Association of synuclein alpha (SNCA) gene polymorphisms with spontaneous brain activity in patients with Parkinson’s disease
Introduction
Parkinson’s disease (PD) is the second most common neurodegenerative disease, characterized by loss of dopaminergic neuron in the substantia nigra pars compacta (SNpc) and the formation of Lewy bodies (LBs) and Lewy neurites (1-3). The synuclein alpha (SNCA) gene is an alpha synuclein (α-synuclein) gene and is located at 4q21-q23. The α-synuclein encoded by the SNCA gene is the main component of LBs, which is the characteristic pathological change of PD (4). Mutations in the SNCA gene lead to the misfolding and aggregation of α-synuclein, resulting in neurotoxicity and contributing to the pathogenesis and development of PD (4,5).
In the past few decades, numerous studies have focused on the relationship between single-nucleotide polymorphisms (SNPs) in the SNCA gene and PD susceptibility (6-8). Furthermore, it has been suggested that this association may be caused by the cis-regulation of SNCA gene expression. A meta-analysis that included 36 studies from different countries and regions showed that eight SNP sites of SNCA are related to the risk of PD. Seven SNPs, namely rs2736990, rs356220, rs356165, rs181489, rs356219, rs11931074, and rs2737029, are associated with PD risk, while rs356186 is associated with a low risk of PD. In Southeast Asian populations, rs2736990 and rs11931074 are associated with an increased risk of PD, while in European populations, rs356219, rs181489, rs2737029, rs356165, and rs11931074 are associated with an increased risk of PD, while rs356186 is linked with a lower risk of PD (6). Another study of 1,061 Chinese Han patients with PD showed that seven SNCA SNPs, including rs356165 and rs11931074, are related to PD (9). Other relevant studies have shown that in the Chinese population, the T allele of rs11931074 can increase the risk of developing PD (10) and is related to early-onset PD (11), while the G allele plays a protective role against development of PD (10). In a different study, SNCA rs11931074 was associated with α-synuclein deposition in the gastric and colonic mucosa of patients with PD (12). Another study suggested that the SNCA rs356219 variant may confer an increased susceptibility risk to PD in the Chinese Han population (13). In summary, SNPs rs11931074, rs356219, and rs356165 have been reported to be associated with a susceptibility risk to PD in the Chinese Han population.
Since the effects of PD on brain networks may occur early in the disease process, the findings of functional imaging studies have implications for the diagnosis of PD (14,15). As a noninvasive, repeatable imaging technique, resting-state functional magnetic resonance imaging (rs-fMRI) (16,17) is becoming an important method for the diagnosis of human functional brain disorders (18). rs-fMRI measures brain activity by detecting blood oxygen level-dependent (BOLD) signal changes. The data processing method used in this study was the amplitude of low frequency fluctuation (ALFF) analysis method. For measuring the BOLD signal of the spontaneous brain activity in the resting state, the ALFF approach was first proposed by Zang et al. (19) and then improved upon to the fractional ALFF (fALFF) approach by Zuo et al. (20). ALFF records the BOLD signal, which changes across time with the spontaneous activity of brain neurons in the resting state and uses low frequency signals to reflect the strength of the spontaneous activity of brain neurons. Fractional amplitude of low-frequency fluctuation (fALFF) is the ratio of the amplitude of the low frequency band (0.01–0.08 Hz) to the amplitude of the entire frequency band, which can effectively reduce signals from nonspecific brain areas such as ventricles and large blood vessels and improve sensitivity and specificity (20). ALFF and fALFF need to be normalized before statistical analysis through mean standardization (dividing by the mean of the whole brain signal) and Z transformation (the deviation from the mean divided by the standard deviation of the whole brain signal), with mean ALFF (mALFF), z-score ALFF (zALFF), mean fALFF (mfALFF), and z-score fALFF (zfALFF) each being obtained to improve the normality of the data. The mALFF offers a more precise measurement of low-frequency amplitude signals by modifying the original ALFF values, while zALFF standardizes these values into z-scores for more standardized and fair comparisons across individuals. The combined use of these methods is intended to provide a more comprehensive and accurate framework for analyzing and interpreting the differences in resting-state brain activity. The rs-fMRI technique measures spontaneous brain activity to reflect brain function. Thus far, several rs-fMRI studies in patients with PD have found that changes in ALFF values in certain brain areas can distinguish patients with PD from normal control participants (21,22).
Neuroimaging genetics research has helped elucidate the relationship between genetic variations and the structure and function of the human brain (23). Several studies have reported that the Parkin RBR E3 Ubiquitin Protein Ligase (PRKN), Parkinsonism-associated deglycase (PARK7), HtrA2 serine peptidase 2 (HTRA2), and SNCA genes are significantly correlated with the imaging phenotype of PD (24,25). In contrast, fewer studies have been conducted for examining the relationship between genetic polymorphisms and brain function. Some studies have suggested that the SNCA SNPs rs11931074 (26) and rs894278 (27) may be related to changes in brain activity and motor symptoms in patients with PD; moreover, in Chinese patients with PD, SNCA rs11931074 polymorphisms may modulate altered brain function and may be associated with motor symptoms (26). Additionally, changes in brain function may precede the development of clinical symptoms. Based on these findings, we reasoned that the rs11931074, rs356219, and rs356165 polymorphisms can influence PD clinical symptoms through the abnormal expression of SNCA and the deposition of SNCA and thus hypothesized that SNPs could affect brain function in PD.
To test this hypothesis, this study aimed to characterize the relationship between resting-state brain function and the SNCA rs11931074, rs356219, and rs356165 genotypes in patients with PD and matched control participants. In addition, the relationship between resting-state functional brain activity and cognitive function, disease course, and severity in patients with PD was examined. We present this article in accordance with the STROBE reporting checklist (available at https://qims.amegroups.com/article/view/10.21037/qims-24-14/rc).
Methods
Participants
In this cross-sectional study, data were retrospectively collected from 63 patients with PD and 73 sex- and age-matched healthy control (HC) participants who attended the outpatient and inpatient clinics at Fujian Medical University Union Hospital from August 2017 to November 2019. The patients with PD enrolled were clinically diagnosed according to the Movement Disorder Society (MDS) clinical diagnostic criteria for PD (2015 edition) by two neurology specialists (28). All participants were clinically evaluated by two senior attending physicians who had more than 20 years of relevant experience in neurology department. The exclusion criteria for this study were as follows: (I) secondary parkinsonism and Parkinson-plus syndrome; (II) severe dementia, psychiatric diseases, neurodevelopmental diseases, anxiety, or depression; (III) incomplete data, including for MRI images and clinical evaluations; (IV) brain tumors, hydrocephalus, or other brain diseases found in brain MRI images; (V) severe complications of diabetes and hypertension; and (VI) poor MRI image quality. MRI scans and clinical examinations of patients with PD were performed at least 12 h after withdrawal of their pharmacological treatment. This study was conducted in accordance with the Declaration of Helsinki (as revised in 2013) and was approved by the Institutional Ethics Committee of Fujian Medical University Union Hospital (No. 2019K013). The requirement for individual consent in this retrospective analysis was waived.
Clinical assessments
The general and clinical data of participants, including name, age, education, and medication, were collected from both groups. The Unified Parkinson’s Disease Rating Scale Part III (UPDR-III) (29), Hoehn-Yahr (H-Y) stage (30), and the Mini-Mental State Examination (MMSE) score (31) were used to assess the severity of motor symptoms and cognitive impairment in patients with PD. We implemented a standardized training session for all evaluators before the study began and blinded the evaluators to the study hypotheses and group assignments to ensure consistency in the measurement process.
SNCA genotyping
Elbow venous blood (5 mL) was collected from all participants, DNA was extracted from blood samples using the TIANamp Blood DNA Kit (TianGen Biotech Co., Ltd., Beijing, China), and SNPs were detected via polymerase chain reaction (PCR) amplification and Sanger DNA sequencing. The specific primers used in the above PCR amplification are listed in Table S1 and were designed for SNCA rs356219, rs11931074, and rs356165. The PCR amplification reaction procedure is detailed in Table S2. Participants were stratified into genotype groups based on their alleles at specific SNPs to investigate the potential associations with PD and to facilitate comparison to the HC group. For the rs11931074 SNP, the genotype groups were defined as GG, GT, and TT. For rs356219 and rs356165, participants were categorized into GG, GA, and AA genotype groups.
MRI data acquisition and processing
MRI data acquisition
Images of all patients were acquired using a Discovery 750 3.0 T MRI scanner (GE HealthCare, Chicago, IL, USA), using standard HDx 8NV Head_A coil channels as transmitting and receiving coils. During scanning, participants were awake and lying quietly on the examination bed with their eyes closed and head fixed. Both groups underwent conventional brain MRI scans, including T1/T2-weighted imaging, T2 fluid-attenuated inversion recovery (FLAIR) imaging, and diffusion-weighted imaging, among others. The rs-fMRI data were collected via an echo-planar imaging (EPI) sequence under the following parameters: repetition time (TR) =2,000 ms; echo time (TE) =30 ms; flip angle =90°; matrix =64×64; field of view (FOV) =256×256 mm2; voxel size =4×4×4 mm3; slice thickness =4 mm; slice number =40; slice sequence, continuous ascending scanning; and scanning time point =200.
MRI data preprocessing and ALFF analysis
Functional MRI data analyses were performed using MRIcron Software (https://www.nitrc.org/projects/mricron), Connectivity Toolbox (CONN) software (https://www.nitrc.org/projects/conn), and the toolbox for Data Processing & Analysis of Brain Imaging (DPABI) implemented in the MATLAB R2013b platform (MathWorks, Natick, MA, USA) (32). The following steps were performed (as shown in Figure S1): after image preprocessing, fast Fourier transform (FFT) was performed on the filtered time series to transform the time-domain signal to the frequency threshold to obtain the power spectrum using CONN. ALFF was calculated using the DPABI tool by taking the square root of the power spectrum. The amplitude of the analysis frequency band was divided by the amplitude of the full frequency band to obtain the fALFF diagram. For standardization purposes, the ALFF or fALFF value of each voxel was divided by the global mean ALFF value to obtain the mALFF or mfALFF value via the DPABI tool. In addition, Z transformation was performed, in which the deviation of the ALFF or fALFF value of each voxel was divided by the standard deviation of the whole brain ALFF of fALFF value in order to better align the data with a normal distribution and to obtain zALFF and zfALFF, respectively. An automated anatomical labeling (AAL) brain atlas was used to mark brain areas with significant differences in ALFF values between different groups. The presentation of significant differences in brain regions was implemented using the Xjview 95 software.
Statistical analysis
The demographic and clinical data of the patients were analyzed using SPSS 22.0 software (IBM Corporation, Armonk, NY, USA), and the measurement data are expressed as the mean ± standard deviation. The Hardy-Weinberg equilibrium (HWE) of the SNCA rs11931074, rs356219, and rs356165 in the PD group and HC group were determined using the Chi-square test, and the linkage disequilibrium analysis was realized on the SHEsis platform (33) (http://analysis.bio-x.cn/myAnalysis.php). For data with a normal distribution and uniform variance, a two-sample t-test was used to compare data of two independent samples, and an analysis of variance (ANOVA) test was used to compare data from multiple independent samples. The Wilcoxon rank sum test was used to compare data that did not conform to a normal distribution and that had uneven variance. The data from the four grid tables were analyzed using the Chi-square test for the R×C contingency tables. All statistical tests were two-sided, and P<0.05 was considered statistically significant.
The magnetic resonance functional data were analyzed with SPM12 software (https://www.fil.ion.ucl.ac.uk/spm/software/SPM12). The comparison of the brain functional data in the PD group and the HC group was performed with a two-sample t-test, and the covariates included age, gender, and MMSE score. Multivariate regression analysis was used to calculate the correlation between the brain function data and MMSE score, UPDRS III score, and disease course, with the covariates being age and gender. For the analysis of the influence of rs11931074 on brain function data, full factor analysis was used to analyze the main effects of diseases and genes and their interaction effects on functional data, with the covariates being age and gender. Post hoc comparisons were performed using the Bonferroni test. The two-sample t-test was used to analyze the differences in functional data between G-allele carriers and non-G allele carriers, with the covariates being age, gender, and MMSE score. The analysis process for the influence of rs356219 and rs356165 on brain functional data were the same as that for rs11931074. All rs-fMRI data analyzed with the SPM12 software were adjusted according to the false discovery rate (FDR) at the cluster level. Brain regions with a voxel level of P<0.001 and a cluster level of P<0.05 were considered statistically significant.
Results
Demographic and clinical characteristics
Participants in this study included 63 patients with PD in the PD group and 73 sex- and age-matched healthy participants in the HC group (Figure S2). The inheritance of SNCA SNPs rs11931074, rs356219, and rs356165 in the PD group and the HC group was in accordance with the HWE and was constant (P>0.05; Table S3), with the three SNP sites conforming to the linkage disequilibrium (Table S4). There was no statistically significant differences in sex, age, education, or the levodopa equivalent daily dose (LEDD) between the PD and HC groups. The MMSE score of the PD group was lower than that of the HC group (P<0.001; Table 1). For the different genotype groups (GG, GA/GT, TT/AA) of rs11931074, rs356219, and rs356165 in the PD group, there were no significant differences in sex, age, MMSE score, UPDRS III score, disease course, H-Y stage, or LEDD. Moreover, the sex, age, and MMSE score among the genotype groups in the PD and HC group showed no statistically significant differences (Tables 2-4). In addition, there were no significant differences in age, sex, education, MMSE score, UPDRS III score, H-Y stage, disease course, or LEDD between the rs11931074 G-allele carriers (GG + GT group) and non-G allele carriers (TT group) in patients with PD or among all participants (Table S5).
Table 1
Characteristic | PD (n=63) | HC (n=73) | P |
---|---|---|---|
Age, years | 63.24±7.99 | 63.03±6.78 | 0.868a |
Sex (male/female) | 37/26 | 33/40 | 0.116b |
MMSE score | 24.89±2.76 | 27.40±1.57 | <0.001c*** |
UPDRS III score | 30.67±11.34 | – | – |
Course of disease (months) | 54.51±41.93 | – | – |
Hoehn-Yahr stage | 2.36±0.55 | – | – |
Education (1/2/3/4/5) | 10/16/12/17/8 | 3/14/25/22/9 | 0.075c |
LEDD, mg/day | 377.3 ±236.2 | – | – |
Data are presented as the mean ± standard deviation or number. a, two-sample t-test; b, Chi-square test; c, Kruskal-Wallis test. 1, illiterate; 2, elementary school; 3, junior high school; 4, high school/technical secondary school; 5, university/college. ***, P<0.001. PD, Parkinson’s disease; HC, healthy control; MMSE, Mini-Mental State Examination; UPDRS III, Unified Parkinson’s Disease Rating Scale Part III; LEDD, levodopa equivalent daily dose.
Table 2
Characteristic | Group | GG | GT | TT | P |
---|---|---|---|---|---|
Age, years | PD | 65.80±9.78 | 64.00±9.25 | 61.89±8.54 | 0.658c |
HC | 60.58±9.12 | 64.21±5.62 | 62.63±6.86 | 0.132c | |
Sex (male/female) | PD | 2/3 | 19/12 | 16/11 | 0.793d |
HC | 3/9 | 15/19 | 15/12 | 0.206b | |
MMSE score | PD | 24.80±2.86 | 24.84±2.61 | 24.96±3.01 | 0.871c |
HC | 27.58±1.16 | 27.44±1.81 | 27.26±1.43 | 0.637c | |
UPDRS III score | PD | 30.00 ±10.05 | 28.10±10.87 | 33.74±11.71 | 0.167e |
Course of disease (months) | PD | 40.20± 29.01 | 48.26±31.59 | 64.33±52.19 | 0.548c |
Hoehn-Yahr stage | PD | 2.50±0.00 | 2.23±0.55 | 2.44±0.64 | 0.535c |
LEDD, mg/day | PD | 250.00±165.83 | 365.27±221.61 | 414.57±259.67 | 0.330c |
Data are presented as the mean ± standard deviation or number. b, Chi-square test; c, Kruskal-Wallis test; d, Fisher exact test; e, ANOVA. PD, Parkinson’s disease; HC, healthy control; GG, rs11931074 GG genotype; GT, rs11931074 GT genotype; TT, rs11931074 TT genotype; MMSE, Mini-Mental State Examination; UPDRS III, Unified Parkinson’s Disease Rating Scale Part III; LEDD, levodopa equivalent daily dose; ANOVA, analysis of variance.
Table 3
Characteristic | Group | GG | GA | AA | P |
---|---|---|---|---|---|
Age | PD | 61.89±8.54 | 64.33±7.13 | 63.83±9.99 | 0.742c |
HC | 62.32±6.93 | 64.52±5.40 | 60.58±9.12 | 0.09c | |
Sex (male/female) | PD | 16/11 | 19/11 | 2/4 | 0.401d |
HC | 15/13 | 15/18 | 3/9 | 0.250b | |
MMSE score | PD | 24.96±3.01 | 24.90±2.63 | 24.50±2.67 | 0.819c |
HC | 27.29±1.41 | 27.42±1.84 | 27.58±1.16 | 0.679c | |
UPDRS III score | PD | 33.74±11.71 | 28.17±11.05 | 29.33±9.14 | 0.173e |
Course of disease (months) | PD | 64.33±52.19 | 47.87±32.05 | 43.50±27.25 | 0.581c |
Hoehn-Yahr stage | PD | 2.44±0.64 | 2.27±0.50 | 2.50±0.00 | 0.600c |
LEDD, mg/day | PD | 414.57±259.67 | 367.45± 225.06 | 258.33 ±149.72 | 0.332c |
Data are presented as the mean ± standard deviation or number. b, Chi-square test; c, Kruskal-Wallis test; d, Fisher exact test; e, ANOVA. PD, Parkinson’s disease; HC, healthy control; GG, rs356219 GG genotype; GA, rs356219 GA genotype; AA, rs356219 AA genotype; MMSE, Mini-Mental State Examination; UPDRS III, Unified Parkinson’s Disease Rating Scale Part III; LEDD, levodopa equivalent daily dose; ANOVA, analysis of variance.
Table 4
Characteristic | Group | GG | GA | AA | P |
---|---|---|---|---|---|
Age | PD | 61.89±8.54 | 64.33±7.13 | 63.83±9.99 | 0.742c |
HC | 62.26±7.05 | 64.50±5.33 | 60.58± 9.12 | 0.089c | |
Sex (male/female) | PD | 16/11 | 19/12 | 2/3 | 0.793d |
HC | 14/13 | 16/18 | 3/9 | 0.286b | |
MMSE score | PD | 24.96±3.01 | 24.90±2.63 | 24.50±2.66 | 0.819c |
HC | 27.26±1.43 | 27.44±1.81 | 27.44±1.81 | 0.637c | |
UPDRS III score | PD | 33.74±11.71 | 28.17±11.05 | 29.33±9.14 | 0.173e |
Course of disease (months) | PD | 64.33±52.19 | 47.87±32.05 | 43.50±27.25 | 0.581c |
Hoehn-Yahr stage | PD | 2.44±0.64 | 2.27±0.50 | 2.50±0.00 | 0.600c |
LEDD, mg/day | PD | 414.57±259.67 | 365.27±221.61 | 250.00±165.83 | 0.330c |
Data are presented as the mean ± standard deviation or number. b, Chi-square test; c, Kruskal-Wallis test; d, Fisher exact test; e, ANOVA. PD, Parkinson’s disease; HC, healthy control; GG, rs356165 GG genotype; GA, rs356165 GA genotype; AA, rs356165 AA genotype; MMSE, Mini-Mental State Examination; UPDRS III, Unified Parkinson’ Disease Rating Scale Part III; LEDD, levodopa equivalent daily dose; ANOVA, analysis of variance.
ALFF analysis
ALFF analysis between the PD group and HC group
The mALFF, and zALFF values of the right superior cerebellum, cerebellar vermis, and left supplementary motor area were decreased in patients in the PD group as compared with those in the HC group (Table S6, Figure 1A,1B). There was no significant difference in the mfALFF or zfALFF values of brain area between the HC group and PD group.
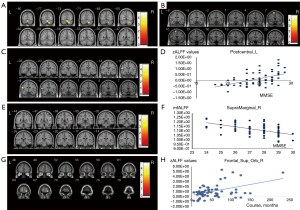
Correlation analysis of ALFF values and the clinical data of the PD group and HC group
The zALFF values of the left central posterior gyrus of the PD group were positively correlated with the MMSE score (r=0.542; P<0.001; Figure 1C,1D, Table S7). The mfALFF values of the upper right margin of the HC group were negatively correlated with the MMSE score to a moderate extent (r=0.528; P<0.001; Figure 1E,1F, Table S7). The zALFF values of the right orbital superior frontal gyrus in the PD group was positively and weakly correlated with disease course (r=0.311; P= 0.13; Figure 1G,1H, Table S7).
ALFF analysis of the rs11931074 group
We performed a full factorial analysis on the ALFF maps. The analysis of mALFF maps and zALFF maps showed a significant main genotype effect on the left inferior cerebellum (Figure 2A, Table S8). Analysis of the mfALFF maps and zfALFF maps showed a significant main genotype effect on the right anterior cingulate and paracingulate gyri (Figure 2B, Table S8).
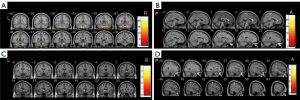
Among all participants, compared with the GG + GT group, the TT group had higher mfALFF and zfALFF values in the left caudate nucleus (Figure 2C, Table S8), but no significant differences in brain areas were observed between the mALFF and zALFF values. In the PD group, compared with the PD-TT group, the PD-GG + GT group had higher mfALFF and zfALFF values in the right postcentral gyrus (Figure 2D, Table S8).
ALFF analysis of the rs356219 group
The full factorial analysis revealed significant genotype effects in the left caudate nucleus for mfALFF values in the PD group (Figure 3A, Table S9). A significant interaction effect was found in the right inferior parietal gyrus for zfALFF values (Figure 3B, Table S9). Further post hoc comparison showed that the zfALFF values of the right inferior parietal gyrus of the PD-GG group were lower than those of the PD-GA group and PD-AA group, while the zfALFF values of the right inferior parietal gyrus (Parietal_Inf_R,) of the HC-GG group was higher than that of the HC-GA group and HC-AA group (Figure 3C).
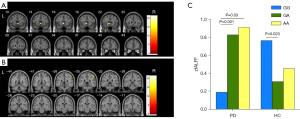
ALFF analysis of the rs356165 group
The full factorial analysis revealed that the mfALFF and zfALFF values of the left caudate nucleus had significant genotype main effects (Figure 4, Table S10), but there was no significant disease main effect or interaction effect found for the mfALFF and zfALFF values.
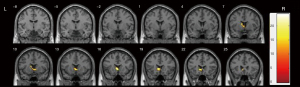
Discussion
The aim of the this study was to investigate the association of SNCA SNPs rs11931074, rs356219, and rs356165 using ALFF in patients with PD and HCs. We found that the mALFF values and zALFF values of the right cerebellum, cerebellar vermis, and the left supplementary motor area in the PD group were lower than those in the HC group. Additionally, the zALFF values of the left central posterior gyrus of the PD group were positively correlated with the MMSE score. The zALFF values of the superior frontal gyrus of the right orbit were positively correlated with the course of PD. The rs11931074 genotype main effect brain areas were the left cerebellum, the right anterior cingulate gyrus, and the paracingulate gyrus. The rs356219 and rs356165 genotype main effect brain area was the left caudate nucleus, and the disease × rs356219 genotype interaction effect brain area was the right inferior parietal gyrus. Spontaneous functional brain activity was enhanced in the right central posterior gyrus in patients with PD carrying the rs11931074 G allele. These findings provide evidence to support the notion that the SNCA SNPs rs11931074, rs356219, and rs356165 are involved in brain function in both patients with PD and HC participants.
The preclinical and prodromal stage of PD can precede the onset of typical motor symptoms in patients with PD by several years (14). People with a known genetic risk of developing PD and without motor symptoms are suitable candidates for studying the preclinical and prodromal stages of PD. A study using ALFF analysis found that in the PD group, this index showed a decrease in activity in a number of regions, including the supplementary motor cortex, the mesial prefrontal cortex, the right middle frontal gyrus, and the left cerebellum (21). Another rs-fMRI study showed that, compared to baseline, the fALFF values of the right cerebellum, right thalamus, right striatum, left superior parietal lobule, left inferior parietal lobule, left central anterior gyrus, and left central posterior gyrus were lower in the PD group than in the control group after two years. Further correlation analysis revealed that the fALFF values of the right cerebellum were positively correlated with the UPDRS score. It has been suggested that the cortical-basal ganglia-thalamic-cortical loop and cerebellar-thalamic-cortical loop dysfunctions are involved in the development of PD, and the spontaneous neural activity of the cerebellum may play an important role in cognitive function and movement in patients with PD (34). This is speculated to be related to dopaminergic neuron degeneration, abnormal signal transmission from the basal ganglia, and dopamine replacement therapy. Our study found that the spontaneous neurological activity of the left supplementary motor area, the right cerebellum, and cerebellar vermis were weakened in patients of the PD group, which is similar to the findings of previous studies (34). However, we found no abnormality in certain brain areas, such as the right thalamus and right striatum, which may be attributed to the fact that different studies may employ different samples, imaging techniques, and data-processing methods. Specifically, mALFF accurately measures brain activity by focusing on low-frequency amplitudes, while zALFF standardizes these measurements for consistent comparisons across individuals. Combining both methods, we aimed to gather a detailed and comparable overview of brain activity during rest.
Previous studies have examined the relationship between fMRI activity and genes in patients with PD, such as COMT gene rs4680 and MAPT gene rs9468 (35). Zhang et al. evaluated the effect of the SNCA SNP rs894278 site on resting-brain function in patients with PD and found that the ALFF values of the lingual gyrus and left caudate nucleus in patients with PD were lower than those in the control group (27). Some studies have shown that the T allele at rs11931074 is associated with an increased risk of developing PD in the Chinese population and that the G allele plays a protective role in patients with PD (10). Another study reported a significant interaction of disease and gene genotype in the right gyrus, suggesting that the SNCA rs11931074 polymorphism may modulate brain function changes in Chinese patients with PD and may be associated with motor symptoms (26,36). Our study also showed that for all participants, the mfALFF and zfALFF values in the left caudate nucleus of the non-G allele carriers of rs11931074 were higher than those of the G-allele carriers. The caudate nucleus is one of the components of the striatum, which plays an important role in the cortical-basal ganglia-thalamic-cortical loop in PD. The central posterior gyrus is located in the parietal lobe and also has a close structural and functional connection with the striatum. In our study, the mfALFF and zfALFF values in the right central posterior gyrus of the G-allele carriers were higher than those of the non-G allele carriers in the PD group. These findings suggest that the rs11931074 G allele may participate in the pathogenesis and development of PD by affecting the function of the left caudate nucleus and the right central posterior gyrus. Moreover, this points to an interaction effect of gene and disease on functional brain activity, with this association potentially being prominently implicated in the pathogenesis of PD.
In this study, rs356219 and rs356165 were found in genes that have major effects on the left caudate nucleus, and these two SNPs exert an important effect on the spontaneous brain activity in the left caudate nucleus during the occurrence and development of PD. The angular gyrus is related to the nonmotor symptoms of PD, such as cognitive dysfunction and emotional disorder (36,37). Studies have found that patients with PD and PD with dementia (PDD) may have reduced grey matter volume (GMV) in portions of the occipital lobes, temporal lobes, and hippocampus (38). A meta-analysis of the GMV in patients with PDD reported a significantly reduced regional GMV in some brain regions including the right angular gyrus in the patients with PDD compared with HC participants (39). Additionally, in our study, significant interaction effects were observed in the right inferior parietal marginal angular gyrus, which is consistent with the findings in structural imaging studies (39). Another study showed that the functional brain activity of the posterior putamen and primary motor cortex was weakened in heathy participants with rs356219 G, and the functional connection between the posterior putamen and motor cortex was reduced when participants performed motor tasks, suggesting that carriers of the rs356219 PD-risk allele have abnormal function of the striatal-thalamic-cortical loop (40). Moreover, the study found the zfALFF value of the rs356219 locus showed a significant disease-gene interaction effect in the right parietal marginal gyrus. Further comparison revealed that the spontaneous brain activity of the right inferior parietal marginal angular gyrus of the PD-GG genotype group was weaker than that of the PD-GA and PD-AA genotype groups, while the spontaneous brain activity of the right inferior parietal marginal angular gyrus of the HC-GG genotype group was higher than that of HC-GA genotype (40). The study also indicated that in healthy participants, carrying rs356219 G can lead to abnormal functional activity in specific brain regions, which can explain the abnormal activity across the different genotypes (40). This finding suggests that the rs356219 G allele has a different effect on the functional brain activity of those with PD versus in those without PD, which further confirms that the rs356219 G allele is a PD risk allele. With consideration to previous studies and our results, we can surmise that different genes or the different loci of the same gene may have different effects on the brain function of different regions of the brain. Thus, variation in specific gene polymorphic loci may have more significant effects in brain regions with higher expression levels and less effects in other regions. The interaction of genes and environmental factors may affect various brain regions to various degrees. The phenotypic effects of specific gene polymorphism loci may be more pronounced in some brain regions when interacting with environmental factors.
It is worth noting that among the three SNCA SNPs (rs11931074, rs356219, and rs356165), the interaction effect of brain regions were only found for rs356219. However, how the mutation of the rs356219 gene locus affects the spontaneous functional brain activity of the right inferior parietal marginal angular gyrus remains to be further elucidated. This result also suggests that future studies can concentrate on the rs356219 locus and the related effects of gene polymorphisms on the spontaneous functional activity of the brain and further clarify its impact on functional brain activity during the development of PD.
Conclusions
We evaluated the association of SNCA SNPs rs11931074, rs356219, and rs356165 and ALFF in patients with PD and HC participants and found altered spontaneous activity in certain brain regions that was associated with PD status and genotype. Our results also revealed that the interaction effect of brain regions was only present for rs356219. Furthermore, changes in the functional brain activity may play a key role in the prediagnosis of PD. Overall, the findings of this study may contribute to furthering our understanding of the influence of genes on brain function and may also help arrive at deeper insights into the mechanisms underlying PD pathogenesis via imaging.
Limitations
This study involved certain limitations which should be mentioned. First, in the analysis the correlation between ALFF values and UPDRS III scores in this study, there was no correlation analysis for the “on” or “off” state of the UPDRS III scores, respectively. Therefore, in the next study, the motor assessment of the “on” and “off” state could be separately evaluated in order to study the brain network connection and neuron activity of PD in the “on and off” states, respectively. Second, we employed a cross-sectional study without follow-up and thus could not discern the changes of functional brain activity related to the progress of PD. Third, compared with ALFF, fALFF has improved sensitivity and specificity for detecting spontaneous brain activity. However, the mechanism of this signal is still unclear. The fALFF technique does not include bandpass filtering, and so similarly to ALFF, concerns about its biological artifacts remain. Finally, our study only examined the association of the SNCA SNPs rs11931074, rs356219, and rs356165 with ALFF in sporadic patients with PD and matched HC participants. Brain spontaneous activity might be influenced by additional gene variants, such as other SNCA SNPs and other gene variants. Thus, the linkage disequilibrium between rs11931074, rs356219, and rs356165 or the others, gene-gene interactions, and more complex haplotypes should be investigated in future work.
Acknowledgments
Funding: This study was supported by grants from
Footnote
Reporting Checklist: The authors have completed the STROBE reporting checklist. Available at https://qims.amegroups.com/article/view/10.21037/qims-24-14/rc
Conflicts of Interest: All authors have completed the ICMJE uniform disclosure form (available at https://qims.amegroups.com/article/view/10.21037/qims-24-14/coif). The authors have no conflicts of interest to declare.
Ethical Statement:
Open Access Statement: This is an Open Access article distributed in accordance with the Creative Commons Attribution-NonCommercial-NoDerivs 4.0 International License (CC BY-NC-ND 4.0), which permits the non-commercial replication and distribution of the article with the strict proviso that no changes or edits are made and the original work is properly cited (including links to both the formal publication through the relevant DOI and the license). See: https://creativecommons.org/licenses/by-nc-nd/4.0/.
References
- Recchia A, Debetto P, Negro A, Guidolin D, Skaper SD, Giusti P. Alpha-synuclein and Parkinson's disease. FASEB J 2004;18:617-26. [Crossref] [PubMed]
- Emamzadeh FN, Surguchov A. Parkinson's Disease: Biomarkers, Treatment, and Risk Factors. Front Neurosci 2018;12:612. [Crossref] [PubMed]
- Jankovic J, Tan EK. Parkinson's disease: etiopathogenesis and treatment. J Neurol Neurosurg Psychiatry 2020;91:795-808. [Crossref] [PubMed]
- Shahmohammadibeni N, Rahimi-Aliabadi S, Jamshidi J, Emamalizadeh B, Shahmohammadibeni HA, Zare Bidoki A, et al. The analysis of association between SNCA, HUSEYO and CSMD1 gene variants and Parkinson's disease in Iranian population. Neurol Sci 2016;37:731-6. [Crossref] [PubMed]
- Ghosh D, Mehra S, Sahay S, Singh PK, Maji SK. α-synuclein aggregation and its modulation. Int J Biol Macromol 2017;100:37-54. [Crossref] [PubMed]
- Zhang Y, Shu L, Sun Q, Pan H, Guo J, Tang B. A Comprehensive Analysis of the Association Between SNCA Polymorphisms and the Risk of Parkinson's Disease. Front Mol Neurosci 2018;11:391. [Crossref] [PubMed]
- Pihlstrøm L, Blauwendraat C, Cappelletti C, Berge-Seidl V, Langmyhr M, Henriksen SP, van de Berg WDJ, Gibbs JR, Cookson MR, Singleton AB, Nalls MA, Toft M. A comprehensive analysis of SNCA-related genetic risk in sporadic parkinson disease. Ann Neurol 2018;84:117-29. [Crossref] [PubMed]
- Du B, Xue Q, Liang C, Fan C, Liang M, Zhang Y, Bi X, Hou L. Association between alpha-synuclein (SNCA) rs11931074 variability and susceptibility to Parkinson's disease: an updated meta-analysis of 41,811 patients. Neurol Sci 2020;41:271-80. [Crossref] [PubMed]
- Guo JF, Li K, Yu RL, Sun QY, Wang L, Yao LY, Hu YC, Lv ZY, Luo LZ, Shen L, Jiang H, Yan XX, Pan Q, Xia K, Tang BS. Polygenic determinants of Parkinson's disease in a Chinese population. Neurobiol Aging 2015;36:1765.e1-6. [Crossref] [PubMed]
- Liu J, Xiao Q, Wang Y, Xu ZM, Wang Y, Yang Q, Wang G, Tan YY, Ma JF, Zhang J, Huang W, Chen SD. Analysis of genome-wide association study-linked loci in Parkinson's disease of Mainland China. Mov Disord 2013;28:1892-5. [Crossref] [PubMed]
- Huang Y, Wang G, Rowe D, Wang Y, Kwok JB, Xiao Q, Mastaglia F, Liu J, Chen SD, Halliday G. SNCA Gene, but Not MAPT, Influences Onset Age of Parkinson's Disease in Chinese and Australians. Biomed Res Int 2015;2015:135674. [PubMed]
- Chung SJ, König IR, Lohmann K, Hinrichs F, Kim J, Ryu HS, Lee HJ, Kim K, Lee JH, Jung KW, Kim MJ, Kim MJ, Kim YJ, Yun SC, Hong SM, Myung SJ, Klein C. Association of SNCA variants with α-synuclein of gastric and colonic mucosa in Parkinson's disease. Parkinsonism Relat Disord 2019;61:151-5. [Crossref] [PubMed]
- Pan F, Dong H, Ding H, Ye M, Liu W, Wu Y, Zhang X, Chen Z, Luo Y, Ding X. SNP rs356219 of the α-synuclein (SNCA) gene is associated with Parkinson's disease in a Chinese Han population. Parkinsonism Relat Disord 2012;18:632-4. [Crossref] [PubMed]
- Meles SK, Oertel WH, Leenders KL. Circuit imaging biomarkers in preclinical and prodromal Parkinson's disease. Mol Med 2021;27:111. [Crossref] [PubMed]
- Tolosa E, Garrido A, Scholz SW, Poewe W. Challenges in the diagnosis of Parkinson's disease. Lancet Neurol 2021;20:385-97. [Crossref] [PubMed]
- Filippi M, Basaia S, Sarasso E, Stojkovic T, Stankovic I, Fontana A, Tomic A, Piramide N, Stefanova E, Markovic V, Kostic VS, Agosta F. Longitudinal brain connectivity changes and clinical evolution in Parkinson's disease. Mol Psychiatry 2021;26:5429-40. [Crossref] [PubMed]
- Tessitore A, Cirillo M, De Micco R. Functional Connectivity Signatures of Parkinson's Disease. J Parkinsons Dis 2019;9:637-52. [Crossref] [PubMed]
- Detre JA, Floyd TF. Functional MRI and its applications to the clinical neurosciences. Neuroscientist 2001;7:64-79. [Crossref] [PubMed]
- Zang YF, He Y, Zhu CZ, Cao QJ, Sui MQ, Liang M, Tian LX, Jiang TZ, Wang YF. Altered baseline brain activity in children with ADHD revealed by resting-state functional MRI. Brain Dev 2007;29:83-91. [Crossref] [PubMed]
- Zou QH, Zhu CZ, Yang Y, Zuo XN, Long XY, Cao QJ, Wang YF, Zang YF. An improved approach to detection of amplitude of low-frequency fluctuation (ALFF) for resting-state fMRI: fractional ALFF. J Neurosci Methods 2008;172:137-41. [Crossref] [PubMed]
- Skidmore FM, Yang M, Baxter L, von Deneen KM, Collingwood J, He G, White K, Korenkevych D, Savenkov A, Heilman KM, Gold M, Liu Y. Reliability analysis of the resting state can sensitively and specifically identify the presence of Parkinson disease. Neuroimage 2013;75:249-61. [Crossref] [PubMed]
- Tang Y, Meng L, Wan CM, Liu ZH, Liao WH, Yan XX, Wang XY, Tang BS, Guo JF. Identifying the presence of Parkinson's disease using low-frequency fluctuations in BOLD signals. Neurosci Lett 2017;645:1-6. [Crossref] [PubMed]
- Gerber AJ, Peterson BS, Muñoz KE, Hyde LW, Hariri AR. Imaging genetics. J Am Acad Child Adolesc Psychiatry 2009;48:356-61. [Crossref] [PubMed]
- van Nuenen BF, van Eimeren T, van der Vegt JP, Buhmann C, Klein C, Bloem BR, Siebner HR. Mapping preclinical compensation in Parkinson's disease: an imaging genomics approach. Mov Disord 2009;24:S703-10. [Crossref] [PubMed]
- Kim M, Kim J, Lee SH, Park H. Imaging genetics approach to Parkinson's disease and its correlation with clinical score. Sci Rep 2017;7:46700. [Crossref] [PubMed]
- Si QQ, Yuan YS, Zhi Y, Wang M, Wang JW, Shen YT, Wang LN, Li JY, Wang XX, Zhang KZ. SNCA rs11931074 polymorphism correlates with spontaneous brain activity and motor symptoms in Chinese patients with Parkinson's disease. J Neural Transm (Vienna) 2019;126:1037-45. [Crossref] [PubMed]
- Zhang K, Tang Y, Meng L, Zhu L, Zhou X, Zhao Y, Yan X, Tang B, Guo J. The Effects of SNCA rs894278 on Resting-State Brain Activity in Parkinson's Disease. Front Neurosci 2019;13:47. [Crossref] [PubMed]
- Postuma RB, Berg D, Stern M, Poewe W, Olanow CW, Oertel W, Obeso J, Marek K, Litvan I, Lang AE, Halliday G, Goetz CG, Gasser T, Dubois B, Chan P, Bloem BR, Adler CH, Deuschl G. MDS clinical diagnostic criteria for Parkinson's disease. Mov Disord 2015;30:1591-601. [Crossref] [PubMed]
- The Unified Parkinson's Disease Rating Scale (UPDRS): status and recommendations. Mov Disord 2003;18:738-50. [Crossref] [PubMed]
- Hoehn MM, Yahr MD. Parkinsonism: onset, progression and mortality. Neurology 1967;17:427-42. [Crossref] [PubMed]
- Katzman R, Zhang MY. Ouang-Ya-Qu, Wang ZY, Liu WT, Yu E, Wong SC, Salmon DP, Grant I. A Chinese version of the Mini-Mental State Examination; impact of illiteracy in a Shanghai dementia survey. J Clin Epidemiol 1988;41:971-8. [Crossref] [PubMed]
- Yan CG, Wang XD, Zuo XN, Zang YF. DPABI: Data Processing & Analysis for (Resting-State) Brain Imaging. Neuroinformatics 2016;14:339-51. [Crossref] [PubMed]
- Shi YY, He L. SHEsis, a powerful software platform for analyses of linkage disequilibrium, haplotype construction, and genetic association at polymorphism loci. Cell Res 2005;15:97-8. [Crossref] [PubMed]
- Hu XF, Zhang JQ, Jiang XM, Zhou CY, Wei LQ, Yin XT, Li J, Zhang YL, Wang J. Amplitude of low-frequency oscillations in Parkinson's disease: a 2-year longitudinal resting-state functional magnetic resonance imaging study. Chin Med J (Engl) 2015;128:593-601. [Crossref] [PubMed]
- Nombela C, Rowe JB, Winder-Rhodes SE, Hampshire A, Owen AM, Breen DP, Duncan GW, Khoo TK, Yarnall AJ, Firbank MJ, Chinnery PF, Robbins TW, O'Brien JT, Brooks DJ, Burn DJ, Barker RA. Genetic impact on cognition and brain function in newly diagnosed Parkinson's disease: ICICLE-PD study. Brain 2014;137:2743-58. [Crossref] [PubMed]
- Wang L, Day J, Roe CM, Brier MR, Thomas JB, Benzinger TL, Morris JC, Ances BM. The effect of APOE ε4 allele on cholinesterase inhibitors in patients with Alzheimer disease: evaluation of the feasibility of resting state functional connectivity magnetic resonance imaging. Alzheimer Dis Assoc Disord 2014;28:122-7. [Crossref] [PubMed]
- Burton EJ, McKeith IG, Burn DJ, Williams ED, O'Brien JT. Cerebral atrophy in Parkinson's disease with and without dementia: a comparison with Alzheimer's disease, dementia with Lewy bodies and controls. Brain 2004;127:791-800. [Crossref] [PubMed]
- Cromarty RA, Schumacher J, Graziadio S, Gallagher P, Killen A, Firbank MJ, Blamire A, Kaiser M, Thomas AJ, O'Brien JT, Peraza LR, Taylor JP. Structural Brain Correlates of Attention Dysfunction in Lewy Body Dementias and Alzheimer's Disease. Front Aging Neurosci 2018;10:347. [Crossref] [PubMed]
- Xu X, Han Q, Lin J, Wang L, Wu F, Shang H. Grey matter abnormalities in Parkinson's disease: a voxel-wise meta-analysis. Eur J Neurol 2020;27:653-9. [Crossref] [PubMed]
- Burciu RG, Seidler RD, Shukla P, Nalls MA, Singleton AB, Okun MS, Vaillancourt DE. Multimodal neuroimaging and behavioral assessment of α-synuclein polymorphism rs356219 in older adults. Neurobiol Aging 2018;66:32-9. [Crossref] [PubMed]