Glymphatic function assessment with diffusion tensor imaging along the perivascular space in patients with major depressive disorder and its relation to cerebral white-matter alteration
Introduction
Major depressive disorder (MDD), a common mental health disorder, has become a major global health problem, seriously affecting human physical and mental health (1,2). An increasing number of MDD mechanisms are being discovered, including alterations in neurotransmission, changes in neurotrophic factors, inflammation, dysregulation in the brain-gut axis, and shifts in neuroendocrine function such as in the hypothalamic-pituitary-adrenal (HPA) axis (3). Specifically, brain dysregulation includes changes in synaptic plasticity, imbalances in excitatory and inhibitory neurotransmission, and structural changes in regions such as the prefrontal cortex and hippocampus (4). Recent studies indicate that glymphatic dysfunction is one of the major triggers of MDD (5,6). The cerebral glymphatic system is a specialized drainage network whose decline in function restricts the expelling of fluids and various compounds, such as macromolecules, reactive oxygen species, and cytokines, from the interstitial fluid to the meningeal glymphatic vessels and finally to the peripheral glymphatic system (7). These compounds significantly impact the development of MDD (8,9). The glymphatic system is crucial to the brain’s immune system, as it is responsible for clearing waste from the brain waste during sleeping and plays a critical role in maintaining brain homeostasis (10). Therefore, dysfunction of the cerebral glymphatic system has been linked to various diseases (11-14).
Most preliminary studies on the human glymphatic system have employed magnetic resonance imaging (MRI) enhanced by gadolinium-based contrast agents (15,16). Intrathecal injection of contrast agents, although invasive and restricted in some countries, can indicate whether glymphatic dysfunction is occurring by demonstrating delayed clearance (15). However, administration of gadolinium-based contrast agents can result in gadolinium accumulation in the brain (17). The glymphatic system’s efficiency diminishes with age (18), and dysfunction of this system is linked to the buildup of β-amyloid and tau proteins (19). The accumulation of these proteins is associated with the neurodegenerative mechanisms of Alzheimer disease (7). Although there is ongoing research into the glymphatic system, the in vivo assessment of its function remains challenging due to the absence of nonintrusive imaging methods in quantifying glymphatic activity.
The glymphatic system is composed of various structures, such as the perivascular space (PVS), interstitial space, arachnoid membrane, and meningeal glymphatic vessels (10). However, detecting the morphological changes of these structures in human bodies can be challenging (20). The PVS, which contains glymphoid fluid around perforating vessels, is the only glymphatic system visible on MRI (10). Previous studies have examined the link between observable glymphatic dysfunction and psychological trauma in patients with depression (21-23). Recently, diffusion tensor imaging (DTI), specifically, the DTI along the PVS (DTI-ALPS) index, has been proposed as a noninvasive alternative method for partially assessing glymphatic system function. DTI-ALPS assesses the diffusion rate of water within the PVS by using the vertical orientation of medullary veins (x-axis), association fibers (y-axis), and projection fibers (z-axis) at lateral ventricular body level (24). By considering the primary difference between the apparent diffusivity within the x-axis and the diffusion coefficient perpendicular to PVS (y-axis and z-axis) as being indicative of PVS flow, the ALPS method can partially evaluate glymphatic system. The DTI-ALPS index score has been observed to be reduced in various conditions including those with idiopathic normal pressure hydrocephalus (25,26), multiple sclerosis (12), Alzheimer disease (27), or traumatic brain injury (13,28). In addition, a growing body of evidence suggests there being a link between the alteration of white-matter and depression, and thus white-matter microstructure changes have been considered to be a potential biomarker for MDD (29,30). Carotenuto et al. demonstrated the association between glymphatic system damage and white-matter microstructure impairment in patients with multiple sclerosis, which may indicate a close interaction between these processes (12). It has been speculated that choroid plexus volume drives glymphatic function. Bravi et al. demonstrated that an increase in choroid plexus volume in patients with bipolar disorder (BP) and MDD was correlated with circulating inflammatory cytokines (31). However, to our knowledge, there are no reports regarding the connection between DTI-ALPS score and white-matter alteration in patients with MDD.
In this study, patients with MDD were compared with healthy controls (HC) in terms of glymphatic function as assessed by DTI-ALPS, whose results were then correlated with other MRI indexes including white-matter abnormalities and neuropsychological scale scores. We present this article in accordance with the STROBE reporting checklist (available at https://qims.amegroups.com/article/view/10.21037/qims-24-510/rc).
Methods
Study participants
This prospective study was conducted in accordance with the Declaration of Helsinki (as revised in 2013) and approved by the Institutional Review Board of the First Affiliated Hospital of Dalian Medical University (No. PJ-KS-KY-2023-421). All participants signed informed consent forms. We prospectively recruited consecutive patients from the Psychosomatic Diseases Department of the First Affiliated Hospital of Dalian Medical University from February 2023 to November 2023. The inclusion criteria for patients were as follows: (I) meeting the diagnostic criteria for depression of the Diagnostic and Statistical Manual of Mental Disorders, Fifth Edition, (II) a Hamilton Depression Rating Scale (HAMD) 17 score >7 points, (III) age older than 18 years old, and (IV) right-handedness. Meanwhile, the exclusion criteria were as follows: (I) a medical history of other mental illnesses or symptoms, (II) organic lesions in the brain (such as concussion, stroke, infarction, tumor, or neuroinflammatory disease), (III) a history of long-term chronic disease (such as autoimmune disease, chronic kidney disease, liver disease, or heart disease), (IV) conditions that could affect cerebral blood flow or metabolism (such as hypertension), and (V) contraindications to MR scanning. A total of 35 consecutive patients with MDD were enrolled in the study. After recruitment was completed, all participants underwent MRI examination.
Additionally, control participants were recruited from the community and were matched with MDD cohort in terms of years of education, gender, and age. The inclusion criteria for the HCs were as follows: (I) age older than 18 years, (II) right-handedness, and (III) the ability to independently complete neuropsychological scales. Meanwhile, the exclusion criteria for HCs were as follows: (I) HAMD-17 score >7 points; (II) previous or current diagnosis of any mental illness; and (III) severe physical diseases, such as severe cardiovascular and cerebrovascular diseases. The HC group provided a necessary baseline against which we could compare the MRI results of the patients with MDD, as the absence of mental illness and cardiovascular diseases in the HC group allowed us to control for potential confounding factors. This control ensured that the differences observed in the MRI scans could be more reliably attributed to MDD rather than other underlying conditions.
MRI scanning
With a 32-channel phased-array head coil, the MRI scans were conducted on a 3-T MRI scanner (Ingenia CX, Philips, Amsterdam, the Netherlands). Three-dimensional (3D) T1-weighted imaging (3D-T1WI) images were acquired using a multishot turbo field echo (MS-TFE) sequence. The scanning parameters were as follows: repetition time (TR), 6.6 ms; echo time (TE), 3.0 ms; flip angle, 12°; matrix size, 256×256; field of view (FOV), 256 mm × 256 mm; number of slices, 188; and slice thickness, 1.0 mm. DTI imaging was conducted using a single-shot echo-planar imaging (SS-EPI). At b=1,000 s/mm2, 64 directions were used, with 1 baseline image acquired at b=0 s/mm2. The other scanning parameters were as follows: TR, 6,000 ms; TE, 92 ms; flip angle, 90°; matrix size, 128×128; FOV, 256 mm × 256 mm; number of axial slices, 68; and slice thickness, 2 mm.
DTI data preprocessing
The FMRIB Software Library (FSL) toolbox was used to compute diffusion parameters. Prior to the analysis, image quality was assessed by a professional neuroimaging radiologist, and images with motion artifacts were excluded. Distortions caused by eddy currents 9r motion artifacts were corrected (32). We produced maps of mean diffusivity (MD), fractional anisotropy (FA), Dxx, Dyy, Dzz, λ1, λ2, and λ3. From these characteristic values, axial diffusivity (AD; AD = λ1) maps were generated. Radial diffusivity (RD) [RD = (λ2 + λ3)/2] maps were subsequently produced.
Tract-based spatial statistics (TBSS) analysis
The TBSS suite from FSL was applied to draw the FA maps (33). We mapped the registered FA maps on this skeleton. Subsequently, the AD, MD, and RD maps were subjected to the same FA transformation, which included non-linear registration to a common space followed by skeletonization. For FA, AD, MD, and RD voxel-wise comparison, personal skeletonized images were fed into the general linear model (GLM), which included education years, gender, and age as covariates, and was conducted with 5,000 permutations. Multiple-comparisons correction was applied to the family-wise error (FWE) rate and threshold-free cluster enhancement (TFCE) (34,35). Statistically different clusters (P<0.05) were identified using the FSL cluster tool. Finally, we extracted the DTI parameter values for each significant cluster from the skeletal TBSS images of each participant.
Automated fiber quantification (AFQ) analysis
The preprocessing of 3D-T1WI images was performed using FSL software. First, the Brain Extraction Tool (BET) was used to perform brain stripping on 3D structural images and remove nonbrain structures. Second, the images from 3D-T1WI were averaged, rotated, and aligned with the anterior-posterior commissure (AC-PC). At the end, the dtiMakeDt6FromFSL script was employed to align the images from 3D-T1WI with the S0 image, resulting in a dt6 MATLAB format file. In the Linux operating (VM VirtualBox, Oracle Corp., Austin, TX, USA) environment, we performed AFQ processing (36).
DTI-ALPS analysis
The DTI-ALPS index calculation approach is outlined on Figure 1. We generated an FA color map and selected a transverse plane where veins were orthogonal to the lateral ventricle, aligning the PVS along the x-axis. Given that our participants were all right-handed, we positioned the regions of interest (ROIs) in the left cerebral hemisphere (27). Two neuroradiologists, with 4 and 11 years of experience, respectively, who were blinded to the clinical diagnosis, independently measured the DTI-ALPS index. On the FA color map, three ROIs with a 2.5-mm radius were drawn in the regions of the projection fiber, association fiber, and subcortical fiber (Figure 1). The placement of ROIs was manually verified for each participant.
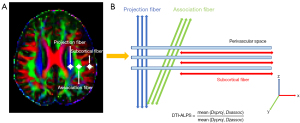
A total of nine individual DTI-ALPS measurements were automatically generated from nine ROIs: (I) Dx for three nerve fiber regions, including projection fiber (Dxproj), association fiber (Dxassoc), and subcortical fiber (Dxsubc); (II) Dy for three nerve fiber regions (Dyproj, Dyassoc, Dysubc); and (III) Dz for three nerve fiber regions (Dzproj, Dzassoc, Dzsub). We used a program named “dpabi”, based on MATLAB (version 2013b), to calculate the DTI-ALPS index. We used the average value of two neuroradiologists for subsequent statistical analysis.
Neuropsychological assessment
An experienced neuropsychologist who was unaware of the group assignments administered three neuropsychological tests to all participants, including the Montreal Cognitive Assessment (MoCA), the HAMD-17 (37), and the Hamilton Anxiety Rating Scale (HAMA), which were completed within 24 hours prior to MRI examinations. HAMD and HAMA are the most commonly used scales in clinical practice to assess depression and anxiety. The more severe the condition is, the higher the HAMD and HAMA scores. MoCA is an assessment tool used for the rapid screening of cognitive dysfunction. The milder the condition is, the higher the MoCA score.
Statistical analysis
SPSS v. 26.0 (IBM Corp., Armonk, NY, USA) was used to conduct the statistical analyses. Depending on the distribution of the variables, either the Mann-Whitney U test or two-sample t-test was applied for comparison. Chi-square (X2) tests were employed to compare count data. The interobserver consistency of DTI-ALPS measurements between two readers was evaluated with intraclass correlation coefficient (ICC). ICC exceeding 0.75 indicated the excellent reliability, 0.4–0.75 fair reliability, and those lower than 0.4 poor reliability (38). The relation between the DTI-ALPS index, TBSS, AFQ, and neuropsychological assessment results were determined via partial correlation analyses, which included education years, gender, and age. Furthermore, we used linear regression analysis to determine the correlation between the DTI-ALPS index, HAMD, HAMA, and MoCA results, controlling for education years, gender, and age. A two-tailed P value <0.05 was considered to indicate statistical significance.
Using the AFQ method for statistical analysis, we evaluated the mean DTI for each fiber tract were evaluated through the Mann-Whitney tests or two-sample t-test. The false discovery rate (FDR) method was used to adjust for multiple testing. The “Randomize” command in FSL was used, and the covariates of years of education, gender, and age were controlled for in the GLM model for node-wise analysis. Nonparametric statistical analysis using permutation testing with FWE correction was conducted based on 5,000 permutations and significant differences at P<0.05 were reported for three or more adjacent nodes.
Results
Participant characteristics
Figure 2 summarizes the inclusion process. Overall, 58 participants were enrolled, comprising 35 patients with MDD [11 men and 24 women; median age 53 years, interquartile range (IQR) 48–64 years] and 23 HCs (9 men and 14 women; median age 55 years, IQR 51–58 years). Between patients with MDD and HCs, no significant differences were observed in years of education (z=−1.336; P=0.182), gender (X2=0.364; P=0.546), or age (z=−1.306; P=0.192). However, patients with MDD had worse scores in the MoCA and higher HAMD and HAMA scores (Table 1). The participants’ other characteristics are presented in Table 1.
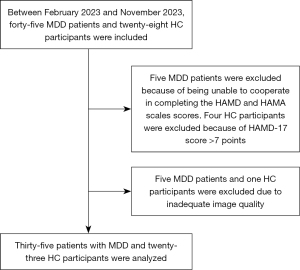
Table 1
Variable | MDD | HC | t/Z/X2 | P value |
---|---|---|---|---|
No. | 35 | 23 | ||
Age (years) | 53 (48, 64) | 55 (51, 58) | −1.306 | 0.192 |
Female gender | 24 [69] | 14 [61] | 0.364 | 0.546 |
Education (years) | 12 (12, 12) | 12 (9, 15) | −1.336 | 0.182 |
MoCA total score | 23.31±3.13 | 26.78±1.86 | −5.287 | <0.001 |
Executive/visuospatial | 3 (2, 4) | |||
Naming | 3 (3, 3) | |||
Delayed memory | 1.5 (0, 3) | |||
Attention | 5 (5, 6) | |||
Language | 2 (2, 3) | |||
Abstraction | 2 (1, 2) | |||
Orientation | 6 (6, 6) | |||
HAMA total score | 23.60±5.05 | 2.91±2.27 | 21.172 | <0.001 |
Somatic anxiety | 10.69±3.64 | |||
Psychic anxiety | 12.91±2.53 | |||
HAMD total score | 18.37±4.17 | 4.48±1.62 | 17.763 | <0.001 |
Anxiety/somatization | 6.29±1.51 | |||
Weight | 0 (0, 1) | |||
Cognitive impairment | 2 (1, 3) | |||
Diurnal variation | 0 (0, 1) | |||
Retardation | 2.91±1.84 | |||
Sleep disturbance | 5 (3, 6) | |||
A sense of despair | 0 (0, 0) | |||
General somatic symptoms | 1 (1, 2) |
Values are reported as the mean ± SD or median (interquartile range) for the quantitative variables and as frequency (percentage) for the categorical variables. MDD, major depressive disorder; HC, healthy control; IQR, interquartile range; MoCA, Montreal Cognitive Assessment; SD, standard deviation; HAMA, Hamilton Anxiety Rating Scale, HAMD, Hamilton Depression Rating Scale.
TBSS results
We found two significantly different clusters in MD values, one significantly different cluster in RD and AD values between the MDD and HC groups. Figure 3 and Table 2 present the detailed post hoc analysis locations for the HC and MDD groups.
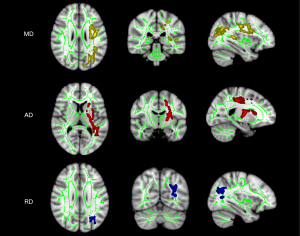
Table 2
Cluster | JHU WM tractography atlas | Voxel coordinates of local maxima (MNI coordinates) | Voxel | t score | P value | ||
---|---|---|---|---|---|---|---|
X | Y | Z | |||||
MD (cluster1) | Anterior thalamic radiation L | 115 | 110 | 105 | 2258 | −2.19 | 0.033 |
Corticospinal tract L | |||||||
Superior longitudinal fasciculus L | |||||||
Uncinate fasciculus L | |||||||
Superior longitudinal fasciculus (temporal part) L | |||||||
MD (cluster2) | Anterior thalamic radiation L | 122 | 46 | 86 | 3382 | −2.19 | 0.033 |
Cingulum (cingulate gyrus) L | |||||||
Cingulum (hippocampus) L | |||||||
Forceps major | |||||||
Inferior fronto-occipital fasciculus L | |||||||
Inferior longitudinal fasciculus L | |||||||
Superior longitudinal fasciculus L | |||||||
Superior longitudinal fasciculus (temporal part) L | |||||||
AD | Anterior thalamic radiation L | 111 | 116 | 81 | 3403 | −2.43 | 0.017 |
Corticospinal tract L | |||||||
Forceps major: 0.0570085 | |||||||
Inferior fronto-occipital fasciculus L | |||||||
Inferior longitudinal fasciculus L | |||||||
Superior longitudinal fasciculus L | |||||||
Uncinate fasciculus L | |||||||
Superior longitudinal fasciculus (temporal part) L | |||||||
RD | Anterior thalamic radiation L | 123 | 61 | 100 | 582 | −2.16 | 0.044 |
Forceps major | |||||||
Inferior fronto-occipital fasciculus L | |||||||
Inferior longitudinal fasciculus L | |||||||
Superior longitudinal fasciculus L | |||||||
Superior longitudinal fasciculus (temporal part) L |
The cluster with low voxels (<100) has been excluded. MD, mean diffusivity; AD, axial diffusivity; RD, radial diffusivity; MDD, major depressive disorder; HC, healthy control; JHU WM tractography atlas, John Hopkins University white-matter tractography atlas; MNI, Montreal Neurological Institute; L, left hemisphere; R, the right hemisphere.
AFQ results
For the results of mean diffusion differences at the tract level, MDD group showed significantly increased AD values in left cingulum bundle (CB_L), left inferior longitudinal fasciculus (ILF_L), and left uncinate fasciculus (UF_L) (Table 3). For diffusion differences at the point-wise level, significant alterations were mainly observed in the AD, MD, and RD values; the details are shown in Figure 4.
Table 3
Parameter | MDD | HC | t/Z | P value | FDR-P |
---|---|---|---|---|---|
CB_L | 1.16±0.05 | 1.11±0.07 | 3.29 | 0.002 | 0.023 |
ILF_L | 1.19 (1.18, 1.21) | 1.14 (1.13, 1.17) | −5.20 | 0.004 | 0.023 |
UF_L | 1.20±0.06 | 1.15±0.04 | 3.31 | 0.002 | 0.023 |
Values are reported as the mean ± SD or median (interquartile range). AD values are presented in units of ×10−3 mm2∙s−1. AD, axial diffusivity; AFQ, automated fiber quantification; MDD, major depressive disorder; HC, healthy control; FDR, false discovery rate; CB_L, left cingulum bundle; ILF_L, left inferior longitudinal fasciculus; UF_L, left uncinate fasciculus.
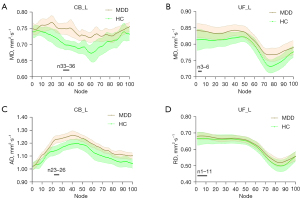
DTI-ALPS measurements
The interobserver agreement for the DTI-ALPS index demonstrated excellent reliability, as indicated by an ICC of 0.844, with a 95% confidence interval (CI) ranging from 0.750 to 0.905. The average DTI-ALPS score for two groups is shown in Table 4. The patients with MDD had markedly reduced DTI-ALPS scores as compared to the HCs (P=0.001).
Table 4
Parameter | MDD | HC | t/Z | P value |
---|---|---|---|---|
DTI-ALPS index | 1.45±0.11 | 1.57±0.13 | −3.50 | 0.001 |
Values are reported as the mean ± SD. DTI-ALPS, diffusion tensor imaging along the perivascular space; TBSS, tract-based spatial statistics; MDD, major depressive disorder; HC, healthy control; SD, standard deviation.
Relationships between diffusion parameters and the DTI‑ALPS index
After adjustments were made for years of education, gender, and age, a partial correlation analysis of the DTI-ALPS index scores and TBSS revealed statistical differences (Figure 5). AD, MD, and RD values were found to have a negative correlation with the DTI-ALPS index (Figure 5). For mean diffusion differences at the tract level of AFQ analysis, the DTI-ALPS index showed a negative correlation with AD values of the CB_L, ILF_L, and UF_L (Figure 6). For diffusion differences at the point-wise level of AFQ analysis, the MD values of CB_L exhibited a negative correlation with the DTI-ALPS index (Figure 7).
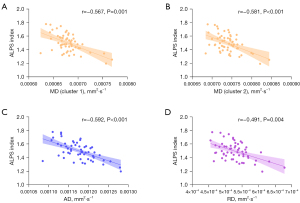
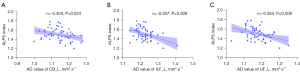
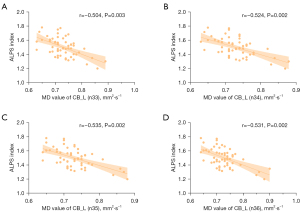
Associations among diffusion parameters and DTI-ALPS index with the HAMD, HAMA scale, and MoCA scales
The results regarding the associations between DTI-ALPS and HAMD scale, HAMA scale, and cognitive test results are summarized in Table 5. Linear regression analysis revealed that the DTI-ALPS index can be used as an independent predictor of the HAMD, HAMA, and MoCA scores. In the MDD group, DTI-ALPS score was inversely associated with the retardation score (r=−0.432; P=0.013). The DTI-ALPS index was positively associated with the MoCA total score in all participants (r=0.405; P=0.021) and was positively associated with orientation score in the MDD group (r=0.468; P=0.007). Among associations between TBSS results and the different scales, the abstraction score was significantly correlated with MD value (cluster 1: r=−0.381, P=0.031; cluster 2: r=−0.465, P=0.007) and RD value score (r=−0.441; P=0.012). Among the associations between the AFQ results and the different scales, mean AD value of the CB_L was significantly and positively correlated with the attention score (r=0.365; P=0.040). The MD value of the CB_L was positively correlated with retardation (n33: r=0.439, P=0.012; n34: r=0.434, P=0.013; n35: r=0.410, P=0.020; n36: r=0.373, P=0.036).
Table 5
Neuropsychological scores | DTI-ALPS index | ||||
---|---|---|---|---|---|
B | β | 95% CI | t | P value | |
HAMD | −25.32 | −0.44 | (−40.35, −11.55) | −3.38 | 0.001 |
HAMA | −33.48 | −0.40 | (−55.38, −11.24) | −3.07 | 0.003 |
MoCA | 8.59 | 0.35 | (2.38, 14.79) | 2.78 | 0.008 |
DTI-ALPS, diffusion tensor imaging along the perivascular space; B, unstandardized coefficient; β, standardized coefficient; CI, confidence interval; HAMD, Hamilton Depression Rating Scale; HAMA, Hamilton Anxiety Rating Scale; MoCA, Montreal Cognitive Assessment.
Discussion
In this study, we found a decrease in the DTI-ALPS index score and white-matter integrity alteration in patients with MDD. Moreover, impairment of glymphatic function, as partially indicated by the DTI-ALPS index, was correlated with white-matter injury, retardation, and cognitive dysfunction in patients with MDD.
There is a growing body of research related to the glymphatic function in neurodegenerative diseases and neuropsychiatric diseases (24,39), but MRI assessment of glymphatic function remains challenging. DTI-ALPS, a method of noninvasive quantitative research, was proposed to analyze glymphatic function (12,13,25,27,40,41). The lower water diffusivity in the space surrounding the blood vessels was demonstrated to reflect the severity of Alzheimer disease, confirming the practicability of DTI-ALPS (24). Whereas, considerable debate remains regarding the extent to which the DTI-ALPS index truly represents glymphatic function. Wright et al. discovered that a DTI-ALPS index score greater than 1 could be due to the projection and association fiber tracts’ radial imbalance, thus not exclusively arising from the diffusivity within the PVS. They further emphasized that the ALPS index might not only indicate modifications in the PVS diffusivity but also contributions from axons (42). However, the λ2:λ3 was relatively under-investigated and not considered in studies of brain white-matter, and there is a lack of research on the exact properties of signals. Nonetheless, DTI-ALPS and intrathecal injection of contrast agents were used for glymphatic analysis in a prior study involving humans, confirming the effectiveness of DTI-ALPS (43), and the possible sensitivity of the DTI-ALPS index to perivascular diffusion warranted recognition. The study also showed that the DTI-ALPS index is linked to delayed clearance of glymphatic system (43). Compared to the circulatory system, the glymphatic system is more intricate. The DTI-ALPS and other alternative approaches can only assess limited facets of glymphatic function with no single method providing comprehensive evaluation of function (44). Although we used one evaluation method, the DTI-ALPS index has gained popularity, and many researchers now recognize it. The DTI-ALPS method as one of the several assessment techniques can continue to provide useful information. In addition, several published studies have demonstrated high internal and interobserver consistency and strong repeatability of DTI-ALPS measurements under fixed imaging parameters (25,43,45). Our results also confirmed the consistency of the measurement results between two readers. Therefore, we can use the DTI scan in a few minutes to calculate the DTI-ALPS index and partially characterize glymphatic function.
There is a dearth of literature on the use of DTI in patients with MDD to measure glymphatic phenomena. Previous animal experimental studies have shown that depression can lead to damage to glymphatic system function (5,46). Using a depressive mouse model, Xia et al. showed that glymphatic system dysfunction is significantly impaired due to accumulation of Aβ42 in the brain parenchyma through intracisternal tracer infusions (5). Liu et al. reported that chronic treatment in an unpredictable mild stress depressive model led to inhibition of the glymphatic system (46). DTI-ALPS has been used in various neurodegenerative diseases and has been proven to be related to clinical scores (25). Consequently, the DTI-ALPS index is also applicable to patients with MDD in whom glymphatic function needs to be assessed with regard to depression and cognitive performance scores.
The DTI-ALPS index measures diffusion of vertical projection fibers and associated fibers from the compartment in the spatial direction surrounding the PVS, thus reflecting the state of the glymphatic system function. A higher DTI-ALPS index score indicates greater water diffusion along the PVS and thus better glymphatic function. In our study, the patients with MDD displayed a lower DTI-ALPS index score relative to HCs, partially reflecting this glymphatic system impairment. Additionally, an inverse association between the DTI-ALPS index and retardation scores in patients with MDD was found, with higher index scores indicating more severe disability. This finding serves as evidence supporting the involvement of the glymphatic system in the development of MDD, suggesting poor brain waste clearance might result in retardation. In assessing cognitive impairment, we discovered a reduced DTI-ALPS index score was linked to poorer MoCA scores in terms of orientation in patients with MDD, indicating that these cognitive aspects are more vulnerable in patients with MDD. Previous studies have shown that one of the risk elements for Alzheimer disease is depression (47). The findings from linear regression analysis demonstrated that the DTI-ALPS index was capable of independently predicting abnormal HAMD, HAMA, and MoCA scores associated with symptoms of depression, anxiety, and cognitive decline.
A link between glymphatic system changes and white-matter microstructural alterations has been established. These white-matter microstructural alterations are linked to the development and relapse of MDD (48). In our study, the AD, MD, and RD values in TBSS analysis were increased in patients with MDD, especially in left corticospinal tract, left anterior thalamic radiation, left inferior fronto-occipital fasciculus, left superior longitudinal fasciculus, and ILF_L in comparison with those of HC group. These regions are involved in executive functioning, emotional regulation, and reward processing, all of which are related to depression (49,50). AD, MD, and RD values exhibited an inverse association with the DTI-ALPS index. Despite TBSS being a voxel-level method, it still cannot provide specific information about white-matter integrity along individual fiber bundles (51). When the average measurement along each fiber is not clear, this fiber-oriented automatic quantification method offers detailed insights into diffusion parameters. When the average measurements are not straightforward, this fiber-oriented automatic quantification method quantifies the diffusion indicators along the fiber bundles at anatomically equivalent positions. For AFQ analysis, the alterations of white-matter fiber bundles primarily take place in the CB_L, ILF_L, and UF_L, especially in n33–36 of the CB_L. Our results suggest that a change in n33–36 of the CB_L is associated with symptoms of retardation. Zhang et al. also found significant differences in the CB_L between patients without suicidality and patients with suicidality (52). However, in our study, we did not find significant differences in the right anterior thalamic radiation and corpus callosum. This lack of observed difference might be due to the fact that the previous study exclusively analyzed patients with suicidality. Due to damage to the glymphatic system, the interstitial clearance rate decreases, which might cause tissue irregularities by facilitating neuronal loss, inflammation reaction, and glial proliferation (10,53). Further research should be conducted on the complex interactions among AD, MD, RD values, DTI-ALPS score, and cognitive impairment, with years of education years, gender, and age being controlled for. We speculate that the abnormal accumulation of β-amyloid and tau protein can exacerbate the widespread white-matter damage induced by glymphatic dysfunction (54). In patients with Alzheimer disease, the spatial correlation between β-amyloid and tau protein accumulation and white-matter damage have been demonstrated (55,56). In our study, the DTI parameters in certain clusters were significantly associated with the DTI-ALPS index, indicating that damage to the glymphatic system induced the increased fragility of white-matter in patients with MDD. Although, RD and MD values showed a significant correlation with the abstraction score, no association was found between AD value and MoCA total score, HAMD total score, and HAMA total score. Zhang et al. suggested that the MD values of the right anterior thalamic radiation was positively associated with HAMD score, which contrasts with our findings (52). We speculate that this may be due to the fact that patients with depression were at different stages of onset. In the future, we will perform studies on different stages of the disease course. In addition, unlike in the study of Zhang et al., the FA values of the MDD group did not significantly decrease compared to the HC group. Constant FA values may suggest that water molecule diffusion in the patient’s body encounters less hindrance, which is potentially caused by a decrease in the numbers of dendrites or axons with no loss of neurons (57).
Our study involved several limitations which should be addressed. First, due to being a preliminary study on DTI-ALPS in patients with MDD and having a small sample size, our study did not employ grouping according to the severity of depression. Further research will be conducted that investigates sensitivity. Moreover, we only drew ROI on the selected layer, and in future studies, ROIs will be drawn on both sides at multiple levels to more comprehensively reflect glymphatic function. Furthermore, glymphatic function may be influenced by various physiological factors, but the assessment of glymphatic system function was conducted using a single method. Therefore, we will endeavor to introduce a variety of approaches to comprehensively analyze glymphatic system function in patients with MDD.
Conclusions
The DTI-ALPS index score was confirmed to be decreased in patients with MDD, which may partially indicate the impairment of glymphatic system function. The DTI-ALPS index was linked to retardation and a decline in cognitive ability. We also found associations between a low DTI-ALPS index score and white-matter microstructural abnormalities. Thus, the DTI-ALPS could serve as a potential biomarker for glymphatic system function in patients with MDD. Nevertheless, its sensitivity and practicality at the onset of MDD necessitates further investigation using a multiparametric MRI approach.
Acknowledgments
Funding: This work was supported in part by
Footnote
Reporting Checklist: The authors have completed the STROBE reporting checklist. Available at https://qims.amegroups.com/article/view/10.21037/qims-24-510/rc
Conflicts of Interest: All authors have completed the ICMJE uniform disclosure form (available at https://qims.amegroups.com/article/view/10.21037/qims-24-510/coif). The authors have no conflicts of interest to declare.
Ethical Statement: The authors are accountable for all aspects of the work in ensuring that questions related to the accuracy or integrity of any part of the work are appropriately investigated and resolved. This study was conducted in accordance with the Declaration of Helsinki (as revised in 2013) and was approved by the Institutional Review Board of the First Affiliated Hospital of Dalian Medical University (No. PJ-KS-KY- 2023- 421). Informed consent was obtained from all individual participants.
Open Access Statement: This is an Open Access article distributed in accordance with the Creative Commons Attribution-NonCommercial-NoDerivs 4.0 International License (CC BY-NC-ND 4.0), which permits the non-commercial replication and distribution of the article with the strict proviso that no changes or edits are made and the original work is properly cited (including links to both the formal publication through the relevant DOI and the license). See: https://creativecommons.org/licenses/by-nc-nd/4.0/.
References
- Zou ML, Li MX, Cho V. Depression and disclosure behavior via social media: A study of university students in China. Heliyon 2020;6:e03368. [Crossref] [PubMed]
- Malhi GS, Mann JJ. Depression. Lancet 2018;392:2299-312. [Crossref] [PubMed]
- Maletic V, Robinson M, Oakes T, Iyengar S, Ball SG, Russell J. Neurobiology of depression: an integrated view of key findings. Int J Clin Pract 2007;61:2030-40. [Crossref] [PubMed]
- Filatova EV, Shadrina MI, Slominsky PA. Major Depression: One Brain, One Disease, One Set of Intertwined Processes. Cells 2021;10:1283. [Crossref] [PubMed]
- Xia M, Yang L, Sun G, Qi S, Li B. Mechanism of depression as a risk factor in the development of Alzheimer's disease: the function of AQP4 and the glymphatic system. Psychopharmacology (Berl) 2017;234:365-79. [Crossref] [PubMed]
- Chakraborty S. ThimmaReddygari J, Selvaraj D. G-Lymphatic, Vascular and Immune Pathways for Aβ Clearance Cascade and Therapeutic Targets For Alzheimer's Disease. Comb Chem High Throughput Screen 2021;24:1083-92. [Crossref] [PubMed]
- Harrison IF, Ismail O, Machhada A, Colgan N, Ohene Y, Nahavandi P, Ahmed Z, Fisher A, Meftah S, Murray TK, Ottersen OP, Nagelhus EA, O'Neill MJ, Wells JA, Lythgoe MF. Impaired glymphatic function and clearance of tau in an Alzheimer's disease model. Brain 2020;143:2576-93. [Crossref] [PubMed]
- Lindqvist D, Dhabhar FS, James SJ, Hough CM, Jain FA, Bersani FS, Reus VI, Verhoeven JE, Epel ES, Mahan L, Rosser R, Wolkowitz OM, Mellon SH. Oxidative stress, inflammation and treatment response in major depression. Psychoneuroendocrinology 2017;76:197-205. [Crossref] [PubMed]
- Slavich GM, Sacher J. Stress, sex hormones, inflammation, and major depressive disorder: Extending Social Signal Transduction Theory of Depression to account for sex differences in mood disorders. Psychopharmacology (Berl) 2019;236:3063-79. [Crossref] [PubMed]
- Louveau A, Smirnov I, Keyes TJ, Eccles JD, Rouhani SJ, Peske JD, Derecki NC, Castle D, Mandell JW, Lee KS, Harris TH, Kipnis J. Structural and functional features of central nervous system lymphatic vessels. Nature 2015;523:337-41. [Crossref] [PubMed]
- Hong H, Hong L, Luo X, Zeng Q, Li K, Wang S, Jiaerken Y, Zhang R, Yu X, Zhang Y, Lei C, Liu Z, Chen Y, Huang P, Zhang M. The relationship between amyloid pathology, cerebral small vessel disease, glymphatic dysfunction, and cognition: a study based on Alzheimer's disease continuum participants. Alzheimers Res Ther 2024;16:43. [Crossref] [PubMed]
- Carotenuto A, Cacciaguerra L, Pagani E, Preziosa P, Filippi M, Rocca MA. Glymphatic system impairment in multiple sclerosis: relation with brain damage and disability. Brain 2022;145:2785-95. [Crossref] [PubMed]
- Yang DX, Sun Z, Yu MM, Zou QQ, Li PY, Zhang JK, Wu X, Li YH, Wang ML. Associations of MRI-Derived Glymphatic System Impairment With Global White Matter Damage and Cognitive Impairment in Mild Traumatic Brain Injury: A DTI-ALPS Study. J Magn Reson Imaging 2024;59:639-47. [Crossref] [PubMed]
- Xu D, Zhou J, Mei H, Li H, Sun W, Xu H. Impediment of Cerebrospinal Fluid Drainage Through Glymphatic System in Glioma. Front Oncol 2021;11:790821. [Crossref] [PubMed]
- Lee MK, Cho SJ, Bae YJ, Kim JM. MRI-Based Demonstration of the Normal Glymphatic System in a Human Population: A Systematic Review. Front Neurol 2022;13:827398. [Crossref] [PubMed]
- Ringstad G, Vatnehol SAS, Eide PK. Glymphatic MRI in idiopathic normal pressure hydrocephalus. Brain 2017;140:2691-705. [Crossref] [PubMed]
- Nguyen NC, Molnar TT, Cummin LG, Kanal E. Dentate Nucleus Signal Intensity Increases Following Repeated Gadobenate Dimeglumine Administrations: A Retrospective Analysis. Radiology 2020;296:122-30. [Crossref] [PubMed]
- Zhou Y, Cai J, Zhang W, Gong X, Yan S, Zhang K, Luo Z, Sun J, Jiang Q, Lou M. Impairment of the Glymphatic Pathway and Putative Meningeal Lymphatic Vessels in the Aging Human. Ann Neurol 2020;87:357-69. [Crossref] [PubMed]
- Xu Z, Xiao N, Chen Y, Huang H, Marshall C, Gao J, Cai Z, Wu T, Hu G, Xiao M. Deletion of aquaporin-4 in APP/PS1 mice exacerbates brain Aβ accumulation and memory deficits. Mol Neurodegener 2015;10:58. [Crossref] [PubMed]
- Rasmussen MK, Mestre H, Nedergaard M. The glymphatic pathway in neurological disorders. Lancet Neurol 2018;17:1016-24. [Crossref] [PubMed]
- Ranti DL, Warburton AJ, Rutland JW, Dullea JT, Markowitz M, Smith DA, Kligler SZK, Rutter S, Langan M, Arrighi-Allisan A, George I, Verma G, Murrough JW, Delman BN, Balchandani P, Morris LS. Perivascular spaces as a marker of psychological trauma in depression: A 7-Tesla MRI study. Brain Behav 2022;12:e32598. [Crossref] [PubMed]
- Liang Y, Chan YL, Deng M, Chen YK, Mok V, Wang F, Ungvari GS, Chu CW, Tang WK. Enlarged perivascular spaces in the centrum semiovale are associated with poststroke depression: A 3-month prospective study. J Affect Disord 2018;228:166-72. [Crossref] [PubMed]
- Zhang D, Li X, Li B. Glymphatic System Dysfunction in Central Nervous System Diseases and Mood Disorders. Front Aging Neurosci 2022;14:873697. [Crossref] [PubMed]
- Zhang X, Wang Y, Jiao B, Wang Z, Shi J, Zhang Y, Bai X, Li Z, Li S, Bai R, Sui B. Glymphatic system impairment in Alzheimer's disease: associations with perivascular space volume and cognitive function. Eur Radiol 2024;34:1314-23. [Crossref] [PubMed]
- Bae YJ, Choi BS, Kim JM, Choi JH, Cho SJ, Kim JH. Altered glymphatic system in idiopathic normal pressure hydrocephalus. Parkinsonism Relat Disord 2021;82:56-60. [Crossref] [PubMed]
- Yokota H, Vijayasarathi A, Cekic M, Hirata Y, Linetsky M, Ho M, Kim W, Salamon N. Diagnostic Performance of Glymphatic System Evaluation Using Diffusion Tensor Imaging in Idiopathic Normal Pressure Hydrocephalus and Mimickers. Curr Gerontol Geriatr Res 2019;2019:5675014. [Crossref] [PubMed]
- Taoka T, Masutani Y, Kawai H, Nakane T, Matsuoka K, Yasuno F, Kishimoto T, Naganawa S. Evaluation of glymphatic system activity with the diffusion MR technique: diffusion tensor image analysis along the perivascular space (DTI-ALPS) in Alzheimer's disease cases. Jpn J Radiol 2017;35:172-8. [Crossref] [PubMed]
- Park JH, Bae YJ, Kim JS, Jung WS, Choi JW, Roh TH, You N, Kim SH, Han M. Glymphatic system evaluation using diffusion tensor imaging in patients with traumatic brain injury. Neuroradiology 2023;65:551-7. [Crossref] [PubMed]
- Parker N, Cheng W, Hindley GFL, Parekh P, Shadrin AA, Maximov II, Smeland OB, Djurovic S, Dale AM, Westlye LT, Frei O, Andreassen OA. Psychiatric disorders and brain white matter exhibit genetic overlap implicating developmental and neural cell biology. Mol Psychiatry 2023;28:4924-32. [Crossref] [PubMed]
- Sun H, Yan R, Hua L, Xia Y, Huang Y, Wang X, Yao Z, Lu Q. Based on white matter microstructure to early identify bipolar disorder from patients with depressive episode. J Affect Disord 2024;350:428-34. [Crossref] [PubMed]
- Bravi B, Melloni EMT, Paolini M, Palladini M, Calesella F, Servidio L, Agnoletto E, Poletti S, Lorenzi C, Colombo C, Benedetti F. Choroid plexus volume is increased in mood disorders and associates with circulating inflammatory cytokines. Brain Behav Immun 2024;116:52-61. [Crossref] [PubMed]
- Andersson JLR, Sotiropoulos SN. An integrated approach to correction for off-resonance effects and subject movement in diffusion MR imaging. Neuroimage 2016;125:1063-78. [Crossref] [PubMed]
- Smith SM, Jenkinson M, Woolrich MW, Beckmann CF, Behrens TE, Johansen-Berg H, Bannister PR, De Luca M, Drobnjak I, Flitney DE, Niazy RK, Saunders J, Vickers J, Zhang Y, De Stefano N, Brady JM, Matthews PM. Advances in functional and structural MR image analysis and implementation as FSL. Neuroimage 2004;23:S208-19. [Crossref] [PubMed]
- Winkler AM, Ridgway GR, Webster MA, Smith SM, Nichols TE. Permutation inference for the general linear model. Neuroimage 2014;92:381-97. [Crossref] [PubMed]
- Smith SM, Nichols TE. Threshold-free cluster enhancement: addressing problems of smoothing, threshold dependence and localisation in cluster inference. Neuroimage 2009;44:83-98. [Crossref] [PubMed]
- Yeatman JD, Dougherty RF, Myall NJ, Wandell BA, Feldman HM. Tract profiles of white matter properties: automating fiber-tract quantification. PLoS One 2012;7:e49790. [Crossref] [PubMed]
- Duan Y, Wei J, Geng W, Jiang J, Zhao X, Li T, Jiang Y, Shi L, Cao J, Zhu G, Zhang K, Yu X. Research on Cognitive Function in Anxious Depression Patients in China. J Affect Disord 2021;280:121-6. [Crossref] [PubMed]
- Landis JR, Koch GG. The measurement of observer agreement for categorical data. Biometrics 1977;33:159-74. [Crossref] [PubMed]
- Chen Y, Wang M, Su S, Dai Y, Zou M, Lin L, Qian L, Li X, Zhang H, Liu M, Chu J, Yang J, Yang Z. Assessment of the glymphatic function in children with attention-deficit/hyperactivity disorder. Eur Radiol 2024;34:1444-52. [Crossref] [PubMed]
- Lin LP, Su S, Hou W, Huang L, Zhou Q, Zou M, Qian L, Cui W, Yang Z, Tang Y, Chen Y. Glymphatic system dysfunction in pediatric acute lymphoblastic leukemia without clinically diagnosed central nervous system infiltration: a novel DTI-ALPS method. Eur Radiol 2023;33:3726-34. [Crossref] [PubMed]
- Zhang C, Xu K, Zhang H, Sha J, Yang H, Zhao H, Chen N, Li K. Recovery of glymphatic system function in patients with temporal lobe epilepsy after surgery. Eur Radiol 2023;33:6116-23. [Crossref] [PubMed]
- Wright AM, Wu YC, Chen NK, Wen Q. Exploring Radial Asymmetry in MR Diffusion Tensor Imaging and Its Impact on the Interpretation of Glymphatic Mechanisms. J Magn Reson Imaging 2023; Epub ahead of print. [Crossref] [PubMed]
- Zhang W, Zhou Y, Wang J, Gong X, Chen Z, Zhang X, Cai J, Chen S, Fang L, Sun J, Lou M. Glymphatic clearance function in patients with cerebral small vessel disease. Neuroimage 2021;238:118257. [Crossref] [PubMed]
- Taoka T, Ito R, Nakamichi R, Nakane T, Kawai H, Naganawa S. Diffusion Tensor Image Analysis ALong the Perivascular Space (DTI-ALPS): Revisiting the Meaning and Significance of the Method. Magn Reson Med Sci 2024;23:268-90. [Crossref] [PubMed]
- Taoka T, Ito R, Nakamichi R, Kamagata K, Sakai M, Kawai H, Nakane T, Abe T, Ichikawa K, Kikuta J, Aoki S, Naganawa S. Reproducibility of diffusion tensor image analysis along the perivascular space (DTI-ALPS) for evaluating interstitial fluid diffusivity and glymphatic function: CHanges in Alps index on Multiple conditiON acquIsition eXperiment (CHAMONIX) study. Jpn J Radiol 2022;40:147-58. [Crossref] [PubMed]
- Liu X, Hao J, Yao E, Cao J, Zheng X, Yao D, Zhang C, Li J, Pan D, Luo X, Wang M, Wang W. Polyunsaturated fatty acid supplement alleviates depression-incident cognitive dysfunction by protecting the cerebrovascular and glymphatic systems. Brain Behav Immun 2020;89:357-70. [Crossref] [PubMed]
- Geerlings MI, den Heijer T, Koudstaal PJ, Hofman A, Breteler MM. History of depression, depressive symptoms, and medial temporal lobe atrophy and the risk of Alzheimer disease. Neurology 2008;70:1258-64. [Crossref] [PubMed]
- Ming Q, Zhang J, Cheng C, Dong D, Sun X, Zhong X, Chen W, Yao S. Trait-like white matter abnormalities in current and remitted depression. Psychiatry Res Neuroimaging 2022;326:111544. [Crossref] [PubMed]
- Richard-Devantoy S, Olié E, Guillaume S, Courtet P. Decision-making in unipolar or bipolar suicide attempters. J Affect Disord 2016;190:128-36. [Crossref] [PubMed]
- Bubb EJ, Metzler-Baddeley C, Aggleton JP. The cingulum bundle: Anatomy, function, and dysfunction. Neurosci Biobehav Rev 2018;92:104-27. [Crossref] [PubMed]
- Bach M, Laun FB, Leemans A, Tax CM, Biessels GJ, Stieltjes B, Maier-Hein KH. Methodological considerations on tract-based spatial statistics (TBSS). Neuroimage 2014;100:358-69. [Crossref] [PubMed]
- Zhang H, Li H, Yin L, Chen Z, Wu B, Huang X, Jia Z, Gong Q. Aberrant White Matter Microstructure in Depressed Patients with Suicidality. J Magn Reson Imaging 2022;55:1141-50. [Crossref] [PubMed]
- Liddelow SA, Guttenplan KA, Clarke LE, Bennett FC, Bohlen CJ, Schirmer L, et al. Neurotoxic reactive astrocytes are induced by activated microglia. Nature 2017;541:481-7. [Crossref] [PubMed]
- Wu KY, Liu CY, Chen CS, Chen CH, Hsiao IT, Hsieh CJ, Lee CP, Yen TC, Lin KJ. Beta-amyloid deposition and cognitive function in patients with major depressive disorder with different subtypes of mild cognitive impairment: (18)F-florbetapir (AV-45/Amyvid) PET study. Eur J Nucl Med Mol Imaging 2016;43:1067-76. [Crossref] [PubMed]
- Strain JF, Smith RX, Beaumont H, Roe CM, Gordon BA, Mishra S, Adeyemo B, Christensen JJ, Su Y, Morris JC, Benzinger TLS, Ances BM. Loss of white matter integrity reflects tau accumulation in Alzheimer disease defined regions. Neurology 2018;91:e313-8. [Crossref] [PubMed]
- Pereira JB, Ossenkoppele R, Palmqvist S, Strandberg TO, Smith R, Westman E, Hansson O. Amyloid and tau accumulate across distinct spatial networks and are differentially associated with brain connectivity. Elife 2019 9;8:e50830.
- Li Y, Xie S, Liu B, Song M, Chen Y, Li P, Lu L, Lv L, Wang H, Yan H, Yan J, Zhang H, Zhang D, Jiang T. Diffusion magnetic resonance imaging study of schizophrenia in the context of abnormal neurodevelopment using multiple site data in a Chinese Han population. Transl Psychiatry 2016;6:e715. [Crossref] [PubMed]