Current status and quality of prognosis prediction models of non-small cell lung cancer constructed using computed tomography (CT)-based radiomics: a systematic review and radiomics quality score 2.0 assessment
Introduction
Radiomics is a new technological tool built on automated algorithms that use non-invasive methods to extract significant amounts of image data from standard medical imaging. It enables feature extraction and model building, and aids doctors in making the best decisions through deeper mining of significant image data, including many features that are difficult to identify or quantify by the human eye (1-3). Numerous studies have been conducted on cancer types such as gastric, oropharyngeal, and breast cancer, and valuable research progress has been made in etiology, diagnosis, and prediction of prognosis (4-11).
Lung cancer is the second most common cancer worldwide and the leading cause of cancer-related death. It was predicted that in 2020, there would be 19.3 million new cases of and 9.9 million fatalities from lung cancer worldwide (12-14). Non-small cell lung cancer (NSCLC), which also accounts for the majority of cancer-related mortality, is the primary cause of the majority of primary lung malignancies (80–90%). Some of the therapeutic modalities used to treat NSCLC include surgery, radiotherapy, including stereotactic ablative radiotherapy, and systemic therapy, which includes cytotoxic chemotherapy, tyrosine kinase inhibitors (TKIs), and immune checkpoint inhibitors (ICIs) (15-20). Signatures based on computed tomography (CT) characteristics allow for stratification of survival risk in NSCLC patients but are influenced by stage, presence of genetic mutations, and receipt of multiple treatments. These features can also be combined with clinical and biological features to create prognostic models. The use of radiogenomics technology enables the early detection of quantitative indicators of treatment response during treatment. Multiple imaging sessions allow for the dynamic observation of feature changes. Therefore, the treatment plan can be adjusted according to the condition in a more timely manner to increase the effectiveness of the disease treatment. It has been demonstrated that patients with resectable lung cancer can be successfully divided into high-, intermediate-, and low-risk categories using the integrated model of radiomics signatures (21-23).
For NSCLC, numerous predictive radiomics signals have been created and validated. The prognostic prediction of NSCLC through artificial intelligence is improving with the use of deep learning (DL) in the field of radiomics, increasing the overall judgment accuracy of thoracic surgery specialists, promising to address the judgment bias caused by an imbalance of medical resources, and paving the way for individualized and precise NSCLC treatment (24).
In 2017, radiomics quality score (RQS), the first scoring standard to evaluate the quality of radiomics research, was introduced. It provides certain guidelines for the research of radiomics, but its practical application value is greatly reduced because its scoring items are too general and lack classification. The RQS 2.0 is an expansive framework developed to bolster the scientific integrity of radiomics research. It features a comprehensive checklist of 36 checkpoints, structured around the FUTURE-AI principles—Fairness, Universality, Traceability, Usability, Robustness, and Explainability. These checkpoints are designed to cover a wide range of aspects in radiomics studies. Employing RQS 2.0 enables a rigorous assessment to ascertain the extent of standardization in relevant studies, evaluate the scope of model applicability, assess the potential for clinical transformation, and determine the comprehensiveness of statistical evaluations conducted during the development of these models. A high RQS is indicative of exceptional research quality, a pivotal factor in propelling the radiomics field forward and broadening its clinical integration. Despite its significance, the lung cancer domain presently lacks a systematic evaluation that employs the RQS 2.0 criteria to gauge the quality of its studies.
Therefore, this study aimed to use RQS 2.0 to evaluate the quality of current CT-based radiomics prognostic models in NSCLC, point out the shortcomings of current research in this field, and improve the quality of studies for clinical transformation. We present this article in accordance with the PRISMA reporting checklist (available at https://qims.amegroups.com/article/view/10.21037/qims-24-22/rc).
Methods
Search strategy
The review methodology has been made available through Prospero (CRD42022373326). The physician (X.J.) outlined a search plan. A thorough database search was carried out (PubMed, Embase, and the Cochrane Library, search dates 1 November 2012 to 30 November 2022) (Figure 1). The last search date was 1 December 2022. The search phrases used were “Carcinoma, Non-Small Cell Lung”, “Prognosis”, “Radiomic*”, and “Quantitative Imaging”, and also included agreement or approximation words for these words. A thorough search plan is provided in Appendix 1.
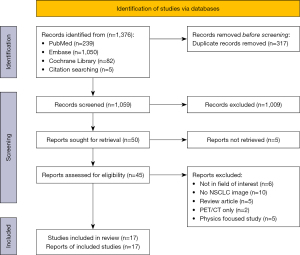
Study selection
The publication’s title and abstract were evaluated by 2 reviewers (X.J. and Y.W.), who separately evaluated them. The 2 reviewers determined together which research should be included in the article, and any discrepancies were settled by discussion and negotiation with a third reviewer (H.Z.). The patient, intervention, comparison, outcome (PICO) criteria served as the basis for inclusion criteria: (I) research on individuals with NSCLC. (II) CT feature extraction for radiomics. (III) The outcomes are models for predicting survival. Studies meeting the following criteria were excluded: (I) research on other types of lung cancer, such as small cell lung cancer. (II) Types of publications other than original research articles (e.g., case studies and series, abstracts from conferences, review articles, editorials, and letters and letter replies). (III) Studies focusing only on imaging techniques, not on the prognostic value. (IV) Studies with small sample sizes (fewer than 10 patients). (V) Animal studies. (VI) Only radio markers were studied. (VII) Models are diagnostic, not predictive
Data collection
Features of interest were extracted from the articles: clinical staging of NSCLC, radiomics features, relevant prognostic outcomes, cohort characteristics, and relevant findings. The studies were classified into handcrafted radiomics (HCR) and DL according to the method used.
Analysis of method quality based on RQS 2.0
With 6 domains—Fairness, Universality, Traceability, Usability, Robustness, and Explainability—the RQS 2.0 score has grown from 16 checkpoints to 36. The new radiomics scoring criterion sets HCR apart from DL, with its phantom study on all scanners, feature reduction, and test feature stability. DL has a total score of 61, whereas HCR has a score of 66. The RQS scoring website (https://www.radiomics.world) was used for scoring. The evaluation procedure involved 3 people, with 2 of them independently conducting the RQS evaluation for each of the 6 literary categories. In the event of a tie, the third assessor’s opinion was sought before finalization.
Results
Included studies
A total of 3 studies addressed stage IV (25-27), 5 studies addressed stage III (24,28-31), and 3 studies addressed stage I and II NSCLC (24,32,33). A total of 7 studies did not specify the specific stage of NSCLC (34-40). A total of 15 studies were HCR and 2 studies were DL.
In 2 studies (30,31), good statistical results were achieved in patients with stage III NSCLC for overall survival (OS) with a model constructed using tumor characterization only. One of the models for patients receiving definitive concomitant radiotherapy (CCRT) showed that entropy, skewness, and mean attenuation in texture features were significantly associated with 3-year OS. The study by Fried et al. (31) further combined these features with conventional prognostic factors (CPFs), and compared with models using CPFs alone, models including textural features and CPFs showed significant improvements in risk stratification for OS (P=0.046) among patients receiving definitive chemotherapy. Hosny et al. (24) and He et al. (40) showed that models including DL features can perform good risk stratification for patients.
In terms of region of interest selection, 13 studies were single-tumor and 4 studies were tumor plus peritumoral volume (PTV); regarding the number of features, 3 studies extracted more than 500 features, and these studies downscaled the features before building the model. A total of 5 studies had 100–500 features, 2 studies had less than 10 features, and 3 studies had an unclear number of features. Among these features, inverse different moment, lobulation sign, entropy, angular second moment, high intensity long-run emphasis, and long-run emphasis were significantly associated with NSCLC prognosis. A total of 7 studies validated the model using independent cohorts (3 studies used >1 independent validation cohort), and 10 studies did not perform independent cohort validation. The prognostic results of the studies are presented in Table 1.
Table 1
Reference | Stage | VOIs | Trial | Study design | Imaging modality | Total number of features | Cohort size | Outcomes tested | Test performance (AUC or C-index) | Conclusion | |||
---|---|---|---|---|---|---|---|---|---|---|---|---|---|
Model development | Independent validation cohort | ||||||||||||
Train | Test | All | |||||||||||
Song et al. (25) | IV | GTV | Multi-center | Retrospective | Contrast CT | 1,032 | 117 | – | 117 | N1 (101), N2 (96) | PFS | Ten months: T1: 0.738; V1: 0.711; V2: 0.722; one year: T1: 0.701; V1: 0.771; V2: 0.822 | CT-based predictive strategy can achieve individualized prediction of PFS probability to EGFR-TKI therapy in NSCLCs |
Wang et al. (34) | NR | GTV | Multi-center | Retrospective | CT | 258 | CV | – | 124 | 49 | 3-year survival | T1: 0.92; V1: 0.84 | CT radiomics features could effectively assist doctors to make more accurate prognosis survival prediction for NSCLC patients |
Huang et al. (35) | NR | GTV | Multi-center | Retrospective | CT | 203 | CV | 63 | 317 | 54 | OS | Targeted therapy group: 0.573; nontargted therapy group: 0.832 | The applicability of a general signature is limited and developing special radiomics signatures for patients treated with tyrosine kinase inhibitors might be necessary |
Vaidya et al. (32) | I, II | GTV + PTV | Multi-center | Retrospective | CT | 124 | 329 | – | 329 | N1 (114), N2 (82) | DFS | 0.74 | A 13-feature based QuRiS, which was prognostic and predictive of benefit to adjuvant chemotherapy following curative resection in ES-NSCLC |
Fave et al. (28) | III | GTV | Single-center | Retrospective | CT | 65 | CV | – | 137 | – | OS, DM | Model 1: 0.579; Model 2: 0.672; Model 3: 0.675 | Radiomics features change due to radiation therapy and their values at the end of treatment may be indicators of tumor response |
Starkov et al. (36) | NR | GTV | Single-center | Retrospective | CT | – | CV | – | 116 | – | OS, LF, RF | Good assessment of PFS | Using imaging histology features in CT images can predict patient survival after SABR in NSCLC |
Khorrami et al. (29) | III | GTV + PTV | Single-center | Retrospective | CT | 1,542 | 45 | 45 | 90 | – | DF, PFS, DFS | T1: 0.90; V1: 0.86 | Texture features extracted within and around the lung tumor on CT images appears to be associated with the likelihood of MPR, OS and DFS to chemoradiation |
Choe et al. (37) | NR | GTV | Single-center | Retrospective | CE-CT | 718 | 754 | – | 754 | 304 | DFS, OS | T1: 0.764; V1: 0.782 | CT radiomics signature was an independent prognostic factor predicting disease-free and overall survival along with clinical risk factors of lung adenocarcinoma (stage, histologic subtype, and age) |
Bak et al. (26) | IV | GTV | Single-center | Retrospective | CT | 23 | 53 | – | 53 | – | OS | 1 year: T1: 0.948; 3 years: T1: 0.862 | Longitudinal change of radiomic tumor features may serve as prognostic biomarkers in patients with advanced NSCLC |
Huang et al. (33) | I, II | GTV | Single-center | Retrospective | CT | 132 | 141 | – | 141 | – | DFS | 0.72 | The radiomics signature is an independent biomarker for the estimation of DFS in patients with early-stage NSCLC |
Ahn et al. (30) | III | GTV | Single-center | Retrospective | CE-CT | 6 | – | – | 98 | – | OS | Good assessment of OS | Computed tomography texture features have the potential to be used as prognostic biomarkers in unresectable NSCLC patients undergoing definitive CCRT |
Fried et al. (31) | III | GTV | Single-center | Retrospective | CE-CT | 33 | CV | – | 91 | – | OS, LRC, FFDM | A significant improvement in risk stratification for OS | Pretreatment tumor texture may provide prognostic information beyond what is obtained from CPFs |
Zhang et al. (38) | NR | GTV + PTV | Single-center | Retrospective | CE-CT | 72 | CV | – | 41 | – | PFS, DSS | LR: 0.722; LNM; 0.771; DM: 0.731–0.855 | Textual features derived from pretreatment CT scans have prognostic value in early-stage NSCLC patients treated with SBRT |
Zhou et al. (39) | NR | GTV | Single-center | Retrospective | CE-CT | 7 | CV | – | 64 | – | OS | Shown to be significantly associated with 5-year OS (P<0.05) | Entropy is an important and potentially non-invasive imaging biomarker for predicting the prognosis of NSCLC undergoing curative resection |
Li et al. (27) | IV | GTV | Single-center | Retrospective | CT | 481 | 32 | – | 31 | 105 | PFS | T1: 0.895; V1: 0.824 | The proposed radiomic signature was found to be an effective prognostic factor in stage IV ALK mutated nonsynchronous nodules in NSCLC patients treated with a TKI |
Hosny et al. (24) | I–III | GTV + PTV | Multi-center | Retrospective | CT | NR | 1,194 | – | – | N1 (771), N2 (391) | OS | Radiotherapy: 0.70; surgery: 0.71 | Deep learning networks may be used for mortality risk stratification based on standard-of-care CT images from NSCLC patients |
He et al. (40) | NR | GTV | Single-center | Retrospective | CT | NR | 164 | 48 | 236 | – | OS, PFS | T1: 0.77; V1: 0.79 | CT radiomics could be applied for individualized immunotherapy of NSCLC |
VOIs, volumes of interest; AUC, area under the curve; GTV, gross tumor volume; CT, computed tomography; N1, independent validation cohort 1; N2, independent validation cohort 2; PFS, progression-free survival; EGFR, epidermal growth factor receptor; TKI, tyrosine kinase inhibitor; NSCLC, non-small cell lung cancer; NR, not recorded; CV, cross validation; OS, overall survival; PTV, peritumoral volume; DFS, disease-free survival; QuRiS, quantitative radiomic risk score; ES, early stage; DM, distant metastasis; LF, local failure; RF, regional failure; DF, distant failure; SABR, stereotactic ablative radiotherapy; MPR, major pathological response; CE-CT, contrast-enhanced computed tomography; CCRT, concomitant chemoradiotherapy; LRC, local-regional control; FFDM, freedom from distant metastases; CPFs, conventional prognostic factors; LR, local recurrence; LNM, lymph node metastasis; SBRT, stereotactic body radiotherapy; ALK, anaplastic lymphoma kinase.
A total of 3 studies showed that the effectiveness of treatment is closely related to the patient’s own disease type and tumor heterogeneity. Song et al. (25) established a model for stage IV epidermal growth factor receptor (EGFR)-mutated NSCLC treated with TKIs. For progression-free survival (PFS), the model was built with an AUC >0.7 at either 10 months or 1 year and was validated using independent cohorts. Further, the prognostic model developed by Li et al. (27) for anaplastic lymphoma kinase (ALK)-positive patients in an EGFR-positive cohort did not show a significant association between radiomic features and PFS (log-rank tests, P=0.41). Meanwhile, Huang et al. (35) constructed a prognostic model of OS for ALK-positive patients by applying general radiomic features (Geom_va_ratio, W_GLCM_Std, W_GLCM_DV, W_GLCM_IM2, and W_his_mean). Its accuracy in predicting OS reached 0.649 [95% confidence interval (CI), 0.640–0.658] in the ALK+ group, but impaired function was observed in the targeted treatment group (C-index =0.573; 95% CI, 0.556–0.589), while significantly improved performance was observed in the non-targeted treatment group (C-index =0.832; 95% CI, 0.832–0.852).
RQS 2.0 assessment
The range of included study scores was 8–27 (12–44%), the median was 18 points, and the corresponding percentage was 27%. All studies were retrospective, and no cohort studies were registered in advance (Table 2). This features were described in each of the 4 studies: classification of the model, hardware used d, image reconstruction method specified, and quality management system. Just 1 study (6%) had clinicians involved in the discussion of interpretable methods. A total of 16 studies (94%) had relatively complete image protocol quality; 14 studies (82%) considered changes in slice thickness/convolution kernel/contrast; 4 studies (24%) collected images of individuals at additional time points and analyzed the robustness of features to temporal changes. The majority of studies described the specifications of CT instruments, but only 1 study (6%) clearly stated the inclusion-exclusion criteria. A total of 2 studies (13%, excluding 2 DL studies) detected inter-scanner differences and vendor-dependent features, and many studies did not propose scientifically feasible solutions, although they mentioned the existence of errors between different devices. Eleven studies (65%) acted on segmentation by different physicians/algorithms/software, by (random) noise perturbing segmentation, at different respiratory cycles. For statistical analysis, 15 studies (88%) had cut-off analyses, 16 studies (94%) had discrimination statistics, and 3 studies (18%) had calibration statistics. It is noteworthy that no studied algorithm was tested in a clinical environment. A total of 6 studies (35%) compared newly created models with known models, 6 studies (40%) conducted multivariable analysis with non-radiomics features, and 10 studies (59%) compared predictive models with gold standards. No study was able to disclose all 6 of the following: Fairness (17%), Universality (27%), Traceability (18%), Usability (21%), Robustness (28%), and Explainability (4%) (Figure 2).
Table 2
RQS number and name | (25) | (34) | (35) | (32) | (28) | (36) | (29) | (37) | (26) | (33) | (30) | (31) | (38) | (39) | (27) | (24) | (40) | % Score |
---|---|---|---|---|---|---|---|---|---|---|---|---|---|---|---|---|---|---|
1: Unmet clinical need defined | 1 | 1 | 2 | 1 | 0 | 0 | 0 | 0 | 0 | 0 | 0 | 0 | 0 | 0 | 0 | 2 | 0 | 24 |
2: Classification of the model | 1 | 1 | 1 | 1 | 1 | 1 | 1 | 1 | 1 | 1 | 1 | 1 | 1 | 1 | 1 | 1 | 1 | 100 |
3: Input from clinicians | 0 | 1 | 0 | 0 | 0 | 0 | 0 | 0 | 0 | 0 | 0 | 0 | 0 | 0 | 0 | 0 | 0 | 6 |
4: Image protocol quality | 1 | 1 | 0 | 1 | 1 | 1 | 1 | 1 | 1 | 1 | 1 | 1 | 1 | 1 | 1 | 1 | 1 | 94 |
5: Hardware’s used described, image reconstruction method specified | 1 | 1 | 1 | 1 | 1 | 1 | 1 | 1 | 1 | 1 | 1 | 1 | 1 | 1 | 1 | 2 | 2 | 100 |
6: Preprocessing of the images | 0 | 1 | 0 | 0 | 1 | 1 | 1 | 1 | 1 | 1 | 1 | 1 | 1 | 1 | 1 | 1 | 1 | 82 |
7: Imaging at multiple time points | 0 | 0 | 1 | 0 | 1 | 0 | 1 | 0 | 0 | 0 | 0 | 1 | 0 | 0 | 0 | 0 | 0 | 24 |
8: Inclusion and exclusion criteria or boundaries of the model defined | 0 | 0 | 0 | 0 | 1 | 0 | 0 | 0 | 0 | 0 | 0 | 0 | 0 | 0 | 0 | 0 | 0 | 6 |
9: Phantom study on all scanners | 0 | 1 | 0 | 0 | 0 | 0 | 1 | 0 | 0 | 0 | 0 | 0 | 0 | 0 | 0 | – | – | 13 |
10: Mitigation strategies applied | 0 | 1 | 0 | 0 | 0 | 0 | 0 | 0 | 0 | 0 | 0 | 0 | 0 | 0 | 0 | 0 | 0 | 6 |
11: Use of post-processing harmonization | 0 | 0 | 0 | 0 | 0 | 0 | 0 | 0 | 0 | 0 | 0 | 0 | 0 | 0 | 0 | 0 | 0 | 0 |
12: Method and statistical plan pre-registered on a public platform | 0 | 0 | 0 | 0 | 0 | 0 | 0 | 0 | 0 | 0 | 0 | 0 | 0 | 0 | 0 | 0 | 0 | 0 |
13: Training dataset | 0 | 0 | 0 | 0 | 0 | 0 | 0 | 0 | 0 | 0 | 0 | 0 | 0 | 0 | 0 | 2 | 0 | 6 |
14: Multiple segmentations | 0 | 1 | 1 | 1 | 1 | 1 | 1 | 1 | 0 | 1 | 0 | 1 | 1 | 0 | 0 | 0 | 1 | 65 |
15: Feature reduction | 3 | 3 | 3 | 3 | 3 | 0 | 3 | 3 | 3 | 3 | 0 | 0 | 0 | 0 | 3 | – | – | 67 |
16: Multivariable analysis with non-radiomics features | 1 | 0 | 0 | 1 | 0 | 0 | 0 | 0 | 0 | 0 | 0 | 1 | 1 | 1 | 1 | – | – | 40 |
17: Cut-off analyses | 1 | 1 | 1 | 1 | 1 | 0 | 1 | 0 | 1 | 1 | 1 | 1 | 1 | 1 | 1 | 1 | 1 | 88 |
18: Random permutations | 1 | 1 | 1 | 0 | 0 | 0 | 0 | 0 | 0 | 0 | 0 | 0 | 0 | 0 | 0 | 0 | 1 | 24 |
19: Comparative analysis or ensemble of HCR and DL approaches | 0 | 0 | 0 | 0 | 0 | 0 | 0 | 0 | 0 | 0 | 0 | 0 | 0 | 0 | 0 | 1 | 0 | 0 |
20: Quality Management System | 1 | 1 | 1 | 1 | 1 | 1 | 1 | 1 | 1 | 1 | 1 | 1 | 1 | 1 | 1 | 1 | 1 | 100 |
21: Discrimination statistics | 1 | 1 | 1 | 1 | 1 | 2 | 2 | 1 | 1 | 1 | 0 | 1 | 1 | 0 | 1 | 1 | 1 | 94 |
22: Calibration statistics | 1 | 0 | 0 | 0 | 0 | 0 | 0 | 2 | 0 | 1 | 0 | 0 | 0 | 0 | 0 | 0 | 0 | 18 |
23: Comparison with previously published radiomics signatures and models | 0 | 0 | 0 | 1 | 1 | 0 | 0 | 1 | 0 | 1 | 0 | 0 | 0 | 0 | 1 | 1 | 0 | 35 |
24: Validation | 4 | 3 | 3 | 4 | 2 | 2 | 2 | 2 | 2 | 2 | 0 | 2 | 2 | 0 | 2 | 5 | 1 | 88 |
25: Prospective study registered in a trial database | 0 | 0 | 0 | 0 | 0 | 0 | 0 | 0 | 0 | 0 | 0 | 0 | 0 | 0 | 0 | 0 | 0 | 0 |
26: Algorithm tested in a clinical environment | 0 | 0 | 0 | 0 | 0 | 0 | 0 | 0 | 0 | 0 | 0 | 0 | 0 | 0 | 0 | 0 | 0 | 0 |
27: Evaluation the identified discriminative biases | 0 | 0 | 1 | 1 | 1 | 0 | 0 | 0 | 0 | 0 | 0 | 0 | 0 | 0 | 1 | 1 | 1 | 35 |
28: Detect and discuss biological correlates | 0 | 0 | 0 | 1 | 0 | 0 | 0 | 0 | 0 | 0 | 0 | 0 | 0 | 1 | 0 | 1 | 1 | 24 |
29: Details on the intrinsic or post-hoc interpretability method or uncertainty estimation method utilized | 0 | 0 | 0 | 0 | 0 | 0 | 0 | 0 | 0 | 0 | 0 | 0 | 0 | 0 | 0 | 0 | 1 | 6 |
30: Comparison to ‘gold standard’ | 2 | 2 | 0 | 2 | 2 | 2 | 2 | 2 | 0 | 2 | 0 | 0 | 0 | 0 | 2 | 2 | 0 | 59 |
31: Potential clinical utility | 2 | 0 | 0 | 2 | 0 | 0 | 2 | 0 | 0 | 2 | 0 | 0 | 0 | 0 | 0 | 0 | 0 | 18 |
32: Cost-effectiveness analysis | 0 | 0 | 1 | 0 | 0 | 0 | 0 | 0 | 0 | 0 | 0 | 0 | 0 | 0 | 0 | 0 | 0 | 6 |
33: Level of automation for the clinical practice | 1 | 1 | 1 | 1 | 1 | 1 | 1 | 1 | 1 | 1 | 1 | 1 | 1 | 1 | 1 | 1 | 1 | 100 |
34: The algorithm, source code, and coefficients are made publicly available | 0 | 0 | 0 | 0 | 0 | 0 | 0 | 0 | 0 | 0 | 1 | 1 | 1 | 1 | 0 | 1 | 1 | 35 |
35: Open data | 1 | 1 | 1 | 1 | 0 | 1 | 1 | 0 | 0 | 1 | 0 | 0 | 0 | 0 | 0 | 1 | 0 | 47 |
36: Strategy to update models | 0 | 0 | 0 | 1 | 1 | 1 | 1 | 0 | 0 | 0 | 0 | 0 | 0 | 0 | 0 | 1 | 1 | 35 |
% RQS (total score) | 35 (23) | 35 (23) | 30 (20) | 39 (26) | 32 (21) | 23 (15) | 35 (23) | 27 (18) | 20 (13) | 32 (21) | 12 (8) | 21 (14) | 20 (13) | 15 (10) | 27 (18) | 44 (27) | 28 (17) | – |
The scoring was carried out on the RQS website (https://www.radiomics.world) and each item is assigned a corresponding score, with the score and total score for each item displayed in the table. RQS, radiomics quality scoring; HCR, handcrafted radiomics; DL, deep learning.
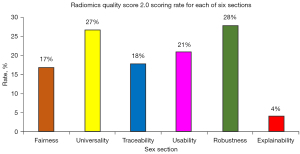
Discussion
The rapid increase in radiomic studies of NSCLC prognosis and the update of RQS scores has made it necessary to comprehensively re-evaluate of the quality of relevant studies to inform subsequent studies. Using the RQS 2.0 scoring system, we found that the scientific aspects of radiomics studies were poor, with a median score of only 18 for RQS 2.0. For radiomics models to become clinically meaningful tools, future studies need to adopt standardized methods that meet the RQS 2.0 criteria. Compared with previous studies, this study elucidates the shortcomings of this field in 6 aspects in detail, and proposes some improvement ideas for RQS 2.0.
Recent years have seen a remarkable advancement in radiomics research in NSCLC, with the publication of several hundred articles covering genesis, diagnosis, and prognosis (41-44). Of all the evidence on prognosis, the strongest level of evidence supporting the clinical validity and utility of the radiomics biomarker needs to come from a prospective study that is registered in a trial database (real-world or in silico), including sample size calculation. However, the need for large-sample prospective research is so far unmet, and the majority of studies have been straightforward retrospective studies that lack independent external validation. Therefore, it is vital to achieve a suitable design for scientific studies as well as the stability and transparency of the relevant data throughout the investigation.
We have drawn several conclusions from these studies: firstly, entropy is frequently mentioned as a predictor and warrants more study (45). The included studies demonstrate that entropy serves as an independent prognostic factor for predicting PFS in NSCLC stereotactic body radiotherapy (SBRT) (38). Entropy is significantly correlated with 5-year OS in NSCLC (39), and longitudinal changes in entropy values at different time points may serve as prognostic biomarkers for advanced NSCLC patients (26). Furthermore, entropy enables personalized prediction of PFS probability after immunotherapy in NSCLC (25). These studies underscore the significance of entropy in radiomics, where varying entropy values reflect different disease states. Similar reports exist in other cancer domains. In gastroesophageal junction carcinoma, entropy is significantly correlated with patients’ relapse-free survival (RFS) and OS, and entropy is an independent prognostic factor for RFS and OS (46). Further, entropy is an important imaging feature to distinguish Luminal A and Luminal B molecular subtypes in patients with invasive breast cancer (47). Entropy is also one of the most important radiomic features for predicting survival after chemotherapy in patients with liver metastases (48). However, it is worth noting that although the models constructed in relevant papers have good efficacy, they are not strictly in accordance with the RQS standard, which has also been verified in the systematic evaluation of gastroenteropancreatic neuroendocrine tumors (45). The level of the entropy value represents differences in significance, and it is of great importance to classify entropy values to guide clinical decision-making in the future. Secondly, building dynamic models of these changing elements will assist physicians in defining more precise treatment plans by allowing them to consider the changes in numerous independent predictive features over the course of treatment (49). Thirdly, it is important to note that none of the included research conducted a cost-effectiveness analysis [report on the cost-effectiveness of the clinical application, e.g., quality-adjusted life-years (QALYs) generated] (50,51). Cost-effectiveness analysis is of great clinical value. The goal of all of our included studies was to maximize patient benefit, not to measure patient quality of life, but only look at survival or mortality, which is of limited significance to patients. QALY has the advantage of simultaneously capturing gains from decreased illness (quality gains) and mortality (quantity gains) and combining both into a single metric. The preferences of society as a whole determine, to some extent, clinician judgment and patient choice. Even for the same health state using the same measurement method [for example, the EQ-5D (52), which is most frequently used in Western Europe], there are varying weighting values because each country has diverse cultural backgrounds and unique socioeconomic contexts. Investigating the relationship between radiomics and patient quality of life is important; however, it is often difficult to take into account the needs of each patient in clinical research. How to construct a prognostic model that can meet the needs of different patients is worth considering.
DL has made great progress in radiomics investigations of NSCLC, both in patients treated with radiation and those treated with surgery (24). It predicts 2-year survival well, with stability in retesting and inter-reader variability scenarios. It has been demonstrated that in radiation therapy patients, DL networks outperform random forest models built using artificial features, but not in surgery patients. Previous studies have shown that DL is mainly used for feature extraction and model construction, where feature extraction is more important because it can extract features of the tumor microenvironment (TME), which is crucial for the growth and development of tumor cells (53). The TME plays an indispensable role in predicting tumor growth and patient OS. In the realm of tumor treatment, immunotherapy is gaining importance, and DL algorithms based on CT image analysis can identify individuals who would benefit from immunotherapy. It has been shown that the accuracy of classifying patients’ OS and PFS by manually extracting segmentation from their 3D tumor images and directly building survival networks for modeling using patient follow-up data was 83.3% and 77.5% in the test set (40), respectively. DL will be increasingly utilized in radiomics since it can continue to be educated as additional data becomes available, boosting its accuracy. Additionally, alternative neural network architectures can be utilized to address a variety of data issues, including those involving genetic, expression, clinical, text, and image (unstructured) data (54). With the rise of the second generation sequencing technology, the construction of radiation genomics model will undoubtedly become the focus of future research. However, there are many shortcomings. Due to the opaque black-box nature of DL networks, the scientific validity of related research needs further study; furthermore, when looking at the cohort section, many of the studies were single-center retrospective studies. When looking at the angle of using the loss function, there have not been enough restrictions on samples with and without endpoints, and the network has not been accurate enough. Future research should focus on increasing testing and improving algorithms and conducting large-scale prospective trials to build state-of-the-art models to improve prognosis prediction in cancer.
Radiomics investigations can be separated into 5 steps: data selection, medical imaging, feature extraction, exploratory analysis, and modeling. In 2017, Lambin et al. proposed the RQS grading standards, which offers a referenceable standard for radiomics investigations, to evaluate the caliber of radiomics studies. The RQS score has been updated recently, and using this for quality evaluation of radiomics research is the focus of this article. Unlike RQS 1.0, RQS 2.0 divides the score into 6 parts (https://www.radiomics.world/rqs2). According to Fairness, relevant studies should report the diversity and distribution of different patient groups in the data set, so as to identify potential bias, evaluate the sources of identified discriminatory bias, and take appropriate corrective measures. Universality encourages researchers to conduct multicenter experiments with well-defined model classifications. This can reduce the overfitting of related studies and improve the applicability of the model. Traceability is essential. A good clinical trial requires a standard workflow and scientific trial design. For a study to have high confidence, the image protocol quality should be documented following the Transparent Reporting of Medical Image Acquisition for a future proof radiomics (TRIAC) level. In addition, for the use of hardware, image reconstruction methods should be standardized across research centers. Researchers should describe any differences in methods to reduce errors. Usability is where most articles currently need to improve. Most studies do not report on the potential clinical utility and level of automation for the clinical practice. This makes many studies less valuable for clinical use. Further, the public availability of the algorithm, source code, and coefficients as well as details on the intrinsic or post hoc interpretability method or uncertainty estimation method used is very important. If all these details are made public, there is a greater chance of reproducing or improving a certain study. A statistically beautiful study is not necessarily a good study, but a good study necessarily requires scientific statistical design and good statistical results. The Robustness part is intended to reduce the occasionality of research, for example, the discrimination statistics score is higher while the calibration statistics score is lower. It is not known whether authors simply ignore the robustness content to cover up the ineffectiveness of the model for those studies with low scores, but future studies must improve in these aspects. The final part, Explainability, means that the content of the research can be understood by experts in other fields, and the relevant algorithms or techniques in the model-building process can be disclosed, which is important. The division into these 6 parts means that RQS 2.0 further defines the norms to be followed and considered in the field of radiomics research.
According to the RQS 2.0 scoring results, most of the prognostic models were not combined with gene and protein expression analysis, and these differences in genes and proteins could affect the individual treatment plan of the patients concerned. The validity of the models constructed using purely radiomic features was insufficient in different scenarios; future studies should concentrate on the construction of composite models. It is also important to note that the models that included the checkpoint level of automation for the clinical practice, were all level 1 (partially helpful to the clinic), showing the limitations of the clinical application of the models in question. Future studies should provide a more detailed description of the specific benefit to the clinic, as well as elaborate on the adjustments made based on the radiomic predictions, and what the results of this were. This needs to be validated by prospective trials. In conclusion, the current radiomic prognostic model is still in the theoretical stage and exposes the shortcomings of poor integration with the clinical setting and clinicians.
However, our study also has some limitations. First, there are relatively few DL articles that meet the research inclusion criteria, which affects the comparison between DL and manual extraction of features. Second, although the RQS 2.0 rating scale is more thorough than that of the previous generation, improvements are constantly being made. Many scoring entries are too idealized, for example, effect of organ motion and organ expansion/contraction on imaging, and eliminate differences between detection scanners and differences between vendor-dependent features. Dismissing a study’s potential significance solely based on insufficient scores may also seem arbitrary. Our research indicates that the scoring proportions across the 6 sections of RQS 2.0 vary. In the future, we might set a threshold value, with each section having its own passing score, indicating that a study is meaningful in that aspect. For different studies, readers can first assess the scores of each section and then use their own experience to determine the value of the article. However, these standards have not yet been established. We will propose our opinions to the RQS officials and closely monitor subsequent developments. It will be necessary to improve RQS 2.0 in the future according to actual needs.
Conclusions
The quality of radiomics studies regarding the prognosis of NSCLC needs to be improved, and future studies should follow RQS 2.0 to improve the stability, transparency, and generalizability of the studies.
Acknowledgments
Funding: This study was supported by
Footnote
Reporting Checklist: The authors have completed the PRISMA reporting checklist. Available at https://qims.amegroups.com/article/view/10.21037/qims-24-22/rc
Conflicts of Interest: All authors have completed the ICMJE uniform disclosure form (available at https://qims.amegroups.com/article/view/10.21037/qims-24-22/coif). The authors have no conflicts of interest to declare.
Ethical Statement: The authors are accountable for all aspects of the work in ensuring that questions related to the accuracy or integrity of any part of the work are appropriately investigated and resolved.
Open Access Statement: This is an Open Access article distributed in accordance with the Creative Commons Attribution-NonCommercial-NoDerivs 4.0 International License (CC BY-NC-ND 4.0), which permits the non-commercial replication and distribution of the article with the strict proviso that no changes or edits are made and the original work is properly cited (including links to both the formal publication through the relevant DOI and the license). See: https://creativecommons.org/licenses/by-nc-nd/4.0/.
References
- Gillies RJ, Kinahan PE, Hricak H. Radiomics: Images Are More than Pictures, They Are Data. Radiology 2016;278:563-77. [Crossref] [PubMed]
- Kumar V, Gu Y, Basu S, Berglund A, Eschrich SA, Schabath MB, Forster K, Aerts HJ, Dekker A, Fenstermacher D, Goldgof DB, Hall LO, Lambin P, Balagurunathan Y, Gatenby RA, Gillies RJ. Radiomics: the process and the challenges. Magn Reson Imaging 2012;30:1234-48. [Crossref] [PubMed]
- Lambin P, Rios-Velazquez E, Leijenaar R, Carvalho S, van Stiphout RG, Granton P, Zegers CM, Gillies R, Boellard R, Dekker A, Aerts HJ. Radiomics: extracting more information from medical images using advanced feature analysis. Eur J Cancer 2012;48:441-6. [Crossref] [PubMed]
- Osman SOS, Leijenaar RTH, Cole AJ, Lyons CA, Hounsell AR, Prise KM, O'Sullivan JM, Lambin P, McGarry CK, Jain S. Computed Tomography-based Radiomics for Risk Stratification in Prostate Cancer. Int J Radiat Oncol Biol Phys 2019;105:448-56. [Crossref] [PubMed]
- Wang K, Lu X, Zhou H, Gao Y, Zheng J, Tong M, Wu C, Liu C, Huang L, Jiang T, Meng F, Lu Y, Ai H, Xie XY, Yin LP, Liang P, Tian J, Zheng R. Deep learning Radiomics of shear wave elastography significantly improved diagnostic performance for assessing liver fibrosis in chronic hepatitis B: a prospective multicentre study. Gut 2019;68:729-41. [Crossref] [PubMed]
- Phi XA, Tagliafico A, Houssami N, Greuter MJW, de Bock GH. Digital breast tomosynthesis for breast cancer screening and diagnosis in women with dense breasts - a systematic review and meta-analysis. BMC Cancer 2018;18:380. [Crossref] [PubMed]
- Yang F, Wang Y, Li Q, Cao L, Sun Z, Jin J, Fang H, Zhu A, Li Y, Zhang W, Wang Y, Xie H, Gustafsson JÅ, Wang S, Guan X. Intratumor heterogeneity predicts metastasis of triple-negative breast cancer. Carcinogenesis 2017;38:900-9. [Crossref] [PubMed]
- Sprague BL, Arao RF, Miglioretti DL, Henderson LM, Buist DS, Onega T, Rauscher GH, Lee JM, Tosteson AN, Kerlikowske K, Lehman CDBreast Cancer Surveillance Consortium. National Performance Benchmarks for Modern Diagnostic Digital Mammography: Update from the Breast Cancer Surveillance Consortium. Radiology 2017;283:59-69. [Crossref] [PubMed]
- Choi ER, Lee HY, Jeong JY, Choi YL, Kim J, Bae J, Lee KS, Shim YM. Quantitative image variables reflect the intratumoral pathologic heterogeneity of lung adenocarcinoma. Oncotarget 2016;7:67302-13. [Crossref] [PubMed]
- Park HJ, Lee SS, Park B, Yun J, Sung YS, Shim WH, Shin YM, Kim SY, Lee SJ, Lee MG. Radiomics Analysis of Gadoxetic Acid-enhanced MRI for Staging Liver Fibrosis. Radiology 2019;290:380-7. [Crossref] [PubMed]
- Li Wen Y, Leech M. Review of the Role of Radiomics in Tumour Risk Classification and Prognosis of Cancer. Anticancer Res 2020;40:3605-18. [Crossref] [PubMed]
- Siegel RL, Giaquinto AN, Jemal A. Cancer statistics, 2024. CA Cancer J Clin 2024;74:12-49. [Crossref] [PubMed]
- Molina JR, Yang P, Cassivi SD, Schild SE, Adjei AA. Non-small cell lung cancer: epidemiology, risk factors, treatment, and survivorship. Mayo Clin Proc 2008;83:584-94. [Crossref] [PubMed]
- Hawkins S, Wang H, Liu Y, Garcia A, Stringfield O, Krewer H, Li Q, Cherezov D, Gatenby RA, Balagurunathan Y, Goldgof D, Schabath MB, Hall L, Gillies RJ. Predicting Malignant Nodules from Screening CT Scans. J Thorac Oncol 2016;11:2120-8. [Crossref] [PubMed]
- Passaro A, Brahmer J, Antonia S, Mok T, Peters S. Managing Resistance to Immune Checkpoint Inhibitors in Lung Cancer: Treatment and Novel Strategies. J Clin Oncol 2022;40:598-610. [Crossref] [PubMed]
- Gandhi L, Rodríguez-Abreu D, Gadgeel S, Esteban E, Felip E, De Angelis F, et al. Pembrolizumab plus Chemotherapy in Metastatic Non-Small-Cell Lung Cancer. N Engl J Med 2018;378:2078-92. [Crossref] [PubMed]
- Deng H, Liu J, Cai X, Chen J, Rocco G, Petersen RH, Brunelli A, Ng CSH, D'Amico TA, Liang W, He J. Radical Minimally Invasive Surgery After Immuno-chemotherapy in Initially-unresectable Stage IIIB Non-small cell Lung Cancer. Ann Surg 2022;275:e600-2. [Crossref] [PubMed]
- Friedlaender A, Naidoo J, Banna GL, Metro G, Forde P, Addeo A. Role and impact of immune checkpoint inhibitors in neoadjuvant treatment for NSCLC. Cancer Treat Rev 2022;104:102350. [Crossref] [PubMed]
- Chang JY, Mehran RJ, Feng L, Verma V, Liao Z, Welsh JW, Lin SH, O'Reilly MS, Jeter MD, Balter PA, McRae SE, Berry D, Heymach JV, Roth JASTARS Lung Cancer Trials Group. Stereotactic ablative radiotherapy for operable stage I non-small-cell lung cancer (revised STARS): long-term results of a single-arm, prospective trial with prespecified comparison to surgery. Lancet Oncol 2021;22:1448-57. [Crossref] [PubMed]
- Chang JY, Senan S, Paul MA, Mehran RJ, Louie AV, Balter P, et al. Stereotactic ablative radiotherapy versus lobectomy for operable stage I non-small-cell lung cancer: a pooled analysis of two randomised trials. Lancet Oncol 2015;16:630-7. [Crossref] [PubMed]
- Perez-Johnston R, Araujo-Filho JA, Connolly JG, Caso R, Whiting K, Tan KS, Zhou J, Gibbs P, Rekhtman N, Ginsberg MS, Jones DR. CT-based Radiogenomic Analysis of Clinical Stage I Lung Adenocarcinoma with Histopathologic Features and Oncologic Outcomes. Radiology 2022;303:664-72. [Crossref] [PubMed]
- Wang T, She Y, Yang Y, Liu X, Chen S, Zhong Y, Deng J, Zhao M, Sun X, Xie D, Chen C. Radiomics for Survival Risk Stratification of Clinical and Pathologic Stage IA Pure-Solid Non-Small Cell Lung Cancer. Radiology 2022;302:425-34. [Crossref] [PubMed]
- Jiménez Londoño GA, García Vicente AM, Bosque JJ, et al. SUVmax to tumor perimeter distance: a robust radiomics prognostic biomarker in resectable non-small cell lung cancer patients. Eur Radiol 2022;32:3889-902. [Crossref] [PubMed]
- Hosny A, Parmar C, Coroller TP, Grossmann P, Zeleznik R, Kumar A, Bussink J, Gillies RJ, Mak RH, Aerts HJ WL. Deep learning for lung cancer prognostication: A retrospective multi-cohort radiomics study. PLoS Med 2018;15:e1002711. [Crossref] [PubMed]
- Song J, Shi J, Dong D, Fang M, Zhong W, Wang K, Wu N, Huang Y, Liu Z, Cheng Y, Gan Y, Zhou Y, Zhou P, Chen B, Liang C, Liu Z, Li W, Tian J. A New Approach to Predict Progression-free Survival in Stage IV EGFR-mutant NSCLC Patients with EGFR-TKI Therapy. Clin Cancer Res 2018;24:3583-92. [Crossref] [PubMed]
- Bak SH, Park H, Sohn I, Lee SH, Ahn MJ, Lee HY. Prognostic Impact of Longitudinal Monitoring of Radiomic Features in Patients with Advanced Non-Small Cell Lung Cancer. Sci Rep 2019;9:8730. [Crossref] [PubMed]
- Li H, Zhang R, Wang S, Fang M, Zhu Y, Hu Z, Dong D, Shi J, Tian J. CT-Based Radiomic Signature as a Prognostic Factor in Stage IV ALK-Positive Non-small-cell Lung Cancer Treated With TKI Crizotinib: A Proof-of-Concept Study. Front Oncol 2020;10:57. [Crossref] [PubMed]
- Fave X, Zhang L, Yang J, Mackin D, Balter P, Gomez D, Followill D, Jones AK, Stingo F, Liao Z, Mohan R, Court L. Delta-radiomics features for the prediction of patient outcomes in non-small cell lung cancer. Sci Rep 2017;7:588. [Crossref] [PubMed]
- Khorrami M, Jain P, Bera K, Alilou M, Thawani R, Patil P, Ahmad U, Murthy S, Stephans K, Fu P, Velcheti V, Madabhushi A. Predicting pathologic response to neoadjuvant chemoradiation in resectable stage III non-small cell lung cancer patients using computed tomography radiomic features. Lung Cancer 2019;135:1-9. [Crossref] [PubMed]
- Ahn SY, Park CM, Park SJ, Kim HJ, Song C, Lee SM, McAdams HP, Goo JM. Prognostic value of computed tomography texture features in non-small cell lung cancers treated with definitive concomitant chemoradiotherapy. Invest Radiol 2015;50:719-25. [Crossref] [PubMed]
- Fried DV, Tucker SL, Zhou S, Liao Z, Mawlawi O, Ibbott G, Court LE. Prognostic value and reproducibility of pretreatment CT texture features in stage III non-small cell lung cancer. Int J Radiat Oncol Biol Phys 2014;90:834-42. [Crossref] [PubMed]
- Vaidya P, Bera K, Gupta A, Wang X, Corredor G, Fu P, Beig N, Prasanna P, Patil P, Velu P, Rajiah P, Gilkeson R, Feldman M, Choi H, Velcheti V, Madabhushi A. CT derived radiomic score for predicting the added benefit of adjuvant chemotherapy following surgery in Stage I, II resectable Non-Small Cell Lung Cancer: a retrospective multi-cohort study for outcome prediction. Lancet Digit Health 2020;2:e116-28. [Crossref] [PubMed]
- Huang Y, Liu Z, He L, Chen X, Pan D, Ma Z, Liang C, Tian J, Liang C. Radiomics Signature: A Potential Biomarker for the Prediction of Disease-Free Survival in Early-Stage (I or II) Non-Small Cell Lung Cancer. Radiology 2016;281:947-57. [Crossref] [PubMed]
- Wang X, Duan H, Li X, Ye X, Huang G, Nie S. A prognostic analysis method for non-small cell lung cancer based on the computed tomography radiomics. Phys Med Biol 2020;65:045006. [Crossref] [PubMed]
- Huang L, Chen J, Hu W, Xu X, Liu D, Wen J, Lu J, Cao J, Zhang J, Gu Y, Wang J, Fan M. Assessment of a Radiomic Signature Developed in a General NSCLC Cohort for Predicting Overall Survival of ALK-Positive Patients With Different Treatment Types. Clin Lung Cancer 2019;20:e638-51. [Crossref] [PubMed]
- Starkov P, Aguilera TA, Golden DI, Shultz DB, Trakul N, Maxim PG, Le QT, Loo BW, Diehn M, Depeursinge A, Rubin DL. The use of texture-based radiomics CT analysis to predict outcomes in early-stage non-small cell lung cancer treated with stereotactic ablative radiotherapy. Br J Radiol 2019;92:20180228. [Crossref] [PubMed]
- Choe J, Lee SM, Do KH, Kim S, Choi S, Lee JG, Seo JB. Outcome prediction in resectable lung adenocarcinoma patients: value of CT radiomics. Eur Radiol 2020;30:4952-63. [Crossref] [PubMed]
- Zhang R, Wang C, Cui K, Chen Y, Sun F, Sun X, Xing L. Prognostic Role Of Computed Tomography Textural Features In Early-Stage Non-Small Cell Lung Cancer Patients Receiving Stereotactic Body Radiotherapy. Cancer Manag Res 2019;11:9921-30. [Crossref] [PubMed]
- Zhou L, Feng F, Yang Y, Zheng X, Yang Y. Prognostic predictors of non-small cell lung cancer treated with curative resection: the role of preoperative CT texture features, clinical features, and laboratory parameters. Clin Radiol 2022;77:e765-70. [Crossref] [PubMed]
- He BX, Zhong YF, Zhu YB, Deng JJ, Fang MJ, She YL, Wang TT, Yang Y, Sun XW, Belluomini L, Watanabe S, Dong D, Tian J, Xie D. Deep learning for predicting immunotherapeutic efficacy in advanced non-small cell lung cancer patients: a retrospective study combining progression-free survival risk and overall survival risk. Transl Lung Cancer Res 2022;11:670-85. [Crossref] [PubMed]
- Cheng J, Pan Y, Huang W, Huang K, Cui Y, Hong W, Wang L, Ni D, Tan P. Differentiation between immune checkpoint inhibitor-related and radiation pneumonitis in lung cancer by CT radiomics and machine learning. Med Phys 2022;49:1547-58. [Crossref] [PubMed]
- Han Y, Ma Y, Wu Z, Zhang F, Zheng D, Liu X, Tao L, Liang Z, Yang Z, Li X, Huang J, Guo X. Histologic subtype classification of non-small cell lung cancer using PET/CT images. Eur J Nucl Med Mol Imaging 2021;48:350-60. [Crossref] [PubMed]
- Sugai Y, Kadoya N, Tanaka S, Tanabe S, Umeda M, Yamamoto T, Takeda K, Dobashi S, Ohashi H, Takeda K, Jingu K. Impact of feature selection methods and subgroup factors on prognostic analysis with CT-based radiomics in non-small cell lung cancer patients. Radiat Oncol 2021;16:80. [Crossref] [PubMed]
- Amini M, Nazari M, Shiri I, Hajianfar G, Deevband MR, Abdollahi H, Arabi H, Rahmim A, Zaidi H. Multi-level multi-modality (PET and CT) fusion radiomics: prognostic modeling for non-small cell lung carcinoma. Phys Med Biol 2021; [Crossref] [PubMed]
- Staal FCR, Aalbersberg EA, van der Velden D, Wilthagen EA, Tesselaar MET, Beets-Tan RGH, Maas M. GEP-NET radiomics: a systematic review and radiomics quality score assessment. Eur Radiol 2022;32:7278-94. [Crossref] [PubMed]
- Amrane K, Thuillier P, Bourhis D, Le Meur C, Quere C, Leclere JC, Ferec M, Jestin-Le Tallec V, Doucet L, Alemany P, Salaun PY, Metges JP, Schick U, Abgral R. Prognostic value of pre-therapeutic FDG-PET radiomic analysis in gastro-esophageal junction cancer. Sci Rep 2023;13:5789. [Crossref] [PubMed]
- Lafcı O, Celepli P, Seher Öztekin P, Koşar PN. DCE-MRI Radiomics Analysis in Differentiating Luminal A and Luminal B Breast Cancer Molecular Subtypes. Acad Radiol 2023;30:22-9. [Crossref] [PubMed]
- Fiz F, Viganò L, Gennaro N, Costa G, La Bella L, Boichuk A, Cavinato L, Sollini M, Politi LS, Chiti A, Torzilli G. Radiomics of Liver Metastases: A Systematic Review. Cancers (Basel) 2020;12:2881. [Crossref] [PubMed]
- Lambin P, Leijenaar RTH, Deist TM, Peerlings J, de Jong EEC, van Timmeren J, Sanduleanu S, Larue RTHM, Even AJG, Jochems A, van Wijk Y, Woodruff H, van Soest J, Lustberg T, Roelofs E, van Elmpt W, Dekker A, Mottaghy FM, Wildberger JE, Walsh S. Radiomics: the bridge between medical imaging and personalized medicine. Nat Rev Clin Oncol 2017;14:749-62. [Crossref] [PubMed]
- Verma V, Sprave T, Haque W, Simone CB 2nd, Chang JY, Welsh JW, Thomas CR Jr. A systematic review of the cost and cost-effectiveness studies of immune checkpoint inhibitors. J Immunother Cancer 2018;6:128. [Crossref] [PubMed]
- Singer ME, Applegate KE. Cost-effectiveness analysis in radiology. Radiology 2001;219:611-20. [Crossref] [PubMed]
- Devlin NJ, Brooks R. EQ-5D and the EuroQol Group: Past, Present and Future. Appl Health Econ Health Policy 2017;15:127-37. [Crossref] [PubMed]
- Feng L, Liu Z, Li C, Li Z, Lou X, Shao L, et al. Development and validation of a radiopathomics model to predict pathological complete response to neoadjuvant chemoradiotherapy in locally advanced rectal cancer: a multicentre observational study. Lancet Digit Health 2022;4:e8-e17. [Crossref] [PubMed]
- Zhu W, Xie L, Han J, Guo X. The Application of Deep Learning in Cancer Prognosis Prediction. Cancers (Basel) 2020;12:603. [Crossref] [PubMed]