Accelerating brain three-dimensional T2 fluid-attenuated inversion recovery using artificial intelligence-assisted compressed sensing: a comparison study with parallel imaging
Introduction
The T2 fluid-attenuated inversion recovery (FLAIR) technique has emerged as a pivotal tool in magnetic resonance imaging (MRI) for the diagnosis of a wide range of intracranial lesions, including stroke, hemorrhage, tumor, and inflammatory lesions, such as multiple sclerosis (1-3). Traditionally, T2 FLAIR imaging has been performed with a two-dimensional (2D) acquisition method and slice thicknesses ranging from 5 to 6 mm (4-7), but there has been a growing demand for a higher image resolution that can facilitate the detailed visualization of small intracranial lesions. The advent of three-dimensional (3D) T2 FLAIR represents a major advancement in MRI technology, leveraging the benefits of thin contiguous slices, isotropic voxels, and the capability of multiplanar reformatting. Compared to 2D T2 FLAIR, 3D T2 FLAIR offers a superior signal-to-noise ratio (SNR) and contrast-to-noise ratio (CNR), minimizes pulsation artifacts, facilitates the detection of a greater detail and number of lesions, and progressively expands the application of MRI in the field of neuroscience (4,6-8). However, the long acquisition time of conventional 3D T2 FLAIR can cause motion artifacts in images, limiting its utility in clinical contexts (4,9).
In order to shorten the scanning time and enhance the 3D T2 FLAIR image quality, parallel imaging (PI) is commonly applied in practice, which involves sensitivity encoding (SENSE) and controlled aliasing in parallel imaging resulting in higher acceleration (CAIPIRINHA) (10-12). Recently, compressed sensing (CS) has also been used for accelerating 3D T2 FLAIR (13-16). However, achieving substantial acceleration, such as reducing the 3D T2 FLAIR sequence time to under 120 seconds, while still maintaining high image quality remains elusive (11,13,14).
Artificial intelligence (AI) is increasingly being recognized as an innovative solution in MRI data acquisition, reconstruction, and image synthesis, enabling levels of image quality and computational efficiency that rival—or in some instances—surpass those of traditional methods (17,18). A pioneering methodology that combines partial Fourier transform, PI, CS, and convolutional neural networks (CNNs), named AI-assisted compressed sensing (ACS), has been developed. This approach has been shown to significantly reduce MRI acquisition times, with promising outcomes already observed in 2D T2 FLAIR applications (19,20). However, the effectiveness and applicability of ACS for brain 3D T2 FLAIR have not yet been fully established. Thus, the objective of this study was to assess the effectiveness of ACS-accelerated 3D T2 FLAIR via comparison to PI-accelerated 3D T2 FLAIR in both healthy individuals and patients with specific intracranial conditions. The findings of our study may contribute to developing more rapid and comfortable scanning techniques and providing more accurate diagnoses for patients with range of neurological challenges. We present this article in accordance with the STROBE reporting checklist (available at https://qims.amegroups.com/article/view/10.21037/qims-24-722/rc).
Methods
Patients
This prospective cohort study was conducted in accordance with the Declaration of Helsinki (as revised in 2013) and was approved by the Ethics Committee of Beijing Tiantan Hospital (No. KY2022-078-02). Written informed consent was obtained from the patients. From February 2023 to October 2023, 111 consecutive participants (52 males and 59 females; mean age 50.1±16.5 years; age range, 14–83 years) with suspected symptomatic brain diseases or healthy participants who could complete the subsequent MRI scanning were enrolled in this study. The inclusion criteria were as follows: participants with suspected intracranial lesions (mainly including space-occupying lesions and vascular diseases), completion of postoperative re-examination, and healthy volunteers. The exclusion criteria were images with severe motion artifacts.
Image acquisition
Each participant was scanned head first in the supine position with a 3.0-T system (uMR 880; United Imaging Healthcare, Shanghai, China) equipped with a 48-channel head and neck coil. A routine brain MRI sequence including transverse T2-weighted fast spin echo (FSE), sagittal 3D T1-weighted ultrafast spoiled gradient echo (3D T1W GRE_FSP), and transverse diffusion-weighted echo planar imaging was carried out for all participants, with a portion undergoing additional transverse magnetic resonance angiography (MRA) or contrast-enhanced 3D T1W GRE_FSP scanning. Two specialized non-contrast-enhanced sagittal 3D T2 FLAIR sequences with medium resolution (1.1×1.1×1.1 mm3) were tailored to assess the performance of ACS versus that of the conventional PI technique [under generalized autocalibrating partially parallel acquisitions (GRAPPA)], as a slice thickness <1.5 mm is recommended for multiple sclerosis and brain tumors examined under brain 3D FALIR (21,22). A preliminary experiment was conducted before this study, in which a wide range of ACS accelerations from 2 to 10 were tested, and finally, ACS with an acceleration factor of 7.34 was determined to provide the optimal balance between acquisition time and overall image quality. The specific details of the imaging parameters are provided in Table 1.
Table 1
Parameter | ACS-T2 FLAIR | PI-T2 FLAIR |
---|---|---|
Scan mode | 3D | 3D |
Position | Sagittal | Sagittal |
Phase encoding | A-P | A-P |
TR/TE/TI (ms) | 5,000/433/1,569 | 5,000/433/1,569 |
Average | 1 | 1 |
Voxel (mm) | 1.1×1.1×1.1 | 1.1×1.1×1.1 |
Field of view (mm) | 264×232 | 264×232 |
Number of slices | 160 | 160 |
Acceleration type/factor | ACS/7.34 | PI/2.23 |
Bandwidth (Hz/pixel) | 750 | 750 |
Echo train length | 250 | 250 |
Fat suppression | Yes | Yes |
Scan time (s) | 105 | 320 |
3D T2 FLAIR, three-dimensional T2 fluid-attenuated inversion recovery; ACS, artificial intelligence-assisted compressed sensing; PI, parallel imaging; 3D, three-dimensional; A-P, anterior-posterior; TR, repetition time; TE, echo time; TI, inversion time.
ACS image reconstruction
ACS is an imaging acceleration technology for MRI, comprising partial Fourier transform, PI, CS, and CNNs. It has garnered US Food and Drug Administration (FDA) approval, underscoring its safety and effectiveness for clinical use. The architectural design of the CNNs employed in the iteration process are adapted from the k-space, incorporating multiscale sparsification. The center point of the acquired k-space data is fully sampled, while the periphery of the k-space data involves random sampling. With the assistance of a deep learning neural network, the under-sampled k-space data can be used to generate fully sampled data (23,24). The network’s main architecture, based on U-net and detailed in Sheng et al. study (25), incorporates residual blocks with skip connections to expedite learning and employs a least squares generative adversarial approach for enhanced image reconstruction. The mathematical model assimilates components of compressed sensing, partial Fourier, and PI. The ACS neural networks in this study were trained using a dataset of two million fully sampled images, derived from both phantoms (2%) and human volunteers (98%). The ACS framework introduces a novel integration mechanism that incorporates the AI module’s output as a supplementary constraint within the compressed sensing paradigm, aligning reconstructed images with the gold standard of fully sampled data (26). This is mathematically represented by the addition of a regularization term to the reconstruction equation, aimed at minimizing the discrepancy between AI-predicted images and actual reconstructed images. The specific equation is given as follows:
where is the image being reconstructed; is the Fourier encoding matrix applied alongside a binary k-space sampling mask; is the acquired k-space data, is the sparse transform indicating a total variation; is the image reconstructed by the AI module; us the regularization parameters for each term (all regularization parameters are normalized to the same scale in the regularization calculation, in which the , , , and are the parameters that the algorithm automatically calculates); and are the L1 norm; m is the L2 norm; and PPA and PF are the parallel imaging (GRAPPA) and partial Fourier components, respectively. After integration of these items, the acceleration factor was set as the only adjustable parameter for ACS technique, representing the acceleration level.
Image evaluation
The evaluation of MRI images was conducted at the UWS-MR: R005 workstation (United Imaging Healthcare), which followed the completion of a two-step procedure for ensuring data quality and participant privacy. Initially, images were visually inspected to exclude those with severe motion artifacts that could seriously diminish the diagnostic value. Subsequently, all participant and sequence identifiers were removed from the images to maintain anonymity.
Quantitative assessment: SNR of white matter (SNRWM), SNR of gray matter (SNRGM,), CNR of white matter or gray matter (CNRWM/GM,), tumor volume, and image sharpness were calculated quantitatively. Regions of interest (ROIs) with the same size and location near the precentral and postcentral gyrus were chosen in the images from the two specialized FLAIR sequences in the same patient for signal intensity (SI) measurements of the gray matter (GM) and the adjacent white matter (WM), with areas of edema being avoided. The ROIs were primarily from the left side of the brain, and the corresponding right side was used if the lesions covered the ROIs in the left side. The formulas used to calculate the SNR and CNR were as follows (27):
where is the mean SI of WM or GM, and is the variance of background.
The SNR and CNR calculations were performed twice and under the guidance of two neuroradiologists to ensure accuracy. The average of these values was then used for further analysis. Image sharpness was calculated by measuring the proportion of active signals in the frequency measurement of the image (28), which was performed on MATLAB R2016b (MathWorks, Natick, MA, USA). The tumor volume was calculated through sketching the tumor profile slice by slice on ITK-SNAP software (29) by two radiologists working independently, and the mean value was used for analysis.
Qualitative assessment: qualitative assessment was independently conducted by three board-certified neuroradiologists with more than 10 years’ experience in neuroimaging who examined the original sagittal FALIR images. All the images were anonymized, and the neuroradiologist were not aware that there were two types of acceleration techniques for 3D FLAIR.
The overall image quality was rated according to the following 4-point scale (27): 0, indistinguishable observation with severe artifacts; 1, distinguishable observation with moderate artifacts; 2, good observation with mild artifacts; and 3, excellent observation without obvious artifacts. GM-WM border sharpness was assessed with the following 4-point scale (25,30): 0, indistinguishable GM-WM border; 1, GM-WM border with poor sharpness; 2, distinguishable GM-WM border with acceptable sharpness; and 3, clear GM-WM border with well-defined sharpness. Diagnostic confidence in detecting diseased lesions was evaluated with the following 4-point scale (31): 0, no diagnostic value; 1, blurry observation of the lesions with poor diagnostic value; 2, moderate observation of the lesions with a degree of diagnostic value; and 3, clear observation of the lesions with good diagnostic value.
Statistical analysis
Statistical analyses were carried out using SPSS 26.0 (IBM Corp., Armonk, NY, USA). The Shapiro-Wilks test was used to assess the normality of the data. Differences between PI-3D T2 FLAIR and ACS-3D T2 FLAIR were evaluated using the Wilcoxon signed rank test. A sample size of 102 was used for the quantitative metrics and image ratings, a sample size of 21 was used for the tumor volume, and a sample size of 88 was used to determine the diagnostic confidence in detection of diseased lesions. Interreader agreement was measured with the Kendall coefficient (W). The intraclass correlation coefficient (ICC) was used to test the consistency of tumor volume between the ACS and PI images. W and ICC were interpreted according to the following scheme (24): ≤0.20, poor; 0.21–0.40, fair; 0.41–0.60, moderate; 0.61–0.80, good; and 0.81–1.00, excellent. A two-sided P value <0.05 was considered to indicate statistical significance.
Results
Participant cohort
From the initial group of 111 participants, 9 were excluded due to severe motion artifacts in images (8 from PI-3D T2 FLAIR and 1 from ACS-3D T2 FLAIR), leaving 102 participants (49 males and 53 females; mean age 49.2±16.8 years; age range, 14–82 years) for analysis. The cohort included 14 healthy controls, 21 participants with space-occupying lesions (preoperative), 25 postoperative participants, and 42 patients with cerebrovascular diseases (Figure 1). The final diagnosis was based on all images and other available clinical data of the participants.
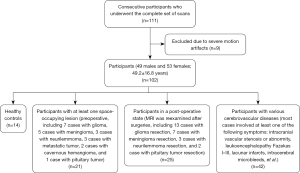
Scan time reduction
The ACS-accelerated 3D T2 FLAIR showed a reduced acquisition time of just 105 seconds. In comparison, the conventional 3D T2 FLAIR acceleration under PI required 320 seconds. This improvement translates to a reduction in the FLAIR acquisition time of 67.2%, as illustrated in Table 1.
Quantitative assessment
Quantitative data for SNR, CNR, and sharpness are summarized as the mean and standard deviation (SD) in Figure 2 and Table 2. ACS-3D T2 FLAIR, compared to PI-3D T2 FLAIR, demonstrated a higher mean SNRWM (P<0.001), SNRGM (P=0.049), CNRWM/GM (P<0.001), and sharpness (P<0.001). A good agreement on tumor volume was achieved between ACS-3D T2 FLAIR and PI-3D T2 FLAIR images (ICC =0.999; 0.998–1.000; P<0.001).
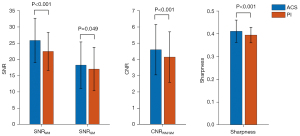
Table 2
ACS | PI | P | |
---|---|---|---|
SNRWM | 25.922±6.811 | 22.544±5.853 | <0.001 |
SNRGM | 18.324±7.137 | 17.102±6.659 | 0.049 |
CNRWM/GM | 4.613±1.547 | 4.160±1.552 | <0.001 |
Sharpness | 0.413±0.049 | 0.396±0.034 | <0.001 |
Data are presented as mean ± standard deviation. SNR, signal-to-noise ratio; CNR, contrast-to-noise ratio; T2 FLAIR, T2 fluid-attenuated inversion recovery; PI, parallel imaging; ACS, artificial intelligence-assisted compressed sensing; WM, white matter; GM, gray matter.
Qualitative assessment
Image quality ratings (interquartile range) are presented in Table 3. No significant differences between the three readers were found for the overall image quality ratings (P=0.063) or GM-WM border sharpness ratings (P=0.125). Images acquired with ACS showed almost the equivalent ratings to those of PI-acquired images in terms of diagnostic confidence in diseased lesion detection for all readers (P>0.05).
Table 3
Subjective evaluation | Comparison between PI and ACS | Interreader agreement | ||||||||
---|---|---|---|---|---|---|---|---|---|---|
PI | ACS | |||||||||
Reader | PI | ACS | P | W | P | W | P | |||
Overall image quality | 1 | 3 [3–3] | 3 [3–3] | 0.063 | 0.61 | <0.001* | >0.99 | <0.001* | ||
2 | 3 [3–3] | 3 [3–3] | 0.063 | |||||||
3 | 3 [3–3] | 3 [3–3] | 0.063 | |||||||
Sharpness | 1 | 3 [3–3] | 3 [3–3] | 0.125 | 0.54 | <0.001* | >0.99 | <0.001* | ||
2 | 3 [3–3] | 3 [3–3] | 0.125 | |||||||
3 | 3 [3–3] | 3 [3–3] | 0.125 | |||||||
Diagnosis | 1 | 3 [3–3] | 3 [3–3] | 0.500 | 0.43 | <0.001* | >0.99 | <0.001* | ||
2 | 3 [3–3] | 3 [3–3] | 0.250 | |||||||
3 | 3 [3–3] | 3 [3–3] | 0.125 |
Data are presented as the median [lower quartile–upper quartile]. Interreader agreement for 3D T2 FLAIR image was evaluated via the W. *, P with significance. ACS, artificial intelligence-assisted compressed sensing; 3D T2 FLAIR, three-dimensional T2 fluid-attenuated inversion recovery; PI, parallel imaging; W, Kendall coefficient.
For PI-3D T2 FLAIR, good interreader agreement was found for the overall image quality (W=0.61; P<0.001), GM-WM border sharpness (W=0.54; P<0.001), and diagnostic confidence in diseased lesion detection (W =0.43; P<0.001). Meanwhile, the interreader agreement for ACS-3D T2 FLAIR was good to excellent (W>0.99; P<0.001). The difference between the ACS-3D T2 FLAIR and PI-3D T2 FLAIR images for cases involving metastatic tumor, meningioma, and neurilemmoma are illustrated in Figures 3-5. Representative images (from a 67-year-old female patient with) from the original sagittal direction and the reconstructed directions (transverse and coronal) are provided in Figure S1.
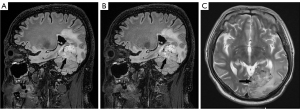
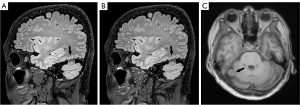
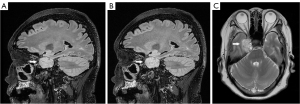
Discussion
In this study, we investigated the effectiveness of the ACS technique in acquiring brain 3D T2 FLAIR images through comparison with the images derived using the PI technique. Our study population consisted of 102 patients including healthy controls, individuals with space-occupying lesions (preoperative), postoperative cases, and those with various cerebrovascular diseases. Notably, the ACS method demonstrated a remarkable reduction in the acquisition time required for brain 3D T2 FLAIR images, requiring only 105 seconds compared to the 320 seconds of PI. Moreover, this reduction in scan time did not compromise the image quality as indicated by both quantitative and qualitative measures.
Isotropic 3D T2 FLAIR has been well-established as a fundamental sequence for the comprehensive evaluation of various intracranial diseases, such as subarachnoid hemorrhage, meningitis, acute infarction, hippocampal sclerosis, brain tumors, and multiple sclerosis (10,32-34). However, one of the primary challenges associated with isotropic 3D T2 FLAIR is its long acquisition time. Patients with brain diseases often struggle to tolerate such prolonged scanning sessions, and their involuntary head movements during the process frequently result in noticeable motion artifacts that can significantly reduce the diagnostic quality of the images (35,36). Previous research efforts have explored various methods to accelerate the acquisition of 3D T2 FLAIR, such as the PI or CS technique, achieving scan times ranging from 230 to 357 seconds (4,11,13,14). However, there has been limited discussion and progress in achieving a 3D T2 FLAIR acquisition time of under 120 seconds while maintaining good image quality. Notably, in this study, a significant reduction in the total acquisition time for 3D T2 FLAIR images (105 seconds) was achieved via the ACS technique.
For the qualitative assessment of 3D T2 FLAIR, noteworthy improvements on the mean SNRWM and SNRGM values when employing ACS as compared to the PI technique were observed. These could be attributed to the iterative calculations and deep-learning reconstructions integrated into the ACS technique, and this finding aligned with prior studies on FLAIR imaging of the brain (19), shoulder (37), lumbar spine (38), kidney (30), and liver (25). Moreover, a significant improvement of CNRWM/GM for ACS-3D T2 FLAIR compared to that of PI-3D T2 FLAIR indicated another advantage of ACS that can facilitate accurate diagnosis of intracranial diseases: distinguishing WM from GM. This improved CNR can be explained by the denoising algorithms integrated within the ACS technique, which provide better-defined image boundaries. The improved sharpness of the ACS-3D T2 FLAIR images and the consistent ICC results indicate that ACS has potential provide better image quality and equivalent tumor imaging as compared to PI. In the qualitative assessment, ACS-3D T2 FLAIR and PI-3D T2 FLAIR demonstrated similar results in terms of the overall image quality, GM-WM border sharpness, and diagnostic confidence in diseased lesion detection. Remarkably, all the highest scores (either 2 or 3) given by the readers were for ACS, whereas the few low scores (either 1 or 2) were given for PI-3D T2 FLAIR. The reduced scores in the PI-3D T2 FLAIR assessments were primarily attributed to the mild motion artifacts. It is worth noting that the shortening of acquisition through use of fast imaging techniques has the potential to reduce the probability of motion artifacts in routine clinical MRI examinations. Furthermore, the ACS-3D T2 FLAIR and PI-3D T2 FLAIR images for cases involving metastatic tumor, meningioma, and neurilemmoma showed no major differences in the visualization of health tissues and lesions (as shown in Figures 3-5). Additionally, the deformation and artifacts reported in previous studies when accelerating 2D FLAIR with a higher ACS acceleration factor were not encountered when ACS-3D T2 FLAIR was used (19). Our qualitative and quantitative assessments collectively indicate the capacity of ACS to maintain good image quality while substantially reducing acquisition time.
It should be noted, however, that overly aggressive ACS may lead to an undesirable oversmoothing of images, potentially resulting in lesions being inadequately visualized or missed entirely (39). This phenomenon has been observed in accelerating FLAIR (19) but was not encountered in our study. In methods involving deep learning, a major challenge is optimizing the loss function. Under the deep learning reconstruction methods developed thus far, the resulting images occasionally appear oversmoothed, rendering such images easily distinguishable from those reconstructed conventionally (19,39). Nonetheless, the potential impact on diagnosis should be explored further in future investigations, especially when different ACS acceleration factors are used.
There were several limitations to this study which should be addressed. First, the data for this study were sourced from a single center, and the sample size, especially for abnormal cases, was relatively small. Second, lesion size and other morphological characteristics were not considered in the analysis, and the heterogeneity of the various disease groups might hamper the comprehensive analysis of the images. Third different ACS acceleration factors were not considered but should be explored to optimize the potential clinical applications; moreover, the influence of different sequence parameters on ACS remain to be examined. Fourth, different parameters for 3D FLAIR that can influence image quality were not discussed. For example, 1.1×1.1×1.1 mm3 was adopted for the 3D FLAIR in this study, and higher resolutions, such 1×1×1 mm3 [which was preferred in some clinical trials (22,40)], 0.8×0.8×0.8 mm3, or higher, will be assessed in future work. Moreover, repetition time of 5,000 ms was used for the FLAIR sequences, whether a different repetition time, such as the recommended 6,000 ms for a standardized brain tumor imaging protocol (21), had influence on ACS would be explored in future work. Fifth, we only examined a single location near the precentral and postcentral gyrus, and a quantitative comparison of various locations would provide a more comprehensive evaluation. Additionally, the SI, SNR, and CNR of the intracranial lesions were not measured or calculated in this study, and due to the inherent denoising processing within CS, image quality evaluation based on SNR and CNR might be controversial (15,16). Future efforts should be focused on other quantitative assessments, such as segmentations of the WM, GM, and cerebrospinal fluid, as well as the determination of lesion volume and the line profiles crossing the tumors. Finally, the qualitative assessment in this study was rated visually on 4-point scales, which only allowed for a coarse image quality assessment compared to visual analogue scales or 10-point scales.
Conclusions
Our study demonstrated that brain 3D T2 FLAIR using ACS significantly reduced scanning time while maintaining image quality. ACS is valuable for examining patients with space-occupying lesions, those who have undergone operation, and those with cerebrovascular diseases who would benefit from a faster and a more tolerable 3D T2 FLAIR method.
Acknowledgments
Funding: This work was supported by
Footnote
Reporting Checklist: The authors have completed the STROBE reporting checklist. Available at https://qims.amegroups.com/article/view/10.21037/qims-24-722/rc
Conflicts of Interest: All authors have completed the ICMJE uniform disclosure form (available at https://qims.amegroups.com/article/view/10.21037/qims-24-722/coif). The authors have no conflicts of interest to declare.
Ethical Statement: The authors are accountable for all aspects of the work in ensuring that questions related to the accuracy or integrity of any part of the work are appropriately investigated and resolved. This study was conducted in accordance with the Declaration of Helsinki (as revised in 2013) and was approved by the Ethics Committee of Beijing Tiantan Hospital (No. KY2022-078-02). Written informed consent was obtained from all participants.
Open Access Statement: This is an Open Access article distributed in accordance with the Creative Commons Attribution-NonCommercial-NoDerivs 4.0 International License (CC BY-NC-ND 4.0), which permits the non-commercial replication and distribution of the article with the strict proviso that no changes or edits are made and the original work is properly cited (including links to both the formal publication through the relevant DOI and the license). See: https://creativecommons.org/licenses/by-nc-nd/4.0/.
References
- Dong X, Nao J. Fluid-attenuated inversion recovery vascular hyperintensities in anterior circulation acute ischemic stroke: associations with cortical brain infarct volume and 90-day prognosis. Neurol Sci 2019;40:1675-82. [Crossref] [PubMed]
- Lu M, Fu ZH, He XJ, Lu JK, Deng XQ, Lin DL, Gu YM, Fan YF, Lai MY, Li J, Yang MM, Chen ZP. T2 Fluid-Attenuated Inversion Recovery Resection for Glioblastoma Involving Eloquent Brain Areas Facilitated Through Awake Craniotomy and Clinical Outcome. World Neurosurg 2020;135:e738-47. [Crossref] [PubMed]
- Nistorec L, Renard D, Parvu T. Perivascular and Subarachnoid Fluid-Attenuated Inversion Recovery Hyperintensities Related to Delayed Gadolinium Leakage After Stroke. Neurology 2021;97:1000-1. [Crossref] [PubMed]
- Kakeda S, Korogi Y, Hiai Y, Ohnari N, Sato T, Hirai T. Pitfalls of 3D FLAIR brain imaging: a prospective comparison with 2D FLAIR. Acad Radiol 2012;19:1225-32. [Crossref] [PubMed]
- Paniagua Bravo Á, Sánchez Hernández JJ, Ibáñez Sanz L, Alba de Cáceres I, Crespo San José JL, García-Castaño Gandariaga B. A comparative MRI study for white matter hyperintensities detection: 2D-FLAIR, FSE PD 2D, 3D-FLAIR and FLAIR MIP. Br J Radiol 2014;87:20130360. [Crossref] [PubMed]
- Patzig M, Burke M, Brückmann H, Fesl G. Comparison of 3D cube FLAIR with 2D FLAIR for multiple sclerosis imaging at 3 Tesla. Rofo 2014;186:484-8. [PubMed]
- Wang KY, Uribe TA, Lincoln CM. Comparing lesion detection of infratentorial multiple sclerosis lesions between T2-weighted spin-echo, 2D-FLAIR, and 3D-FLAIR sequences. Clin Imaging 2018;51:229-34. [Crossref] [PubMed]
- Chagla GH, Busse RF, Sydnor R, Rowley HA, Turski PA. Three-dimensional fluid attenuated inversion recovery imaging with isotropic resolution and nonselective adiabatic inversion provides improved three-dimensional visualization and cerebrospinal fluid suppression compared to two-dimensional flair at 3 tesla. Invest Radiol 2008;43:547-51. [Crossref] [PubMed]
- Moraal B, Roosendaal SD, Pouwels PJ, Vrenken H, van Schijndel RA, Meier DS, Guttmann CR, Geurts JJ, Barkhof F. Multi-contrast, isotropic, single-slab 3D MR imaging in multiple sclerosis. Eur Radiol 2008;18:2311-20. [Crossref] [PubMed]
- Ngamsombat C, Gonçalves Filho ALM, Longo MGF, Cauley SF, Setsompop K, Kirsch JE, Tian Q, Fan Q, Polak D, Liu W, Lo WC, Gilberto González R, Schaefer PW, Rapalino O, Conklin J, Huang SY. Evaluation of Ultrafast Wave-Controlled Aliasing in Parallel Imaging 3D-FLAIR in the Visualization and Volumetric Estimation of Cerebral White Matter Lesions. AJNR Am J Neuroradiol 2021;42:1584-90. [Crossref] [PubMed]
- Crop F, Guillaud O, Ben Haj Amor M, Gaignierre A, Barre C, Fayard C, Vandendorpe B, Lodyga K, Mouttet-Audouard R, Mirabel X. Comparison of compressed sensing and controlled aliasing in parallel imaging acceleration for 3D magnetic resonance imaging for radiotherapy preparation. Phys Imaging Radiat Oncol 2022;23:44-7. [Crossref] [PubMed]
- Molnar U, Nikolov J, Nikolić O, Boban N, Subašić V, Till V. Diagnostic quality assessment of compressed SENSE accelerated magnetic resonance images in standard neuroimaging protocol: Choosing the right acceleration. Phys Med 2021;88:158-66. [Crossref] [PubMed]
- Mönch S, Sollmann N, Hock A, Zimmer C, Kirschke JS, Hedderich DM. Magnetic Resonance Imaging of the Brain Using Compressed Sensing - Quality Assessment in Daily Clinical Routine. Clin Neuroradiol 2020;30:279-86. [Crossref] [PubMed]
- Vranic JE, Cross NM, Wang Y, Hippe DS, de Weerdt E, Mossa-Basha M. Compressed Sensing-Sensitivity Encoding (CS-SENSE) Accelerated Brain Imaging: Reduced Scan Time without Reduced Image Quality. AJNR Am J Neuroradiol 2019;40:92-8. [Crossref] [PubMed]
- Sundermann B, Billebaut B, Bauer J, Iacoban CG, Alykova O, Schülke C, Gerdes M, Kugel H, Neduvakkattu S, Bösenberg H, Mathys C. Practical Aspects of novel MRI Techniques in Neuroradiology: Part 1-3D Acquisitions, Dixon Techniques and Artefact Reduction. Rofo 2022;194:1100-8. [Crossref] [PubMed]
- Sundermann B, Billebaut B, Bauer J, Iacoban CG, Alykova O, Schülke C, Gerdes M, Kugel H, Neduvakkattu S, Bösenberg H, Mathys C. Practical Aspects of novel MRI Techniques in Neuroradiology: Part 2 - Acceleration Methods and Implications for Individual Regions. Rofo 2022;194:1195-203. [Crossref] [PubMed]
- Yan X, Ran L, Zou L, Luo Y, Yang Z, Zhang S, Zhang S, Xu J, Huang L, Xia L. Dark blood T2-weighted imaging of the human heart with AI-assisted compressed sensing: a patient cohort study. Quant Imaging Med Surg 2023;13:1699-710. [Crossref] [PubMed]
- Lin DJ, Johnson PM, Knoll F, Lui YW. Artificial Intelligence for MR Image Reconstruction: An Overview for Clinicians. J Magn Reson Imaging 2021;53:1015-28. [Crossref] [PubMed]
- Hou Y, Liu Q, Chen J, Wu B, Zeng F, Yang Z, Song H, Liu Y. Application value of T2 fluid-attenuated inversion recovery sequence based on deep learning in static lacunar infarction. Acta Radiol 2023;64:1650-8. [Crossref] [PubMed]
- Zegers CML, Posch J, Traverso A, Eekers D, Postma AA, Backes W, Dekker A, van Elmpt W. Current applications of deep-learning in neuro-oncological MRI. Phys Med 2021;83:161-73. [Crossref] [PubMed]
- Ellingson BM, Bendszus M, Boxerman J, Barboriak D, Erickson BJ, Smits M, et al. Consensus recommendations for a standardized Brain Tumor Imaging Protocol in clinical trials. Neuro Oncol 2015;17:1188-98. [PubMed]
- Wattjes MP, Ciccarelli O, Reich DS, Banwell B, de Stefano N, Enzinger C, et al. 2021 MAGNIMS-CMSC-NAIMS consensus recommendations on the use of MRI in patients with multiple sclerosis. Lancet Neurol 2021;20:653-70. [Crossref] [PubMed]
- Wang S, Cao G, Wang Y, Liao S, Wang Q, Shi J, Li C, Shen D. Review and Prospect: Artificial Intelligence in Advanced Medical Imaging. Front Radiol 2021;1:781868. [Crossref] [PubMed]
- Liu K, Xi B, Sun H, Wang J, Chen C, Wen X, Zhang Y, Zeng M. The clinical feasibility of artificial intelligence-assisted compressed sensing single-shot fluid-attenuated inversion recovery (ACS-SS-FLAIR) for evaluation of uncooperative patients with brain diseases: comparison with the conventional T2-FLAIR with parallel imaging. Acta Radiol 2023;64:1943-9. [Crossref] [PubMed]
- Sheng RF, Zheng LY, Jin KP, Sun W, Liao S, Zeng MS, Dai YM. Single-breath-hold T2WI liver MRI with deep learning-based reconstruction: A clinical feasibility study in comparison to conventional multi-breath-hold T2WI liver MRI. Magn Reson Imaging 2021;81:75-81. [Crossref] [PubMed]
- Zhai R, Huang X, Zhao Y, Ji M, Lv X, Qian M, Liao S, Li G. Intelligent Incorporation of AI with Model Constraints for MRI Acceleration. Proceedings of the 29th Annual Meeting of ISMRM [Virtual] 2021.
- Ding J, Duan Y, Zhuo Z, Yuan Y, Zhang G, Song Q, Gao B, Zhang B, Wang M, Yang L, Hou Y, Yuan J, Feng C, Wang J, Lin L, Liu Y. Acceleration of Brain TOF-MRA with Compressed Sensitivity Encoding: A Multicenter Clinical Study. AJNR Am J Neuroradiol 2021;42:1208-15. [Crossref] [PubMed]
- De K, Masilamani V. Image Sharpness Measure for Blurred Images in Frequency Domain. Procedia Engineering 2013;64:149-58. [Crossref]
- Yushkevich PA, Piven J, Hazlett HC, Smith RG, Ho S, Gee JC, Gerig G. User-guided 3D active contour segmentation of anatomical structures: significantly improved efficiency and reliability. Neuroimage 2006;31:1116-28. [Crossref] [PubMed]
- Zhao Y, Peng C, Wang S, Liang X, Meng X. The feasibility investigation of AI -assisted compressed sensing in kidney MR imaging: an ultra-fast T2WI imaging technology. BMC Med Imaging 2022;22:119. [Crossref] [PubMed]
- Herrmann J, Gassenmaier S, Nickel D, Arberet S, Afat S, Lingg A, Kündel M, Othman AE. Diagnostic Confidence and Feasibility of a Deep Learning Accelerated HASTE Sequence of the Abdomen in a Single Breath-Hold. Invest Radiol 2021;56:313-9. [Crossref] [PubMed]
- Heo YJ, Baek HJ, Cho E, Lee K. 3D Synthetic Brain MRI with Compressed Sensing: Can It Be a Promising Way Forward for Daily Neuroimaging? Curr Med Imaging 2023; Epub ahead of print. [Crossref] [PubMed]
- Lecler A, Bouzad C, Deschamps R, Maizeroi F, Sadik JC, Gueguen A, Gout O, Picard H, Savatovsky J. Optimizing 3D FLAIR to detect MS lesions: pushing past factory settings for precise results. J Neurol 2019;266:2786-95. [Crossref] [PubMed]
- Umino M, Maeda M, Kogue R, Nakamura S, Ii Y, Tomimoto H, Sakuma H. Evaluation of cortical superficial siderosis in patients with cognitive dysfunction using 3D FLAIR and 3D DIR. Eur Radiol 2021;31:6411-8. [Crossref] [PubMed]
- Ryu KH, Choi DS, Baek HJ, Cho SB, Ha JY, Kim TB, Hwang MJ. Clinical feasibility of 1-min ultrafast brain MRI compared with routine brain MRI using synthetic MRI: a single center pilot study. J Neurol 2019;266:431-9. [Crossref] [PubMed]
- Igata N, Kakeda S, Watanabe K, Nozaki A, Rettmann D, Narimatsu H, Ide S, Abe O, Korogi Y. Utility of real-time prospective motion correction (PROMO) for segmentation of cerebral cortex on 3D T1-weighted imaging: Voxel-based morphometry analysis for uncooperative patients. Eur Radiol 2017;27:3554-62. [Crossref] [PubMed]
- Liu J, Li W, Li Z, Yang J, Wang K, Cao X, Qin N, Xue K, Dai Y, Wu P, Qiu J. Magnetic resonance shoulder imaging using deep learning-based algorithm. Eur Radiol 2023;33:4864-74. [Crossref] [PubMed]
- Sui H, Gong Y, Liu L, Lv Z, Zhang Y, Dai Y, Mo Z. Comparison of Artificial Intelligence-Assisted Compressed Sensing (ACS) and Routine Two-Dimensional Sequences on Lumbar Spine Imaging. J Pain Res 2023;16:257-67. [Crossref] [PubMed]
- Quan TM, Nguyen-Duc T, Jeong WK. Compressed Sensing MRI Reconstruction Using a Generative Adversarial Network With a Cyclic Loss. IEEE Trans Med Imaging 2018;37:1488-97. [Crossref] [PubMed]
- Kaufmann TJ, Smits M, Boxerman J, Huang R, Barboriak DP, Weller M, Chung C, Tsien C, Brown PD, Shankar L, Galanis E, Gerstner E, van den Bent MJ, Burns TC, Parney IF, Dunn G, Brastianos PK, Lin NU, Wen PY, Ellingson BM. Consensus recommendations for a standardized brain tumor imaging protocol for clinical trials in brain metastases. Neuro Oncol 2020;22:757-72. [Crossref] [PubMed]