Cone beam computed tomography (CBCT) and megavoltage computed tomography (MVCT)-based radiomics in head and neck cancers: a systematic review and radiomics quality score assessment
Introduction
Radiomics is a quantitative image analysis that extracts imaging information to clinical and biological endpoints. The data are mineable from digital medical images using advanced algorithms (1-4) that explore multiple dimensions of the images such as shape and size, textural features, histogram-based and filter-based. The potential of radiomics as a non-invasive imaging (5-7) has been indicated in various studies to improve diagnostic and prognostic performance and subsequently clinical decision support in oncology (8,9).
However, there is a concern about the quality of radiomics research as it has developed for more than one decade in the field of oncology. With the rapid growth in radiomics, a standardized quality evaluation of radiomics studies become crucial (10). Therefore, the radiomics quality score (RQS) was introduced in 2017 to assess the characteristics and essentially the quality of radiomics study including the reporting of it. Radiomics studies also need to be carried out well to ensure a smooth transition to clinical utility (11).
Both cone beam computed tomography (CBCT) and megavoltage computed tomography (MVCT) were routinely used in image-guided radiotherapy (IGRT) processes. These modalities allow online verification of the 3-dimensional patient’s setup before radiotherapy delivery. Images procured during CBCT and MVCT acquisition are useful in head and neck radiomics studies. In particular, CBCT-based radiomics studies demonstrated the ability to support adaptive treatment plans and prediction of oncological outcomes (12-14). Meanwhile, MVCT-based radiomics in head and neck cancer notably existed in recent years. Mirestean et al. (15) in a review of delta radiomics studies highlighted the variation of radiomics features extracted from MVCT images that are useful as a predictor for late xerostomia and distinguished predictive power compared to pre-treatment dosimetric models. In a phantom study, MVCT images provided reproducible radiomics features and could be used for clinical prediction (16). This has opened up more CBCT and MVCT-based radiomics studies in head and neck cancers.
This study aims to systematically review the current research applying radiomics in patients with head and neck cancers and to assess the methodological and reporting quality of CBCT-based and MVCT-based radiomics related to treatment response, recurrence or local failure, survivals, and radiation-induced toxicities. A RQS assessment and analyses on correlation with CBCT-based and MVCT-based radiomics are presented in this review. We present this article in accordance with the Preferred Reporting Items for Systematic Reviews and Meta-Analyses (PRISMA) reporting checklist (available at https://qims.amegroups.com/article/view/10.21037/qims-24-334/rc).
Methods
The review protocol was registered on the PROSPERO (registration number CRD42024497459).
Articles search strategy
A systematic search was performed to identify available studies on PubMed, Web of Science, and Scopus databases. The last search was performed on 29 September 2023. The following search strings were used to identify related studies, (head and neck cancer*) OR (scc) AND (radiotherapy) OR (cone beam ct) OR (cbct) AND (radiomic*) AND (toxicity) OR (prognostic*) OR (survival) OR (recurrence) which can be refered in Table S1. No restrictions related to language and date or publication years were applied during the search process. The inclusion studies were based on Patient, Intervention, Comparator, Outcome, and Study design (PICOS) criteria: (I) Patient—head and neck cancer patients, (II) Intervention—radiotherapy, (III) Comparison—radiomics analysis using CBCT and MVCT, (IV) Outcome—toxicity, recurrence, survival, and response (V) Study design—a retrospective or prospective study. Only radiomics studies analysed using CBCT and MVCT imaging were eligible in this review.
Inclusion and exclusion criteria
The inclusion and exclusion criteria have been outlined before initiating the process of this systematic review. Firstly, the inclusion criteria were restricted to studies related to oncology/radiotherapy fields. Secondly, studies evaluating radiomics or textural analysis using CBCT and MVCT imaging for head and neck cancer patients treated with radiotherapy. In terms of opposite criteria, the studies were excluded if: (I) duplicated studies within searches, (II) non-human studies, (III) systematic review papers, and (IV) conference abstract papers or abstracts not related to the main research.
Data review and extraction
Initially, the data was extracted using an Excel spreadsheet in the elimination process of duplicate studies. After the elimination of duplicates, the studies were screened by reviewing their titles and abstracts. The titles and abstracts screening was initially performed by two independent reviewers (N.Y. and M.I.). Finally, all the studies that passed the titles and abstracts screening were read the full text. From all the selected studies, the following data were collected: (I) author and publication year, (II) type of study, (III) number of patients (IV) type of imaging, and (V) study endpoints. The studies were grouped according to the main purpose of investigation: (I) diagnostic (including toxicity) and (II) prognostic (including prediction of local failure, overall survival, volume changes, and treatment response).
RQS
RQS assessment was carried out using radiomics quality evaluation according to Lambin et al. (10). The RQS comprised 16 key components and was divided into checkpoint 1 (item 1), checkpoint 2 (items 2 to 4), and checkpoint 3 (items 5 to 16). These 16 key components were included in 6 key domains of the RQS which Domain 1 (items 1, 2, 3, and 4), Domain 2 (items 5, and 12), Domain 3 (items 6, 7, 13, and 14), Domain 4 (items 8, 9 and 10), Domain 5 (items 11 and 15), Domain 6 (item 16). To enhance the understanding and knowledge of the reviewers, a discussion and training session focusing on items, criteria, and scores were conducted earlier before the included studies were assessed. This radiomics quality assessment tool was used in line with the initiated training session to evaluate the methodological and reporting quality of studies applying CBCT and MVCT-based radiomics. However, the most recent radiomics quality assessment tools (17) would certainly be very useful for other review work in the future.
Two reviewers (N.Y. and M.I.) independently reviewed the full text and supplementary materials of selected studies and evaluated them using RQS. The assessment was performed accordingly with no modification to the original RQS checklist. The sum of the RQS total score was reported and also presented in percentage. The range of total scores is from −8 to 36 and percentage scores are defined as 0% to 100%. The RQS scoring and detailed report are summarized in Table 1. Discrepancies between reviewers were resolved by consensus.
Table 1
Author & year | Image protocol quality | Multiple segmentations | Phantom study on all scanners | Imaging at multiple time points | Feature reduction or adjustment for multiple testing | Multivariable analysis with non-radiomics features | Detect and discuss biological correlates | Cut-off analyses | Discrimination statistics | Calibration statistics | Prospective study registered in a trial database | Validation | Comparison to ‘gold standard’ | Potential clinical utility | Cost-effectiveness analysis | Open science and data | RQS (total) | RQS (%) |
---|---|---|---|---|---|---|---|---|---|---|---|---|---|---|---|---|---|---|
Iliadou V, 2022 (18) | 1 | 1 | 0 | 1 | 3 | 0 | 0 | 0 | 0 | 0 | 0 | 0 | 0 | 0 | 0 | 0 | 6 | 16.67 |
Morgan HE, 2021 (19) | 1 | 1 | 0 | 1 | −3 | 0 | 1 | 0 | 2 | 1 | 0 | 2 | 0 | 0 | 0 | 4 | 10 | 27.8 |
Abe K, 2023 (20) | 1 | 0 | 0 | 1 | 3 | 1 | 0 | 1 | 1 | 2 | 0 | 2 | 0 | 0 | 0 | 3 | 15 | 41.67 |
Sellami S, 2022 (21) | 1 | 0 | 0 | 1 | 3 | 1 | 1 | 1 | 2 | 1 | 0 | 2 | 0 | 0 | 0 | 1 | 14 | 38.89 |
Berger T, 2022 (22) | 0 | 1 | 0 | 1 | 3 | 0 | 0 | 0 | 2 | 2 | 0 | 2 | 0 | 0 | 1 | 2 | 14 | 38.89 |
Berger T, 2023 (23) | 1 | 1 | 0 | 1 | 3 | 1 | 1 | 0 | 2 | 2 | 0 | 2 | 0 | 0 | 0 | 2 | 16 | 44.44 |
RQS, radiomics quality score.
Statistical analysis
The RQS according to 16 key components of each included study was extracted. Subgroup analyses were proportioned according to published journal type, publication year, and number of patients. The overall RQS according to six key domains of all included studies was plotted and summed as shown in Table S2. The Mann-Whitney U test was conducted as most of the sample data was small. This non-parametric test was performed to compare all the variables according to subgroups. Data were demonstrated in the median and interquartile range (IQR) of each subgroup. The P values <0.05 were considered statistically significant. The analyses were conducted using IBM SPSS Statistics (version 25).
Results
Literature search
From the initial systematic search, a total of 743 studies were identified from PubMed (n=70), Web of Science (n=63) and Scopus (n=610) databases. A total of 623 irrelevant studies and 94 duplicated studies were removed, and 26 studies were eligible for full-text screening. From the 26 that were eligible, 16 review studies, three phantom studies, and one study with a small number of patients (24) were also excluded to avoid bias during statistical analysis. Finally, after the full-text screening (based on PICOS criteria) six studies met the inclusion and were analyzed in the review. The study selection process according to PRISMA Statement 2020 (25) is reported in Figure 1.
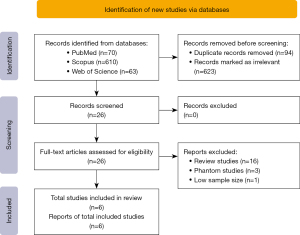
Characteristics of radiomics studies
The relevant studies are all written in English and were published in the earliest record in June 2021. The study’s characteristics are summarized in Table 2. Most of the studies were published in 2022 (50%), in 2023 (33.3%) and one study was published in 2021 (16.7%). Of all included studies, the median patient number was 97 patients (range, 40–112). Overall, studies on head and neck cancers are oropharyngeal cancer (n=213), hypopharyngeal (n=78), laryngeal (n=36), oral cavity (n=35), mesopharyngeal (n=32), base of tongue (n=26), tonsil (n=21), supraglottic (n=20), unspecified (n=18), unknown (n=12), maxilla (n=8), glottic (n=6), soft palate (n=2) and one study (18) had specified all the patients as head and neck squamous cell cancer (HN SCC; n=40).
Table 2
First author & year | Study design | Diagnosis (%) | No of patients | Intervention & dose | Endpoint |
---|---|---|---|---|---|
Iliadou V, 2022 (18) | Retrospective | HN SCC (100%) | 40 | VMAT (66 Gy/30#/6 weeks) | Tumor-volume alterations |
Morgan HE, 2021 (19) | Retrospective | Base of tongue (29.0%) | 90 | IMRT (70 Gy) | LF at completion of RT in primary and nodal structures |
Tonsil (23.3%) | |||||
Supraglottic (22.2%) | |||||
Hypopharynx (9.0%) | |||||
Oropharynx (7.7%) | |||||
Glottic (6.6%) | |||||
Soft palate (3.3%) | |||||
Abe K, 2023 (20) | Retrospective | Hypopharynx (56.0%) | 100 | Tomotherapy (70 Gy/35#/7 weeks) | Overall survival |
Mesopharynx (32.0%) | |||||
Larynx (11.0%) | |||||
Oral cavity (1.0%) | |||||
Sellami S, 2022 (21) | Retrospective | Oropharynx (51.7%) | 93 | VMAT (56 Gy/28#/5.5 weeks); (70 Gy/35#/7 weeks) | Treatment response (at the fourth week of treatment) |
Oral cavity (17.2%) | |||||
Larynx (16.1%) | |||||
Hypopharynx (15.0%) | |||||
Berger T, 2022 (22) | Retrospective | Oropharynx (70.5%) | 112 | Tomotherapy (60 Gy/30#/6 weeks); (65 Gy/30#/6 weeks); (70 Gy/35#/7 weeks) | Moderate-to-severe late xerostomia (Grade ≥2 at 6, 12 and 24 months post RT) |
Oral cavity (8.0%) | |||||
Unspecified (8.0%) | |||||
Unknown primary (5.4%) | |||||
Larynx (5.0%) | |||||
Maxilla (3.6%) | |||||
Berger T, 2023 (23) | Retrospective | Oropharynx (70.5%) | 112 | Tomotherapy (60 Gy/30#/6 weeks); (65 Gy/30#/6 weeks); (70 Gy/35#/7 weeks) | Late xerostomia (Grade ≥2 at 6 and 12 months post RT) |
Oral cavity (8.0%) | |||||
Unknown primary (5.4%) | |||||
Larynx (4.5%) | |||||
Maxilla (3.6%) | |||||
Nasopharynx (2.7%) | |||||
Hypopharynx (2.7%) | |||||
Unspecified (2.6%) |
HN, head and neck; SCC, squamous cell carcinoma; VMAT, volumetric modulated arc therapy; Gy, Gray; IMRT, intensity modulated radiotherapy; LF, local failure; RT, radiation therapy.
The studies endpoints included the tumour-volume alterations (1/6, 16.7%) (18), the prediction of local failure after radiotherapy (1/6, 16.7%) (19), overall survival (1/6, 16.7%) (20), treatment response (1/6, 16.7%) (21), and two studies worked out on the prediction of radiotherapy toxicities (2/6, 33.3%) (22,23).
From all six studies, equal numbers of the studies were CBCT-based (18,19,21) (3/6, 50%) and MVCT-based (20,22,23) (3/6 50%) radiomics studies. Moreover, equal numbers of them were published in imaging journals (3/6, 50%) and general journals (3/6, 50%). All the included papers were retrospective radiomics studies (6/6, 100%) and were performed at a single institution.
In regards to radiomics features, most of the studies utilized morphological features (100%), first-order features (100%), second-order features (100%), and dosimetric (83%) as single study were not reported on dosimetric information. Region of interest (ROI) segmentation according to the study aims was performed semi-automatically in all studies. Four studies reported the ROI segmentation conducted as semi-automated and manually corrected in specific. The details of radiomics feature information are shown in Table 3.
Table 3
Author & year | Imaging | ROI segmentation | VOI | Software | No. of features | Radiomics features | Feature selection | Model development | Morphological features | First-order | Second-order | Dosimetric | Pre-processing |
---|---|---|---|---|---|---|---|---|---|---|---|---|---|
Iliadou V, 2022 (18) | CBCT | Semi-automated | CTV and PGs | 3D Slicer & PyRadiomics | 104 features | Shape | -Binary classification to isolate early anatomical variations and improve classification performance | Pearson correlation (P<0.05) to assess associations of CTV and PGs alteration post week 2 | Yes | Yes | Yes | Yes | Dataset divided into Class 1 (>20% volume changes) and Class 2 (<20% volume changes) |
First-order GLDM | -LOOCV to assess overfitting and bias | ||||||||||||
GLCM | |||||||||||||
GLRLM GLSZM NGTDM | |||||||||||||
Morgan HE, 2021 (19) | CT and CBCT | Semi-automated & manually corrected | Primary tumour and nodal structures | PyRadiomics | 102 features | Shape | -ICC coefficients with threshold ≥0.95 for features comparison | Sample t-test (P<0.05) with ROC analysis | Yes | Yes | Yes | Yes | Clinical and radiomics features separated to EBM classifier and EBM exploratory sets |
First order | - EBM classifier with 5-fold cross-validation of the exploratory sets | ||||||||||||
GLCM | - 25 times reiteration to reduce impact of randomness on biasing | ||||||||||||
GLRLM | |||||||||||||
GLSZM | |||||||||||||
GLDM | |||||||||||||
Abe K, 2023 (20) | CT & MVCT | Semi-automated | GTV | PyRadiomics | 104 features | GLCM | -Spearman correlation coefficients >0.85 to confirm interchangeable features between MVCT and kVCT | -Kaplan-Meier analysis | Yes | Yes | Yes | NR | -GTV propagation from kVCT to MVCT |
GLRLM | -LASSO Cox regression modeling and survival analysis | -Resampled into equal voxel sizes of 1×1×1 mm3 | |||||||||||
GLSZM | |||||||||||||
NGTDM | |||||||||||||
GLDM | |||||||||||||
Sellami S, 2022 (21) | CBCT | Semi-automated & manually corrected | GTV | MIRAS (in-house software) | 88 features | Busyness_NGTDM | -Youden index to determine cutoff values | -Multivariate logistic | Yes | Yes | Yes | Yes | Single time point feature selection and longitudinal feature selection with AUC >0.65 at every week |
Coarseness_NGTDM | -Internal five-fold cross validation to evaluate predictive efficiency | ||||||||||||
GLNU_area | |||||||||||||
Least_axis_length | |||||||||||||
Major_axis_length | |||||||||||||
Max_3D_diam | |||||||||||||
Volume | |||||||||||||
Berger T, 2022 (22) | MVCT | Semi-automated & manually corrected | PGs | MATLAB | 123 features | GLCM | -Pearson correlation coefficient exceeding 0.8 and the lower uni-variable association (Wilcoxon test) | -Sequential backward/forward selection | Yes | Yes | Yes | Yes | Normalized the MVCTs by shifting the HU of PGs |
GLRLM | -Excluded predictors (P>0.051) with univariate Wilcoxon test | -Logistic regression models | |||||||||||
-Bootstrapping | |||||||||||||
Berger T, 2023 (23) | MVCT | Semi-automated & manually corrected | PGs | MATLAB | 123 features | GLCM | -Univariate (Wilcoxon) | -Sequential backward/forward selection | Yes | Yes | Yes | Yes | Contralateral PGs divided into nine subregions and whole PG |
GLSZM | -3 folds cross validation | ||||||||||||
GLDZM | |||||||||||||
GLRLM | |||||||||||||
NGTDM | |||||||||||||
NGLDM |
ROI, regions of interest; VOI, volume of interest; CBCT, cone-beam computed tomography; CTV, clinical target volume; PG, parotid gland; 3D, three-dimensional; Shape, shape statistics features; First order, first order statistics features; GLDM, gray-level dependence matrices; GLCM, gray-level co-occurrence matrices; GLRLM, gray-level run length matrices; GLSZM, gray-level size zone matrices; NGTDM, neighborhood gray-tone difference matrices; LOOVC, leave-one-out cross validation; CT, computed tomography; ICC, intraclass correlation coefficient; EBM, explainable boosting machine; ROC, receiver operating characteristic; MVCT, megavoltage computed tomography; GTV, gross tumour volume; kVCT, kilovoltage computed tomography; LASSO, least absolute shrinkage and selection operator; GLNU, gray-level non-uniformity; AUC, area under the curve; HU, Hounsfield units; NGLDM, neighborhood grey-level dependence matrix.
Basic adherence rate of the reporting quality following six key domains
The basic adherence rate of all included studies according to six key domains is summarized in Table 4. In Domain 1, most of the studies reported well-documented protocols (83.3%). Four studies carried out multiple segmentation (66.7%). None of the studies performed phantom assessment. All authors reported multiple time point imaging (100%) in their studies. The majority of studies conducted on feature selection and validation have produced an impressive score of 83.3% in Domain 2. The adherence rate in Domain 3 showed half of the studies (50%) implemented multivariable analysis with non-radiomics features and biologic correlates that represent item 6 and item 7 whereas no studies had any score for item 13 and item 14 within the same domain. Scoring in Domain 4 in regards to the model performance index, the discrimination statistics (83.3%) and calibration statistics (83.3%) were available in five studies. While two articles (33.3%) reported cut-off analysis in their studies. Of all includes papers, none of them were prospectively registered in a trial database. Only one study discussed cost-effective analysis given the score of 16.7%. Regarding Domain 6 which focused on open source and data, almost all of the included radiomics studies (83.3%) had made their code and data publicly available and accessible for review.
Table 4
Six key domain of RQS | Adherence rate |
---|---|
Domain 1: protocol quality and stability in image segmentation | 83.3% |
Protocol quality | 5 (83.3%) |
Multiple segmentation | 4 (66.7%) |
Phantom study | 0 (0%) |
Multiple time point imaging | 6 (100%) |
Domain 2: feature selection and validation | 83.3% |
Feature reduction or adjustment | 5 (83.3%) |
Validation | 5 (83.3%) |
Domain 3: biologic/clinical validation and utility | 100% |
Multivariable analysis with non-radiomics features | 3 (50%) |
Biologic correlates | 3 (50%) |
Comparison to ‘gold standard’ | 0 (0%) |
Potential clinical utility | 0 (0%) |
Domain 4: model performance index | 83.3% |
Discrimination statistics | 5 (83.3%) |
Calibration statistics | 5 (83.3%) |
Cut-off analysis | 2 (33.3%) |
Domain 5: high level of evidence | 0% |
Prospective study | 0 (0%) |
Cost-effective analysis | 1 (16.7%) |
Domain 6: open science and data | 83.3% |
RQS, radiomics quality score.
Subgroup analysis
The total RQS of included studies according to six key domains is provided. The details scoring of the first reviewer and second reviewer are also presented in Tables S3,S4 respectively. An intraclass correlation coefficient (ICC) with 95% confidence interval (CI) based on absolute agreement with the two-way mixed model was used for the two-reviewer agreement on total RQS according to six key domains. The ICC was excellent with 0.99 (95% CI of 0.946< ICC <0.999) using the categories of poor (ICC <0.50), moderate (ICC =0.50–0.75), good (ICC =0.75–0.90), and excellent (ICC >0.90) (26). The scoring trend of RQS in MVCT-based studies showed higher scores than CBCT-based (41.7% vs. 27.8%) respectively. The result of subgroup analyses according to publication year, number of patients relative to the median (median =97), and published journal type are provided in Table 5 respectively. There were no statistical differences according to published journal type, publication year, and number of patients. No significant differences in the analyses between each RQS domain and subgroup components. The trend showed a higher RQS domain score for publication year ≤2022 than 2023 and number of patients > median than ≤ median but not statistically significant.
Table 5
Subgroup | Domain | Subgroup category | Median and IQR | Statistics | P value |
---|---|---|---|---|---|
Publication year | Domain 1 | ≤2022 | Median | 2.500 | >0.99 |
Interquartile range | 1.000 | ||||
2023 | Median | 2.500 | |||
Interquartile range | 1.000 | ||||
Domain 2 | ≤2022 | Median | 4.000 | 0.53 | |
Interquartile range | 5.000 | ||||
2023 | Median | 5.000 | |||
Interquartile range | 0.000 | ||||
Domain 3 | ≤2022 | Median | 0.500 | 0.53 | |
Interquartile range | 1.750 | ||||
2023 | Median | 1.500 | |||
Interquartile range | 0.000 | ||||
Domain 4 | ≤2022 | Median | 3.500 | 0.53 | |
Interquartile range | 3.250 | ||||
2023 | Median | 4.000 | |||
Interquartile range | 0.000 | ||||
Domain 5 | ≤2022 | Median | 0.000 | 0.80 | |
Interquartile range | 0.750 | ||||
2023 | Median | 0.000 | |||
Interquartile range | 0.000 | ||||
Domain 6 | ≤2022 | Median | 1.500 | 0.53 | |
Interquartile range | 3.250 | ||||
2023 | Median | 2.500 | |||
Interquartile range | 0.000 | ||||
Number of patients | Domain 1 | ≤ Median | Median | 3.000 | 0.70 |
Interquartile range | 0.000 | ||||
> Median | Median | 2.000 | |||
Interquartile range | 0.000 | ||||
Domain 2 | ≤ Median | Median | 3.000 | 0.20 | |
Interquartile range | 0.000 | ||||
> Median | Median | 5.000 | |||
Interquartile range | 0.000 | ||||
Domain 3 | ≤ Median | Median | 1.000 | >0.99 | |
Interquartile range | 0.000 | ||||
> Median | Median | 1.000 | |||
Interquartile range | 0.000 | ||||
Domain 4 | ≤ Median | Median | 3.000 | 0.20 | |
Interquartile range | 0.000 | ||||
> Median | Median | 4.000 | |||
Interquartile range | 0.000 | ||||
Domain 5 | ≤ Median | Median | 0.000 | 0.70 | |
Interquartile range | 0.000 | ||||
> Median | Median | 0.000 | |||
Interquartile range | 0.000 | ||||
Domain 6 | ≤ Median | Median | 1.000 | 0.70 | |
Interquartile range | 0.000 | ||||
> Median | Median | 2.000 | |||
Interquartile range | 0.000 | ||||
Published journal type |
Domain 1 | Imaging | Median | 0.000 | 0.70 |
Interquartile range | 2.000 | ||||
General | Median | 0.000 | |||
Interquartile range | 3.000 | ||||
Domain 2 | Imaging | Median | 5.000 | >0.99 | |
Interquartile range | 0.000 | ||||
General | Median | 5.000 | |||
Interquartile range | 0.000 | ||||
Domain 3 | Imaging | Median | 1.000 | 0.40 | |
Interquartile range | 0.000 | ||||
General | Median | 2.000 | |||
Interquartile range | 0.000 | ||||
Domain 4 | Imaging | Median | 4.000 | >0.99 | |
Interquartile range | 0.000 | ||||
General | Median | 4.000 | |||
Interquartile range | 0.000 | ||||
Domain 5 | Imaging | Median | 0.000 | 0.70 | |
Interquartile range | 0.000 | ||||
General | Median | 0.000 | |||
Interquartile range | 0.000 | ||||
Domain 6 | Imaging | Median | 3.000 | 0.10 | |
Interquartile range | 0.000 | ||||
General | Median | 1.000 | |||
Interquartile range | 0.000 |
RQS, radiomics quality score; IQR, interquartile range.
Discussion
In recent years, imaging modalities including ultrasonography (US), magnetic resonance imaging (MRI), computed tomography (CT), positron emission tomography (PET), and CBCT (27-32) have been utilized in radiomics research. Extraction of data from medical images is useful for diagnostic, predictive, or prognostic modeling purposes (15). However, no study of MVCT-based radiomics was conducted in that period until this work provided related studies from the search results.
In the present systematic review, three published studies evaluating CBCT-based radiomics and 3 studies evaluating MVCT-based radiomics utilization in head and neck cancers were reviewed. All the studies were evaluated regarding the quality of reporting using RQS. According to the presented results, CBCT-based radiomics and MVCT-based radiomics showed a basic adherence rate of 27.8% and 41.7% respectively. The difference in scoring may be due to the included CBCT-based studies that were mostly conducted earlier compared to MVCT-based. Hence, the basic adherence rate has increased for MVCT-based radiomics studies in more recent years. This is also comparable with other papers that conducted RQS assessments focusing on head and neck cancers. The total score of 21.33% for MRI-based radiomics (33) and 31.27% for multiple imaging-based radiomics (34) studies (US, CT, MRI).
Furthermore, the six key domain scores used in this study showed the absence of a high level of evidence in terms of a prospective study using CBCT-based or MVCT-based textural analysis in head and neck cancers. The lack of evidence in the prospective study also reported on a study focusing on MRI-based radiomics (33). On top of that, Aringhieri et al. revealed no studies earned >0 scores for this RQS item 11 (34). In addition, the application of phantom study in analyzing feature robustness of variability was still unexplored in all studies. As all six studies in this review employed retrospective design, the application of phantom study was implied as a challenge in radiomics studies due to the need to carry out detect inter-scanner differences as well as analyse the robustness of the source of variability. This context has been emphasized in previous studies (33,35,36).
Moreover, other findings from this study, particularly RQS items in Domain 2—comparison with the ‘gold standard’ and potential clinical utility, demonstrated that no score was obtained from all included studies. No decisive conclusion can be made considering the limited number of studies in this review. However, to elevate these as an added value of radiomics and as a potential application of the model in the clinical setting, it is important to further analyses on CBCT-based and MVCT-based radiomics studies in future requirements. This aspect has also been specifically highlighted in a study (37).
Mainly, delta radiomics studies indicated great advantages of CBCT-based and MVCT-based in multiple imaging points aspect. The benefits of CBCT imaging have been addressed in a CBCT-based delta-radiomics study in detecting tumour-volume deviation during radiotherapy (20). The proposed study successfully achieved high classification performance from the first week of treatment. These significant changes of volume provided in CBCT image features had built a delta radiomic model that subsequently might be used for planning adaptation. In a delta radiomics study predicting local failure for primary and nodal structures, Morgan et al. (19) with the utilization of CBCT images incorporated with CT planning images features have resulted in the highest discrimination that enabled the prediction of local failure at the completion of RT in head and neck cancer.
The employment of CBCT imaging as routine for pre-treatment verification became an interest for this prognostication radiomics study with no additional imaging required. Similar to (19,21) another CBCT-based radiomics study was conducted to investigate the potential of using radiomics features extracted from CBCT images (18). The features derived from CBCT imaging were usable given its potential and robustness compared to single time point imaging features. This study determined the prognostic information on treatment response in HNSCC derived from CBCT images. The analyzed result in the delta radiomics model showed higher balance accuracy in the utilization of CBCT imaging modality in this radiomics study.
Although only three studies of radiomics with the use of MVCT images, the potential of the predictive model was demonstrated clearly in the analyses. Berger et al. (22) performed day-to-day kinetics of radiomics features and showed improved predictive performance at 6 months. Uniquely, Berger et al. (23) used MVCT images to investigate the predictive power of radiomics features by dividing the parotid glands into nine sub-regions. This has been adopted from Zhang et al. (38) who defined boundary splits of the parotid glands in their study, superficial parotid sparing to reduce the incidence of high-grade xerostomia (39) and the sparing of parotid ducts to useful in the reduction of patient-rated xerostomia (40).
Another MVCT-based radiomics study (22) utilized MVCT images and demonstrated stronger prognostic power in predicting overall survival in HNSCC than any single clinical factor. MVCT images were also had higher prediction accuracy and comparable to CBCT in the radiomics studies. In assumption regarding the potential of MVCT in radiomics, our review and RQS assessment have also supported these MVCT-based radiomics studies showed quality as higher scores obtained from these three studies (38.89%, 44.44%, and 41.67%) compared to CBCT-based studies.
In both CBCT-based and MVCT-based some weaknesses need to be taken into account in supporting the practicality of these modalities in radiomics research. The imaging parameters are important in CBCT-based to avoid them affecting the feature extraction. The unstandardized acquisition of images is vulnerable to outcomes and radiomics model performance (7,20,41,42). As mentioned by van Timmeren et al. (43) variance in imaging parameters, image resolution, slice thickness, and reconstruction will increase the tendency to produce poor image quality.
Similarly, the variation of imaging protocol will lead to batch effects in post-image acquisition. The batch effects which included acquisition technique and reconstruction settings affected the radiomics features and further evaluation (44). Hu et al. (45) have marked batch effects as challenges when radiomics-derived imaging study was involved. Concerning the feasibility of CBCT-based radiomics, a standardized imaging protocol will be useful in confounder and noise reduction (42) and the calibration process of image information ought to be concluded to minimize the risk of unreproducible features analysis (18).
The disadvantages of MVCT images are always outlined as higher noise and lower contrast due to the less efficient detection of megavoltage X-rays. Resolution and image quality were prominently weak points in the head and neck radiomics studies that may affected the extracted features (27). Longer time for the imaging process also influenced image quality (i.e. motion artifact) and variations of acquisition pitch and reconstruction interval may result in differences in image quality. Gu et al. (16) emphasized these issues and strongly suggested the scanning protocol should be kept consistent if MVCT-based radiomics are utilized.
Undoubtedly, this systematic review has limitations mainly concerning the small number of included papers in this study that must be acknowledged for room of improvement. It is unpreventable as radiomics analysis using CBCT and MVCT modalities is still very deficient at present which may increase in bias. Moreover, because of fulfilling inclusion criteria, the study was limited to head and neck cancer resulting in small numbers of previous research. However, this inclusion criteria is important as studies involving head and neck cancers pose different challenges than in other regions. This issue should be enhanced to consider CBCT and MVCT indicated as imaging biomarkers in future radiomics studies. Overall, radiomics studies still need to develop with higher basic adherence in conducting and reporting. However, improved research and reporting quality are required to ensure sufficient reliability and reproducibility before implementation into clinical practice.
Conclusions
The study highlights the potential of CBCT and MVCT for predictive models in head and neck cancer radiomics studies. Additionally, it emphasizes the importance of adhering to guidelines for quality improvement of radiomics studies. Future studies should consider standardized methods including adherence to the image biomarker standardisation initiative (IBSI) guidelines to elevate the quality of the radiomics model.
Acknowledgments
Funding: This work was financially supported by
Footnote
Reporting Checklist: The authors have completed the PRISMA reporting checklist. Available at https://qims.amegroups.com/article/view/10.21037/qims-24-334/rc
Conflicts of Interest: All authors have completed the ICMJE uniform disclosure form (available at https://qims.amegroups.com/article/view/10.21037/qims-24-334/coif). N.Y. reports that this work was financially supported by the Ministry of Higher Learning (Malaysia), under the Fundamental Research Grant (No. FRGS/1/2021/SS03/UKM/02/1); and the National University of Malaysia grant (No. GP-2021-K017963). The other authors have no conflicts of interest to declare.
Ethical Statement: The authors are accountable for all aspects of the work in ensuring that questions related to the accuracy or integrity of any part of the work are appropriately investigated and resolved.
Open Access Statement: This is an Open Access article distributed in accordance with the Creative Commons Attribution-NonCommercial-NoDerivs 4.0 International License (CC BY-NC-ND 4.0), which permits the non-commercial replication and distribution of the article with the strict proviso that no changes or edits are made and the original work is properly cited (including links to both the formal publication through the relevant DOI and the license). See: https://creativecommons.org/licenses/by-nc-nd/4.0/.
References
- Gillies RJ, Kinahan PE, Hricak H. Radiomics: Images Are More than Pictures, They Are Data. Radiology 2016;278:563-77. [Crossref] [PubMed]
- Scapicchio C, Gabelloni M, Barucci A, Cioni D, Saba L, Neri E. A deep look into radiomics. Radiol Med 2021;126:1296-311. [Crossref] [PubMed]
- Lambin P, Rios-Velazquez E, Leijenaar R, Carvalho S, van Stiphout RG, Granton P, Zegers CM, Gillies R, Boellard R, Dekker A, Aerts HJ. Radiomics: extracting more information from medical images using advanced feature analysis. Eur J Cancer 2012;48:441-6. [Crossref] [PubMed]
- Kumar V, Gu Y, Basu S, Berglund A, Eschrich SA, Schabath MB, Forster K, Aerts HJ, Dekker A, Fenstermacher D, Goldgof DB, Hall LO, Lambin P, Balagurunathan Y, Gatenby RA, Gillies RJ. Radiomics: the process and the challenges. Magn Reson Imaging 2012;30:1234-48. [Crossref] [PubMed]
- Haider SP, Burtness B, Yarbrough WG, Payabvash S. Applications of radiomics in precision diagnosis, prognostication and treatment planning of head and neck squamous cell carcinomas. Cancers Head Neck 2020;5:6. [Crossref] [PubMed]
- Aerts HJ, Grossmann P, Tan Y, Oxnard GR, Rizvi N, Schwartz LH, Zhao B. Defining a Radiomic Response Phenotype: A Pilot Study using targeted therapy in NSCLC. Sci Rep 2016;6:33860. [Crossref] [PubMed]
- Tan D, Mohamad Salleh SA, Manan HA, Yahya N. Delta-radiomics-based models for toxicity prediction in radiotherapy: A systematic review and meta-analysis. J Med Imaging Radiat Oncol 2023;67:564-79. [Crossref] [PubMed]
- Rizzo S, Botta F, Raimondi S, Origgi D, Fanciullo C, Morganti AG, Bellomi M. Radiomics: the facts and the challenges of image analysis. Eur Radiol Exp 2018;2:36. [Crossref] [PubMed]
- Liu Z, Wang S, Dong D, Wei J, Fang C, Zhou X, Sun K, Li L, Li B, Wang M, Tian J. The Applications of Radiomics in Precision Diagnosis and Treatment of Oncology: Opportunities and Challenges. Theranostics 2019;9:1303-22. [Crossref] [PubMed]
- Lambin P, Leijenaar RTH, Deist TM, Peerlings J, de Jong EEC, van Timmeren J, Sanduleanu S, Larue RTHM, Even AJG, Jochems A, van Wijk Y, Woodruff H, van Soest J, Lustberg T, Roelofs E, van Elmpt W, Dekker A, Mottaghy FM, Wildberger JE, Walsh S. Radiomics: the bridge between medical imaging and personalized medicine. Nat Rev Clin Oncol 2017;14:749-62. [Crossref] [PubMed]
- Avanzo M, Wei L, Stancanello J, Vallières M, Rao A, Morin O, Mattonen SA, El Naqa I. Machine and deep learning methods for radiomics. Med Phys 2020;47:e185-202. [Crossref] [PubMed]
- Qin Q, Shi A, Zhang R, Wen Q, Niu T, Chen J, Qiu Q, Wan Y, Sun X, Xing L. Cone-beam CT radiomics features might improve the prediction of lung toxicity after SBRT in stage I NSCLC patients. Thorac Cancer 2020;11:964-72. [Crossref] [PubMed]
- Fave X, Mackin D, Yang J, Zhang J, Fried D, Balter P, Followill D, Gomez D, Jones AK, Stingo F, Fontenot J, Court L. Can radiomics features be reproducibly measured from CBCT images for patients with non-small cell lung cancer? Med Phys 2015;42:6784-97. [Crossref] [PubMed]
- Sumner W, Kim SS, Vitzthum L, Moore K, Atwood T, Murphy J, Miyauchi S, Califano JA, Mell LK, Mundt AJ, Sharabi AB. End of treatment cone-beam computed tomography (CBCT) is predictive of radiation response and overall survival in oropharyngeal squamous cell carcinoma. Radiat Oncol 2021;16:147. [Crossref] [PubMed]
- Mireștean CC, Iancu RI, Iancu DPT. Image Guided Radiotherapy (IGRT) and Delta (Δ) Radiomics-An Urgent Alliance for the Front Line of the War against Head and Neck Cancers. Diagnostics (Basel) 2023;13:2045. [Crossref] [PubMed]
- Gu J, Zhu J, Qiu Q, Wang Y, Bai T, Duan J, Yin Y. The Feasibility Study of Megavoltage Computed Tomographic (MVCT) Image for Texture Feature Analysis. Front Oncol 2018;8:586. [Crossref] [PubMed]
- Kocak B, Akinci D'Antonoli T, Mercaldo N, Alberich-Bayarri A, Baessler B, Ambrosini I, et al. METhodological RadiomICs Score (METRICS): a quality scoring tool for radiomics research endorsed by EuSoMII. Insights Imaging 2024;15:8. [Crossref] [PubMed]
- Iliadou V, Kakkos I, Karaiskos P, Kouloulias V, Platoni K, Zygogianni A, Matsopoulos GK. Early Prediction of Planning Adaptation Requirement Indication Due to Volumetric Alterations in Head and Neck Cancer Radiotherapy: A Machine Learning Approach. Cancers (Basel) 2022;14:3573. [Crossref] [PubMed]
- Morgan HE, Wang K, Dohopolski M, Liang X, Folkert MR, Sher DJ, Wang J. Exploratory ensemble interpretable model for predicting local failure in head and neck cancer: the additive benefit of CT and intra-treatment cone-beam computed tomography features. Quant Imaging Med Surg 2021;11:4781-96. [Crossref] [PubMed]
- Abe K, Kadoya N, Ito K, Tanaka S, Nakajima Y, Hashimoto S, Suda Y, Uno T, Jingu K. Evaluation of the MVCT-based radiomic features as prognostic factor in patients with head and neck squamous cell carcinoma. BMC Med Imaging 2023;23:102. [Crossref] [PubMed]
- Sellami S, Bourbonne V, Hatt M, Tixier F, Bouzid D, Lucia F, Pradier O, Goasduff G, Visvikis D, Schick U. Predicting response to radiotherapy of head and neck squamous cell carcinoma using radiomics from cone-beam CT images. Acta Oncol 2022;61:73-80. [Crossref] [PubMed]
- Berger T, Noble DJ, Shelley LEA, McMullan T, Bates A, Thomas S, Carruthers LJ, Beckett G, Duffton A, Paterson C, Jena R, McLaren DB, Burnet NG, Nailon WH. Predicting radiotherapy-induced xerostomia in head and neck cancer patients using day-to-day kinetics of radiomics features. Phys Imaging Radiat Oncol 2022;24:95-101. [Crossref] [PubMed]
- Berger T, Noble DJ, Yang Z, Shelley LE, McMullan T, Bates A, Thomas S, Carruthers LJ, Beckett G, Duffton A, Paterson C, Jena R, McLaren DB, Burnet NG, Nailon WH. Sub-regional analysis of the parotid glands: model development for predicting late xerostomia with radiomics features in head and neck cancer patients. Acta Oncol 2023;62:166-73. [Crossref] [PubMed]
- Wang H, Zhou Y, Wang X, Zhang Y, Ma C, Liu B, Kong Q, Yue N, Xu Z, Nie K. Reproducibility and Repeatability of CBCT-Derived Radiomics Features. Front Oncol 2021;11:773512. [Crossref] [PubMed]
- Page MJ, McKenzie JE, Bossuyt PM, Boutron I, Hoffmann TC, Mulrow CD, et al. The PRISMA 2020 statement: an updated guideline for reporting systematic reviews. BMJ 2021;372: [Crossref] [PubMed]
- Benchoufi M, Matzner-Lober E, Molinari N, Jannot AS, Soyer P. Interobserver agreement issues in radiology. Diagn Interv Imaging 2020;101:639-41. [Crossref] [PubMed]
- Tran WT, Suraweera H, Quiaoit K, DiCenzo D, Fatima K, Jang D, Bhardwaj D, Kolios C, Karam I, Poon I, Sannachi L, Gangeh M, Sadeghi-Naini A, Dasgupta A, Czarnota GJ. Quantitative ultrasound delta-radiomics during radiotherapy for monitoring treatment responses in head and neck malignancies. Future Sci OA 2020;6:FSO624. [Crossref] [PubMed]
- Fatima K, Dasgupta A, DiCenzo D, Kolios C, Quiaoit K, Saifuddin M, Sandhu M, Bhardwaj D, Karam I, Poon I, Husain Z, Sannachi L, Czarnota GJ. Ultrasound delta-radiomics during radiotherapy to predict recurrence in patients with head and neck squamous cell carcinoma. Clin Transl Radiat Oncol 2021;28:62-70. [Crossref] [PubMed]
- Pota M, Scalco E, Sanguineti G, Farneti A, Cattaneo GM, Rizzo G, Esposito M. Early prediction of radiotherapy-induced parotid shrinkage and toxicity based on CT radiomics and fuzzy classification. Artif Intell Med 2017;81:41-53. [Crossref] [PubMed]
- Liu Y, Shi H, Huang S, Chen X, Zhou H, Chang H, Xia Y, Wang G, Yang X. Early prediction of acute xerostomia during radiation therapy for nasopharyngeal cancer based on delta radiomics from CT images. Quant Imaging Med Surg 2019;9:1288-302. [Crossref] [PubMed]
- van Dijk LV, Langendijk JA, Zhai TT, Vedelaar TA, Noordzij W, Steenbakkers RJHM, Sijtsema NM. Delta-radiomics features during radiotherapy improve the prediction of late xerostomia. Sci Rep 2019;9:12483. [Crossref] [PubMed]
- Bogowicz M, Leijenaar RTH, Tanadini-Lang S, Riesterer O, Pruschy M, Studer G, Unkelbach J, Guckenberger M, Konukoglu E, Lambin P. Post-radiochemotherapy PET radiomics in head and neck cancer - The influence of radiomics implementation on the reproducibility of local control tumor models. Radiother Oncol 2017;125:385-91. [Crossref] [PubMed]
- Spadarella G, Calareso G, Garanzini E, Ugga L, Cuocolo A, Cuocolo R. MRI based radiomics in nasopharyngeal cancer: Systematic review and perspectives using radiomic quality score (RQS) assessment. Eur J Radiol 2021;140:109744. [Crossref] [PubMed]
- Aringhieri G, Fanni SC, Febi M, Colligiani L, Cioni D, Neri E. The Role of Radiomics in Salivary Gland Imaging: A Systematic Review and Radiomics Quality Assessment. Diagnostics (Basel) 2022;12:3002. [Crossref] [PubMed]
- Park JE, Kim HS, Kim D, Park SY, Kim JY, Cho SJ, Kim JH. A systematic review reporting quality of radiomics research in neuro-oncology: toward clinical utility and quality improvement using high-dimensional imaging features. BMC Cancer 2020;20:29. [Crossref] [PubMed]
- Pinto Dos Santos D, Dietzel M, Baessler B. A decade of radiomics research: are images really data or just patterns in the noise? Eur Radiol 2021;31:1-4. [Crossref] [PubMed]
- Cannella R, Vernuccio F, Klontzas ME, Ponsiglione A, Petrash E, Ugga L, Pinto Dos Santos D, Cuocolo R. Systematic review with radiomics quality score of cholangiocarcinoma: an EuSoMII Radiomics Auditing Group Initiative. Insights Imaging 2023;14:21. [Crossref] [PubMed]
- Zhang HB, Lu X, Huang SM, Wang L, Zhao C, Xia WX, Li SW, Wang FL, Zhu YL, Guo X, Xiang YQ. Superficial parotid lobe-sparing delineation approach: a better method of dose optimization to protect the parotid gland in intensity-modulated radiotherapy for nasopharyngeal carcinoma. Curr Oncol 2013;20:e577-84. [Crossref] [PubMed]
- Miah AB, Gulliford SL, Morden J, Newbold KL, Bhide SA, Zaidi SH, Hall E, Harrington KJ, Nutting CM. Recovery of Salivary Function: Contralateral Parotid-sparing Intensity-modulated Radiotherapy versus Bilateral Superficial Lobe Parotid-sparing Intensity-modulated Radiotherapy. Clin Oncol (R Coll Radiol) 2016;28:e69-e76. [Crossref] [PubMed]
- Fried DV, Zhu T, Das SK, Shen C, Marks LB, Tan X, Chera BS. Prospective assessment of sparing the parotid ducts via MRI sialography for reducing patient reported xerostomia. Radiother Oncol 2022;172:42-9. [Crossref] [PubMed]
- Alam SR, Zhang P, Zhang SY, Chen I, Rimner A, Tyagi N, Hu YC, Lu W, Yorke ED, Deasy JO, Thor M. Early Prediction of Acute Esophagitis for Adaptive Radiation Therapy. Int J Radiat Oncol Biol Phys 2021;110:883-92. [Crossref] [PubMed]
- Shur JD, Doran SJ, Kumar S, Ap Dafydd D, Downey K, O'Connor JPB, Papanikolaou N, Messiou C, Koh DM, Orton MR. Radiomics in Oncology: A Practical Guide. Radiographics 2021;41:1717-32. [Crossref] [PubMed]
- van Timmeren JE, Leijenaar RTH, van Elmpt W, Reymen B, Lambin P. Feature selection methodology for longitudinal cone-beam CT radiomics. Acta Oncol 2017;56:1537-43. [Crossref] [PubMed]
- Ligero M, Jordi-Ollero O, Bernatowicz K, Garcia-Ruiz A, Delgado-Muñoz E, Leiva D, Mast R, Suarez C, Sala-Llonch R, Calvo N, Escobar M, Navarro-Martin A, Villacampa G, Dienstmann R, Perez-Lopez R. Minimizing acquisition-related radiomics variability by image resampling and batch effect correction to allow for large-scale data analysis. Eur Radiol 2021;31:1460-70. [Crossref] [PubMed]
- Hu F, Chen AA, Horng H, Bashyam V, Davatzikos C, Alexander-Bloch A, Li M, Shou H, Satterthwaite TD, Yu M, Shinohara RT. Image harmonization: A review of statistical and deep learning methods for removing batch effects and evaluation metrics for effective harmonization. Neuroimage 2023;274:120125. [Crossref] [PubMed]