Diagnostic value of an interpretable machine learning model based on clinical ultrasound features for follicular thyroid carcinoma
Introduction
Thyroid follicular tumors, including malignant follicular thyroid carcinoma (FTC) and benign follicular thyroid adenoma (FTA), originate from thyroid follicular epithelial cells. FTC is the second most common subtype of thyroid cancer after papillary thyroid cancer, accounting for 10–15% of all thyroid cancers (1). Moreover, patients with FTC have a higher risk of developing lung and bone metastases (two- and 10-fold higher, respectively) than those with papillary thyroid cancer (2,3). The only criteria for distinguishing between benign and malignant thyroid follicular tumors are tumor capsular invasion and/or vascular invasion in surgical specimens. However, FTA and FTC have been shown to have overlapping clinical, ultrasound, and molecular biological features (4). Further, the current ultrasound risk stratification systems for thyroid nodules exhibit poor diagnostic performance for thyroid follicular tumors (5). For example, most ultrasound signs of FTC do not have or possess only one or two malignant features. Additionally, FTCs are easily misdiagnosed as benign tumors, such as FTA and nodular goiter, due to the low Thyroid Imaging Reporting and Data System (TI-RADS) scores (6,7). Similarly, thyroid puncture biopsy cannot accurately confirm a diagnosis of FTC, and the corresponding characteristic tumor markers of FTC are yet to be identified. All these challenges make the early diagnosis of FTC challenging. Improving the preoperative diagnosis rate and reducing the misdiagnosis rate of FTC can significantly enhance the prognosis and quality of life of the patients and greatly contribute to the clinical management of this disease.
Machine learning (ML), a branch of artificial intelligence, encompasses two primary types of actions: classification and regression. In classification tasks, ML algorithms categorize data into predefined classes based on patterns identified in the training data. Meanwhile, in regression tasks, ML algorithms predict continuous numerical values by establishing relationships between input features and output variables. In the medical field, ML can be applied to build models via data sets obtained from various sources (e.g., clinical data, laboratory results, and medical images) for the identification, diagnosis, treatment, and prognosis prediction of diseases (8,9). Therefore, ML has been increasingly employed as a non-invasive method in radiology to reveal medical imaging-based tumor features (10,11). Furthermore, various ML algorithms have been utilized to construct classifier models for thyroid ultrasound imaging (12-14). To date, only a few studies have used ML to differentiate the benign and malignant nature of thyroid follicular tumors according to preoperative clinical parameters and ultrasound features (15). Existing models are based on radiomics, which have poor biological interpretability, and the black box problem in ML remains unresolved. Hence, in this study, we investigated the use of ML model to distinguish between benign and malignant thyroid follicular tumors based on preoperative clinical parameters and ultrasound imaging features. We interpreted the model using SHapley Additive ExPlanations (SHAP). We present this article in accordance with the TRIPOD-AI reporting checklist (available at https://qims.amegroups.com/article/view/10.21037/qims-24-601/rc).
Methods
Research patients
From January 2009 to February 2023, a total of 562 patients diagnosed with FTA or FTC based on their surgical pathology were consecutively included from Zhejiang Provincial Cancer Hospital for the training set, and 218 patients were consecutively included from Zhejiang Provincial People’s Hospital for the external validation set. The study was conducted in accordance with the Declaration of Helsinki (as revised in 2013). The study design and protocol were approved by the Ethics Committees of Zhejiang Cancer Hospital (No. IRB-2020-287) and Zhejiang Provincial People’s Hospital (No. QT-2024-023). Individual consent for this retrospective analysis was waived.
The patient inclusion criteria were as follows: (I) thyroid ultrasonography in the hospital 2 weeks before surgery and (II) no other treatment modality before surgery. The patients were excluded if they met any of the following exclusion criteria: (I) poor or missing ultrasound images or other incomplete clinical data or (II) the location of thyroid lesions on preoperative ultrasound examination did not coincide with the location of postoperative surgical gross pathology.
Clinical information
Ten clinical indicators (including age and gender) and eight thyroid serological indicators [i.e., thyroid-stimulating hormone (TSH), free triiodothyronine (FT3), free tetraiodothyronine (FT4), triiodothyronine (T3), thyroxine (T4), thyroglobulin antibody (TGAb), thyroid peroxidase antibody (TPOAb), and thyroglobulin (TG)], were included in this study.
Ultrasound features
Ultrasonography was performed using Esaote MyLab90, Logiq E9, Philips iU22, and Toshiba 790A ultrasound systems equipped with LA523, ML6-15, L12-5, and PLT-805AT linear array probes, respectively, at a frequency range of 5–13 MHz. In this procedure, the patients were placed in a supine position (without a pillow) to fully expose the neck region. Next, the probes were placed on the thyroid and neck regions for multisection scanning. Further, two senior ultrasonographers recorded the parameters of all nodule ultrasound features with reference to the thyroid TI-RADS grading criteria published by the American College of Radiology in 2017, without knowledge of the postoperative pathology of the thyroid nodules. These parameters comprised the thyroid nodule size (maximum tumor diameter), location (left lobe, right lobe, or isthmus), composition (cystic, mixed cystic-solid, or solid), internal echogenicity (hypo- or isoechoic, hypoechoic, or extremely hypoechoic), echogenicity texture (homogeneous or inhomogeneous), margins (smooth or ill-defined, lobular or irregular, or extra-thyroidal invasion), calcifications (no or large comet tail, gross calcification, peripheral cyclic calcification, or microcalcification), and an acoustic halo of >2 mm (yes or no). In the case of inconsistency in interpreting the images, a third senior sonographer was consulted to confirm the diagnosis.
Feature screening and the development, validation, and evaluation of the predictive model
The 10 clinical and eight ultrasound parameters were screened using least absolute shrinkage and selection operator (LASSO) and multivariate logistic regression. Seven ML methods, including logistic regression model (LM), Light Gradient Boosting Machine (LightGBM), k-nearest neighbor (KNN), support vector machine (SVM), eXtreme Gradient Boosting (XGBoost), random forest (RF), and decision tree (DT), were employed for comprehensive analysis based on the training set data. We used 5-fold cross-validation, repeated five times to compare the competing models. Subsequently, the area under the receiver operating characteristic (ROC) curve (AUC), accuracy, sensitivity, specificity, positive predicted value (PPV), negative predicted value (NPV), precision, recall, and comprehensive evaluation index (F-score) were calculated to compare the diagnostic efficacy among the seven models. The model with the highest AUC in the validation set was chosen as the optimal model. Additionally, the optimal model was evaluated using five-fold cross-validation to determine the predictive ability of the model and ensure its stability. Next, model discrimination was quantified via ROC, calibration, and decision curve analysis (DCA) curve analyses, and the model’s predictive performance was evaluated using the obtained AUC. Finally, feature importance was examined by applying the SHAP method. SHAP based on cooperative game theory, has global and local interpretability, interpreting the predicted value of the model as the sum of the contribution values of each input feature, that is, the shapley value. Compared with other explanation methods in previous literature, SHAP can visualize the prediction process of complex ML prediction models. Figure 1 illustrates the flowchart in the present study.
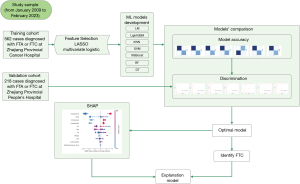
Statistical analysis
All statistical analyses were performed using the Python (version 3.9.19), Scikit-learn (version 1.4.2), XGBoost (version 2.0.3), LightGBM (version 4.3.0) and Shap (version 0.42.1). Data that were normally distributed according to the Kolmogorov–Smirnov normality test were expressed as mean ± standard deviation, and between-group comparisons were conducted using Student’s t-test or the Mann-Whitney U test. Non-normally distributed data were presented as median (lower quartile, upper quartile), while between-group comparisons were performed utilizing the rank-sum test. Categorical variables were described as the number of patients (rate), with between-group comparisons conducted via the Chi-squared test. Statistical significance was set at P<0.05.
Results
Clinical characteristics of patients and baseline ultrasound parameters of the thyroid nodules
A total of 780 patients were included in this study, including 562 in the training set (362 with benign nodules and 200 with malignant nodules) and 218 in the validation set (161 with benign nodules and 57 with malignant nodules). The patients had a mean age of 52.5 [40.0, 61.0] years and a mean nodule size of 30.0 [18.0, 40.0] mm. The baseline comparisons of the clinical characteristics (e.g., gender, age, and serological data) and ultrasonographic features of the patients in the training and validation sets are provided in Table 1.
Table 1
Characteristic | Total patients (N=780) | Training cohort (N=562) | Validation cohort (N=218) | P value |
---|---|---|---|---|
Sex | 0.635 | |||
Men | 208 (26.7) | 153 (27.2) | 55 (25.2) | |
Women | 572 (73.3) | 409 (72.8) | 163 (74.8) | |
Age (years) | 52.5 [40.0, 61.0] | 53.0 [41.0, 61.0] | 52.0 [37.0, 62.0] | 0.602 |
Diameter (mm) | 30.0 [18.0, 40.0] | 28.0 [18.0, 40.0] | 32.0 [19.2, 43.0] | 0.076 |
Nodule location | 0.350 | |||
Left lobe of the thyroid gland | 364 (46.7) | 268 (47.7) | 96 (44.0) | |
Right lobe of the thyroid gland | 407 (52.2) | 289 (51.4) | 118 (54.1) | |
Thyroid-isthmus | 9 (1.15) | 5 (0.89) | 4 (1.83) | |
Composition | 0.804 | |||
Mixed solid-cystic | 272 (34.9) | 194 (34.5) | 78 (35.8) | |
Solid | 508 (65.1) | 368 (65.5) | 140 (64.2) | |
Echotexture | 0.178 | |||
Homogeneous | 270 (34.6) | 186 (33.1) | 84 (38.5) | |
Heterogeneous | 510 (65.4) | 376 (66.9) | 134 (61.5) | |
Echogenicity | 0.842 | |||
Isoechoic/hyperechoic | 502 (64.4) | 360 (64.1) | 142 (65.1) | |
Hypoechoic | 278 (35.6) | 202 (35.9) | 76 (34.9) | |
Margin | 0.481 | |||
Smooth | 687 (88.1) | 491 (87.4) | 196 (89.9) | |
Irregular/lobulated | 36 (4.62) | 26 (4.63) | 10 (4.59) | |
Extrathyroidal extension | 57 (7.31) | 45 (8.01) | 12 (5.50) | |
Calcifications | 0.075 | |||
None | 586 (75.1) | 423 (75.3) | 163 (74.8) | |
Macrocalcification | 112 (14.4) | 75 (13.3) | 37 (17.0) | |
Rim calcification | 70 (8.97) | 52 (9.25) | 18 (8.26) | |
Microcalcification | 12 (1.54) | 12 (2.14) | 0 (0.00) | |
Halo thickness >2 mm | 0.367 | |||
No | 721 (92.4) | 516 (91.8) | 205 (94.0) | |
Yes | 59 (7.56) | 46 (8.19) | 13 (5.96) | |
TGAb (U/mL) | 15.0 [15.0, 27.1] | 15.0 [15.0, 26.5] | 15.0 [15.0, 33.3] | 0.971 |
TG (ng/mL) | 61.2 [23.2, 260] | 63.8 [23.7, 272] | 53.3 [22.4, 243] | 0.438 |
TPOAb (U/mL) | 28.0 [19.3, 38.2] | 28.0 [18.3, 38.0] | 28.0 [28.0, 38.6] | 0.244 |
TSH (μIU/mL) | 1.43 [0.92, 2.17] | 1.40 [0.92, 2.15] | 1.51 [0.93, 2.20] | 0.431 |
T4 (μg/dL) | 8.10 [7.09, 9.25] | 8.10 [7.00, 9.30] | 8.12 [7.10, 9.19] | 0.844 |
FT4 (ng/dL) | 1.19 [1.07, 1.32] | 1.20 [1.08, 1.33] | 1.15 [1.02, 1.30] | 0.002 |
T3 (ng/mL) | 1.11 [0.98, 1.24] | 1.11 [0.98, 1.24] | 1.11 [0.98, 1.26] | 0.735 |
FT3 (pg/mL) | 3.26 [2.98, 3.53] | 3.25 [2.98, 3.52] | 3.26 [2.98, 3.61] | 0.461 |
Numbers in brackets indicate percentages, and numbers in square brackets denote quartiles. TGAb, thyroglobulin antibody; TG, thyroglobulin; TPOAb, thyroid peroxidase antibody; TSH, thyroid-stimulating hormone; T4, thyroxine; FT4, free tetraiodothyronine; T3, triiodothyronine; FT3, free triiodothyronine.
Screening of risk factors for FTC
A total of 18 variables from the clinical parameters and ultrasound characteristics underwent LASSO regression analysis (Figure 2). After selecting lambda.min, which is the optimal regularization parameter selected through cross-validation that minimizes the mean squared error (MSE), the number of variables was reduced from 18 to 15, consisting of sex, age, composition, echotexture, echogenicity, halo thickness >2 mm, TGAb, TG, TPOAb, TSH, T4, T3, FT3, margin, and calcifications. Subsequently, the effect of confounders was controlled for by performing backward stepwise multivariate logistic regression analysis on the 15 variables. The confounders that were controlled included sex, TPOAb, T4, and FT3. Finally, 11 variables were retained, comprising age, composition, echotexture, echogenicity, halo thickness >2 mm, TGAb, TG, TSH, T3, margin, and calcifications (Table 2).
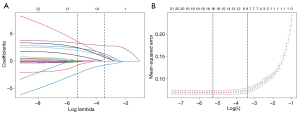
Table 2
Characteristics | B | SE | OR (95% CI) | Z | P |
---|---|---|---|---|---|
Intercept | −11.433 | 1.74771 | 1.083 (0.000–2.660) | −6.542 | 0 |
Age (years) | −0.023 | 0.01342 | 0.977 (0.951–1.002) | −1.73 | 0.084 |
Composition | 2.931 | 0.47992 | 18.74 (7.657–50.81) | 6.107 | <0.001 |
Echotexture | 2.261 | 0.45288 | 9.592 (4.073–24.32) | 4.993 | <0.001 |
Echogenicity | 3.612 | 0.40064 | 37.04 (17.51–85.03) | 9.016 | <0.001 |
Margin | 16.788 | 1,197.84102 | 19,542 (3.851–3.937) | 0.014 | 0.989 |
Calcifications | 0.808 | 0.27094 | 2.242 (1.338–3.890) | 2.981 | 0.003 |
Halo thickness >2 mm | 3.369 | 0.80714 | 29.03 (6.085–146.9) | 4.174 | <0.001 |
TGAb | 0.003 | 0.00159 | 1.002 (0.999–1.005) | 1.722 | 0.085 |
TG | 0.003 | 0.00093 | 1.003 (1.001–1.005) | 3.651 | <0.001 |
TSH | 0.09 | 0.04634 | 1.093 (1.003–1.266) | 1.936 | 0.053 |
T3 | −1.236 | 0.84061 | 0.290 (0.053–1.470) | −1.47 | 0.142 |
TGAb, thyroglobulin antibody; TG, thyroglobulin; TSH, thyroid-stimulating hormone; T3, triiodothyronine; SE, standard error; OR, odd ratio; CI, confidence interval; B, regression coefficient.
Integrated analysis of the seven ML models
Seven models, including LM, DT, RF, XGBoost, SVM, KNN, and LightGBM, were constructed and trained using the training set (562 patients). In the training set, the KNN and RF models had relatively high AUCs of 1.000 each. Moreover, eight indicators of the KNN and RF models, including precision, sensitivity, specificity, accuracy, PPV, NPV, recall, and F1 score, were all equal to 1.000. In the validation set (218 patients), the XGBoost model had the highest AUC (Figure 3) of 0.969 [95% confidence interval (CI), 0.946–0.992], along with good accuracy, sensitivity, specificity, PPV, NPV, precision, recall, and F1 score of 0.917, 0.930, 0.913, 0.791, 0.974, 0.791, 0.930, and 0.855, respectively (Table 3), among the seven models. The heatmap of the confusion matrix for XGBoost is shown in Figure 4.
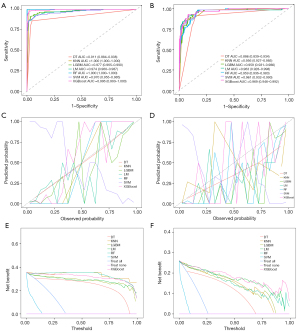
Table 3
Variables | AUC | Accuracy | Sensitivity | Specificity | PPV | NPV | Precision | Recall | F1 |
---|---|---|---|---|---|---|---|---|---|
Training cohort | |||||||||
LM | 0.974 | 0.929 | 0.925 | 0.931 | 0.881 | 0.957 | 0.881 | 0.925 | 0.902 |
LightGBM | 0.977 | 0.948 | 0.915 | 0.967 | 0.939 | 0.954 | 0.939 | 0.915 | 0.927 |
KNN | 1.000 | 1.000 | 1.000 | 1.000 | 1.000 | 1.000 | 1.000 | 1.000 | 1.000 |
SVM | 0.970 | 0.921 | 0.924 | 0.872 | 0.878 | 0.872 | 0.878 | 0.924 | 0.878 |
XGBoost | 0.996 | 0.980 | 0.980 | 0.981 | 0.966 | 0.989 | 0.966 | 0.980 | 0.973 |
RF | 1.000 | 1.000 | 1.000 | 1.000 | 1.000 | 1.000 | 1.000 | 1.000 | 1.000 |
DT | 0.911 | 0.920 | 0.910 | 0.925 | 0.860 | 0.953 | 0.860 | 0.910 | 0.884 |
Validation cohort | |||||||||
LM | 0.963 | 0.876 | 0.930 | 0.857 | 0.697 | 0.857 | 0.697 | 0.930 | 0.797 |
LightGBM | 0.959 | 0.904 | 0.860 | 0.919 | 0.790 | 0.949 | 0.790 | 0.860 | 0.824 |
KNN | 0.956 | 0.899 | 0.860 | 0.913 | 0.778 | 0.948 | 0.778 | 0.860 | 0.817 |
SVM | 0.961 | 0.882 | 0.656 | 0.871 | 0.884 | 0.716 | 0.884 | 0.656 | 0.751 |
XGBoost | 0.969 | 0.917 | 0.930 | 0.913 | 0.791 | 0.974 | 0.791 | 0.930 | 0.855 |
RF | 0.959 | 0.881 | 0.825 | 0.901 | 0.746 | 0.936 | 0.746 | 0.842 | 0.825 |
DT | 0.886 | 0.858 | 0.691 | 0.933 | 0.824 | 0.870 | 0.824 | 0.691 | 0.752 |
AUC, areas under the curve; PPV, positive predicted value; NPV, negative predicted value; F-score, comprehensive evaluation index; LM, logistic regression model; LightGBM, Light Gradient Boosting Machine; KNN, k-nearest neighbor; SVM, support vector machine; XGBoost, eXtreme Gradient Boosting; RF, random forest; DT, decision tree.
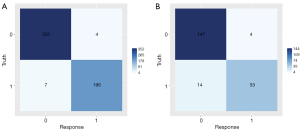
Validation and evaluation of the optimal model
In the external validation set, there was no evidence of a difference for AUC values between XGBoost and RF, LightGBM, or KNN (P=0.285, 0.363, and 0.477, respectively, DeLong test). However, there was evidence of a difference for AUC values between XGBoost and LM, DT, and SVM (P=0.014, 0.022, and <0.001, respectively, DeLong test). After comprehensive comparison of other diagnostic metrics of the models, the XGBoost model was selected as the best model for predicting FTC. Next, the robustness of the XGBoost model was examined by merging the data from the training and validation sets and subjecting them to five-fold cross-validation, resulting in an average AUC of 0.96. Finally, the calibration and DCA curves were plotted (Figure 5).
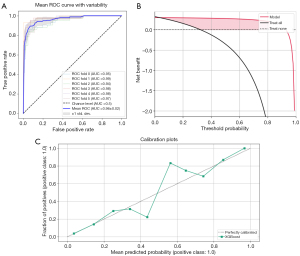
Furthermore, a SHAP summary plot of the XGBoost model was generated using the SHAP interpretability analysis method. The top 10 feature contributions were as follows: echogenicity, TGAb, echotexture, composition, T3, age, TG, margin, TSH, calcifications, and halo thickness >2 mm. On each line of feature importance, all patient attributions for the outcome are plotted with differently colored dots, wherein red dots represent high-risk values and blue dots denote low-risk values. For predicting the benign and malignant nature of the thyroid follicular tumors, a SHAP value of >0 for samples in the red distribution region indicated a positive impact on the model, whereas a SHAP value of <0 for samples in the blue distribution region signified a negative impact on the model. In the XGBoost model, echogenicity, echotexture, and composition contributed positively to the model Figure 6. An example plot of SHAP values for a single sample is depicted in. The SHAP force plots illustrate explanations for all samples (Figure 7) as well as individual samples (Figure 8).
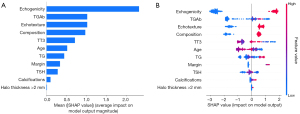
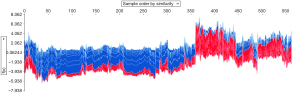
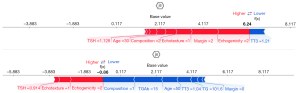
Discussion
Preoperative ultrasonography and fine-needle aspiration cytology exhibit good performance in identifying papillary thyroid carcinoma (16,17). However, these methods have limited performance in distinguishing between benign and malignant thyroid follicular tumors. The diagnosis of malignant thyroid follicular neoplasms (FNs) relies on the histological confirmation of tumor/peritumoral invasion and/or vascular invasion in surgically resected specimens. Therefore, the preoperative differential diagnosis of benign and malignant FNs remains challenging. In this study, seven ML algorithms were developed based on clinical parameters and ultrasound features. Further comparative analysis showed that the XGBoost model achieved the best performance in the validation set, and this model was selected to predict the benign and malignant nature of thyroid follicular tumors. Subsequently, automatic parameter tuning and internal cross-validation were employed to optimize the accuracy and clinical validity of the model. Additionally, the model was explained using the SHAP interpretability approach. The XGBoost prediction model found that several risk factors for malignant thyroid follicular tumors, including echogenicity, TGAb, echotexture, composition, T3, age, TG, margin, TSH, calcifications, and halo thickness >2 mm, were the most critical predictors of FTC.
Previous studies (18,19) on thyroid follicular tumors have suggested that the internal echogenicity of the nodules has diagnostic value in distinguishing benign from malignant thyroid follicular tumors. FTC is generally more hypoechoic than FTA, while FTA tends to have greater isoechoicity. A recent study (6) on the risk stratification system for thyroid follicular tumors reported that nodal hypoechoicity is associated with an increased malignancy risk in cases where thyroid fine-needle aspiration is suggestive of follicular tumors, consistent with our study findings. A multicenter Korean study (20) showed that more than 50% of follicular tumors have a solid component. Although follicular thyroid lesions may present with similar structural growth patterns, FTAs typically exhibit a normal follicular growth pattern, whereas FTCs usually display a parenchymal/trabecular growth pattern. This finding potentially explains the echogenic heterogeneity within these tumors. Further, the echogenic heterogeneity in the tumors could be attributed to necrosis or hemorrhage in FTC.
In the XGBoost prediction model, TG and TGAb levels were considered crucial features for distinguishing between benign and malignant thyroid follicular tumors. TG is a specific protein produced by the thyroid gland. However, the lack of specific serum TG values for establishing the etiological diagnosis of thyroid diseases has hindered the general application of preoperative serum TG levels as a criterion in the clinical diagnosis of thyroid cancer. Nevertheless, serum TG levels are a critical indicator for detecting residual, recurrent, or metastatic tumors in patients after surgery (21). Additionally, previous studies (22,23) have revealed that preoperative serum TG levels are significantly elevated in patients with FTC and that these levels increase dramatically according to follicular carcinoma severity. In the case of suspected follicular tumors, preoperative TG levels are highly specific in predicting thyroid cancer. Furthermore, elevated TG concentration may indicate the early tearing of the lesion capsule, which leads to TG entry into the bloodstream. TGAb is an immunoglobulin that specifically binds to TG, the target antigen of TGAb. Similar to TG levels, persistently high or escalating levels of TGAb after surgery often indicate persistent disease or a higher recurrence risk.
FTC exhibits peak incidence rates in two age groups, i.e., 45–49 and 60–70 years (24). Moreover, the median age of patients with FTC is significantly higher than that of those with FTA (18). In contrast, another study observed no significant differences in the mean age between the patients with FTC and those with FTA (19). In the current study, the mean age of the patients in the FTC group was 52.0 [37.0, 62.0] years. Based on all these findings, the significance of age in the preoperative diagnosis of follicular thyroid tumors is still debated.
The definition of margin irregularity is ambiguous in the context of thyroid nodules. Nevertheless, a prior study (25) has suggested that margin irregularity may be an indicator to differentiate FTA from FTC. Another investigation demonstrated (26) a significant difference in the tumor margins between FTA and FTC. In terms of the mechanism related to this feature, localized cancer cells repeatedly break through the thyroid capsule in FTC, which is then covered with fibrous tissue. The deeper invasion of the cancer cells into the surrounding normal tissue further leads to irregular nodular margins on the ultrasonogram.
Microcalcifications are considered a typical sign of thyroid malignancy, whereas gross calcifications or macrocalcifications are more prevalent in benign nodules. An earlier study (27) proposed that calcification on ultrasound images is an independent predictor of FTC. Conversely, other research (28) indicated that calcifications with a comet tail sign in the cystic component were predictors of benign thyroid nodules, while calcifications in the solid component were not absolute predictors of benign thyroid nodules. Therefore, calcification foci with a comet tail sign may be useful in identifying the benign or malignant nature of thyroid nodules. However, the diagnostic value of calcification in FTC requires further exploration.
An additional approach in differentiating FTA from FTC involves the detection of the “halo”. The halo or hypoechoic margin surrounding the nodule is histologically composed of the nodal capsule or pseudocapsule, fibrous connective tissue, compressed thyroid parenchyma, and chronic inflammatory infiltrate. Thus, identifying a halo via needle biopsy may be valuable in the differential diagnosis of follicular lesions (7).
There are several limitations in this study that should be considered. First, this study had a retrospective design. Second, we only included patients who had undergone total thyroidectomy with complete follow-up. Therefore, selection bias may have occurred. Finally, elastography and rheography were not included in the ultrasound characteristics.
Conclusions
In conclusion, our study developed seven ML models based on the preoperative clinical parameters and ultrasound features of patients with follicular thyroid tumors. Additionally, an optimal model was validated and explained using the SHAP interpretability method. The model showed high clinical applicability and potential to aid in the preoperative differentiation between benign and malignant thyroid follicular tumors.
Acknowledgments
Funding: This work was supported by
Footnote
Reporting Checklist: The authors have completed the TRIPOD-AI reporting checklist. Available at https://qims.amegroups.com/article/view/10.21037/qims-24-601/rc
Conflicts of Interest: All authors have completed the ICMJE uniform disclosure form (available at https://qims.amegroups.com/article/view/10.21037/qims-24-601/coif). The authors have no conflicts of interest to declare.
Ethical Statement: The authors are accountable for all aspects of the work in ensuring that questions related to the accuracy or integrity of any part of the work are appropriately investigated and resolved. The study was conducted in accordance with the Declaration of Helsinki (as revised in 2013). The study design and protocol were approved by the Ethics Committees of Zhejiang Cancer Hospital (No. IRB-2020-287) and Zhejiang Provincial People’s Hospital (No. QT-2024-023). Individual consent for this retrospective analysis was waived.
Open Access Statement: This is an Open Access article distributed in accordance with the Creative Commons Attribution-NonCommercial-NoDerivs 4.0 International License (CC BY-NC-ND 4.0), which permits the non-commercial replication and distribution of the article with the strict proviso that no changes or edits are made and the original work is properly cited (including links to both the formal publication through the relevant DOI and the license). See: https://creativecommons.org/licenses/by-nc-nd/4.0/.
References
- Dralle H, Machens A, Basa J, Fatourechi V, Franceschi S, Hay ID, Nikiforov YE, Pacini F, Pasieka JL, Sherman SI. Follicular cell-derived thyroid cancer. Nat Rev Dis Primers 2015;1:15077. [Crossref] [PubMed]
- Schubert L, Mbekwe-Yepnang AM, Wassermann J, Braik-Djellas Y, Jaffrelot L, Pani F, Deniziaut G, Lussey-Lepoutre C, Chereau N, Leenhardt L, Bernier MO, Buffet C. Clinico-pathological factors associated with radioiodine refractory differentiated thyroid carcinoma status. J Endocrinol Invest 2024;47:1573-81. [Crossref] [PubMed]
- Zhao H, Liu CH, Cao Y, Zhang LY, Zhao Y, Liu YW, Liu HF, Lin YS, Li XY. Survival prognostic factors for differentiated thyroid cancer patients with pulmonary metastases: A systematic review and meta-analysis. Front Oncol 2022;12:990154. [Crossref] [PubMed]
- Chakrabarty N, Mahajan A, Basu S, D'Cruz AK. Comprehensive Review of the Imaging Recommendations for Diagnosis, Staging, and Management of Thyroid Carcinoma. J Clin Med 2024;13:2904. [Crossref] [PubMed]
- Lin Y, Lai S, Wang P, Li J, Chen Z, Wang L, Guan H, Kuang J. Performance of current ultrasound-based malignancy risk stratification systems for thyroid nodules in patients with follicular neoplasms. Eur Radiol 2022;32:3617-30. [Crossref] [PubMed]
- Li J, Li C, Zhou X, Huang J, Yang P, Cang Y, Zhai H, Huang R, Mu Y, Gou X, Zhang Y, Yu J, Liang P. US Risk Stratification System for Follicular Thyroid Neoplasms. Radiology 2023;309:e230949. [Crossref] [PubMed]
- Kuo TC, Wu MH, Chen KY, Hsieh MS, Chen A, Chen CN. Ultrasonographic features for differentiating follicular thyroid carcinoma and follicular adenoma. Asian J Surg 2020;43:339-46. [Crossref] [PubMed]
- Alhussaini AJ, Steele JD, Jawli A, Nabi G. Radiomics Machine Learning Analysis of Clear Cell Renal Cell Carcinoma for Tumour Grade Prediction Based on Intra-Tumoural Sub-Region Heterogeneity. Cancers (Basel) 2024;16:1454. [Crossref] [PubMed]
- Chen S, Guo T, Zhang E, Wang T, Jiang G, Wu Y, Wang X, Na R, Zhang N. Machine learning-based prognosis signature for survival prediction of patients with clear cell renal cell carcinoma. Heliyon 2022;8:e10578. [Crossref] [PubMed]
- Majumder S, Katz S, Kontos D, Roshkovan L. State of the art: radiomics and radiomics-related artificial intelligence on the road to clinical translation. BJR Open 2024;6:tzad004. [Crossref] [PubMed]
- Zhu M, Yang Z, Wang M, Zhao W, Zhu Q, Shi W, Yu H, Liang Z, Chen L. A computerized tomography-based radiomic model for assessing the invasiveness of lung adenocarcinoma manifesting as ground-glass opacity nodules. Respir Res 2022;23:96. [Crossref] [PubMed]
- Feng N, Wei P, Kong X, Xu J, Yao J, Cheng F, Ou D, Wang L, Xu D, Han Z. The value of ultrasound grayscale ratio in the diagnosis of papillary thyroid microcarcinomas and benign micronodules in patients with Hashimoto's thyroiditis: A two-center controlled study. Front Endocrinol (Lausanne) 2022;13:949847. [Crossref] [PubMed]
- Yadav N, Dass R, Virmani J. A systematic review of machine learning based thyroid tumor characterisation using ultrasonographic images. J Ultrasound 2024;27:209-24. [Crossref] [PubMed]
- Yu Q, Jiang T, Zhou A, Zhang L, Zhang C, Xu P. Computer-aided diagnosis of malignant or benign thyroid nodes based on ultrasound images. Eur Arch Otorhinolaryngol 2017;274:2891-7. [Crossref] [PubMed]
- Shin I, Kim YJ, Han K, Lee E, Kim HJ, Shin JH, Moon HJ, Youk JH, Kim KG, Kwak JY. Application of machine learning to ultrasound images to differentiate follicular neoplasms of the thyroid gland. Ultrasonography 2020;39:257-65. [Crossref] [PubMed]
- Giovanella L, Campennì A, Tuncel M, Petranović Ovčariček P. Integrated Diagnostics of Thyroid Nodules. Cancers (Basel) 2024;16:311. [Crossref] [PubMed]
- Sultan SR. B-mode Ultrasound Characteristics of Thyroid Nodules With High-Benign Probability and Nodules With Risk of Malignancy. Cureus 2023;15:e39281. [Crossref] [PubMed]
- Wang CY, Li Y, Zhang MM, Yu ZL, Wu ZZ, Li C, Zhang DC, Ye YJ, Wang S, Jiang KW. Analysis of Differential Diagnosis of Benign and Malignant Partially Cystic Thyroid Nodules Based on Ultrasound Characterization With a TIRADS Grade-4a or Higher Nodules. Front Endocrinol (Lausanne) 2022;13:861070. [Crossref] [PubMed]
- Shi M, Nong D, Xin M, Lin L. Accuracy of Ultrasound Diagnosis of Benign and Malignant Thyroid Nodules: A Systematic Review and Meta-Analysis. Int J Clin Pract 2022;2022:5056082. [Crossref] [PubMed]
- Lee JH, Ha EJ, Lee DH, Han M, Park JH, Kim JH. Clinicoradiological Characteristics in the Differential Diagnosis of Follicular-Patterned Lesions of the Thyroid: A Multicenter Cohort Study. Korean J Radiol 2022;23:763-72. [Crossref] [PubMed]
- Haugen BR, Alexander EK, Bible KC, Doherty GM, Mandel SJ, Nikiforov YE, Pacini F, Randolph GW, Sawka AM, Schlumberger M, Schuff KG, Sherman SI, Sosa JA, Steward DL, Tuttle RM, Wartofsky L. 2015 American Thyroid Association Management Guidelines for Adult Patients with Thyroid Nodules and Differentiated Thyroid Cancer: The American Thyroid Association Guidelines Task Force on Thyroid Nodules and Differentiated Thyroid Cancer. Thyroid 2016;26:1-133. [Crossref] [PubMed]
- Kim HJ, Mok JO, Kim CH, Kim YJ, Kim SJ, Park HK, Byun DW, Suh K, Yoo MH. Preoperative serum thyroglobulin and changes in serum thyroglobulin during TSH suppression independently predict follicular thyroid carcinoma in thyroid nodules with a cytological diagnosis of follicular lesion. Endocr Res 2017;42:154-62. [Crossref] [PubMed]
- Lee EK, Chung KW, Min HS, Kim TS, Kim TH, Ryu JS, Jung YS, Kim SK, Lee YJ. Preoperative serum thyroglobulin as a useful predictive marker to differentiate follicular thyroid cancer from benign nodules in indeterminate nodules. J Korean Med Sci 2012;27:1014-8. [Crossref] [PubMed]
- Zhang T, He L, Wang Z, Dong W, Sun W, Zhang P, Zhang H. Risk factors for death of follicular thyroid carcinoma: a systematic review and meta-analysis. Endocrine 2023;82:457-66. [Crossref] [PubMed]
- Wong KT, Ahuja AT. Ultrasound of thyroid cancer. Cancer Imaging 2005;5:157-66. [Crossref] [PubMed]
- Park JW, Kim DW, Kim D, Baek JW, Lee YJ, Baek HJ. Korean Thyroid Imaging Reporting and Data System features of follicular thyroid adenoma and carcinoma: a single-center study. Ultrasonography 2017;36:349-54. [Crossref] [PubMed]
- Malhi H, Beland MD, Cen SY, Allgood E, Daley K, Martin SE, Cronan JJ, Grant EG. Echogenic foci in thyroid nodules: significance of posterior acoustic artifacts. AJR Am J Roentgenol 2014;203:1310-6. [Crossref] [PubMed]
- Na DG, Baek JH, Jung SL, Kim JH, Sung JY, Kim KS, et al. Core Needle Biopsy of the Thyroid: 2016 Consensus Statement and Recommendations from Korean Society of Thyroid Radiology. Korean J Radiol 2017;18:217-37. [Crossref] [PubMed]