Diameter-dependent classification of dermal vasculature using optical coherence tomography angiography
Introduction
Cutaneous alteration with aging has been extensively studied for decades, with increasing interest in the medical and beauty industries. Given that the skin tissue is the outermost layer of the human body, extrinsic aging (photoaging) occurs due to exposure to the sun. Extrinsically aged skin is characterized by rough skin textures, uneven pigmentation, and deep wrinkles (1-4). Superficial vascular alterations, such as telangiectasias (5,6) and a decrease in capillary vessels (7,8) can also occur. These capillary alterations may be associated with changes in epidermal homeostasis (9). Non-invasive measurements of dermal vasculature would be beneficial to assess various skin conditions.
Based on optical technology, non-invasive assessment systems for assessing dermal vasculature have been developed. Representative measurement techniques include photoacoustic microscopy (10) and optical coherence tomography angiography (OCTA) (11). In the dermatological field, the OCTA system is considered to be beneficial for evaluating the capillaries beneath the epidermis with capillary-level resolution (11,12). Using OCTA, it has been suggested that skin vascularity is associated with various skin conditions in which dermal-epidermal interactions play an important role, for example, solar lentigo, psoriasis, and wound healing (13-15). Furthermore, OCTA could evaluate vascular alteration with photoaging (16,17). Using OCTA and OCT, our previous study indicated decreasing vessel density and epidermal thinning with photoaging (16). One of the limitations in the prior OCTA studies, including ours, is the use of two-dimensional (2D) vessel metrics (e.g., area density and diameter) to analyze 2D vascular images generated by the maximum intensity projection (MIP) of the 3D OCTA dataset. MIP analysis is particularly useful when tail-like artifacts emerge beneath blood vessels because these artifacts can prevent OCTA from visualizing the three-dimensional (3D) structural images of vascular networks (18,19). As Untracht et al. (20) demonstrated, 3D-based vessel analysis can potentially visualize the details of vascular geometry that cannot be observed using 2D analysis. Several attempts have been made to explore the possibility of 3D-based vascular analysis in OCTA measurements, showing improved vessel density assessments (21-23). Because 3D analysis is considered to retain more information about the actual vascular architecture than 2D analysis, it is beneficial for classifying the characteristics of each vessel’s functional type, such as capillary and post-capillary venules. For instance, capillaries can be recognized as thin vessels comprising a limited number of pericyte layers, implying a potential connection between their structures and the maintenance of epidermal homeostasis via nutrient supply (24). These vessel types could be roughly characterized based on their diameters in the dermatological field (25); however, this has not been proposed.
This study aimed to develop a diameter-dependent analysis method for dermal blood vessels exhibiting the complex structure shown in Figure 1. Various types of vessel metrics that preserve 3D information were proposed. The effects of photoaging and the correlation with epidermal thickness of diameter-dependent vascular types were investigated using the proposed method. We present this article in accordance with the STROBE reporting checklist (available at https://qims.amegroups.com/article/view/10.21037/qims-23-1790/rc).
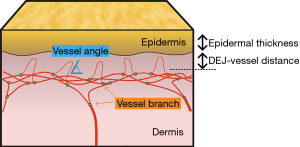
Methods
Optical coherence tomography (OCT) system
In this study, we employed a prototype swept-source type OCT (SS-OCT) system equipped with a swept-source laser with a central wavelength of 1,310 nm and a scanning rate of 200 kHz (SL1310 V1-20048, Thorlabs, Inc., NJ, USA). The SS-OCT system was designed with a handheld measurement probe, including a galvanometric mirror (GV S002, Thorlabs, Inc.) and an objective lens (LSM03, Thorlabs, Inc.) (26). The system delivers a dynamic range of ~105 dB with an axial resolution of ~6 µm in the tissues and a lateral resolution of ~15 µm. The field of view (FoV) was designed to be 8 mm × 8 mm. To achieve OCTA imaging, the volume scanning pattern for this FoV included 800 A-scans per B-scan and 800 B-scans per volume, with each B-scan repeated four times. This scanning pattern provided a pixel spacing between adjacent A-scans of 10 µm (pixel size of 10 µm in x and y dimensions). In the axial direction (z dimension), the pixel size was 3.75 µm. The penetration depth was approximately 2.0 mm at maximum; however, due to the attenuation of the OCT signal, it can be visualized up to a depth of 140 pixels (500 µm) from the surface of the skin. To further improve the image quality for quantitative analysis, the volume scan was repeated twice at the targeted region of interest and then registered and averaged to give rise to a final single volume for later quantification (see below). The total scanning time to obtain the final single volume was approximately 26 s.
Post-processing
After data acquisition using the SS-OCT system, the raw OCT data were processed using several imaging filters: an optical microangiography (OMAG) imaging filter, a vesselness imaging filter, and a diameter map filter, as shown in the flowchart in Figure 2A. The OMAG technique was used to convert the OCT signal into an angiographic image with high accuracy (11,27). In this method, the OCT signal C can be described as follows:
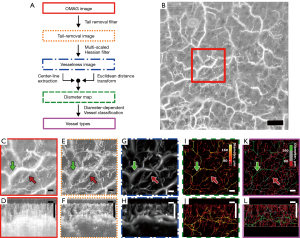
where A(r, t) is the amplitude component of the OCTA signal, F(r, t) is the phase component, and r is the spatial position of the OCTA signal. In the OMAG imaging, the intensity (IOMAG) of the image due to blood flow was calculated using Eq. [2].
where N is the number of repeats of the B-scans and Ci(r) is the OCT signal at the spatial position r during the i-th repetition of the B-scan. A representative example of an OCTA image of human forearm skin is shown in Figure 2B,2C. As we know, an OCTA image naturally contains noise in the z-direction, called a tail-like artifact (28), which can be easily appreciated in each B-scan OCTA image (Figure 2D). While there are several methods available in the literature for tail artifact removal [e.g., (28)], here we adopted a method using an exponentially decaying function in the z-direction (29) to remove the tailing artifacts in the OCTA datasets. In this case, the tailing artifact removed from the OMAG image ITailRemoved can be expressed as:
where represents the distance in the z-direction (depth) from the superficial vessel, a0 is the decay coefficient, and k is the decay rate that determines the degree of decay. The operation of this tail-removal filter would result in tail artifact-free OCTA images (Figure 2E,2F).
Next, the tubular structure (i.e., vesselness) of the vessels was extracted by assuming that the shape of the dermal vessels in OCTA is tubular. A multiscale Hessian filter was applied to the tail-removed image to obtain a vesselness image as follows:
where H represents the 3D Hessian matrix, defined as . The tubular structure was extracted by calculating the eigenvalues of the Hessian matrix (Figure 2G,2H). In the vesselness imaging filter, the tubular structures were set to a non-zero value, whereas other structure types, such as planes or blobs, were set to zero. While calculating the Hessian matrix, the maximum value of vesselness for each position was determined by varying the vesselness scale s from 0.1 to 10 with logarithmic step size (30,31). Lastly, the tubular vessel structures derived through the above process were skeletonized to shrink all vessel types into one pixel in size (i.e., vessel cross-section only represented by a one-pixel width). In doing so, a centerline extraction filter was passed through the vessel image. The decision-tree method was used for this process (32). In this method, pixels were removed from the outermost layer of the tube, resulting in a skeleton of the vesselness image. The diameter-based information within the vessel was color-mapped onto the skeleton using a method developed using the Euclidean distance transform (33) to preserve the actual 3D morphology of the blood vessels (Figure 2I,2J). Finally, diameter-dependent vessel classification was achieved based on a visual observation of the OMAG images and diameter maps.
Vessel metrics
In this study, various vessel metrics were derived from the vessel images generated through post-processing and are summarized in Table 1. Figure 1 illustrates the vessel metrics for skin tissue. The epidermal thickness (ET) was determined by detecting the dermal-epidermal interface, that is, the dermal-epidermal junction (DEJ), using intensity profiles of OCT signals (34). All the vessel-related indicators, except ET, were calculated using the depth obtained from ET detection as the starting depth. The DEJ-vessel distance was defined as the distance from the location of the DEJ to the depth where the vascular density in the MIP image exceeded 5%, which is the estimated threshold excluding the noise such as dot-like structures through vesselness filters. This metric indicates that the smaller the value, the greater the number of blood vessels present beneath the epidermis. The vessel area fraction (VAF) was calculated from the MIP image relative to the vessel from the depth of the DEJ to 80-pixel depth (300 µm) by considering the individual variations in the epidermal thickness and the degree of attenuation. The VAF was calculated by counting the pixel number of the MIP of the vesselness image. The equation can be written as follows:
Table 1
Metric | Abbrv. | Unit | Description |
---|---|---|---|
Epidermal thickness | ET | μm | ET of a skin tissue |
DEJ-vessel distance | – | μm | Distance between dermal-epidermal junction and peripheral blood vessels |
Vessel areal fraction | VAF | % | MIP density of the vesselness image |
Vessel volume density | VVD | % | Vessel density calculated by the vessel diameter map (all vessels) |
Micro-vessel volume density | mVVD | % | Vessel density of micro-vessels calculated by the vessel diameter map (a diameter of ≤80 μm) |
Intermediate-vessel volume density | iVVD | % | Vessel density of intermediate-vessels calculated by the vessel diameter map (80 μm < a diameter <160 μm) |
Thick vessel volume density | tVVD | % | Vessel density of thick vessels calculated by the vessel diameter map (a diameter of ≥160 μm) |
Vessel branch | – | m−3 | Number of branching points of blood vessels per volume |
Vessel angle | mVA | ° | Mean angle of peripheral micro-vessels (horizontal =0°, vertical =90°) |
DEJ, dermal-epidermal junction; MIP, maximum intensity projection.
where a represents the area of the ROI, MIP is an operation function taking the max value along the z-direction at position (x,y), and COUNT is an operation function for counting the number of pixels at which the MIP value was non-zero, namely, tubular structure.
The volume density of the vessels from the depth of the DEJ to the 80-pixel depth was denoted as the vessel volume density (VVD). The VVD was calculated by converting the diameter of the skeleton into the effective area of the tubular section and integrating it over the skeleton. VVD can be written as:
where π represents a circular constant, R represents the radius of the voxel in the skeleton, and the integrated volume is divided by the total voxel volume n within the analysis range. The integration process was diameter selective because VVD was calculated using a diameter map image. Here, VVDs comprising micro-vessels, intermediate vessels and thick vessels were defined as micro-vessel volume density (mVVD), intermediate-vessel volume density (iVVD) and thick vessel volume density (tVVD), respectively. Correlations between ET and the diameter-selective VVDs were investigated to validate the diameter thresholds by setting up with two VVDs, i.e., VVDs of vessels with diameters larger or smaller than a given threshold.
The number of vessel branches was derived by calculating the number of branching points from the skeletonized vessels and dividing it by the volume of the analysis, ranging from the depth of the DEJ to 80-pixel depth. The micro-vessel angle (mVA) was defined as the mean angle of the core line from the depth of the DEJ to an 11-pixel depth (approximately 40 µm) corresponding to the thickness of the papillary dermis (35), focusing on the angle in the micro-vessels of the superficial layer. The mVA was derived by calculating the arctangent from the slope of the least-square regression line. This operation was conducted along the skeleton, and the box size of the least-square regression was set to be (7,7,7) voxels. The mVA can be expressed as:
where l is the slope derived from the least-square regression analysis. The absolute value was taken to be in the range of the resulting angle from 0° to 90°.
Subjects
The study was conducted in accordance with the Declaration of Helsinki (as revised in 2013) and approved by the Ethics Committee of Shiseido Co., Ltd. (No. C02109). The subjects were 129 healthy Asian females aged 20–79 years, they were enrolled after obtaining informed consent. The measurement positions were on the right sides of their cheeks, in the sun-exposed area. The measurements began after a moderate acclimatization period at approximately 23.0 ℃ and 45% RH. Five subjects were excluded due to the large body motion during OCTA measurements. The number of subjects, identified on the basis of the Fitzpatrick classification of skin type, were I (n=1), II (n=3), III (n=53), IV (n=61), and V (n=6), as determined using a questionnaire. The subjects were divided into three groups based on age, G0 (20–39 years old), G1 (40–59 years old) and G2 (60–79 years old), to examine the relationship between age and vessel metrics. Table 2 summarizes the population, smoking habits, and hormone status for each age group.
Table 2
Group | Age range (years) | Subject number | Fitzpatrick skin type | Smoking habits | Postmenopausal | ||||
---|---|---|---|---|---|---|---|---|---|
I | II | III | IV | V | |||||
G0 | 20–39 | 37 | 0 | 2 | 14 | 19 | 2 | 5 | 0 |
G1 | 40–59 | 44 | 0 | 1 | 19 | 21 | 3 | 2 | 17 |
G2 | 60–79 | 43 | 1 | 0 | 20 | 21 | 1 | 3 | 43 |
Statistical analysis
The Wilcoxon rank correlation test, in which P values were adjusted using the Bonferroni method, was employed to investigate whether there were statistically significant differences between the groups. Spearman’s rank correlation coefficients were calculated for the vessel metrics.
Results
Figure 2E-2L show vascular images passing through our post-processing filters after being processed using the OMAG algorithm. The tail-like artifacts in the OMAG image (Figure 2E,2F) were eliminated by applying the tail removal filter. Additionally, the multi-scaled Hessian filter enhanced the visibility of the vessel structures (Figure 2G,2H). Skeletonization and Euclidean distance transformation of the vesselness image allowed for a resulting diameter map in 3D (Figure 2I,2J). Visual observations of the OMAG images and the diameter maps (e.g., Figure 2C,2I) allowed for a diameter-dependent vessel classification, resulting in micro-vessels, estimated as having a diameter less than 80 µm, intermediate-vessels, estimated having a diameter between 80 and 160 µm, and thick vessels, estimated having a diameter greater than 160 µm (Figure 2K,2L). Following these post-processing steps, a 3D vascular structure was visualized, with micro-vessels predominantly in the upper layer of the dermis and thicker vessels in the subsequent layers.
Figure 3 illustrates the aging alteration for the age groups of G0, G1 and G2. Aging alterations in ET and VVD were plotted as boxplots in Figure 3A,3B. Figure 3C,3D present a typical vesselness image for G0, G1, and G2. ET shows a decreasing trend with age. Conversely, no statistically significant difference was found in VVD between groups G0 and G1, although a significant difference was observed for groups G0 and G2.
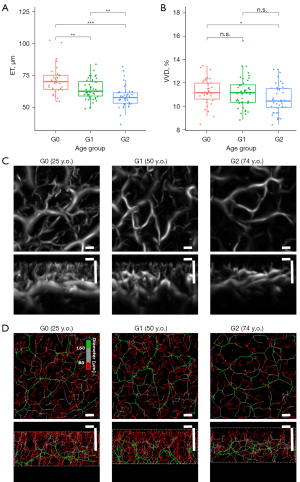
In Figure 4, the scatter plots of the ET and vessel volume densities, mVVD, and tVVD are presented. The residuals between the vessel metrics and age were calculated to exclude the influence of aging alterations. There was a positive correlation between mVVDresid and ETresid (r=0.50, P<0.001), whereas there was no significant correlation between tVVDresid and ETresid. All correlations between vessel metrics are summarized in Table 3. Significant correlations with ETresid were also observed for iVVDresid, DEJ-vessel distanceresid, and vessel branchresid, although not for mVAresid.
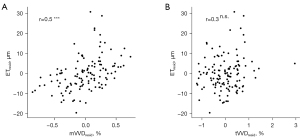
Table 3
Metric | ET (μm) | DEJ-vessel distance (μm) | VAF (%) | VVD (%) | mVVD (%) | iVVD (%) | tVVD (%) | Vessel branch (m−3) | mVA (°) |
---|---|---|---|---|---|---|---|---|---|
Age | −0.56** | 0.23* | −0.22* | −0.24** | −0.36*** | −0.34*** | 0.20* | −0.37*** | −0.21* |
ET | – | −0.54*** | 0.42*** | 0.48*** | 0.56*** | 0.47*** | −0.01(n.s.) | 0.58*** | 0.17(n.s.) |
ETresid | – | −0.53*** | 0.37*** | 0.43*** | 0.50*** | 0.36*** | 0.13(n.s.) | 0.49*** | 0.05(n.s.) |
†, Spearman’s rank correlation test: ***, P<0.001; **, P<0.01; *, P<0.05; n.s., P≥0.05. ET, epidermal thickness; DEJ, dermal-epidermal junction; VAF, vessel areal fraction; VVD, vessel volume density; mVVD, micro-vessel volume density; iVVD, intermediate-vessel volume density; tVVD, thick vessel volume density; mVA, vessel angle.
Figure 5 shows a plot of the correlation coefficient between ETresid and the diameter-selective vessel volume densities as a function of the diameter threshold. A significant correlation was observed between ETresid and VVDresid composed of vessels thinner than the diameter threshold of 80 µm, while there is no significant correlation between ETresid and VVDresid composed of vessels thicker than the diameter threshold of 160 µm.
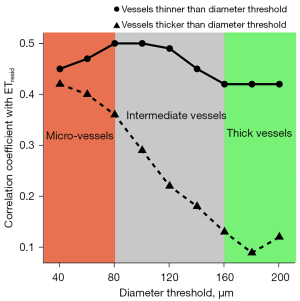
Discussion
In this study, we proposed an analysis method for OCTA that preserved the 3D information of blood vessels in the skin and investigated both aging-related alterations and their association with the epidermis. Although several OCTA studies have examined the contribution of vessels to aging alterations in the skin (16,17,36), these studies were primarily limited to two-dimensional analyses, that is, MIP vessel density analysis. This limitation is largely attributed to the tail artifacts in the OCTA signals. Our post-processing method mitigated this limitation and successfully visualized vesselness in 3D with high accuracy by applying a tail-removal imaging filter (11) to an OMAG image and passing it through a multiscale Hessian filter. Consequently, we could evaluate the vessel densities on the basis of vessel diameters, namely on the basis of vessels being micro, intermediate and thick.
Regarding photoaging alteration, this study shows that the VVD in the cheek area decreased with age. A similar aging trend was observed in the histological section images (37) and the MIP images of OCTA (16). As the VVD retains non-invasive 3D information, it represents a more accurate vessel structure of the organism. The aging trend of VVD was not monotonic and was found to decrease significantly in the G0–G2 group. However, there was no significant difference in the G0–G1 group, possibly due to a significant change in the secretion of female hormones caused by menopause (38). Classifying VVD on the basis of vessel diameter, mVVD decreased with age, but tVVD increased. In ovariectomized rats, mimicking human menopause, it has been reported that the capillary diameter decreases, suggesting a decrease in capillaries (38). Based on past knowledge, mVVD is suggested as being representative of the density of capillaries. Herein, it is known that there is no change in the level of skin redness with age (39,40). This unchanged skin redness may be associated with increased noncapillary, larger vessel types, such as post-capillary venules, with increased tVVD. Whether these alterations in mVVD and tVVD are specific to photoaging needs further investigation.
Dermal blood vessels visualized by OCTA may also play a crucial role in maintaining epidermal homeostasis. The correlation between vessel metrics and the epidermis was investigated to clarify the effect. Considering that both vessel metrics and ET are influenced by aging, we conducted a correlation analysis after accounting for the effect of age. A significant positive correlation with ETresid for mVVDresid, not for tVVDresid, was observed here, suggesting that the number of micro-vessels, mVVD, may play an important role in securing epidermal thickness rather than the number of thick vessel, tVVD. Capillaries beneath the epidermis maintain homeostasis in the epidermis by exchanging nutrients and waste products, regulated by Starling’s force (25,41). The results of this study suggested that blood vessels with diameters of 80 µm or less, as visualized by OCTA, contributed to epidermal homeostasis as capillaries, which in turn may be associated with ET.
To further investigate the association of micro- and thick vessels with epidermis, the correlation of ET for diameter-selective vessel volume densities was calculated by systematically changing the diameter threshold. The highest correlation was observed between ETresid and VVDresid composed of vessels thinner than the diameter threshold of 80 µm, suggesting that the diameter threshold of micro-vessels estimated by the visual observation was reasonable. A positive correlation was observed between ETresid and the vessel angle composed of vessels thinner than the diameter threshold of 40 µm (data not shown), implying that vessels whose diameters were approximately 40 µm can be dedicated to efficient nutrition and other aspects, for example, supporting mechanical stiffness (42), through their vertical alignment. It was also found that the correlation coefficient between ETresid and VVDresid for vessels thinner than the those having the diameter threshold of 40 µm was less than that of vessels of diameter 80 µm, suggesting that the number of the vessels having a diameter of less than 40 µm was relatively small even though the association with epidermis is high. In contrast, no significant correlation was found between ETresid and VVDresid for vessels having a diameter greater than the diameter threshold of 160 µm, which may be due to their dissociation from epidermal homeostasis.
Regarding the intermediate vessels, a similar trend against ETresid was found for iVVDresid (r=0.36, P<0.001). It is suggested that vessels with intermediate diameter may contribute to maintaining epidermal homeostasis by supporting capillaries. Our study is the first to focus on diameter-dependent vascular classification and examine its relationship with the epidermis, non-invasively. In addition to mVVD and iVVD, DEJ-vessel distance and vessel branch correlate with ET as shown in Table 2. Because capillaries are densely distributed beneath the epidermis, these metrics could also represent the condition of the capillaries as a separate aspect from mVVD.
In this study, we proposed 3D quantitative vessel parameters, represented by VVD, among the skin and vessel metrics obtained by OCTA, to investigate the aging alteration of dermal blood vessels and their contribution to the epidermis. The proposed diameter-dependent classification using OCTA is expected to be beneficial in assessing the degree of photoaging and epidermal conditions.
One limitation is that the skin types are imbalanced for Types III and IV, and it is not known whether the results obtained in this study are similar for other skin types. Another limitation is that the shape of a single vessel (e.g., dots, curved, or coiled) is not reflected in this study (43,44). The association of dermal vasculature with aging and the epidermis may become stronger by assessing the 3D geometry of a single vessel. Finally, statistical analysis revealed that the behavior of micro and intermediate vessels is similar. The interpretation of the role of intermediate vessels is still ambiguous. Further investigation regarding every vessel type is necessary to clarify the process of photoaging or intrinsic aging of the vessel in the skin.
Conclusions
In this study, we proposed a 3D quantification method for dermal blood vessels and various vessel metrics, such as VVD. Our results revealed that the mVVD decreased with age and was positively correlated with ET. These suggest that the micro-vessels observed using OCTA may play a vital role in maintaining the thickness of the epidermis. The proposed diameter-dependent vascular analysis using OCTA could be beneficial for understanding the roles of dermal blood vessels and assessing photoaging.
Acknowledgments
We would like to thank Drs. Ryo Yokota, Munetaka Kawamoto, and Tatsuya Yoshikawa for their technical support in the statistical analyses. We would also like to acknowledge the contribution of Dr. Nhan Le for his useful technical support in the OCTA instrumentation.
Funding: None.
Footnote
Reporting Checklist: The authors have completed the STROBE reporting checklist. Available at https://qims.amegroups.com/article/view/10.21037/qims-23-1790/rc
Conflicts of Interest: All authors have completed the ICMJE uniform disclosure form (available at https://qims.amegroups.com/article/view/10.21037/qims-23-1790/coif). R.K.W. serves as an unpaid Deputy Editor of Quantitative Imaging in Medicine and Surgery. R.K.W. received research support from Shiseido Co. Ltd. M.N. and T.Y. are former employees of Shiseido Co., Ltd. T.H., M.K., C.K., and Y.H. are current employees of Shiseido Co., Ltd. The other author has no conflicts of interest to declare.
Ethical Statement: The authors are accountable for all aspects of the work in ensuring that questions related to the accuracy or integrity of any part of the work are appropriately investigated and resolved. The study was conducted in accordance with the Declaration of Helsinki (as revised in 2013). The study was approved by the Ethics Committee of Shiseido Co., Ltd. (No. C02109) and informed consent was obtained from all individual participants.
Open Access Statement: This is an Open Access article distributed in accordance with the Creative Commons Attribution-NonCommercial-NoDerivs 4.0 International License (CC BY-NC-ND 4.0), which permits the non-commercial replication and distribution of the article with the strict proviso that no changes or edits are made and the original work is properly cited (including links to both the formal publication through the relevant DOI and the license). See: https://creativecommons.org/licenses/by-nc-nd/4.0/.
References
- Tobin DJ. Introduction to skin aging. J Tissue Viability 2017;26:37-46. [Crossref] [PubMed]
- Ganceviciene R, Liakou AI, Theodoridis A, Makrantonaki E, Zouboulis CC. Skin anti-aging strategies. Dermatoendocrinol 2012;4:308-19. [Crossref] [PubMed]
- Venkatesh S, Maymone MBC, Vashi NA. Aging in skin of color. Clin Dermatol 2019;37:351-7. [Crossref] [PubMed]
- Zouboulis CC, Makrantonaki E, Nikolakis G. When the skin is in the center of interest: An aging issue. Clin Dermatol 2019;37:296-305. [Crossref] [PubMed]
- Rokhsar CK, Lee S, Fitzpatrick RE. Review of photorejuvenation: devices, cosmeceuticals, or both? Dermatol Surg 2005;31:1166-78; discussion 1178. [Crossref] [PubMed]
- Minkis K, Swary JH, Alam M. Photoaging. Cosmet Dermatol 2015:13-22.
- Chung JH, Eun HC. Angiogenesis in skin aging and photoaging. J Dermatol 2007;34:593-600. [Crossref] [PubMed]
- Kajiya K, Kim YK, Kinemura Y, Kishimoto J, Chung JH. Structural alterations of the cutaneous vasculature in aged and in photoaged human skin in vivo. J Dermatol Sci 2011;61:206-8. [Crossref] [PubMed]
- Lugo LM, Lei P, Andreadis ST. Vascularization of the dermal support enhances wound re-epithelialization by in situ delivery of epidermal keratinocytes. Tissue Eng Part A 2011;17:665-75. [Crossref] [PubMed]
- Favazza CP, Jassim O, Cornelius LA, Wang LV. In vivo photoacoustic microscopy of human cutaneous microvasculature and a nevus. J Biomed Opt 2011;16:016015. [Crossref] [PubMed]
- Chen CL, Wang RK. Optical coherence tomography based angiography. Biomed Opt Express 2017;8:1056. [Crossref] [PubMed]
- Zabihian B, Chen Z, Rank E, Sinz C, Bonesi M, Sattmann H, Ensher J, Minneman MP, Hoover E, Weingast J, Ginner L, Leitgeb R, Kittler H, Zhang E, Beard P, Drexler W, Liu M. Comprehensive vascular imaging using optical coherence tomography-based angiography and photoacoustic tomography. J Biomed Opt 2016;21:96011. [Crossref] [PubMed]
- Hara Y, Yamashita T, Ninomiya M, Kubo Y, Katagiri C, Saeki S, Iizuka H. Vascular morphology in facial solar lentigo assessed by optical coherence tomography angiography. J Dermatol Sci 2021;102:193-5. [Crossref] [PubMed]
- Deegan AJ, Talebi-Liasi F, Song S, Li Y, Xu J, Men S, Shinohara MM, Flowers ME, Lee SJ, Wang RK. Optical coherence tomography angiography of normal skin and inflammatory dermatologic conditions. Lasers Surg Med 2018;50:183-93. [Crossref] [PubMed]
- Deegan AJ, Wang W, Men S, Li Y, Song S, Xu J, Wang RK. Optical coherence tomography angiography monitors human cutaneous wound healing over time. Quant Imaging Med Surg 2018;8:135-50. [Crossref] [PubMed]
- Hara Y, Yamashita T, Kikuchi K, Kubo Y, Katagiri C, Kajiya K, Saeki S. Visualization of age-related vascular alterations in facial skin using optical coherence tomography-based angiography. J Dermatol Sci 2018;90:96-8. [Crossref] [PubMed]
- Barrera-Patiño CP, de Oliveira LO, Mattos VS, Stringasci MD, Requena MB, Bagnato VS. Skin microvasculature determined using OCT angiography: first evidence of its relation with aging. Laser Phys Lett 2023;20:25602.
- Kashani AH, Chen CL, Gahm JK, Zheng F, Richter GM, Rosenfeld PJ, Shi Y, Wang RK. Optical coherence tomography angiography: A comprehensive review of current methods and clinical applications. Prog Retin Eye Res 2017;60:66-100. [Crossref] [PubMed]
- Li Y, Tang J. Blood vessel tail artifacts suppression in optical coherence tomography angiography. Neurophotonics 2022;9:021906. [Crossref] [PubMed]
- Untracht GR, Matos RS, Dikaios N, Bapir M, Durrani AK, Butsabong T, Campagnolo P, Sampson DD, Heiss C, Sampson DM. OCTAVA: An open-source toolbox for quantitative analysis of optical coherence tomography angiography images. PLoS One 2021;16:e0261052. [Crossref] [PubMed]
- Zhang J, Qiao Y, Sarabi MS, Khansari MM, Gahm JK, Kashani AH, Shi Y. 3D Shape Modeling and Analysis of Retinal Microvasculature in OCT-Angiography Images. IEEE Trans Med Imaging 2020;39:1335-46. [Crossref] [PubMed]
- Zhang Y, Li H, Cao T, Chen R, Qiu H, Gu Y, Li P. Automatic 3D adaptive vessel segmentation based on linear relationship between intensity and complex-decorrelation in optical coherence tomography angiography. Quant Imaging Med Surg 2021;11:895-906. [Crossref] [PubMed]
- Borrelli E, Parravano M, Costanzo E, Sacconi R, Querques L, Pennisi F, De Geronimo D, Bandello F, Querques G. Using three-dimensional optical coherence tomography angiography metrics improves repeatability on quantification of ischemia in eyes with diabetic macular edema. Retina 2021;41:1660-7. [Crossref] [PubMed]
- Augustin HG, Koh GY, Thurston G, Alitalo K. Control of vascular morphogenesis and homeostasis through the angiopoietin-Tie system. Nat Rev Mol Cell Biol 2009;10:165-77. [Crossref] [PubMed]
- Braverman IM. The cutaneous microcirculation. J Investig Dermatol Symp Proc 2000;5:3-9. [Crossref] [PubMed]
- Le N, Lu J, Tang P, Chung KH, Subhash H, Kilpatrick-Liverman L, Wang RK. Intraoral optical coherence tomography and angiography combined with autofluorescence for dental assessment. Biomed Opt Express 2022;13:3629-46. [Crossref] [PubMed]
- Wang RK. Optical Microangiography: A Label Free 3D Imaging Technology to Visualize and Quantify Blood Circulations within Tissue Beds in vivo. IEEE J Sel Top Quantum Electron 2010;16:545-54. [Crossref] [PubMed]
- Zhang A, Zhang Q, Wang RK. Minimizing projection artifacts for accurate presentation of choroidal neovascularization in OCT micro-angiography. Biomed Opt Express 2015;6:4130-43. [Crossref] [PubMed]
- Baran U, Choi WJ, Li Y, Wang RK. Tail artifact removal in OCT angiography images of rodent cortex. J Biophotonics 2017;10:1421-9. [Crossref] [PubMed]
- Frangi AF, Niessen WJ, Vincken KL, Viergever MA. Multiscale vessel enhancement filtering. In: Wells WM, Colchester A, Delp S. editors. Medical Image Computing and Computer-Assisted Intervention — MICCAI’98. Lecture Notes in Computer Science, Springer, Berlin, Heidelberg, 2006;1496:130-7.
- Longo A, Morscher S, Najafababdi JM, Jüstel D, Zakian C, Ntziachristos V. Assessment of hessian-based Frangi vesselness filter in optoacoustic imaging. Photoacoustics 2020;20:100200. [Crossref] [PubMed]
- Lee TC, Kashyap RL, Chu CN. Building Skeleton Models via 3-D Medial Surface Axis Thinning Algorithms. CVGIP: Graphical Models and Image Processing 1994;56:462-78.
- Maurer CR, Qi R, Raghavan V. A linear time algorithm for computing exact Euclidean distance transforms of binary images in arbitrary dimensions. IEEE Transactions on Pattern Analysis and Machine Intelligence 2003;25:265-70.
- Gambichler T, Matip R, Moussa G, Altmeyer P, Hoffmann K. In vivo data of epidermal thickness evaluated by optical coherence tomography: effects of age, gender, skin type, and anatomic site. J Dermatol Sci 2006;44:145-52. [Crossref] [PubMed]
- Neerken S, Lucassen GW, Bisschop MA, Lenderink E, Nuijs TA. Characterization of age-related effects in human skin: A comparative study that applies confocal laser scanning microscopy and optical coherence tomography. J Biomed Opt 2004;9:274-81. [Crossref] [PubMed]
- Olsen J, Gaetti G, Grandahl K, Jemec GBE. Optical coherence tomography quantifying photo aging: skin microvasculature depth, epidermal thickness and UV exposure. Arch Dermatol Res 2022;314:469-76. [Crossref] [PubMed]
- Chung JH, Yano K, Lee MK, Youn CS, Seo JY, Kim KH, Cho KH, Eun HC, Detmar M. Differential effects of photoaging vs intrinsic aging on the vascularization of human skin. Arch Dermatol 2002;138:1437-42. [Crossref] [PubMed]
- Tanaka M, Kanazashi M, Maezawa T, Kondo H, Fujino H. Abnormalities in three-dimensional capillary architecture and imbalance between vascular endothelial growth factor-A and thrombospondin-1 in soleus muscle of ovariectomized rat. Acta Histochem 2015;117:605-11. [Crossref] [PubMed]
- Warren R, Gartstein V, Kligman AM, Montagna W, Allendorf RA, Ridder GM. Age, sunlight, and facial skin: a histologic and quantitative study. J Am Acad Dermatol 1991;25:751-60. [Crossref] [PubMed]
- de Rigal J, Des Mazis I, Diridollou S, Querleux B, Yang G, Leroy F, Barbosa VH. The effect of age on skin color and color heterogeneity in four ethnic groups. Skin Res Technol 2010;16:168-78. [Crossref] [PubMed]
- Michel CC. Starling: the formulation of his hypothesis of microvascular fluid exchange and its significance after 100 years. Exp Physiol 1997;82:1-30. [Crossref] [PubMed]
- Sawane M, Ogura Y, Nakamura A, Otaka H, Kidoya H, Sato K, Takakura N, Kajiya K. Blood vessels sense dermal stiffness via a novel mechanotransducer, APJ. Angiogenesis 2022;25:151-4. [Crossref] [PubMed]
- Ulrich M, Themstrup L, de Carvalho N, Ciardo S, Holmes J, Whitehead R, Welzel J, Jemec GBE, Pellacani G. Dynamic optical coherence tomography of skin blood vessels - proposed terminology and practical guidelines. J Eur Acad Dermatol Venereol 2018;32:152-5. [Crossref] [PubMed]
- Welzel J, Schuh S, De Carvalho N, Themstrup L, Ulrich M, Jemec GBE, Holmes J, Pellacani G. Dynamic optical coherence tomography shows characteristic alterations of blood vessels in malignant melanoma. J Eur Acad Dermatol Venereol 2021;35:1087-93. [Crossref] [PubMed]