Preliminary experiments on interpretable ChatGPT-assisted diagnosis for breast ultrasound radiologists
Introduction
Breast cancer is one of the most common malignancies among women, with its incidence and mortality rates on the rise globally (1). Ultrasound plays a vital role in detecting breast lesions, serving as a first-line screening tool. In China, where many women have dense breast tissue, ultrasound is often the preferred imaging modality (2). The American College of Radiology’s Breast Imaging Reporting and Data System (BI-RADS) (3) provides a clear classification of breast tumor malignancy, which is essential for devising treatment plans and assessing prognosis, and is widely applied in clinical practice. However, due to its subjective nature, diagnostic results may vary across physicians with different levels of experience and from different regions (4).
In recent years, artificial intelligence (AI) has demonstrated outstanding performance in cognitive tasks (5-10). The introduction of the large language model (LLM) ChatGPT by OpenAI represents a significant advancement in natural language (6) processing, offering substantial potential for improving diagnostic accuracy and efficiency (9) while reducing human errors in the medical field (11).
Despite the potential benefits, AI models face limitations in specialized domains such as medical diagnosis, including the scarcity of training data, which can impair the model’s capacity for generalization and precise prediction making (12). Furthermore, AI models may not be sufficiently effective or dependable for use in difficult medical diagnostic tasks (13). Moreover, the “black box” nature of AI models, particularly in the context of medicine, can present significant challenges due to the lack of transparency in decision-making (14). This opacity can lead to mistrust and hinder the wider adoption of AI technologies in critical areas such as healthcare. Consequently, research into model interpretability is not only essential, but also timely (15). The “chain of thought” (CoT) methodology we employed in our previous study represents an attempt at improving model interpretability (16). This method provides a visual breakdown of the AI’s decision-making process, thereby enhancing our understanding of how the AI model arrives at a given conclusion. Illuminating the AI decision-making process can improve AI performance, foster trust, and facilitate the smoother integration of AI into healthcare by addressing one of the major concerns of healthcare professionals—the unpredictability and opacity of AI decision-making.
This study aimed to clarify the potential of the ChatGPT-3.5 model to help ultrasound doctors effectively determine BI-RADS classification, improve diagnostic performance in clinical settings, and analyze the causes of misdiagnosis, to better understand the limitations of LLM in this context. We present this article in accordance with the STROBE reporting checklist (available at https://qims.amegroups.com/article/view/10.21037/qims-24-141/rc).
Methods
Data collection
From March 2023 to April 2023, we retrospectively collected data from patients with breast cancer treated at Beijing Friendship Hospital, Capital Medical University. All patients with breast masses classified as BI-RADS 4a or higher underwent either core needle biopsy or surgical pathology to confirm their diagnosis. Patients with BI-RADS 2 and 3 lesions were followed up for 3–5 years as typical benign cases. In total, 131 ultrasound reports from 131 patients were included, all of whom were female, with an average age of 43 (range, 21–78) years. Benign cases included breast cysts, fibroadenomas, and mammary gland diseases, while malignant cases were all invasive breast cancers. This study was conducted in accordance with the Declaration of Helsinki (as revised in 2013) and received ethical approval from the Medical Ethics Committee of Beijing Friendship Hospital, Capital Medical University (No. 2022-P2-060-01). The requirement for individual consent was waived due to the retrospective nature of the analysis.
A total of 57 evaluating doctors participated in the study, including 20 junior doctors (1–5 years of experience), 18 intermediate doctors (6–10 years of experience), and 19 senior doctors (>10 years of experience). They were from 57 hospitals, including Binzhou Central Hospital in Shandong Province, Beijing Children’s Hospital Affiliated with Capital Medical University, and Beijing Friendship Hospital Affiliated with Capital Medical University.
Diagnostic results generated by ChatGPT
The ChatGPT (17) series, created by OpenAI, is a cutting-edge pretrained language model that is capable of performing intricate natural language processing tasks, including generating articles, answering questions (18), translating languages, and producing code. The workflow of this study is illustrated in Figure 1. In our analysis, we input breast ultrasound medical reports into ChatGPT-3.5 and prompted it to generate diagnoses and treatment recommendations (TR). We subsequently collected the output reports for evaluation. Figure S1 shows the process of question and answer collection. The prompt provided was as follows: “Based on the following breast ultrasound description, please provide a comprehensive diagnosis (BI-RADS classification) and corresponding treatment recommendations”.
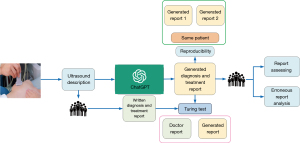
Evaluation of report performance
In order to gain deeper insights into ChatGPT’s effectiveness in producing diagnostic reports for breast cancer, we gathered and assessed the ratings of these reports based on a specific set of evaluation criteria (see Table S1). These criteria included structure and organization (S&O), professional terminology and expression (PTE), BI-RADS classification, malignancy diagnosis (MD), TR, clarity and comprehensibility (C&C), likelihood of being written by a physician (LWBP), ultrasound doctor AI acceptance (UDAIA), and overall evaluation (OE). Each criterion was rated on a scale of 1 to 5, with 1 indicating completely incorrect or unsatisfactory and 5 indicating completely correct or satisfactory. The details of the scoring table can be found in the supplementary materials (Table S2). Furthermore, we assessed the proficiency of AI-generated reports by juxtaposing their evaluations with those provided by physicians possessing varying degrees of clinical experience.
Turing test and reproducibility experiment
To evaluate doctors’ ability to distinguish between human-written and AI-generated reports (19), we incorporated 50% of the ChatGPT-generated reports into the evaluation set. Doctors assessed the likelihood that each report was authored by a physician, and we calculated the rate of accurate identifications. If their accuracy surpassed random guessing (50%), it would indicate that ChatGPT successfully passed the Turing test.
To evaluate the consistency of ChatGPT’s responses, we conducted a comparison of two outputs produced by distinct transient model instances (20). For each inquiry, we analyzed the scores allocated to both responses and conducted a statistical assessment to identify significant disparities (21), thereby offering insights into the reliability of ChatGPT’s performance.
Erroneous report analysis
We collected and examined misdiagnosed reports, defined as those with low scores (1–2 points). Two doctors with 12 years of experience analyzed and categorized the reasons for these errors (22). This analysis aimed to identify patterns and potential weaknesses in the ChatGPT model in order to guide future improvements and training strategies (23).
GPT CoT visualization
The CoT (16) method breaks down the decision-making process of the GPT model into several stages, depicting it as a flowchart. This method provides a clear and insightful means to scrutinizing the model’s decision-making patterns, enhancing our understanding of its diagnostic process. The visual representation elucidates the decisions made by ChatGPT-3.5 in assigning a BI-RADS score, assessing malignancy, suggesting a treatment plan, and generating a diagnostic report.
Statistical analyses
The statistical analysis was carried out using the Mann-Whitney test (24), provided by the SciPy package (25) with all code written in Python 3.8 (Python Software Foundation, Wilmington, DE, USA). A P value lower than 0.05 was deemed to be statistically significant.
Results
Report generation performance evaluation result
The mean values of the ChatGPT-3.5 performance in medical reports for different metrics were as follows: S&O, 4.08 [95% confidence interval (CI): 3.99–4.17], PTE, 4.08 (95% CI: 3.99–4.18); BI-RADS classification, 3.77 (95% CI: 3.64–3.90); MD, 3.86 (95% CI: 3.74–3.98); TR, 4.03 (95% CI: 3.93–4.14); C&C, 4.00 (95% CI: 3.89–4.10); UDAIA, 3.92 (95% CI: 3.81–4.03); and OE, 3.89 (95% CI: 3.77–4.00). The results can be found in Figure 2A. ChatGPT-3.5 exhibited remarkable performance in S&O, PTE, TR, and C&C, with scores approaching or surpassing 4. However, the scores for BI-RADS, MD, LWBP, and UDAIA were slightly lower, indicating areas in need of improvement. In summary, ChatGPT-3.5 achieved an OE score of 3.89, indicating that its performance was deemed generally acceptable. We employed a radar chart to exhibit the performance of various types of physicians and the AI system (see Figure 2B), which indicated that ChatGPT-3.5 has comparable performance to doctors in multiple aspects, particularly excelling in S&O, PTE, TR, and C&C. We conducted statistical analyses to compare the performance of ChatGPT-3.5 with that of doctors. The Mann-Whitney test indicated that the differences between the AI and doctors were statistically significant for BI-RADS classification (P value =0.028) and MD (P value =0.033). These findings suggest that while ChatGPT-3.5 performs well, there are certain areas in which expertise still outperforms AI.
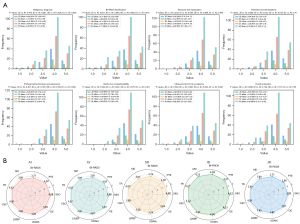
Agreement analysis
To further evaluate the agreement between doctors and ChatGPT, we performed Cohen kappa analysis. The Cohen kappa coefficient for BI-RADS Classification was 0.68, indicating substantial agreement between the AI and physicians. This suggests that while there are discrepancies, the AI-generated reports are generally in alignment with those written by doctors.
Turing test results
We used comparative bar charts and pie charts to evaluate the distinctions between AI-generated reports and those authored by human doctors (with a score of 5 representing a high likelihood of being human written and a score of 1 denoting a very low likelihood). The proportion of doctor-written reports that garnered a score of 5 was 33.70%, whereas AI-generated reports exhibited a marginally higher proportion in this category at 35.34%. This observation suggests that AI-generated reports convincingly approximate the characteristics of reports composed by medical professionals.
Reproducibility analysis
Figure 3 Boxplot illustrating the score distribution of ChatGPT-generated reports for the same patient across various time intervals. The results indicated consistent a performance across various evaluation criteria. The key mean scores for both experiments included those for S&O (4.12 and 4.07; P=0.59), PTE (4.18 and 4.00; P=0.19), and C&C (4.09 and 3.63; P=0.048). The AI-generated medical reports showed consistent performance throughout the experiments, with high mean scores being maintained for most criteria. Although some variations were observed in specific areas, such as in BI-RADS classification (3.88 and 3.37; P=0.06) and MD (3.91 and 3.40; P=0.047), the overall performance of the AI in generating medical reports remains promising. The consistency in scores across most of the evaluation criteria warrants further investigation into the potential applications and development of AI-generated medical reports.
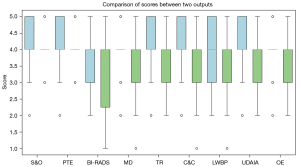
Erroneous report analysis
The following is a summary of results for the erroneous reports generated by ChatGPT and reviewed by clinical doctors: For incorrect diagnoses, cases with low scores (score 1–2) indicated errors in distinguishing between benign and malignant diagnoses. For example, a benign case was diagnosed as BI-RADS 4b even though we defined 4a and above as malignant.
In terms of inconsistencies, the BI-RADS classification did not always correspond with the appropriate clinical recommendations, leading to inconsistencies in the generated report’s content. For instance, a report indicated a benign diagnosis but suggested a biopsy. This inconsistency may be due to the model’s inability to fully understand the context and relationships between different sections of the report. For overdiagnosis, there was overdiagnosis in some benign lesions.
CoT visualization
The visualization results in Figure 4 depict the key steps and considerations in the decision-making process of the ChatGPT model. First, the model extracts crucial information from the patient’s ultrasound reports, such as breast echogenicity, presence or absence of masses and abnormal blood flow in the breasts, characteristics of any nodules found, and axillary lymph node status. Next, with this data, the model calculates the BI-RADS score, a crucial metric in assessing breast cancer. The calculation involves an evaluation of the breast echogenicity, structural disorder, presence or absence of masses and abnormal blood flow, characteristics of nodules, and lymph node status. Further, the model combines the previously calculated BI-RADS score. This step is not merely an evaluation of individual parameters but also an integrated risk assessment that computes the likelihood of cancer. Finally, based on the above information and diagnosis result, the model synthesizes all this information to provide a suggestion on what treatment might be suitable. This implies that the final suggestion is not solely dependent on a single parameter or result but is a comprehensive consideration of the risk level of breast cancer. Our visualization chart provides a clear and explicit representation of this process, enabling us to better understand the decision-making logic of the model in the diagnostic and treatment suggestion process. Key nodes in the model’s thought chain, such as BI-RADS score and nodule characteristics, are clearly highlighted. This research offers insight into the cognitive processes underlying the decision-making framework of the ChatGPT model in the diagnosis and recommendation of therapeutic interventions for breast cancer.
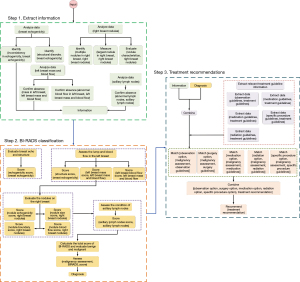
Discussion
In this study, we assessed ChatGPT’s performance in generating breast cancer diagnosis reports, concentrating on report scoring, quality comparisons among doctors with varying experience levels, Turing test outcomes, reproducibility analysis, and erroneous report examination. Our findings offer valuable insights into ChatGPT’s present capabilities, highlighting potential areas for improvement and practical applications within the medical domain.
Report performance
The evaluation of ChatGPT-3.5’s performance in generating medical reports, based on metrics such as S&O, PTE, TR, and C&C, yielded promising results. With mean scores around or above 4, the AI demonstrated potential for producing high-quality reports comparable to those written by radiologists. Literature also supports the promise of automated systems in medical documentation (26,27).
However, the AI’s performance in BI-RADS classification, MD, and UDAIA needs improvement. This aligns with the findings of Pang et al. (28), who noted issues in AI’s accuracy in specific medical classification tasks, and of Zhou et al. (29), who identified challenges in complex decision support and multidimensional data analysis. Thus, while ChatGPT-3.5 excels in various areas, further development is needed for comprehensive and accurate performance.
The radar chart comparison (Figure 2B) between different doctors and the AI highlights its potential in medical report generation. The literature suggests AI’s significant promise in assisting healthcare professionals (30), supporting these findings.
Turning test and reproducibility
The Turing test results provide valuable insights into AI’s ability emulate reports written by human physicians. Figure 5 shows that 35.34% of AI-generated reports achieved a score of 5, indicating a high likelihood of being perceived as human written. This slightly surpassed the 33.70% for doctor-authored reports, suggesting that AI-generated reports can closely resemble doctor-authored reports and sometimes even surpass them in perceived authenticity. Thus, AI could streamline the medical reporting process, reduce healthcare professionals’ workload, and allow more time for patient care (30-32).
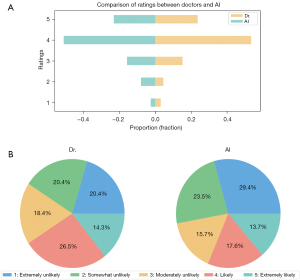
The reproducibility of experiment results (Figure 3) further demonstrated the consistency and reliability of AI-generated reports across multiple evaluation criteria. The AI’s performance showed high consistency in S&O, PTE, and C&C, underscoring its potential in maintaining high quality in medical reporting (31,32).
However, the inconsistency in BI-RADS classification and MD still needs to be addressed. Previous studies have noted similar challenges, where AI systems exhibited variability in accuracy for certain medical tasks (32,33). Resolving these issue will improve AI-generated report quality and bolster healthcare professionals’ confidence in in them for decision-making.
Erroneous reports
The results of erroneous reports indicated several shortcomings in ChatGPT’s handling of medical texts. First, the inconsistencies suggest that the model struggles with long-text comprehension, leading to context and relationship discrepancies within reports (34,35). Improving attention mechanisms could mitigate these issues. Second, ChatGPT’s reliance on physician descriptions without independent image analysis resulted in overdiagnosis in benign cases. Integrating computer vision techniques could improve diagnostic accuracy (36).
Finally, the model often overlooked details in cases with multiple lesions, focusing on high-malignancy descriptions and missing others. Enhancing multisource information processing could address this flaw (37).
CoT
The interpretability of AI models is crucial in healthcare, as it allows doctors and patients to understand and trust AI decisions, significantly improving patient outcomes. The CoT concept helps trace the AI’s thought process, identifying potential weaknesses and biases, thereby enhancing performance and building user trust. This is vital for the integration of AI into healthcare settings (38,39).
Explainability involves understanding why the model makes a given classification, such as assigning a BI-RADS score. This requires evaluating features such as nodule size, shape, margins, and microcalcifications to provide a clear rationale behind recommendations. Previous studies emphasize the importance of explainability in AI for healthcare, highlighting its role in improving trust and acceptance among users (40,41).
Limitations and future work
ChatGPT still has several limitations in the medical context. First, the model’s inability to analyze images directly, relying solely on physician-provided text, suggests there is a need to integrate computer vision techniques (42-44). Second, longer texts can lead to inconsistencies, and addressing this requires improving the comprehension and generation of longer texts. Third, specialized fields such as ultrasound report analysis require more domain-specific knowledge. Future research should focus on incorporating expert knowledge and clinical guidelines (36,45). Fourth, potential biases should be considered, as physicians’ familiarity with AI-generated reports might influence their assessments. Ensuring trust and transparency involves robust validation processes, clear documentation, and human oversight (46,47). Finally, the generalizability of this study may be limited to breast cancer diagnosis. Further research should explore AI-generated reports in other medical domains (23,48).
Conclusions
The findings of this study support ChatGPT’s potential in analyzing breast ultrasound reports and providing diagnostic and TR. It exhibited strong performance across various evaluation criteria and convincingly emulated reports written by physicians. Moreover, the reproducibility results indicate a high level of consistency in essential aspects of medical reporting. However, the analysis of erroneous reports suggests that there are several areas where improvements are needed, including model understanding and context, image analysis, and the handling of multiple lesions. Furthermore, the visual dissection of the AI’s CoT provides invaluable insights into the decision-making process, highlighting the importance of model interpretability for enhancing performance, building user trust, and effectively integrating AI into healthcare environments.
Acknowledgments
We express our sincere gratitude to the 57 medical professionals from various institutions who participated in this study.
Funding: This study was supported by
Footnote
Reporting Checklist: The authors have completed the STROBE reporting checklist. Available at https://qims.amegroups.com/article/view/10.21037/qims-24-141/rc
Conflicts of Interest: All authors have completed the ICMJE uniform disclosure form (available at https://qims.amegroups.com/article/view/10.21037/qims-24-141/coif). The authors have no conflicts of interest to declare.
Ethical Statement: The authors are accountable for all aspects of the work in ensuring that questions related to the accuracy or integrity of any part of the work are appropriately investigated and resolved. This study was conducted in accordance with the Declaration of Helsinki (as revised in 2013) and ethical approval for this study was obtained from the Medical Ethics Committee of Beijing Friendship Hospital, Capital Medical University (No. 2022-P2-060-01). The requirement for individual consent was waived due to the retrospective nature of the analysis.
Open Access Statement: This is an Open Access article distributed in accordance with the Creative Commons Attribution-NonCommercial-NoDerivs 4.0 International License (CC BY-NC-ND 4.0), which permits the non-commercial replication and distribution of the article with the strict proviso that no changes or edits are made and the original work is properly cited (including links to both the formal publication through the relevant DOI and the license). See: https://creativecommons.org/licenses/by-nc-nd/4.0/.
References
- Bray F, Ferlay J, Soerjomataram I, Siegel RL, Torre LA, Jemal A. Global cancer statistics 2018: GLOBOCAN estimates of incidence and mortality worldwide for 36 cancers in 185 countries. CA Cancer J Clin 2018;68:394-424. [Crossref] [PubMed]
- Madjar H. Role of Breast Ultrasound for the Detection and Differentiation of Breast Lesions. Breast Care (Basel) 2010;5:109-14. [Crossref] [PubMed]
- Amuasi AA, Acheampong AO, Anarfi E, Sagoe ES, Poku RD, Abu-Sakyi J. Effect of malocclusion on quality of life among persons aged 7-25 years: A cross-sectional study. J Biosci Med (Irvine) 2020;8:26-35.
- Elmore JG, Longton GM, Carney PA, Geller BM, Onega T, Tosteson AN, Nelson HD, Pepe MS, Allison KH, Schnitt SJ, O'Malley FP, Weaver DL. Diagnostic concordance among pathologists interpreting breast biopsy specimens. JAMA 2015;313:1122-32. [Crossref] [PubMed]
- Silver D, Huang A, Maddison CJ, Guez A, Sifre L, van den Driessche G, Schrittwieser J, Antonoglou I, Panneershelvam V, Lanctot M, Dieleman S, Grewe D, Nham J, Kalchbrenner N, Sutskever I, Lillicrap T, Leach M, Kavukcuoglu K, Graepel T, Hassabis D. Mastering the game of Go with deep neural networks and tree search. Nature 2016;529:484-9. [Crossref] [PubMed]
- Brown TB, Mann B, Ryder N, Subbiah M, Amodei D. Language Models are Few-Shot Learners. Part of Advances in Neural Information Processing Systems 33 (NeurIPS 2020), 2020.
- Mallio CA, Bernetti C, Sertorio AC, Zobel BB. ChatGPT in radiology structured reporting: analysis of ChatGPT-3.5 Turbo and GPT-4 in reducing word count and recalling findings. Quant Imaging Med Surg 2024;14:2096-102. [Crossref] [PubMed]
- Wang Z, Zhang Z, Traverso A, Dekker A, Qian L, Sun P. Assessing the role of GPT-4 in thyroid ultrasound diagnosis and treatment recommendations: enhancing interpretability with a chain of thought approach. Quant Imaging Med Surg 2024;14:1602-15. [Crossref] [PubMed]
- Ferrucci D, Brown E, Chu-Carroll J, Fan J, Gondek D, Kalyanpur AA, Lally A, Murdock JW, Nyberg E, Prager J, Schlaefer N, Welty C. Building Watson: An Overview of the DeepQA Project. Ai Magazine 2010;31:59-79.
- Schrittwieser J, Antonoglou I, Hubert T, Simonyan K, Sifre L, Schmitt S, Guez A, Lockhart E, Hassabis D, Graepel T, Lillicrap T, Silver D. Mastering Atari, Go, chess and shogi by planning with a learned model. Nature 2020;588:604-9. [Crossref] [PubMed]
- Wartman SA, Combs CD. Reimagining Medical Education in the Age of AI. AMA J Ethics 2019;21:E146-52. [Crossref] [PubMed]
- Gulshan V, Peng L, Coram M, Stumpe MC, Wu D, Narayanaswamy A, Venugopalan S, Widner K, Madams T, Cuadros J, Kim R, Raman R, Nelson PC, Mega JL, Webster DR. Development and Validation of a Deep Learning Algorithm for Detection of Diabetic Retinopathy in Retinal Fundus Photographs. JAMA 2016;316:2402-10. [Crossref] [PubMed]
- Finlayson SG, Bowers JD, Ito J, Zittrain JL, Beam AL, Kohane IS. Adversarial attacks on medical machine learning. Science 2019;363:1287-9. [Crossref] [PubMed]
- Castelvecchi D. Can we open the black box of AI? Nature 2016;538:20-3. [Crossref] [PubMed]
- Lipton ZC. The Mythos of Model Interpretability: In machine learning, the concept of interpretability is both important and slippery. Queue 2018;16:31-57.
- Wei J, Wang X, Schuurmans D, Bosma M, Ichter B, Xia F, Chi E, Le QV, Zhou D. Chain-of-Thought Prompting Elicits Reasoning in Large Language Models. Part of Advances in Neural Information Processing Systems 35 (NeurIPS 2022) Main Conference Track, 2022.
- Radford A, Narasimhan K, Salimans T, Sutskever I. Improving language understanding by generative pre-training. 2018.
- Jiang F, Jiang Y, Zhi H, Dong Y, Li H, Ma S, Wang Y, Dong Q, Shen H, Wang Y. Artificial intelligence in healthcare: past, present and future. Stroke Vasc Neurol 2017;2:230-43. [Crossref] [PubMed]
- Turing AM. Computing Machinery and Intelligence. In: Epstein R, Roberts G, Beber G. editors. Parsing the Turing Test. Springer, Dordrecht, 2009:23-65.
Bengio Y Léonard N Courville A. Estimating or Propagating Gradients Through Stochastic Neurons for Conditional Computation. arXiv: 1308.3432,2013 .- Bland JM, Altman DG. Statistical methods for assessing agreement between two methods of clinical measurement. Lancet 1986;1:307-10.
- Graber ML, Franklin N, Gordon R. Diagnostic error in internal medicine. Arch Intern Med 2005;165:1493-9. [Crossref] [PubMed]
- Topol EJ. High-performance medicine: the convergence of human and artificial intelligence. Nat Med 2019;25:44-56. [Crossref] [PubMed]
- Mcknight PE, Najab J. Mann-Whitney U Test. The Corsini Encyclopedia of Psychology, 2010.
- Virtanen P, Gommers R, Oliphant TE, Haberland M, Reddy T, Cournapeau D, et al. Author Correction: SciPy 1.0: fundamental algorithms for scientific computing in Python. Nat Methods 2020;17:352. [Crossref] [PubMed]
- Najjar R. Redefining Radiology: A Review of Artificial Intelligence Integration in Medical Imaging. Diagnostics (Basel) 2023;13:2760. [Crossref] [PubMed]
- Ahmed SB, Solis-Oba R, Ilie L. Explainable-AI in Automated Medical Report Generation Using Chest X-ray Images. Appl Sci 2022;12:11750.
- Pang T, Li P, Zhao L. A survey on automatic generation of medical imaging reports based on deep learning. Biomed Eng Online 2023;22:48. [Crossref] [PubMed]
- Zhou Y, Wang B, He X, Cui S, Shao L DR-GAN. Conditional Generative Adversarial Network for Fine-Grained Lesion Synthesis on Diabetic Retinopathy Images. IEEE J Biomed Health Inform 2022;26:56-66. [Crossref] [PubMed]
- Acosta JN, Falcone GJ, Rajpurkar P, Topol EJ. Multimodal biomedical AI. Nat Med 2022;28:1773-84. [Crossref] [PubMed]
- Lundervold AS, Lundervold A. An overview of deep learning in medical imaging focusing on MRI. Z Med Phys 2019;29:102-27. [Crossref] [PubMed]
- Shen D, Wu G, Suk HI. Deep Learning in Medical Image Analysis. Annu Rev Biomed Eng 2017;19:221-48. [Crossref] [PubMed]
- Jha S, Topol EJ. Adapting to Artificial Intelligence: Radiologists and Pathologists as Information Specialists. JAMA 2016;316:2353-4. [Crossref] [PubMed]
- Vaswani A, Shazeer N. armar N, Uszkoreit J, Jones L, Gomez AN, Kaiser L, Polosukhin I. Attention is all you need. Advances in Neural Information Processing Systems 2017;30:5998-6008.
.Devlin J Chang MW Lee K Toutanova K BERT: Pre-training of Deep Bidirectional Transformers for Language Understanding. arXiv: 1810.04805,2019 .Rajpurkar P Irvin J Zhu K Yang B Mehta H Duan T Ding D Bagul A Langlotz C Shpanskaya K. CheXNet: Radiologist-Level Pneumonia Detection on Chest X-Rays with Deep Learning. arXiv:1711.05225,2017 .- Ardila D, Kiraly AP, Bharadwaj S, Choi B, Reicher JJ, Peng L, Tse D, Etemadi M, Ye W, Corrado G, Naidich DP, Shetty S. Author Correction: End-to-end lung cancer screening with three-dimensional deep learning on low-dose chest computed tomography. Nat Med 2019;25:1319. [Crossref] [PubMed]
- Frasca M, Torre DL, Pravettoni G, Cutica I. Explainable and interpretable artificial intelligence in medicine: a systematic bibliometric review. Explainable and interpretable artificial intelligence in medicine: a systematic bibliometric review. Discov Artif Intell 2024;4:15.
- Amann J, Blasimme A, Vayena E, Frey D, Madai VI. Precise4Q consortium. Explainability for artificial intelligence in healthcare: a multidisciplinary perspective. BMC Med Inform Decis Mak 2020;20:310. [Crossref] [PubMed]
- Chaddad A, Peng J, Xu J, Bouridane A. Survey of Explainable AI Techniques in Healthcare. Sensors (Basel) 2023;23:634. [Crossref] [PubMed]
- Gerdes A. The role of explainability in AI-supported medical decision-making. Discov Artif Intell 2024;4:29.
- Esteva A, Robicquet A, Ramsundar B, Kuleshov V, DePristo M, Chou K, Cui C, Corrado G, Thrun S, Dean J. A guide to deep learning in healthcare. Nat Med 2019;25:24-9. [Crossref] [PubMed]
- Sakamoto SI, Hutabarat Y, Owaki D, Hayashibe M. Ground Reaction Force and Moment Estimation through EMG Sensing Using Long Short-Term Memory Network during Posture Coordination. Cyborg Bionic Syst 2023;4:0016.
- Zhan G, Wang W, Sun H, Hou Y, Feng L. Auto-CSC: A Transfer Learning Based Automatic Cell Segmentation and Count Framework. Cyborg Bionic Syst 2022;2022:9842349. [Crossref] [PubMed]
- Tschandl P, Rinner C, Apalla Z, Argenziano G, Codella N, Halpern A, Janda M, Lallas A, Longo C, Malvehy J, Paoli J, Puig S, Rosendahl C, Soyer HP, Zalaudek I, Kittler H. Human-computer collaboration for skin cancer recognition. Nat Med 2020;26:1229-34. [Crossref] [PubMed]
.Samek W Wiegand T Müller KR Explainable Artificial Intelligence: Understanding, Visualizing and Interpreting Deep Learning Models. arXiv:1708.08296,2017 .- Holzinger A, Langs G, Denk H, Zatloukal K, Müller H. Causability and explainability of artificial intelligence in medicine. Wiley Interdiscip Rev Data Min Knowl Discov 2019;9:e1312. [Crossref] [PubMed]
- Sun G, Zhou YH. AI in healthcare: navigating opportunities and challenges in digital communication. Front Digit Health 2023;5:1291132. [Crossref] [PubMed]