GPT-4 to obtain Pfirrmann grade from lumbar spine magnetic resonance imaging (MRI) reports
Introduction
The established use of lumbar spine magnetic resonance imaging (MRI), now considered the gold standard in the diagnosis of spinal pathologies, reflects the medical field’s evolution, especially in the study of intervertebral disc degenerative diseases. Through its excellent capability to precisely visualize spinal structures, lumbar spine MRI constitutes a fundamental pillar in the accurate and non-invasive assessment of various pathological conditions affecting the spine.
The adoption of artificial intelligence (AI), particularly through the use of generative pre-trained models (GPTs) like GPT-4 and ChatGPT, is redefining diagnostic procedures in the field of radiology. These cutting-edge systems introduce innovative ways to process radiological reports, transforming them from free text into organized formats, with the potential to radically improve daily operations and communication among medical professionals (1,2). Such progress promises to optimize information management and clarify radiological data interpretations, positively influencing clinical practice and therapeutic decisions (3-5).
The Pfirrmann Classification is a tool to evaluate intervertebral disc degeneration which is based on morphological and signal criteria in MRI (6). The Pfirrmann Classification, assesses intervertebral disc degeneration based on MRI features, using primarily T2-weighted MRI images, evaluating intervertebral disc degeneration based on the following criteria:
- Signal intensity: changes in signal reflect alterations in disc hydration and composition.
- Nucleus pulposus structure: the internal structure and integrity of the disc’s central part.
- Nucleus-annulus distinction: clarity of the boundary between the nucleus pulposus and the annulus fibrosus.
- Disc height: measurement of the disc’s height relative to adjacent healthy discs.
This classification system can be used in both clinical and research settings. The terminology used is well known within the imaging community, ensuring consistent and reliable classification across different practitioners and studies, providing a standardized method for assessing disc degeneration, often associated with symptoms of pain and disability (7-9).
Each disc is graded from I (normal) to V (severe degeneration), as detailed in the Table 1.
Table 1
Grade | Description |
---|---|
I | Homogeneous, bright hyperintense disc with normal height |
II | Heterogeneous disc with maintained hyperintense signal and normal height |
III | Heterogeneous disc with intermittent gray signal and slightly reduced height |
IV | Dark hypointense, heterogeneous disc with moderately reduced height |
V | Black hypointense, collapsed disc |
The ability to determine the degree of disc degeneration through the Pfirrmann Classification reflects the level of involvement and deterioration of the spine, offering information for potential patient clinical management.
The transition towards structured radiological reporting represents a significant paradigm shift, addressing the inherent limitations of traditional report formats. The use of large language models (LLMs) like GPT-4 in the context of structured radiological reporting explores new frontiers, testing the capability of these advanced AI systems to automatically structure radiological reports (10,11). The potential of these models to automatically apply the Pfirrmann classification to lumbar spine MRI reports represents a promising area of study. Therefore, this study aims to conduct an in-depth analysis of the effectiveness and reliability of GPT-4 in Pfirrmann classification, comparing the results with the expert judgment of a radiologist. The study intends to contribute to new understandings in the AI landscape in radiology, emphasizing the interaction between human expertise and technological innovation.
Methods
Ethical committee approval was not required as no patients or identifiable data were involved. Data search was conducted in December 2023.
Creation of synthetic lumbar spine MRI reports
Synthetic lumbar spine MRI reports were created and crafted to mimic real-life reports. Various degrees of intervertebral disc degeneration were present in the reports, corresponding to the Pfirrmann classifications from 1 to 5.
The reports were initially written in Italian and lacked a structured format. We have used a targeted approach in prompting our GPT models in order to create a balanced sample of 50 reports with 20% representation for each Pfirrmann grade, ensuring that each grade from I to V was equally represented. Specific prompts were designed to guide the models in generating reports with findings corresponding to the desired Pfirrmann grade. For instance, to generate a report describing a grade IV, the prompt used was: “Generate a lumbar MRI report that describes at least one finding with disc degeneration consistent with grade IV of the Pfirrmann classification.” This approach enabled us to accurately maintain a proportional distribution of 20% for each grade, providing a comprehensive and representative dataset for analysis.
To enhance the robustness of the study we have later created an additional sample of 50 synthetic reports, again equally distributed across the five Pfirrmann grades. This second data search was conducted in May 2024.
We employed GPT-4 and two specific “GPTs” (Generative Pre-trained Transformers), an innovation introduced by OpenAI in the field of AI. As defined by OpenAI, GPTs represent innovative ways to create customized versions of GPT-4, tailored for daily life, specific tasks, work, or home, with sharing capabilities. This customization allows users to adapt these AI models to the specific needs of the current study. The two models were as follows:
- SinteticRMPfirrmannGPT: this model was used to generate synthetic reports, creating data that simulate real-life reports and include various information classifiable under the Pfirrmann classification.
- PfirrmannGPT: this model analyzed the synthetic reports, emulating a radiologist’s approach. Its goal was to identify and classify any findings relevant to the Pfirrmann classification in the reports, assigning an appropriate classification level. PfirrmannGPT represents a specific application of a customized GPT for accurate and reliable radiological data analysis.
These two specific GPTs correspond to custom versions of GPT-4 that were created by the authors of this paper, one radiology resident (A.C.S., 4 years of experience), using specific system prompts through the ChatGPT web interface. These are not separate models but rather tailored versions and customized instances of GPT-4, specifically adapted for our study’s tasks. The prompts were carefully crafted to ensure they guide the model in generating and analyzing reports according to the Pfirrmann classification. To avoid any potential data leakage, we did not use the synthetic reports tested in the study for crafting the prompts. This step was crucial to maintain the integrity and validity of our research results.
All reports were first verified for consistency through the consensus of two researchers: one expert radiologist (C.A.M., 12 years of experience) and one radiology resident (A.C.S., 4 years of experience). Subsequently, the reports were rated for the Pfirrmann Classification by an expert radiologist (C.A.M., 12 years of experience). One single Pfirrmann grade was assigned for each report, both by GPT-4 and by the radiologist, taking into account only the description of the most degenerated disc.
The term “consistency” refers to the agreement between the Pfirrmann classifications assigned by GPT-4 and those provided by the expert radiologist. This step ensures that the AI model’s classifications reliably replicate the expert’s judgment, thereby validating the model’s accuracy in interpreting lumbar spine MRI reports. Consistent classification across different instances highlights the AI’s ability to produce reliable and replicable results.
Data analysis
After generating and analyzing the reports through GPT-4, we compared the ratings provided by PfirrmannGPT with those given by the expert radiologist. We organized and labeled the data ensuring Pfirrmann classifications from both the radiologist and the LLM. Pfirrmann scores were presented in counts and percentages and tabulated in a 5×2 contingency table. The concordance between the radiologist and GPT-4 was assessed using Cohen’s Kappa coefficient (12). The significance of the difference between the observed agreement and the expected agreement by chance was calculated, with a 5% threshold for a Type I error.
Results
Our study included a first group of 50 reports distributed among various Pfirrmann classifications as follows: 10 (20%) Pfirrmann 1, 10 (20%) Pfirrmann 2, 10 (20%) Pfirrmann 3, 10 (20%) Pfirrmann 4, and 10 (20%) Pfirrmann 5. The agreement between GPT-4 and the radiologist was total (100%) for Pfirrmann classifications 1, 4, and 5. However, out of the 10 reports with a Pfirrmann 3 classification, 9 (90%) were correctly classified by GPT-4, while the remaining 1 (10%) was classified as Pfirrmann 2, as shown in Table 2 and Figure 1. Overall, the agreement was 98%, compared to an expected chance agreement of 20%. The Cohen’s Kappa value was 0.975 (P<0.001), indicating an almost perfect agreement.
Table 2
Radiologist | Total | |||||
---|---|---|---|---|---|---|
Grade 1 | Grade 2 | Grade 3 | Grade 4 | Grade 5 | ||
GPT-4 | ||||||
Grade 1 | 10 (100%) | 0 (0%) | 0 (0%) | 0 (0%) | 0 (0%) | 10 (20%) |
Grade 2 | 0 (0%) | 10 (100%) | 1 (10%) | 0 (0%) | 0 (0%) | 11 (22%) |
Grade 3 | 0 (0%) | 0 (0%) | 9 (90%) | 0 (0%) | 0 (0%) | 9 (18%) |
Grade 4 | 0 (0%) | 0 (0%) | 0 (0%) | 10 (100%) | 0 (0%) | 10 (20%) |
Grade 5 | 0 (0%) | 0 (0%) | 0 (0%) | 0 (0%) | 10 (100%) | 10 (20%) |
Total | 10 (20%) | 10 (20%) | 10 (20%) | 10 (20%) | 10 (20%) | 50 (100%) |
Data are presented as n (%). Grade 1: both the radiologist and GPT-4 correctly classified 10 reports, representing 100% agreement. Grade 2: radiologist classified 10 reports as Grade 2, and GPT-4 classified 11 reports, one of which was misclassified from Grade 3. Grade 3: radiologist classified 10 reports as Grade 3, and GPT-4 correctly classified 9 reports as Grade 3, with one misclassified under Grade 2. Grade 4: both radiologist and GPT-4 correctly classified 10 reports, representing 100% agreement. Grade 5: both radiologist and GPT-4 correctly classified 10 reports, representing 100% agreement. GPT, generative pre-trained model; MRI, magnetic resonance imaging.
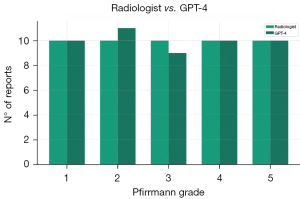
The misclassification by GPT-4 occurred with a report intended to describe a Pfirrmann grade 3 disc degeneration, which the model erroneously classified as grade 2. This error likely resulted from the unstructured format of the reports and the fact that they were written in Italian, which may have led to confusion in interpreting the descriptors accurately. Additionally, it’s important to recognize that LLMs, including GPT-4, are not infallible and may make occasional errors. Understanding these limitations is a key aspect of our study, as we aim to assess and improve the model’s performance. To illustrate this, Figure 2 shows the specific report that was misclassified by GPT-4. This figure highlights the textual descriptions and the relevant features that may have led to the confusion in classification.
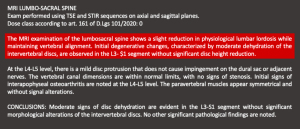
The second group of 50 reports was divided as follow: 10 (20%) Pfirrmann 1, 10 (20%) Pfirrmann 2, 10 (20%) Pfirrmann 3, 10 (20%) Pfirrmann 4, and 10 (20%) Pfirrmann 5.
For the second group of 50 reports included, GPT-4 accurately classified all the reports correctly, achieving a perfect 100% accuracy.
Comments
Our study focused on evaluating the accuracy of GPT-4 in classifying radiological reports according to the Pfirrmann Classification, compared with the evaluations of an expert radiologist. The analysis included reports with varying degrees of intervertebral disc degeneration. The results showed an almost perfect agreement between the radiologist and GPT-4, underscoring the effectiveness of the LLM in interpreting lumbar spine MRI reports for Pfirrmann classification. This task represents a novel effort tested with GPT-4 and holds potential to assist radiologists and clinicians in obtaining Pfirrmann classification from free-text lumbar spine MRI reports.
This high concordance between the classifications attributed by the radiologist and those determined by GPT-4 underscores the effectiveness of AI in recognizing and classifying the degrees of degeneration of the lumbar intervertebral discs in accordance with the Pfirrmann Classification. The almost total absence of discrepancies in recognizing the extreme categories (Pfirrmann 1, 4, and 5) demonstrates the AI’s ability to precisely identify both cases of minor and major degeneration. The slight discrepancy observed in the classification of Pfirrmann 3 reflects the intrinsic challenge in discerning between intermediate degrees of disc degeneration, both in interpreting subtle imaging variations and overlapping descriptors within text-based reports. In fact, subtle differences in descriptors could be more difficult for text-based classification models, such as GPT-4, to discern compared to the visual assessment performed by a radiologist. This area could benefit from further improvements in the AI algorithm.
These results represent a significant step towards integrating AI into radiological practice, offering a potentially reliable tool to assist physicians in evaluating degenerative spinal pathologies. GPT-4’s ability to provide accurate classifications across a wide spectrum of disc degeneration could facilitate early diagnosis, treatment planning, and monitoring of disease progression, significantly contributing to patient care.
The Pfirrmann Classification, recognized as a good tool in the assessment of intervertebral disc degeneration, demonstrates its applicability well beyond its original scope (6-9). Indeed, Pfirrmann classification has been correlated to molecular pathways of disk degeneration also to potentially improve therapeutic choices (13). Changes in the structure of the bony end plate and the reduction of glycosaminoglycans content in the nucleus pulposus could be key indicators of disc degeneration progression as depicted by the Pfirrmann classification, which could be considered a bridge between MRI and biomechanical/molecular changes related to intervertebral disc degeneration (14).
However, it has been emphasized that the Pfirrmann classification, which is based on the disk appearance with T2 weighted MR images, is not able to precisely distinguish between changes occurring due to disk degeneration and physiological aging (15).
Standardized structured reporting in radiology has become a topic of great interest. Indeed, structured reporting could improve a shared language across institutions, communication, interpretation of results, categorization, workflow, and data analysis for both research and healthcare management (16,17).
LLMs, given their capabilities to analyze and generate text, have been recently applied to the field of structured reporting with interesting results (1,11). In this regard, Adams et al. recently reported that GPT-4 can convert free-text into structured reports with minimal effort, potentially facilitating structured reporting in radiology, standardization, and data extraction (2). Moreover, Lyu et al. highlighted that ChatGPT can robustly convert radiology reports into plain language, obtaining a score of 4.27 (based on a five-point system) with 0.08 places of missing information and 0.07 misinformation (5). However, GPT-3.5 Turbo and GPT-4, while capable of effectively transforming free-text radiological reports into a structured format, might overlook some findings, even those of potential clinical importance (11). Indeed, it is known that LLMs are subject to some drawbacks, including hallucinations, data drifts, and factual errors, and that ethics, privacy, and data security remain critical issues not to be overlooked when dealing with LLM applications in medicine (3,10,18).
This is the first study to apply GPT-4 to the task of obtaining Pfirrmann Classification from lumbar spine MRI reports. Despite the promising results, our study is not without limitations. The primary limitation is the need for further validation in different clinical settings and with larger data sets. Additionally, it’s crucial to recognize that LLMs, not being specifically trained for radiology, may not fully capture the complexity and nuances of this discipline. However, the innovative nature of the findings reported by the present study suggests unexplored territory within the capabilities of GPT-4, specifically tailored to meet the unique needs of medical professionals working with complex MRI data. Lastly, it should be underlined that only one expert was involved in the rating of the reports. Further studies should be conducted to evaluate the GPT-4 performance with a multi-rater approach and comparing synthetic and human-produced reports.
In conclusion, here we tested a powerful language model, GPT-4, to automatically analyze MRI reports of the spine and categorize the Pfirrmann grade for disc degeneration. GPT-4 showed an excellent performance, nearly matching expert radiologists. This AI technology has the potential to significantly improve radiology by streamlining analysis, potentially leading to faster diagnoses and better monitoring of spinal conditions. The study highlights the promise of AI in medicine, suggesting it can improve efficiency, accuracy, and ultimately patient care.
Acknowledgments
Funding: None.
Footnote
Conflicts of Interest: All authors have completed the ICMJE uniform disclosure form (available at https://qims.amegroups.com/article/view/10.21037/qims-24-883/coif). C.A.M. serves as an unpaid editorial board member of Quantitative Imaging in Medicine and Surgery. The other authors have no conflicts of interest to declare.
Ethical Statement: The authors are accountable for all aspects of the work in ensuring that questions related to the accuracy or integrity of any part of the work are appropriately investigated and resolved.
Open Access Statement: This is an Open Access article distributed in accordance with the Creative Commons Attribution-NonCommercial-NoDerivs 4.0 International License (CC BY-NC-ND 4.0), which permits the non-commercial replication and distribution of the article with the strict proviso that no changes or edits are made and the original work is properly cited (including links to both the formal publication through the relevant DOI and the license). See: https://creativecommons.org/licenses/by-nc-nd/4.0/.
References
- Mallio CA, Sertorio AC, Bernetti C, Beomonte Zobel B. Large language models for structured reporting in radiology: performance of GPT-4, ChatGPT-3.5, Perplexity and Bing. Radiol Med 2023;128:808-12. [Crossref] [PubMed]
- Adams LC, Truhn D, Busch F, Kader A, Niehues SM, Makowski MR, Bressem KK. Leveraging GPT-4 for Post Hoc Transformation of Free-text Radiology Reports into Structured Reporting: A Multilingual Feasibility Study. Radiology 2023;307:e230725. [Crossref] [PubMed]
- Mallio CA, Sertorio AC, Bernetti C, Beomonte Zobel B. Radiology, structured reporting and large language models: who is running faster? Radiol Med 2023;128:1443-4. [Crossref] [PubMed]
- Lecler A, Duron L, Soyer P. Revolutionizing radiology with GPT-based models: Current applications, future possibilities and limitations of ChatGPT. Diagn Interv Imaging 2023;104:269-74. [Crossref] [PubMed]
- Lyu Q, Tan J, Zapadka ME, Ponnatapura J, Niu C, Myers KJ, Wang G, Whitlow CT. Translating radiology reports into plain language using ChatGPT and GPT-4 with prompt learning: results, limitations, and potential. Vis Comput Ind Biomed Art 2023;6:9. [Crossref] [PubMed]
- Pfirrmann CW, Metzdorf A, Zanetti M, Hodler J, Boos N. Magnetic resonance classification of lumbar intervertebral disc degeneration. Spine (Phila Pa 1976) 2001;26:1873-8. [Crossref] [PubMed]
- Kaliya-Perumal AK, Ariputhiran-Tamilselvam SK, Luo CA, Thiagarajan S, Selvam U, Sumathi-Edirolimanian RP. Revalidating Pfirrmann's Magnetic Resonance Image-Based Grading of Lumbar Nerve Root Compromise by Calculating Reliability among Orthopaedic Residents. Clin Orthop Surg 2018;10:210-5. [Crossref] [PubMed]
- Griffith JF, Wang YX, Antonio GE, Choi KC, Yu A, Ahuja AT, Leung PC. Modified Pfirrmann grading system for lumbar intervertebral disc degeneration. Spine (Phila Pa 1976) 2007;32:E708-12. [Crossref] [PubMed]
- Xu C, Yin M, Mo W. An independent agreement study of modified Pfirrmann grading system for cervical inter-vertebral disc degeneration in cervical spondylotic myelopathy. Br J Neurosurg 2024;38:260-4. [Crossref] [PubMed]
- Akinci D'Antonoli T, Stanzione A, Bluethgen C, Vernuccio F, Ugga L, Klontzas ME, Cuocolo R, Cannella R, Koçak B. Large language models in radiology: fundamentals, applications, ethical considerations, risks, and future directions. Diagn Interv Radiol 2024;30:80-90. [Crossref] [PubMed]
- Mallio CA, Bernetti C, Sertorio AC, Zobel BB. ChatGPT in radiology structured reporting: analysis of ChatGPT-3.5 Turbo and GPT-4 in reducing word count and recalling findings. Quant Imaging Med Surg 2024;14:2096-102. [Crossref] [PubMed]
- McHugh ML. Interrater reliability: the kappa statistic. Biochem Med (Zagreb) 2012;22:276-82. [Crossref] [PubMed]
- Hollenberg AM, Maqsoodi N, Phan A, Huber A, Jubril A, Baldwin AL, Yokogawa N, Eliseev RA, Mesfin A. Bone morphogenic protein-2 signaling in human disc degeneration and correlation to the Pfirrmann MRI grading system. Spine J 2021;21:1205-16. [Crossref] [PubMed]
- Che YJ, Guo JB, Liang T, Chen X, Zhang W, Yang HL, Luo ZP. Assessment of changes in the micro-nano environment of intervertebral disc degeneration based on Pfirrmann grade. Spine J 2019;19:1242-53. [Crossref] [PubMed]
- Wang YXJ. Several concerns on grading lumbar disc degeneration on MR image with Pfirrmann criteria. J Orthop Translat 2022;32:101-2. [Crossref] [PubMed]
- Granata V, Faggioni L, Grassi R, Fusco R, Reginelli A, Rega D, et al. Structured reporting of computed tomography in the staging of colon cancer: a Delphi consensus proposal. Radiol Med 2022;127:21-9. [Crossref] [PubMed]
- Goel AK, DiLella D, Dotsikas G, Hilts M, Kwan D, Paxton L. Unlocking Radiology Reporting Data: an Implementation of Synoptic Radiology Reporting in Low-Dose CT Cancer Screening. J Digit Imaging 2019;32:1044-51. [Crossref] [PubMed]
- Alkaissi H, McFarlane SI. Artificial Hallucinations in ChatGPT: Implications in Scientific Writing. Cureus 2023;15:e35179. [Crossref] [PubMed]