <0.001 | |
Male, n (%) | 194 (43.02) | 140 (72.54) | <0.001 | 202 (43.82) | 132 (72.13) | <0.001 | |
Smoke, n (%) | 39 (8.65) | 20 (10.36) | 0.489 | 40 (8.68) | 19 (10.38) | 0.499 | |
Hypertension, n (%) | 93 (20.62) | 42 (21.76) | 0.745 | 96 (20.82) | 39 (21.31) | 0.891 | |
Hyperlipidemia, n (%) | 177 (39.25) | 79 (40.93) | 0.689 | 190 (41.21) | 66 (36.07) | 0.228 | |
DM, n (%) | 89 (19.73) | 52 (26.94) | 0.043 | 95 (20.61) | 46 (25.14) | 0.210 | |
BMI (kg/m2) | 25.90±3.50 | 26.51±3.13 | 0.037 | 25.98±3.47 | 26.34±3.21 | 0.228 | |
Waist (cm) | 91.00 (83.00–98.00) | 95.00 (87.00–102.00) | <0.001 | 91.00 (83.00–98.00) | 94.00 (86.50–101.00) | 0.004 | |
TG (mmol/L) | 1.41 (0.98–2.03) | 1.52 (1.04–2.23) | 0.205 | 1.44 (1.00–2.19) | 1.45 (1.02–2.05) | 0.755 | |
Cholesterol (mmol/L) | 4.81 (4.08–5.62) | 4.55 (3.72–5.29) | 0.003 | 4.83 (4.10–5.64) | 4.46 (3.68–5.27) | 0.001 | |
HDL-cholesterol (mmol/L) | 1.23 (1.03–1.47) | 1.08 (0.96–1.31) | <0.001 | 1.21 (1.02–1.46) | 1.10 (0.97–1.34) | 0.003 | |
LDL-cholesterol (mmol/L) | 3.00 (2.29–3.70) | 2.73 (2.09–3.56) | 0.008 | 3.00 (2.30–3.71) | 2.70 (2.07–3.53) | 0.003 |
Data are presented as n (%), mean ± standard deviation, or median (25th–75th) percentiles. SSS, segmental stenosis score; SIS, segmental involvement score; DM, diabetes mellitus; BMI, body mass index; TG, triglycerides; HDL, high-density lipoprotein; LDL, low-density lipoprotein.
Table 2 shows the differences in liver spectral multiparameter images, conventional polychromatic images, and composition of visceral and subcutaneous fat according to the extent and severity of coronary plaque involvement. CT40 kev, CT70 kev, Zeff, λ, VAT, and SAT were significantly different among populations in different SIS and SSS groups (All P<0.001), while CTpoly was statistically significant in different SIS groups (P=0.045); there was no significant difference in SSS groups (P=0.115).
Table 2
Characteristics | SIS | SSS | |||||
---|---|---|---|---|---|---|---|
<5 (n=451) | ≥5 (n=193) | P | <5 (n=461) | ≥5 (n=183) | P | ||
CT40 kev (HU) | 39.60 (28.10–49.30) | 33.70 (22.80–41.70) | <0.001 | 39.10 (27.70–49.00) | 34.20 (23.40–42.00) | <0.001 | |
CT70 kev (HU) | 53.80 (46.95–58.55) | 51.20 (42.50–55.90) | <0.001 | 53.70 (46.80–58.50) | 51.00 (43.35–55.95) | 0.001 | |
λ (HU) | −0.47 (−0.67 to −0.24) | −0.53 (−0.81 to −0.39) | 0.001 | −0.48 (−0.68 to −0.25) | −0.51 (−0.75 to −0.37) | 0.023 | |
Zeff | 7.10 (7.03–7.16) | 7.04 (6.97–7.11) | <0.001 | 7.10 (7.02–7.16) | 7.04 (6.98–7.11) | <0.001 | |
CTpoly (HU) | 53.80 (47.10–58.45) | 52.10 (46.20–57.00) | 0.045 | 53.80 (46.80–58.30) | 52.20 (46.10–57.30) | 0.115 | |
VAT (cm²) | 180.53±28.30 | 198.23±26.80 | <0.001 | 181.24±28.60 | 197.43±26.74 | <0.001 | |
SAT (cm²) | 161.10 (138.70–179.80) | 171.30 (152.10–186.50) | <0.001 | 162.50 (139.10–181.40) | 168.70 (151.55–184.80) | 0.003 |
Data are presented as mean ± standard deviation, or median (25th–75th) percentiles. SIS, segmental involvement score; SSS, segmental stenosis score; CT40 kev, liver fat attenuation assessed by virtual mono-energetic images at 40 kev; HU, Hounsfield unit; CT70 kev, liver fat attenuation assessed by virtual mono-energetic imaging at 70 kev; Zeff, effective atomic number; CTpoly, liver fat attenuation assessed by polychromatic imaging; VAT, visceral adipose tissue; SAT, subcutaneous adipose tissue.
Measurement agreement assessment
Excellent interobserver agreement was observed for CT40 kev measurements (ICC =0.960; 95% CI: 0.954–0.966), CT70 kev (ICC =0.931; 95% CI: 0.920–0.941), Zeff (ICC =0.937; 95% CI: 0.926–0.945), and CTpoly (ICC =0.916; 95% CI: 0.902–0.927). We also analysed the systematic bias of different CT parameters between the two viewers (Figure 4). Bland-Altman plots revealed that there was no statistically significant bias among all the quantitative parameters, and the number with large deviations was controlled within 5%. Therefore, the mean values of the two measurements were used for all calculations and statistical comparisons.
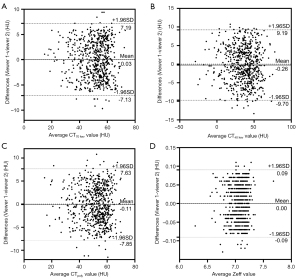
Screening of the most powerful quantitative parameters of the liver
On multivariate binary logistic analysis, after adjusting the conventional cardiovascular risk factors (age, sex, BMI, DM, HTN, smoking, drinking, TC, TG, HDL, and LDL), CT40 kev, CT70 kev, λHU, Zeff, and CTpoly were found to be significantly associated with high SIS and SSS (Table 3). This is due to the collinearity between the above-mentioned hepatic steatosis-related parameters. We substituted these parameters into the regression equation for analysis. According to the standard partial regression coefficient, among these parameters, Zeff was the most powerful independent indicator, whether in the SIS (standard partial regression coefficient =−0.422, P<0.001) or SSS groups (standard partial regression coefficient =−0.346, P<0.001). Thus, Zeff was selected as the best quantitative indicator of the changes in liver parenchyma and was divided into four parts according to the quartile: Zeff ≤7.00 was defined as the Q4 quartile; 7.00< Zeff ≤7.08, Q3 quartile; 7.08< Zeff ≤7.14, Q2 quartile; and Zeff >7.14, Q1 quartile.
Table 3
Variables | SIS | SSS | |||||
---|---|---|---|---|---|---|---|
Standard partial regression coefficient | OR (95% CI) | P | Standard partial regression coefficient | OR (95% CI) | P | ||
CT40 kev (HU) | −0.360 | 0.698 (0.589–0.826) | <0.001 | −0.266 | 0.767 (0.647–0.908) | 0.002 | |
CT70 kev (HU) | −0.296 | 0.744 (0.630–0.879) | <0.001 | −0.252 | 0.777 (0.657–0.919) | 0.003 | |
λ (HU) | −0.311 | 0.719 (0.607–0.851) | <0.001 | −0.200 | 0.819 (0.691–0.970) | 0.021 | |
Zeff | −0.422 | 0.655 (0.552–0.779) | <0.001 | −0.346 | 0.707 (0.597–0.838) | <0.001 | |
CTpoly (HU) | −0.135 | 0.874 (0.740–1.031) | 0.110 | −0.084 | 0.919 (0.776–1.088) | 0.327 |
SIS, segmental involvement score; SSS, segmental stenosis score; OR, odds ratio; CI, confidence interval; CT40 kev, liver fat attenuation assessed by virtual mono-energetic images at 40 kev; HU, Hounsfield unit; CT70 kev, liver fat attenuation assessed by virtual mono-energetic imaging at 70 kev; Zeff, effective atomic number; CTpoly, liver fat attenuation assessed by polychromatic imaging.
Association between liver quantitative parameters and coronary plaque scores
We analyzed the predictive value of the liver SDCT quantitative parameter Zeff for high coronary plaque scores (SSS ≥5, SIS ≥5) (Table 4). To mitigate the confounding bias introduced by multiple metabolic risk factors, we conducted an assessment using adjusted models. In logistic regression analysis, continuous variables can be converted into binary categorical variables by determining the optimal binary classification threshold through the calculation of the corresponding Youden’s index. In multivariate analysis adjusted for age, sex, VAT/SAT ratio, and traditional CAD risk factors, the liver Zeff distribution was also an independent predictor of high coronary plaque scores. Compared with patients in the lowest quartile of Zeff, the adjusted ORs and 95% CIs for SIS were 2.116 (1.134–3.949), 2.832 (1.461–5.491), and 3.584 (1.857–6.918) for those in the second, third, and fourth quartiles, respectively. The corresponding ORs (95% CI) for SSS were 1.933 (1.040–3.592), 2.900 (1.499–5.609), and 3.368 (1.743–6.510) for the upper three quartiles of Zeff.
Table 4
Variable | SIS ≥5 | SSS ≥5 | |||
---|---|---|---|---|---|
OR (95% CI) | P | OR (95% CI) | P | ||
Crude | |||||
Zeff-Q1 | Ref | Ref | |||
Q2 | 2.360 (1.312–4.243) | <0.001 | 1.922 (1.082–3.415) | 0.026 | |
Q3 | 3.124 (1.716–5.690) | <0.001 | 2.691 (1.499–4.830) | 0.001 | |
Q4 | 5.196 (2.958–9.126) | <0.001 | 3.873 (2.238–6.703) | <0.001 | |
Model 1 | |||||
Zeff-Q1 | Ref | Ref | |||
Q2 | 2.134 (1.159–3.929) | 0.015 | 1.853 (1.013–3.389) | 0.045 | |
Q3 | 2.968 (1.582–5.567) | 0.001 | 2.785 (1.496–5.188) | 0.001 | |
Q4 | 4.091 (2.236–7.485) | <0.001 | 3.458 (1.900–6.295) | <0.001 | |
Model 2 | |||||
Zeff-Q1 | Ref | Ref | |||
Q2 | 2.116 (1.134–3.949) | 0.018 | 1.933 (1.040–3.592) | 0.037 | |
Q3 | 2.832 (1.461–5.491) | 0.002 | 2.900 (1.499–5.609) | 0.002 | |
Q4 | 3.584 (1.857–6.918) | <0.001 | 3.368 (1.743–6.510) | <0.001 |
Zeff ≤7.00 was defined as the Q4 quartile; 7.00< Zeff ≤7.08, Q3 quartile; 7.08< Zeff ≤7.14, Q2 quartile; and Zeff >7.14, Q1 quartile. Model 1: adjusted for age, gender, smoking, hypertension, hyperlipidemia, diabetes mellitus; Model 2: adjusted for age, gender, smoking, hypertension, hyperlipidemia, diabetes mellitus, waist circumference, body mass index and visceral adipose tissue/subcutaneous adipose. SIS, segmental involvement score; SSS, segmental stenosis score; OR, odds ratio; CI, confidence interval; Zeff, effective atomic number; Ref, reference.
In addition, age, sex, and visceral fat proportion were independent risk factors for high SIS and SSS (All P<0.05). Further subgroups were analysed, as shown in the forest plots of Figures 5,6. After adjusting for CAD risk factors such as HTN, hyperlipidaemia, DM, waist circumference, BMI, and smoking, SDCT-Zeff was still an independent influencing factor for the high coronary plaque scores, especially among those who were <60 years old and male and had VAT/SAT <1.18.
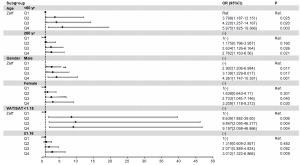
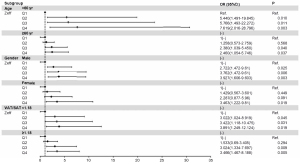
Discussion
Our study demonstrates the association between coronary atherosclerosis plaque scores and liver spectral multi-parameter quantitative metrics (CT40 kev, CT70 kev, λ, Zeff), as determined by SDCT. Compared with patients in the lowest quartile of Zeff, those in the second, third, and fourth quartiles exhibited a higher risk of high coronary plaque burden, especially among those <60 years old, males, and with VAT/SAT <1.18.
Previous studies have predominantly employed obesity as a maker of metabolic dysregulation, utilizing metrics such as BMI, waist circumference, and waist-to-hip ratio to quantify obesity and the risk of coronary heart disease. However, the concept of the ‘obesity paradox’ has complicated the relationship between obesity and the prognosis of coronary heart disease (24-26). The concept of visceral obesity/ectopic fat (hepatic fat, skeletal muscle fat) may provide clues to the obesity paradox in cardiology, as both are involved in the pathophysiological processes of diabetes, insulin resistance, and obesity-related diseases. Therefore, visceral fat and hepatic fat are considered two key drivers of cardiometabolic risks associated with specific levels of body fat (27). The liver, being the most sensitive and initial organ in systemic metabolic dysregulation, when exhibiting ectopic fat deposition, signifies that subcutaneous fat stores are unable to retain excess energy, impairing insulin sensitivity and the body’s ability to rapidly store fat to reduce postprandial blood lipid levels increases. This weakens the inhibitory effect on the deleterious impact of postprandial blood lipid levels on the development of atherosclerosis, thereby accelerating the onset and progression of atherosclerosis (28). Additionally, liver-induced metabolic disorders can exacerbate cardiovascular endothelial damage through various pathophysiological mechanisms, including oxidative stress, inflammation, and endothelial dysfunction (10,11). Therefore, considering the impact of adipose tissue metabolism on the development and progression of atherosclerosis, the assessment of the degree of ectopic fat deposition in the liver is more critical than the assessment of individual obesity indicators. Ultrasound and magnetic resonance imaging (MRI) are two conventional imaging modalities for detecting hepatic fat accumulation (15,29). However, ultrasound results may be influenced by the operator’s experience and instruments. MRI is expensive and time-consuming, limiting its clinical applicability. Although conventional CT is frequently used, its sensitivity is limited. Choi et al. (30) indicated that low-kVp unenhanced CT can effectively diagnose hepatic steatosis while reducing radiation exposure. In comparison, SDCT, as a new imaging technology, can provide more spectral imaging information than conventional CT (31-34). In addition, many studies have shown that the energy spectral indicators of spectral CT have a significant correlation with pathological fat deposition in the liver (35,36). Thus, SDCT multiparameter quantitative metrics may better capture early pathological changes in the liver parenchyma.
Consistent with the findings of previous study (37), patients with metabolic abnormalities were more likely to develop coronary plaques in our study. However, after adjusting for common cardiovascular risk factors, the categorization of Zeff based on SDCT remained an independent risk factor for the extent and severity of coronary plaque involvement, especially among those <60 years, males and those with a VAT/SAT <1.18. Given SDCT imaging properties, Zeff is more sensitive to the change in fat deposition in the liver than other collinear indicators; a lower Zeff value means more fatty infiltration in the liver. This condition is more prone to triggering metabolic disorders, such as hyperlipidaemia, insulin resistance, and aggravation of systemic low-grade inflammatory levels; however, these factors also damage the cardiovascular endothelium (38,39). Additional metabolic risk factors are more frequently present in older adults owing to their impaired metabolic performance (40); thus, the damage to the cardiovascular endothelium caused by hepatic steatosis may be masked by other stronger coronary risk factors. Previous research indicates that women with obesity and type 2 DM lose their cardioprotective effects (41), and microvascular dysfunction is more common in women (42). Therefore, men also tend to have fewer metabolic risk factors than women, which could account for the stronger correlation we found in our study between the quantitative liver parameters and high coronary plaque scores in male. At the same time, in the lower VAT/SAT ratio subgroup, cardiovascular risk factors such as older age (48.9% vs. 53.3%) and DM (21.4% vs. 22.6%) accounted for a relatively small proportion, making the coronary plaque burden caused by liver lipid metabolism disorders appear more prominent. Previous study indicated that the SIS and SSS are associated with the long-term poor prognosis following coronary heart disease (21), and Tanaka et al. (28) demonstrated that higher SAT and lower VAT were inversely correlated with the extent and severity of coronary artery plaques, independent of sex and age, but the study only reflected the difference in the amount of fat distribution rather than the qualitative change of the metabolic state of the body. Moreover, high liver fat content can largely explain the metabolic state of high insulin, hyperglycaemia, hypertriglyceridemia, and elevated apolipoprotein B in visceral obesity (43). However, focusing solely on visceral fat obesity may lead to overlooking individuals with lean fatty liver. Despite this, the study by Semmler et al. demonstrated that lean nonalcoholic fatty liver disease is also associated with metabolic syndrome and increased cardiovascular disease risk (44). The liver, being a sensitive organ of ectopic fat deposition, can more accurately reflect the metabolic imbalance of lipids in the body, and SDCT examination enhances detection sensitivity. Liver multi-parameter metrics based on SDCT can aid in identifying high-risk populations for cardiovascular and cerebrovascular disorders. In clinical practice, it is recommended to utilize SDCT for longitudinal follow-up assessments to evaluate changes in hepatic fat metabolism status. Additionally, early and secondary prevention of diseases should be enhanced through guiding clinical dietary and exercise interventions to reduce the occurrence of adverse cardiovascular outcomes in the future.
There are some limitations in this study. First, histological diagnosis of hepatic steatosis was not validated. Second, because this was a single-centre cross-sectional study with a small sample size, individuals with inadequate clinical data were excluded, which may have led to a selection bias. Consequently, this may exert an influence on the interpretation and generalizability of the study findings. Third, the measurement indicators of liver SDCT were derived from a fixed-size ROI in the right lobe of the liver, which may not reflect the degree of fat deposition throughout the entire liver. Technologies based on artificial intelligence are already capable of assessing VAT, such as pericardial adipose tissue (45) and abdominal adipose tissue (46). This development will offer a new perspective for the subsequent development of artificial intelligence for liver fat quantification based on SDCT, which is also one of our upcoming research directions. Finally, we only explored the relationship between liver SDCT parameters and populations at high risk of coronary disease, and the diagnostic evidence for the prognosis of cardiovascular disease is insufficient. We will conduct a follow-up study to verify the auxiliary incremental predictive value of spectral CT for the long-term prognosis of cardiovascular disease.
Conclusions
Liver multi-parameter metrics can better reflect the changes in liver tissue components than polychromatic images metrics and are closely associated with the extent and severity of coronary plaque scores. In particular, liver quantitative indicators assessed by Zeff based on SDCT may be useful for evaluating the risk of CAD. Early identification of liver tissue components is beneficial for screening individuals at high risk of cardiovascular ailments and enabling early clinical intervention.
Acknowledgments
The authors would like to acknowledge Xiaomei Lu from CT Clinical Science, Philip Healthcare China, for the assistance with CT technology.
Funding: This work was supported by
Footnote
Reporting Checklist: The authors have completed the STROBE reporting checklist. Available at https://qims.amegroups.com/article/view/10.21037/qims-24-53/rc
Conflicts of Interest: All authors have completed the ICMJE uniform disclosure form (available at https://qims.amegroups.com/article/view/10.21037/qims-24-53/coif). The authors have no conflicts of interest to declare.
Ethical Statement: The authors are accountable for all aspects of the work in ensuring that questions related to the accuracy or integrity of any part of the work are appropriately investigated and resolved. The study was conducted in accordance with the Declaration of Helsinki (as revised in 2013). The study was approved by the Ethics Committee of Shengjing Hospital of China Medical University (No. 2022PS958K) and informed consent was taken from all individual participants.
Open Access Statement: This is an Open Access article distributed in accordance with the Creative Commons Attribution-NonCommercial-NoDerivs 4.0 International License (CC BY-NC-ND 4.0), which permits the non-commercial replication and distribution of the article with the strict proviso that no changes or edits are made and the original work is properly cited (including links to both the formal publication through the relevant DOI and the license). See: https://creativecommons.org/licenses/by-nc-nd/4.0/.
References
- He L, Lui KO, Zhou B. The Formation of Coronary Vessels in Cardiac Development and Disease. Cold Spring Harb Perspect Biol 2020;12:a037168. [Crossref] [PubMed]
- Benjamin EJ, Virani SS, Callaway CW, Chamberlain AM, Chang AR, Cheng S, et al. Heart Disease and Stroke Statistics-2018 Update: A Report From the American Heart Association. Circulation 2018;137:e67-e492. [Crossref] [PubMed]
- Sandesara PB, Virani SS, Fazio S, Shapiro MD. The Forgotten Lipids: Triglycerides, Remnant Cholesterol, and Atherosclerotic Cardiovascular Disease Risk. Endocr Rev 2019;40:537-57. [Crossref] [PubMed]
- Ridker PM, Danielson E, Fonseca FA, Genest J, Gotto AM Jr, Kastelein JJ, Koenig W, Libby P, Lorenzatti AJ, MacFadyen JG, Nordestgaard BG, Shepherd J, Willerson JT, Glynn RJJUPITER Study Group. Rosuvastatin to prevent vascular events in men and women with elevated C-reactive protein. N Engl J Med 2008;359:2195-207. [Crossref] [PubMed]
- Lin A, Wong ND, Razipour A, McElhinney PA, Commandeur F, Cadet SJ, Gransar H, Chen X, Cantu S, Miller RJH, Nerlekar N, Wong DTL, Slomka PJ, Rozanski A, Tamarappoo BK, Berman DS, Dey D. Metabolic syndrome, fatty liver, and artificial intelligence-based epicardial adipose tissue measures predict long-term risk of cardiac events: a prospective study. Cardiovasc Diabetol 2021;20:27. [Crossref] [PubMed]
- Meyersohn NM, Mayrhofer T, Corey KE, Bittner DO, Staziaki PV, Szilveszter B, Hallett T, Lu MT, Puchner SB, Simon TG, Foldyna B, Voora D, Ginsburg GS, Douglas PS, Hoffmann U, Ferencik M. Association of Hepatic Steatosis With Major Adverse Cardiovascular Events, Independent of Coronary Artery Disease. Clin Gastroenterol Hepatol 2021;19:1480-1488.e14. [Crossref] [PubMed]
- Yu MM, Tang XL, Jin H, Yang S, Yun H, Wang QB, Zeng MS, Coronary CT. Angiography in Asymptomatic Adults with Hepatic Steatosis. Radiology 2021;301:593-601. [Crossref] [PubMed]
- Puchner SB, Lu MT, Mayrhofer T, Liu T, Pursnani A, Ghoshhajra BB, Truong QA, Wiviott SD, Fleg JL, Hoffmann U, Ferencik M. High-risk coronary plaque at coronary CT angiography is associated with nonalcoholic fatty liver disease, independent of coronary plaque and stenosis burden: results from the ROMICAT II trial. Radiology 2015;274:693-701. [Crossref] [PubMed]
- Doumas SA, Tripathi S, Kashikar A, Khuttan A, Kumar A, Singh H, Canakis JP, Ashish K, Dey D, Oppenheim I, Dey AK. Nonalcoholic Fatty Liver Disease (NAFLD) and Cardiovascular Risk: Is Imaging Helpful? Curr Probl Cardiol 2024;49:102065. [Crossref] [PubMed]
- Simon TG, Roelstraete B, Hagström H, Sundström J, Ludvigsson JF. Non-alcoholic fatty liver disease and incident major adverse cardiovascular events: results from a nationwide histology cohort. Gut 2022;71:1867-75. [Crossref] [PubMed]
- Targher G, Byrne CD, Tilg H. NAFLD and increased risk of cardiovascular disease: clinical associations, pathophysiological mechanisms and pharmacological implications. Gut 2020;69:1691-705. [Crossref] [PubMed]
- Hsu PF, Wang YW, Lin CC, Wang YJ, Ding YZ, Liou TL, Huang SS, Lu TM, Chan WL, Lin SJ, Leu HB. The association of the steatosis severity in fatty liver disease with coronary plaque pattern in general population. Liver Int 2021;41:81-90. [Crossref] [PubMed]
- Wong VW, Wong GL, Yeung JC, Fung CY, Chan JK, Chang ZH, Kwan CT, Lam HW, Limquiaco J, Chim AM, Yu CM, Chan HL. Long-term clinical outcomes after fatty liver screening in patients undergoing coronary angiogram: A prospective cohort study. Hepatology 2016;63:754-63. [Crossref] [PubMed]
- Ozturk A, Grajo JR, Gee MS, Benjamin A, Zubajlo RE, Thomenius KE, Anthony BW, Samir AE, Dhyani M. Quantitative Hepatic Fat Quantification in Non-alcoholic Fatty Liver Disease Using Ultrasound-Based Techniques: A Review of Literature and Their Diagnostic Performance. Ultrasound Med Biol 2018;44:2461-75. [Crossref] [PubMed]
- Ferraioli G, Soares Monteiro LB. Ultrasound-based techniques for the diagnosis of liver steatosis. World J Gastroenterol 2019;25:6053-62. [Crossref] [PubMed]
- Yokoo T, Serai SD, Pirasteh A, Bashir MR, Hamilton G, Hernando D, Hu HH, Hetterich H, Kühn JP, Kukuk GM, Loomba R, Middleton MS, Obuchowski NA, Song JS, Tang A, Wu X, Reeder SB, Sirlin CBRSNA-QIBA PDFF Biomarker Committee. Linearity, Bias, and Precision of Hepatic Proton Density Fat Fraction Measurements by Using MR Imaging: A Meta-Analysis. Radiology 2018;286:486-98. [Crossref] [PubMed]
- Bohte AE, van Werven JR, Bipat S, Stoker J. The diagnostic accuracy of US, CT, MRI and 1H-MRS for the evaluation of hepatic steatosis compared with liver biopsy: a meta-analysis. Eur Radiol 2011;21:87-97. [Crossref] [PubMed]
- Morita K, Nishie A, Ushijima Y, Takayama Y, Fujita N, Kubo Y, Ishimatsu K, Yoshizumi T, Maehara J, Ishigami K. Noninvasive assessment of liver fibrosis by dual-layer spectral detector CT. Eur J Radiol 2021;136:109575. [Crossref] [PubMed]
- Zeng YR, Yang QH, Liu QY, Min J, Li HG, Liu ZF, Li JX. Dual energy computed tomography for detection of metastatic lymph nodes in patients with hepatocellular carcinoma. World J Gastroenterol 2019;25:1986-96. [Crossref] [PubMed]
- Abdelrahman KM, Chen MY, Dey AK, Virmani R, Finn AV, Khamis RY, Choi AD, Min JK, Williams MC, Buckler AJ, Taylor CA, Rogers C, Samady H, Antoniades C, Shaw LJ, Budoff MJ, Hoffmann U, Blankstein R, Narula J, Mehta NN. Coronary Computed Tomography Angiography From Clinical Uses to Emerging Technologies: JACC State-of-the-Art Review. J Am Coll Cardiol 2020;76:1226-43. [Crossref] [PubMed]
- Min JK, Shaw LJ, Devereux RB, Okin PM, Weinsaft JW, Russo DJ, Lippolis NJ, Berman DS, Callister TQ. Prognostic value of multidetector coronary computed tomographic angiography for prediction of all-cause mortality. J Am Coll Cardiol 2007;50:1161-70. [Crossref] [PubMed]
- Andreini D, Pontone G, Mushtaq S, Bartorelli AL, Bertella E, Antonioli L, Formenti A, Cortinovis S, Veglia F, Annoni A, Agostoni P, Montorsi P, Ballerini G, Fiorentini C, Pepi M. A long-term prognostic value of coronary CT angiography in suspected coronary artery disease. JACC Cardiovasc Imaging 2012;5:690-701. [Crossref] [PubMed]
- Hideo-Kajita A, Garcia-Garcia HM, Wopperer SB, Freire AFD, Ozaki Y, Cavalcante R, Bittencourt MS, Dan K, Soud M, Pinheiro TL, Falcão BAA, Falcão JLA, Soares P, Ribeiro E, Rochitte CE, Lemos PA. Correlation between computed tomography adapted leaman score and computed tomography liver and spleen attenuation parameters for non-alcoholic fatty liver disease as well as respective inflammatory mediators. Int J Cardiovasc Imaging 2020;36:2383-91. [Crossref] [PubMed]
- Lavie CJ, De Schutter A, Milani RV. Healthy obese versus unhealthy lean: the obesity paradox. Nat Rev Endocrinol 2015;11:55-62. [Crossref] [PubMed]
- Lavie CJ, Sharma A, Alpert MA, De Schutter A, Lopez-Jimenez F, Milani RV, Ventura HO. Update on Obesity and Obesity Paradox in Heart Failure. Prog Cardiovasc Dis 2016;58:393-400. [Crossref] [PubMed]
- Romero-Corral A, Montori VM, Somers VK, Korinek J, Thomas RJ, Allison TG, Mookadam F, Lopez-Jimenez F. Association of bodyweight with total mortality and with cardiovascular events in coronary artery disease: a systematic review of cohort studies. Lancet 2006;368:666-78. [Crossref] [PubMed]
- Després JP. Body fat distribution and risk of cardiovascular disease: an update. Circulation 2012;126:1301-13. [Crossref] [PubMed]
- Tanaka T, Kishi S, Ninomiya K, Tomii D, Koseki K, Sato Y, Okuno T, Sato K, Koike H, Yahagi K, Komiyama K, Aoki J, Tanabe K. Impact of abdominal fat distribution, visceral fat, and subcutaneous fat on coronary plaque scores assessed by 320-row computed tomography coronary angiography. Atherosclerosis 2019;287:155-61. [Crossref] [PubMed]
- Starekova J, Hernando D, Pickhardt PJ, Reeder SB. Quantification of Liver Fat Content with CT and MRI: State of the Art. Radiology 2021;301:250-62. [Crossref] [PubMed]
- Choi Y, Kim DK, Youn SY, Kim H, Choi JI. Unenhanced computed tomography for non-invasive diagnosis of hepatic steatosis with low tube potential protocol. Quant Imaging Med Surg 2022;12:1348-58. [Crossref] [PubMed]
- Zhao XM, Wang M, Wu RZ, Dharaiya E, Feng F, Li ML, You H, Wang Y, Wang YN, Jin ZY. Dual-layer spectral detector CT monoenergetic reconstruction improves image quality of non-contrast cerebral CT as compared with conventional single energy CT. Eur J Radiol 2018;103:131-8. [Crossref] [PubMed]
- Bai X, Gao P, Zhang D, Zhang S, Liang J, Lu X, Sui B. Plaque burden assessment and attenuation measurement of carotid atherosclerotic plaque using virtual monoenergetic images in comparison to conventional polyenergetic images from dual-layer spectral detector CT. Eur J Radiol 2020;132:109302. [Crossref] [PubMed]
- Albrecht MH, De Cecco CN, Schoepf UJ, Spandorfer A, Eid M, De Santis D, Varga-Szemes A, van Assen M, von Knebel-Doeberitz PL, Tesche C, Puntmann VO, Nagel E, Vogl TJ, Nance JW. Dual-energy CT of the heart current and future status. Eur J Radiol 2018;105:110-8. [Crossref] [PubMed]
- Gao L, Lu X, Wen Q, Hou Y. Added value of spectral parameters for the assessment of lymph node metastasis of lung cancer with dual-layer spectral detector computed tomography. Quant Imaging Med Surg 2021;11:2622-33. [Crossref] [PubMed]
- Zheng X, Ren Y, Phillips WT, Li M, Song M, Hua Y, Zhang G. Assessment of hepatic fatty infiltration using spectral computed tomography imaging: a pilot study. J Comput Assist Tomogr 2013;37:134-41. [Crossref] [PubMed]
- Noh H, Song XL, Heo SH, Kim JW, Shin SS, Ahn KY, Jeong YY, Kang HK. Comparative Study of Ultrasonography, Computed Tomography, Magnetic Resonance Imaging, and Magnetic Resonance Spectroscopy for the Diagnosis of Fatty Liver in a Rat Model. J Korean Soc Radiol 2017;76:14-24. [Crossref]
- van den Hoogen IJ, van Rosendael AR, Lin FY, Lu Y, Dimitriu-Leen AC, Smit JM, et al. Coronary atherosclerosis scoring with semiquantitative CCTA risk scores for prediction of major adverse cardiac events: Propensity score-based analysis of diabetic and non-diabetic patients. J Cardiovasc Comput Tomogr 2020;14:251-7. [Crossref] [PubMed]
- Tada H, Nohara A, Kawashiri MA. Serum Triglycerides and Atherosclerotic Cardiovascular Disease: Insights from Clinical and Genetic Studies. Nutrients 2018;10:1789. [Crossref] [PubMed]
- Hill MA, Yang Y, Zhang L, Sun Z, Jia G, Parrish AR, Sowers JR. Insulin resistance, cardiovascular stiffening and cardiovascular disease. Metabolism 2021;119:154766. [Crossref] [PubMed]
- Ciumărnean L, Milaciu MV, Negrean V, Orășan OH, Vesa SC, Sălăgean O, Iluţ S, Vlaicu SI. Cardiovascular Risk Factors and Physical Activity for the Prevention of Cardiovascular Diseases in the Elderly. Int J Environ Res Public Health 2021;19:207. [Crossref] [PubMed]
- Colafella KMM, Denton KM. Sex-specific differences in hypertension and associated cardiovascular disease. Nat Rev Nephrol 2018;14:185-201. [Crossref] [PubMed]
- Coutinho T, Mielniczuk LM, Srivaratharajah K, deKemp R, Wells GA, Beanlands RS. Coronary artery microvascular dysfunction: Role of sex and arterial load. Int J Cardiol 2018;270:42-7. [Crossref] [PubMed]
- Adiels M, Taskinen MR, Packard C, Caslake MJ, Soro-Paavonen A, Westerbacka J, Vehkavaara S, Häkkinen A, Olofsson SO, Yki-Järvinen H, Borén J. Overproduction of large VLDL particles is driven by increased liver fat content in man. Diabetologia 2006;49:755-65. [Crossref] [PubMed]
- Semmler G, Wernly S, Bachmayer S, Wernly B, Schwenoha L, Huber-Schönauer U, Stickel F, Niederseer D, Aigner E, Datz C. Nonalcoholic Fatty Liver Disease in Lean Subjects: Associations With Metabolic Dysregulation and Cardiovascular Risk-A Single-Center Cross-Sectional Study. Clin Transl Gastroenterol 2021;12:e00326. [Crossref] [PubMed]
- Greco F, Salgado R, Van Hecke W, Del Buono R, Parizel PM, Mallio CA. Epicardial and pericardial fat analysis on CT images and artificial intelligence: a literature review. Quant Imaging Med Surg 2022;12:2075-89. [Crossref] [PubMed]
- Greco F, Mallio CA. Artificial intelligence and abdominal adipose tissue analysis: a literature review. Quant Imaging Med Surg 2021;11:4461-74. [Crossref] [PubMed]