A paradigm shift in oncology imaging: a prospective cross-sectional study to assess low-dose deep learning image reconstruction versus standard-dose iterative reconstruction for comprehensive lesion detection in dual-energy computed tomography
Introduction
Malignant tumors, such as those in the liver, lungs, and gastrointestinal tract, have been shown to exhibit high 5-year recurrence rates (1,2), and multiphase dynamic thoracic-abdominal-pelvic computed tomography (CT) scans are recommended for surveillance. These scans are crucial for excluding the presence of distant metastases and play a significant role in the initial diagnosis stage, follow-up, and evaluation of therapeutic effects (3-5). However, the radiation dosage received due to the repeated scans is a major concern. Reducing the radiation dose in thoracic-abdominal-pelvic CT is beneficial but may increase image noise, degrade image quality, and hinder the detection and diagnosis of tumor lesions, especially for small and low-contrast liver metastases (6). In recent years, dual-energy computed tomography (DECT) has been widely applied due to its capability to provide valuable diagnostic information. Moreover, DECT low-kiloelectron volt (keV) virtual monochromatic images (VMIs) can enhance iodine attenuation and improve the contrast of organ structures and lesions, thereby improving the detection and characterization of lesions (7-10). However, low-keV VMIs suffer from high-image noise. Previous studies (11) have shown that iterative reconstruction (IR) algorithms can suppress image noise, but these IR algorithms may also degrade the spatial resolution of low-contrast and small objects under reduced radiation doses.
Recently, a commercially available deep learning image reconstruction (DLIR) algorithm (TrueFidelity, GE HealthCare, Chicago, IL, USA) has been developed to address the limitations of conventional filtered back projection (FBP) and IR methods (11-14). For DECT, DLIR preserves image sharpness, texture, and artifact qualities by training deep neural networks with low-dose (LD) material (i.e., iodine and water)-decomposed sinograms of different kV measurements as input and high-dose FBP images of dual-energy acquisitions from the same phantoms and patients as the ground truth. Based on large-scale training and testing datasets, the DLIR process is highly capable in differentiating between image signals and noise in both material bases in spectral CT (15). However, studies on the applications of DLIR in LD DECT are rare. Two recent studies reported that DLIR provides superior noise reduction for LD DECT compared to FBP and IR while maintaining good image contrast/texture and lesion conspicuity/detection (16,17). However, both studies solely focused on abdominal CT and applied single-energy CT (SECT) in the control group, and only one study applying DLIR in thoracic-abdominal-pelvic DECT focused on improving the image quality, but it did not evaluate diagnostic lesion detection (16). Thus, the capability of LD low-keV thoracic-abdominal-pelvic DECT with DLIR for detecting various types of tumor lesions in different locations and sizes remains relatively unclear.
Hence, we conducted a study with the aim of assessing the efficacy of LD thoracic-abdominal-pelvic DECT at 40 and 50 keV with DLIR in enhancing image quality and detecting pulmonary nodules, lymph nodes, and liver lesions in oncology patients and compared its performance to that of standard-dose (SD) DECT with IR. To the best of our knowledge, this is the first comprehensive multidetection study using LD thoracic-abdominal-pelvic DECT with DLIR. We present this article in accordance with the STROBE reporting checklist (available at https://qims.amegroups.com/article/view/10.21037/qims-24-197/rc).
Methods
This prospective study was approved by the Human Research Ethics Committee of The First Affiliated Hospital of Zhengzhou University (No. 2022-KY-0752-001) and was conducted in accordance with the Declaration of Helsinki (as revised in 2013). All patients provided written informed consent before participation. This study was registered in the China Clinical Trial Registry (registration No. ChiCTR-DPD-16010302).
Participants and study design
We prospectively recruited participants scheduled for thoracic-abdominal-pelvic contrast-enhanced CT for tumor surveillance during the accrual period spanning from April 2022 to July 2023 at The First Affiliated Hospital of Zhengzhou University. The inclusion criteria were as follows: (I) suspected tumor metastases and/or recurrence after surgery or chemotherapy for malignant tumors, (II) age 18 years or older, and (III) no pregnancy. Meanwhile, the exclusion criteria were as follows: (I) impaired renal function (glomerular filtration rate <30 mL/min/1.73 m2), (II) history of allergic reaction to iodine, (III) presence of more than 20 metastases, and (IV) severe motion artifacts. The inclusion and exclusion criteria are listed in Figure 1.
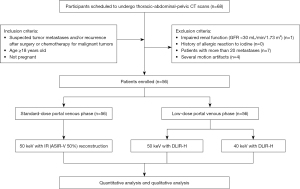
On the basis of expected CT accuracy with an assumed discordant rate of 10% or higher (6), our power analysis, conducted with PASS 15 statistical software (NCSS, Kaysville, UT, USA), indicated that 50 participants would achieve a statistical power of at least 80% with a one-sided type I error rate of 10%. A total of 56 oncology participants (29 males and 27 females; age 58.3±11.9 years) were enrolled in this intraindividual study. Each patient underwent two consecutive thoracic-abdominal-pelvic DECT scans in a single breath hold during the portal venous phase: an SD protocol and a LD protocol (50% of SD protocol). The SD protocol was set to approximate the 25–75th percentile volume CT dose index (CTDIvol) reported in the American College of Radiology Dose Index Registry (9–19 mGy) (18). The study flowchart is illustrated in Figure 1.
Image acquisition
All CT examinations were performed using a Revolution Apex CT scanner (GE HealthCare, Chicago, IL, USA). For each patient, nonionic intravenous contrast material [350 mgI/mL; Omnipaque (iohexol), GE HealthCare] was administered at a rate of 3 mL/s, with a 450 mgI/kg lean-body-weight contrast media protocol (total contrast media volume of 70–120 mL) (19). No adverse reactions to contrast material were reported. Following a bolus tracking of 120 Hounsfield units (HU) in the abdominal aorta, a delay of 12 s was performed. DECT under an SD protocol was then performed for the arterial phase. These arterial phase images were not included for analysis in this study. After another delay of 30 s, two consecutive thoracic-abdominal-pelvic DECT scans were acquired in a single breath hold during the portal venous phase. The first CT scan was performed in the craniocaudal direction with the SD protocol, while the second scan was performed using the LD protocol immediately afterwards in the reverse direction to minimize contrast material time differences (6,20). These portal venous phase images were processed, evaluated, and compared in this study. The scanning parameters for the LD protocol were set to achieve approximately a 50% reduction in radiation dose compared to the SD protocol, and the scanning parameters were as follows: tube voltage, 80/140 kVp; detector configuration, 0.625×128 mm; beam collimation, 80 mm; pitch, 0.992:1; and rotation time, 0.5 s. The tube current modulation was set to 335 mA for the SD scan and to 190 mA for the LD scan. The duration of the venous phase scan was around 6 s, and the time interval between two consecutive venous phase scans was around 1 s.
Image reconstruction and DLIR for DECT
The SD protocol DECT images were reconstructed using IR. Previous studies (21,22) and our clinical experiments have indicated that a hybrid model-based adaptive statistical iterative reconstruction blended with 50% FBP (50% ASIR-V) is suitable for the SD protocol DECT images. Therefore, the raw data from the SD protocol were reconstructed into VMIs at 50 keV using 50%ASIR-V (SD-IR50 keV). The LD protocol DECT images were reconstructed using DLIR (TrueFidelity, GE HealthCare) (13), which is trained on convolutional neural networks with multiple layers and thousands of interlayer connections. DLIR can appropriately handle the material decomposition in the projection domain by learning the unique characteristics of the noise in different material bases (i.e., iodine and water) to achieve noise reduction and texture preservation on DECT. The input data for the networks were LD material-decomposed sinograms of the low- and high-kV measurements, and the ground truth data were the high-dose spectral high-quality FBP images. DLIR learned the characteristics of high-quality images and processed a solid capability to differentiate between image signals and noise in DECT via training on the robust training data set containing both phantom and clinical data that contained multiple anatomies, contrast imaging conditions, and many different noise realizations for various clinical imaging scenarios. Therefore, DLIR could reconstruct high-quality images from noisy LD scanning and preserve the image texture similar to the high-dose FBP, even in challenging LD spectral CT cases. DLIR provides three selectable reconstruction strength levels (low, medium, high) to control the strength of noise reduction, and the high-strength level demonstrated the most substantial noise reduction capability. According to previous studies (17,21,23) and our clinical experience, DLIR at high strength (DLIR-H) was determined appropriate for the LD protocol. The raw data from the LD protocol were reconstructed into VMIs with DLIR-H at 50-keV (LD-DL50 keV) and at 40 keV (LD-DL40 keV), respectively.
Quantitative image analysis
Images were analyzed on a commercially available workstation (Advantage Workstation 4.7, GE HealthCare). All CT images with a slice thickness of 1.25 mm were evaluated by two radiologists (each with 10 years of experience in thoracic-abdominal-pelvic radiology). The quantitative measurement results between the two readers were averaged to ensure accuracy. The HU of attenuation of the liver, pancreas, portal vein, and paraspinal muscle at the level of the portal vein and the CT values of all lesions (including pulmonary nodules, focal liver lesions, and lymph nodes) were each measured within a circular region of interest (ROI). The details of ROIs are described in Appendix 1. Image noise was defined as the standard deviation of HU in a homogeneous region of the subcutaneous fat on the anterior abdominal wall away from artifacts or vessels. The size, shape, and position of the ROI remained consistent, and the measurements were performed three times at different image levels to calculate the average values. The contrast-to-noise ratios (CNRs) relative to the muscle for the organs of interest (liver, pancreas, and lymph node) were calculated as follows: , where CTROI is the mean attenuation of the ROI, CTm is the mean attenuation of the paraspinal muscle, and SDn is the mean image noise (13,24). Similarly, the CNRs of the portal vein, pulmonary nodule, and focal liver lesions were computed as follows: , where CTtarget is the mean attenuation of the organ or structure of interest.
Qualitative image analysis
The three sets of images were anonymized and randomly distributed to two radiologists (with 15 and 6 years of experience in thoracic-abdominal-pelvic radiology, respectively) for review. They were allowed to optimally adjust the window width and level. An interval of at least 4 weeks was set between the two reading sessions to minimize the recall bias. A 5-point Likert scale (5, excellent; 4, good; 3, acceptable; 2, subacceptable; and 1, not acceptable) was used to rate all clinical images in terms of the following criteria (22,24,25): (I) image noise—the amount of graininess or mottle present in the images; (II) vessel conspicuity—ability to identify the margins of the segmental branch level of the portal vein; (III) image contrast—depiction of the contrast between the vessel and adjacent nontumorous liver parenchyma; (IV) artificial sensation—image twisting or altering due to pixilation or blotchy appearance and texture changes; and (V) overall image quality—reader’s confidence in making a reasonable diagnosis from the image (readers were informed that a score of ≤2 would be considered inadequate for diagnosis).
Lesion analysis and reference standard
A reference standard was established using the saved reader marks and all available clinical data. The diagnosis of the lesions was determined through a combination of imaging modalities (e.g., magnetic resonance imaging, positron emission tomography-CT, or follow-ups), and/or pathology (biopsy or surgery). One experienced radiologist (with over 15 years of experience in interpreting thoracic-abdominal-pelvic CT images) was instructed to annotate and save marks for all lesions in the three sets of images, including pulmonary nodules, focal liver lesions, and lymph nodes. The number and size of visible standard lesions were recorded using electronic calipers. The liver lesions were further divided into low- and high-contrast lesions based on CT attenuation relative to the surrounding tissues (26) (see Appendix 2 for details). Subsequently, two radiologists were asked to rate the conspicuity of each lesion independently in the three sets of images using a comparative Likert-type scale as follows (21): 5, optimal reconstruction; 4, slightly inferior (does not affect diagnosis); 3, mildly inferior (may slightly affect the diagnosis); 2, moderately inferior (may affect the diagnosis to some extent); and 1, markedly inferior (interferes with the diagnosis). The two observers were informed about the presence and location of the lesions but were blinded to clinical information and the CT image reconstruction methods, and the review of SD-IR50 keV and LD-DL50 keV and LD-DL40 keV images in the same participant was separated by a 4-week delay to minimize recall. The raters were allowed to scroll and adjust the window level and width while evaluating the cases. Besides qualitative measures, the CNR of each lesion in every image was also calculated.
The lesion detection task was performed on the three image sets via the consensus of two blinded radiologists with 15 and 10 years of experience, respectively. If any reader detected a lesion in a specific image, it was considered detectable. The detection rate for lesions in each image set was calculated as follows: .
Radiation dose
Radiation exposure data, including CTDIvol, and the dose-length product (DLP) displayed on the CT console, were recorded after the examination. The effective radiation dose (ED) in millisievert (mSv) was calculated by multiplying the DLP by the coefficient of 0.015 mSv/(mGy·cm). The size-specific dose estimate (SSDE) was calculated using the method described in AAPM Report 204, which multiplies the CTDIvol by a size-specific conversion factor (27).
Statistical analysis
All statistical analyses were conducted using R version 4.1.3 (R Development Core Team). Numerical values are presented as the mean ± standard deviation. Repeated-measure analysis of variance was used to compare the CTDIvol, CT values, image noise, and CNR between the SD-IR50 keV, LD-DL50 keV, and LD-DL40 keV images. The qualitative image scores were compared using the Friedman test. In the case of significant difference, post hoc pairwise comparisons were conducted with Bonferroni correction. The detection rate was analyzed using the Cochran Q test. In case of significant difference, post hoc pairwise McNemar tests were further conducted. Moreover, the detection rate of lesions was analyzed by subgroups based on (I) lesion location, (II) lesion size (largest diameter), and (III) lesion type. A two-tailed P value <0.05 was considered statistically significant. The weighted Cohen kappa statistic was used to assess interreader agreement and was categorized as follows: 0.01–0.20 poor, 0.21–0.40 fair, 0.41–0.60 moderate, 0.61–0.80 substantial, and 0.81–1.00 excellent agreement (7,17,20).
Results
Participant characteristics and radiation dose
The characteristics of participants and lesions are summarized in Table 1 and Table S1 in the Appendix. The original diagnoses of these 56 participants were confirmed by biopsy or surgical pathology, and the primary tumors were located in the chest for 18 participants, in the abdomen for 33 participants, and in other locations for 5 participants.
Table 1
Parameter | Value |
---|---|
No. of participants | 56 |
Age (years) | 58.3±11.9 |
Sex, n (%) | |
Male | 29 (51.8) |
Female | 27 (48.2) |
Height (cm) | 166.0±8.9 |
Weight (kg) | 65.2±10.3 |
Body mass index (kg/m2) | 23.7±3.7 |
Anterior-posterior diameter (cm) | 23.5±2.6 |
Transverse diameter (cm) | 30.9±2.3 |
Effective diameter (cm) | 26.9±2.3 |
Primary tumor (No. of patients) | |
Lung | 9 |
Stomach | 8 |
Esophageal | 4 |
Liver | 11 |
Pancreas | 2 |
Colorectum | 9 |
Lymph node | 3 |
Breast | 5 |
Other | 5 |
Lesions (No. of lesions/No. of patients)† | |
Pulmonary nodules | 88/13 |
Liver lesions | 212/32 |
Lymph nodes | 126/25 |
Size (cm) | |
Pulmonary nodules | 0.85±0.32 |
Liver lesions | 2.60±1.71 |
Lymph nodes | 1.39±0.43 |
Data are presented as n or mean ± standard deviation or n (%). †, lesion characteristics including type, number, and size are summarized in Table S1 in the Appendix.
For the SD protocol, the mean CTDIvol was 13.8 mGy, the mean SSDE was 19.41±1.70 (range, 16.90–22.83) mGy, and the ED was 13.22±2.57 (range, 7.00–15.83) mSv. Compared with the SD protocol, the LD protocol resulted in a mean dose reduction of approximately 50%, with a mean CTDIvol of 7.30 mGy, a mean SSDE of 10.74±1.33 (range, 9.10–14.23) mGy, and a mean ED of 7.31±1.73 (range, 3.61–9.18) mSv.
Quantitative and qualitative image quality
Table 2 summarizes the results of quantitative and qualitative analyses. The pairwise comparison results on image noise and CNRs of the anatomical structure are depicted in Figure 2. The intra- and interagreements were substantial (weighted kappa statistic 0.67–0.73), and additional details are included in Figure 3 and Table S2.
Table 2
Parameter | SD-IR50 keV | LD-DL50 keV | LD-DL40 keV | P value |
---|---|---|---|---|
CT value (HU) | ||||
Liver | 166.16±30.05* | 166.14±26.38* | 233.21±37.97†‡ | <0.001 |
Pancreas | 154.66±31.24* | 153.55±24.02* | 225.89±35.83†‡ | <0.001 |
Paraspinal muscle | 72.44±9.43†* | 78.19±8.91*‡ | 103.97±13.31†‡ | <0.001 |
Portal vein | 302.12±68.64* | 300.99±58.77* | 426.95±88.03†‡ | <0.001 |
Image noise (HU) | ||||
Subcutaneous fat | 15.60±5.93 | 13.13±4.70* | 18.05±6.95† | <0.001 |
CNR | ||||
Liver | 6.84±3.59* | 7.65±3.90* | 8.33±4.55†‡ | 0.023 |
Pancreas | 6.16±3.65* | 6.66±3.91* | 8.05±4.99†‡ | 0.001 |
Portal vein | 10.38±6.52†* | 12.74±6.89*‡ | 14.51±6.49†‡ | <0.001 |
Qualitative image quality | ||||
Image noise | 3.70±0.61† | 3.95±0.59*‡ | 3.60±0.56† | 0.002 |
Vessel conspicuity | 4.21±0.57† | 3.90±0.63*‡ | 4.08±0.73† | <0.001 |
Image contrast | 4.74±0.37* | 4.72±0.40* | 4.90±0.23†‡ | <0.001 |
Artificial sensation | 4.08±0.47* | 3.99±0.49 | 3.88±0.54‡ | 0.003 |
Overall image quality | 4.10±0.52* | 4.00±0.52* | 3.84±0.59†‡ | 0.009 |
Data are presented as the mean ± standard deviation. *, P<0.01 indicates a statistically significant difference with LD-DL40 keV; †, P<0.05 indicates a statistically significant difference with LD-DL50 keV; ‡, P<0.01 indicates a statistically significant difference with SD-IR50 keV. CT, computed tomography; CNR, contrast-to-noise ratio; SD-IR50 keV, standard-dose 50-keV virtual monochromatic images with iterative reconstruction; LD-DL50 keV, low-dose 50-keV virtual monochromatic images with DLIR-H; LD-DL40 keV, low-dose 40-keV virtual monochromatic images with DLIR-H; DLIR-H, deep learning image reconstruction at high strength; HU, Hounsfield unit.
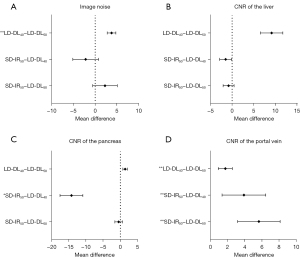
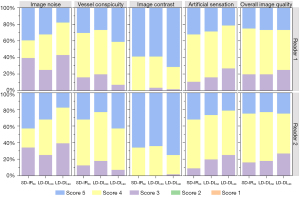
In the quantitative comparison, the CT values, image noise, and CNRs of the liver and pancreas of LD-DL50 keV VMIs were similar to those of SD-IR50 keV VMIs (P>0.05). In the qualitative comparison with SD-IR50 keV VMIs, LD-DL50 keV VMIs showed improved image noise (P<0.05), worse vessel conspicuity (P<0.05), and similar image contrast, artificial sensation, and overall image quality (P>0.05).
Moreover, as compared to those of SD-IR50 keV VMIs, the CT values of LD-DL40 keV VMIs increased by 40.4–47.1% (all P values<0.001), and the CNR values significantly increased by 21.8–39.8% (all P values <0.001). For the qualitative comparison with SD-IR50 keV VMIs, LD-DL40 keV VMIs showed comparable scores on image noise and vessel conspicuity (P>0.05), lower scores on artificial sensation and overall image quality, and higher scores on image contrast (all P values <0.05).
Lesion conspicuity and lesion detectability
This study identified 426 lesions in 56 patients, among whom 13 (23.2%) had a combined 88 pulmonary nodules, 32 (57.1%) a combined 212 liver lesions, and 25 (44.6%) a combined 126 lymph node. The CNRs and lesion conspicuity scores for all types of lesions are presented in Table 3 and Figure 4. The pairwise comparison results on lesion CNRs are depicted in Figure 5. Figure 6 presents examples of different types of lesions in SD-IR50 keV, LD-DL50 keV, and LD-DL40 keV VMIs.
Table 3
Item | SD-IR50 keV | LD-DL50 keV | LD-DL40 keV | P value |
---|---|---|---|---|
CNR | ||||
Pulmonary nodule | 62.86±26.43† | 88.50±21.04*‡ | 62.29±20.74† | <0.001 |
Solid focal liver lesion | ||||
High-contrast lesion | 6.05±2.52 | 7.73±4.36 | 8.44±4.68 | 0.063 |
low-contrast lesion | 8.74±5.22* | 9.87±6.98 | 10.18±6.91‡ | 0.009 |
Lymph node | 4.02±3.30* | 4.74±3.41* | 5.88±3.94†‡ | 0.043 |
Lesion conspicuity | ||||
Pulmonary nodule | 5.00±0.00 | 4.96±0.19 | 4.96±0.19 | 0.135 |
Solid focal liver lesion | ||||
High-contrast lesion | 4.48±0.68 | 4.24±0.83* | 4.71±0.64† | 0.003 |
Low-contrast lesion | 4.63±0.56† | 4.46±0.76*‡ | 4.74±0.56† | <0.001 |
Lymph node | 4.71±0.61* | 4.60±0.79 | 4.79±0.50‡ | <0.001 |
Data are presented as the mean ± standard deviation. P values were calculated with repeated-measure analysis of variance between the three groups. *, P<0.01 indicates a statistically significant difference with LD-DL40 keV; †, P<0.05 indicates a statistically significant difference with LD-DL50 keV; ‡, P<0.01, indicates a statistically significant difference with SD-IR50 keV. CNR, contrast-to-noise ratio; SD-IR50 keV, standard-dose 50-keV virtual monochromatic images with iterative reconstruction; LD-DL50 keV, low-dose 50-keV virtual monochromatic images with DLIR-H; LD-DL40 keV, low-dose 40-keV virtual monochromatic images with DLIR-H; DLIR-H, deep learning image reconstruction at high strength.
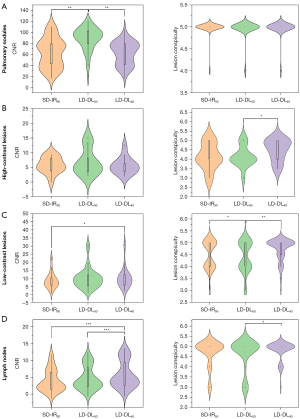
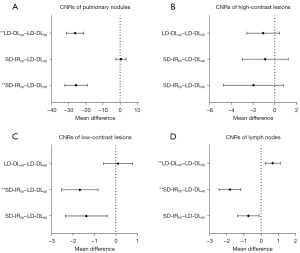
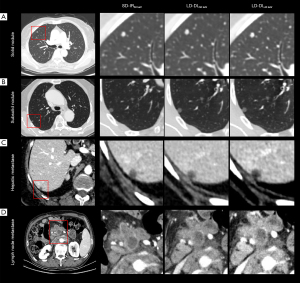
Compared to SD-IR50 keV VMIs, LD-DL50 keV VIMs had higher CNRs in pulmonary nodules (P<0.001) but similar CNRs in solid focal liver lesions and lymph nodes (P>0.05); meanwhile, LD-DL40 keV VMIs had higher CNRs in low-contrast liver lesions and lymph nodes (representing increases of 16.5% and 46.3%, respectively; P<0.001) and equivalent CNRs in high-contrast liver lesions (P>0.05).
The lesion detection rates among the three image sets were evaluated according to lesion location, size, and type, and the findings are presented in Table 4. For pulmonary nodules, both SD protocol and LD protocols could provide full detectability on all nodules regardless of size and type (overall: 88/88, 100%). For liver lesions, 210 of 212 lesions (99.1%) were detected at SD-IR50 keV, and the detection rates for the LD-DL50 keV and LD-DL40 keV images were 87.7% (186/212) and 92.0% (195/212), respectively (P<0.001). This inferior detection of the LD protocol was due to the poorer detection of small lesions (≤0.5 cm) (LD-DL50 keV: 43/65, 66.2%; LD-DL40 keV: 51/65, 78.5%) and low-contrast lesions (LD-DL50 keV: 174/200, 87.0%; LD-DL40 keV: 183/200, 91.5%). Nevertheless, LD-DL40 keV VMIs, compared to LD-DL50 keV VMIs, exhibited higher detection rates for small or low-contrast lesions (P<0.05). No difference was observed in medium- and large-sized or high-contrast liver lesions (all P values >0.05). Regarding lymph nodes, the detection rates in the SD-IR50 keV and LD protocols were comparable with respect to the different types and sizes of nodes. Additional details are provided in Appendix 3.
Table 4
Parameter | SD-IR50 keV | LD-DL50 keV | LD-DL40 keV | P value |
---|---|---|---|---|
Lesion detection rates by location and type (%) | ||||
Pulmonary nodules/overall | 100 (88/88) | 100 (88/88) | 100 (88/88) | >0.99 |
Subsolid nodules | 100 (3/3) | 100 (3/3) | 100 (3/3) | >0.99 |
Solid nodules | 100 (81/81) | 100 (81/81) | 100 (81/81) | >0.99 |
Calcified nodules | 100 (4/4) | 100 (4/4) | 100 (4/4) | >0.99 |
Liver lesions/overall | 99.1 (210/212)* | 87.7 (186/212)† | 92.0 (195/212)‡ | <0.001 |
Low-contrast lesions | 99.0 (198/200)* | 87.0 (174/200)† | 91.5 (183/200)‡ | <0.001 |
High-contrast lesions | 100 (12/12) | 100 (12/12) | 100 (12/12) | >0.99 |
Lymph nodes/overall | 99.2 (125/126) | 97.6 (123/126) | 98.4 (124/126) | 0.223 |
Axillary lymph nodes | 100 (12/12) | 100 (12/12) | 100 (12/12) | >0.99 |
Mediastinal lymph nodes | 100 (20/20) | 95.0 (19/20) | 95.0 (19/20) | 0.368 |
Retroperitoneal lymph nodes | 100 (80/80) | 98.8 (79/80) | 100 (80/80) | 0.368 |
Inguinal lymph nodes | 92.9 (13/14) | 92.9 (13/14) | 92.9 (13/14) | >0.99 |
Lesion detection rates by size (%) | ||||
Pulmonary nodules/overall | 100 (88/88) | 100 (88/88) | 100 (88/88) | >0.99 |
Lesion size ≤0.5 cm | 100 (31/31) | 100 (31/31) | 100 (31/31) | >0.99 |
Lesion size 0.6–1.0 cm | 100 (39/39) | 100 (39/39) | 100 (39/39) | >0.99 |
Lesion size >1.0 cm | 100 (18/18) | 100 (18/18) | 100 (18/18) | >0.99 |
Liver lesions/overall | 99.1 (210/212)* | 87.7 (186/212)† | 92.0 (195/212)‡ | <0.001 |
Lesion size ≤0.5 cm | 96.9 (63/65)* | 66.2 (43/65)† | 78.5 (51/65)‡ | <0.001 |
Lesion size 0.6–1.0 cm | 100 (38/38) | 92.1 (35/38) | 94.7 (36/38) | 0.097 |
Lesion size >1.0 cm | 100 (109/109) | 99.1 (108/109) | 99.1 (108/109) | 0.368 |
Lymph nodes/overall | 99.2 (125/126) | 97.6 (123/126) | 98.4 (124/126) | 0.223 |
Lesion size ≤1.0 cm | 98 (49/50) | 94 (47/50) | 96 (48/50) | 0.223 |
Lesion size >1.0 cm | 100 (76/76) | 100 (76/76) | 100 (76/76) | >0.99 |
Data are relative frequencies based on the total number of lesions; numbers in parentheses indicate the specific count of individual lesions. P values between the three groups were calculated with the Cochran Q test. *, P<0.001 indicates a statistically significant difference between SD-IR50 keV and LD-DL40 keV; †, P<0.001 indicates a statistically significant difference between SD-IR50 keV and LD-DL50 keV; ‡, P<0.05 indicates a statistically significant difference between LD-DL50 keV and LD-DL40 keV. SD-IR50 keV, standard-dose 50-keV virtual monochromatic images with iterative reconstruction; LD-DL50 keV, low-dose 50-keV virtual monochromatic images with DLIR-H; LD-DL40 keV, low-dose 40-keV virtual monochromatic images with DLIR-H; DLIR-H, deep learning image reconstruction at high strength.
Discussion
Our study found that low-keV thoracic-abdominal-pelvic DECT with DLIR, as compared to conventional DECT with IR, allowed for a 50% reduction in radiation dose while largely preserving image quality and lesion detection in oncology patients. Specifically, the lesion detection capabilities were maintained in LD-DL50 keV and LD-DL40 keV VMIs at various locations, including lung, liver, and lymph and for different sizes and types of lesions excepted for small (≤0.5 cm) or low-contrast liver lesions. Furthermore, LD-DL40 keV VMIs showed improved capability in detecting small-sized or low-contrast lesions than did LD-DL50 keV VMIs.
The image quality of VMIs are related to virtual monochromatic energy level, radiation dose, and image reconstruction. Low-keV VMIs (40 and 50 keV) improve iodine contrast through increased iodine attenuation but are also associated with increased image noise and beam-hardening artifacts, especially at low energy levels close to the k-edge of iodine at 33 keV (28,29). This issue can be alleviated by image reconstruction methods, such as DLIR. DLIR for DECT is a new-generation algorithm that is trained on separate deep neural networks for iodine and water materials to improve the ability to decrease image noise and to preserve image sharpness and texture. Therefore, DLIR can improve lesion detectability at reduced radiation doses and facilitate the use of reduced-dose low-keV VMIs for routine clinical applications (15,17). Our study showed that the image quality of 50% radiation dose-reduced DLIR-assisted 50- and 40-keV VMIs was comparable to that of IR-processed 50-keV VMIs under a standard radiation dose. Relative to SD 50-keV VMIs with IR, LD 40-keV VMIs with DLIR could further increase organ enhancement by 40.4–47.1% and increase the CNR values by 21.8–39.8%. All subjective scores for DLIR-processed VMIs fulfilled the clinical diagnostic requirements and preserved the image texture. Our results align with those of previous studies that used low energy levels and DLIR to improve organ enhancement, reduce image noise, and preserve texture in LD settings (16,17). Specifically, Lyu et al. (17) reported that for abdominal CT, DLIR-processed 50-keV VMIs with a 34% radiation dose reduction could improve liver enhancement, maintain image noise, and preserve image texture as compared to IR-processed 120-kVp images with a full radiation dose. In our study, we evaluated the dose reduction ability of DLIR in thoracic-abdominal-pelvic DECT. Noda et al. (16) showed that DLIR-processed 40-keV VMIs with a 63% radiation dose reduction achieved the minimum diagnostic requirement for screening CT in young patients but had increased image noise and reduced CNRs compared to 120-kVp images reconstructed by IR with a full radiation dose. They examined high-contrast tasks at a single 40-keV level but did not include diagnostic lesion detection.
Detection of tumor lesions, especially small or low-contrast ones, is nontrivial and primarily affected by image contrast and image noise (5,30). The ability to detect small or low-contrast tumor lesions is influenced by the increased noise of LD low-keV images, even after DLIR processing. Although powerful, the DLIR may still slightly influence the texture of these small or low-contrast lesions due to the difficulty in differentiating them from the increased image noise in LD low-keV images. Therefore, the detection of small (≤0.5 cm) or low-contrast tumor lesions remains a challenge in LD CT (6). Jensen et al. (6) reported that reduced-dose DLIR had an inferior performance in detecting very small lesions (<0.5 cm) as compared to SD IR, which is consistent with our results. Our study further showed that DLIR-processed 40-keV VMIs obtained higher CNRs, improved perceptual image contrast, similar or better lesion CNRs, and lesion conspicuity in thoracic-abdominal-pelvic imaging as compared to IR- and DLIR-processed 50-keV VMIs. With these improvements, LD 40-keV VMIs with DLIR can achieve high lesion-detection rates, even for small or low-contrast lesions at 50% of the standard radiation dose. Our dose reduction potential was less than that of Noda et al. (16) because we considered multiple diagnostic tasks. To our knowledge, this is the first multidetection study on LD thoracic-abdominal-pelvic DECT with DLIR. Since the evaluation of potential distant metastases of organs takes precedence over radiation protection in oncology patients, we cautiously proposed a 50% reduction in radiation dose for diagnostic thoracic-abdominal-pelvic DECT.
Reducing radiation exposure in multiple dynamic thoracic-abdominal-pelvic CT scans for oncology patients is crucial but may hamper the detection of diagnostic lesions. Previous studies on SECT showed that DLIR could reduce radiation exposure without affecting the detection of pulmonary nodules and live metastases (21,31). Two recent studies demonstrated that reduced-dose low-keV DECT with DLIR provided comparable detection of liver lesions to that of SD SECT (17,24). The diagnostic performance of low-keV DLIR-assisted reduced-dose DECT in regions other than the abdomen has not been investigated. Thus, we systematically evaluated the lesion detection at various locations (including the lungs, liver, and lymph nodes), sizes (small, medium, and large), and types in LD thoracic-abdominal-pelvic DECT. The results revealed that DLIR enables LD thoracic-abdominal-pelvic DECT at 40-keV to detect pulmonary nodules, liver metastases, and lymph nodes of different sizes and types. Specifically, we found that the reduction of radiation by 50% is sufficient for detecting pulmonary nodules, lymph nodes, and high-contrast liver lesions and is clinically acceptable in the detection of small or low-contrast hepatic metastases. The results showed that the radiation dose should be decreased cautiously with respect to multiple diagnostic tasks, especially those involving small or low-contrast lesions. Overall, we recommend DLIR-assisted DECT at 40 keV with a 50% radiation dose reduction in routine clinical practice for follow-up thoracic-abdominal-pelvic imaging in oncology patients.
Several potential limitations of our study warrant consideration. First, despite the use of a self-comparison design to minimize bias and meet statistical standards, our initial experience was based on a limited sample size. Second, we used a single vendor’s fast kilovolt-switching DECT scanner with representative reconstruction levels. External validation with different scanners and CT protocols is needed to confirm LD DLIR’s clinical efficacy at low-keV VMIs. Finally, prioritizing the assessment of distant metastases over radiation protection in oncology patients, we cautiously suggest a 50% dose reduction in diagnostic thoracic-abdominal-pelvic DECT. We anticipate further noninferiority trials on DLIR-assisted VMIs for specific diagnostic tasks.
In conclusion, low-keV VMIs with DLIR, as compared to SD 50-keV VMIs with IR, allow for a 50% reduction in radiation dose while maintaining image quality and lesion detection capabilities for thoracic-abdominal-pelvic CT in oncology patients. Additionally, the LD 40-keV VMIs reconstructed by DLIR provide improved image contrast and a superior ability to detect multiple types of lesions. Overall, we recommend DLIR-assisted DECT at 40 keV with a 50% reduction in radiation dose for follow-up thoracic-abdominal-pelvic imaging in oncology patients.
Acknowledgments
Funding: This study was supported by
Footnote
Reporting Checklist: The authors have completed the STROBE reporting checklist. Available at https://qims.amegroups.com/article/view/10.21037/qims-24-197/rc
Conflicts of Interest: All authors have completed the ICMJE uniform disclosure form (available at https://qims.amegroups.com/article/view/10.21037/qims-24-197/coif). L.W. is an employee of GE HealthCare, the manufacturer of the CT system used in this study. The other authors have no conflicts of interest to declare.
Ethical Statement: The authors are accountable for all aspects of the work in ensuring that questions related to the accuracy or integrity of any part of the work are appropriately investigated and resolved. This study was conducted in accordance with the Declaration of Helsinki (as revised in 2013) and was approved by the Human Research Ethics Committee of The First Affiliated Hospital of Zhengzhou University (No. 2022-KY-0752-001). All enrolled patients signed written informed consent forms. This study was registered in the China Clinical Trial Registry (registration No. ChiCTR-DPD-16010302).
Open Access Statement: This is an Open Access article distributed in accordance with the Creative Commons Attribution-NonCommercial-NoDerivs 4.0 International License (CC BY-NC-ND 4.0), which permits the non-commercial replication and distribution of the article with the strict proviso that no changes or edits are made and the original work is properly cited (including links to both the formal publication through the relevant DOI and the license). See: https://creativecommons.org/licenses/by-nc-nd/4.0/.
References
- Siegel RL, Miller KD, Wagle NS, Jemal A. Cancer statistics, 2023. CA Cancer J Clin 2023;73:17-48. [Crossref] [PubMed]
- Sung H, Ferlay J, Siegel RL, Laversanne M, Soerjomataram I, Jemal A, Bray F. Global Cancer Statistics 2020: GLOBOCAN Estimates of Incidence and Mortality Worldwide for 36 Cancers in 185 Countries. CA Cancer J Clin 2021;71:209-49. [Crossref] [PubMed]
- Zopfs D, Graffe J, Reimer RP, Schäfer S, Persigehl T, Maintz D, Borggrefe J, Haneder S, Lennartz S, Große Hokamp N. Quantitative distribution of iodinated contrast media in body computed tomography: data from a large reference cohort. Eur Radiol 2021;31:2340-8. [Crossref] [PubMed]
- Desch CE, Benson AB 3rd, Somerfield MR, Flynn PJ, Krause C, Loprinzi CL, Minsky BD, Pfister DG, Virgo KS, Petrelli NJAmerican Society of Clinical Oncology. Colorectal cancer surveillance: 2005 update of an American Society of Clinical Oncology practice guideline. J Clin Oncol 2005;23:8512-9. [Crossref] [PubMed]
- Nakamura Y, Higaki T, Tatsugami F, Zhou J, Yu Z, Akino N, Ito Y, Iida M, Awai K. Deep Learning-based CT Image Reconstruction: Initial Evaluation Targeting Hypovascular Hepatic Metastases. Radiol Artif Intell 2019;1:e180011. [Crossref] [PubMed]
- Jensen CT, Gupta S, Saleh MM, Liu X, Wong VK, Salem U, Qiao W, Samei E, Wagner-Bartak NA. Reduced-Dose Deep Learning Reconstruction for Abdominal CT of Liver Metastases. Radiology 2022;303:90-8. [Crossref] [PubMed]
- Nagayama Y, Tanoue S, Inoue T, Oda S, Nakaura T, Utsunomiya D, Yamashita Y. Dual-layer spectral CT improves image quality of multiphasic pancreas CT in patients with pancreatic ductal adenocarcinoma. Eur Radiol 2020;30:394-403. [Crossref] [PubMed]
- Greffier J, Frandon J, Hamard A, Teissier JM, Pasquier H, Beregi JP, Dabli D. Impact of iterative reconstructions on image quality and detectability of focal liver lesions in low-energy monochromatic images. Phys Med 2020;77:36-42. [Crossref] [PubMed]
- Baxa J, Vondráková A, Matoušková T, Růžičková O, Schmidt B, Flohr T, Sedlmair M, Ferda J. Dual-phase dual-energy CT in patients with lung cancer: assessment of the additional value of iodine quantification in lymph node therapy response. Eur Radiol 2014;24:1981-8. [Crossref] [PubMed]
- Sudarski S, Hagelstein C, Weis M, Schoenberg SO, Apfaltrer P. Dual-energy snap-shot perfusion CT in suspect pulmonary nodules and masses and for lung cancer staging. Eur J Radiol 2015;84:2393-400. [Crossref] [PubMed]
- Mileto A, Guimaraes LS, McCollough CH, Fletcher JG, Yu L. State of the Art in Abdominal CT: The Limits of Iterative Reconstruction Algorithms. Radiology 2019;293:491-503. [Crossref] [PubMed]
- Koetzier LR, Mastrodicasa D, Szczykutowicz TP, van der Werf NR, Wang AS, Sandfort V, van der Molen AJ, Fleischmann D, Willemink MJ. Deep Learning Image Reconstruction for CT: Technical Principles and Clinical Prospects. Radiology 2023;306:e221257. [Crossref] [PubMed]
- Tamura A, Mukaida E, Ota Y, Nakamura I, Arakita K, Yoshioka K. Deep learning reconstruction allows low-dose imaging while maintaining image quality: comparison of deep learning reconstruction and hybrid iterative reconstruction in contrast-enhanced abdominal CT. Quant Imaging Med Surg 2022;12:2977-84. [Crossref] [PubMed]
- Wang H, Li X, Wang T, Li J, Sun T, Chen L, Cheng Y, Jia X, Niu X, Guo J. The value of using a deep learning image reconstruction algorithm of thinner slice thickness to balance the image noise and spatial resolution in low-dose abdominal CT. Quant Imaging Med Surg 2023;13:1814-24. [Crossref] [PubMed]
- Thibault J, Nett B, Tang J, Liu E. TrueFidelityTM for GemstoneTM Spectral Imaging: A new generation of spectral imaging powered by deep learning, Technical white paper on deep learning image reconstruction for spectral imaging. GE Healthcare 2022. Available online: https://www.gehealthcare.com/-/jssmedia/gehc/us/files/products/computed-tomography/apex-platform/truefidelity-for-gsi-whitepaper_digital_jb19879xx_v12.pdf?rev=-1
- Noda Y, Kawai N, Kawamura T, Kobori A, Miyase R, Iwashima K, Kaga T, Miyoshi T, Hyodo F, Kato H, Matsuo M. Radiation and iodine dose reduced thoraco-abdomino-pelvic dual-energy CT at 40 keV reconstructed with deep learning image reconstruction. Br J Radiol 2022;95:20211163. [Crossref] [PubMed]
- Lyu P, Li Z, Chen Y, Wang H, Liu N, Liu J, Zhan P, Liu X, Shang B, Wang L, Gao J. Deep learning reconstruction CT for liver metastases: low-dose dual-energy vs standard-dose single-energy. Eur Radiol 2024;34:28-38. [Crossref] [PubMed]
- Kanal KM, Butler PF, Sengupta D, Bhargavan-Chatfield M, Coombs LP, Morin RL U.S.. Diagnostic Reference Levels and Achievable Doses for 10 Adult CT Examinations. Radiology 2017;284:120-33. [Crossref] [PubMed]
- Kang HJ, Lee JM, Ahn C, Bae JS, Han S, Kim SW, Yoon JH, Han JK. Low dose of contrast agent and low radiation liver computed tomography with deep-learning-based contrast boosting model in participants at high-risk for hepatocellular carcinoma: prospective, randomized, double-blind study. Eur Radiol 2023;33:3660-70. [Crossref] [PubMed]
- Li S, Yuan L, Lu T, Yang X, Ren W, Wang L, Zhao J, Deng J, Liu X, Xue C, Sun Q, Zhang W, Zhou J. Deep learning imaging reconstruction of reduced-dose 40 keV virtual monoenergetic imaging for early detection of colorectal cancer liver metastases. Eur J Radiol 2023;168:111128. [Crossref] [PubMed]
- Sato M, Ichikawa Y, Domae K, Yoshikawa K, Kanii Y, Yamazaki A, Nagasawa N, Nagata M, Ishida M, Sakuma H. Deep learning image reconstruction for improving image quality of contrast-enhanced dual-energy CT in abdomen. Eur Radiol 2022;32:5499-507. [Crossref] [PubMed]
- Zhong J, Wang L, Shen H, Li J, Lu W, Shi X, Xing Y, Hu Y, Ge X, Ding D, Yan F, Du L, Yao W, Zhang H. Improving lesion conspicuity in abdominal dual-energy CT with deep learning image reconstruction: a prospective study with five readers. Eur Radiol 2023;33:5331-43. [Crossref] [PubMed]
- Lin X, Gao Y, Zhu C, Song J, Liu L, Li J, Wu X. Improved overall image quality in low-dose dual-energy computed tomography enterography using deep-learning image reconstruction. Abdom Radiol (NY) 2024;49:2979-87. [Crossref] [PubMed]
- Lee HJ, Kim JS, Lee JK, Lee HA, Pak S. Ultra-low-dose hepatic multiphase CT using deep learning-based image reconstruction algorithm focused on arterial phase in chronic liver disease: A non-inferiority study. Eur J Radiol 2023;159:110659. [Crossref] [PubMed]
- Lyu P, Liu N, Harrawood B, Solomon J, Wang H, Chen Y, Rigiroli F, Ding Y, Schwartz FR, Jiang H, Lowry C, Wang L, Samei E, Gao J, Marin D. Is it possible to use low-dose deep learning reconstruction for the detection of liver metastases on CT routinely? Eur Radiol 2023;33:1629-40. [Crossref] [PubMed]
- Solomon J, Marin D, Roy Choudhury K, Patel B, Samei E. Effect of Radiation Dose Reduction and Reconstruction Algorithm on Image Noise, Contrast, Resolution, and Detectability of Subtle Hypoattenuating Liver Lesions at Multidetector CT: Filtered Back Projection versus a Commercial Model-based Iterative Reconstruction Algorithm. Radiology 2017;284:777-87. [Crossref] [PubMed]
- Boone JM. Reply to "Comment on the 'Report of AAPM TG 204: Size-specific dose estimates (SSDE) in pediatric and adult body CT examinations'" Med Phys 2012;39:4615-6. [AAPM Report 204, 2011]. [Crossref] [PubMed]
- D'Angelo T, Cicero G, Mazziotti S, Ascenti G, Albrecht MH, Martin SS, Othman AE, Vogl TJ, Wichmann JL. Dual energy computed tomography virtual monoenergetic imaging: technique and clinical applications. Br J Radiol 2019;92:20180546. [Crossref] [PubMed]
- Agrawal MD, Oliveira GR, Kalva SP, Pinho DF, Arellano RS, Sahani DV. Prospective Comparison of Reduced-Iodine-Dose Virtual Monochromatic Imaging Dataset From Dual-Energy CT Angiography With Standard-Iodine-Dose Single-Energy CT Angiography for Abdominal Aortic Aneurysm. AJR Am J Roentgenol 2016;207:W125-32. [Crossref] [PubMed]
- Fletcher JG, Fidler JL, Venkatesh SK, Hough DM, Takahashi N, Yu L, Johnson M, Leng S, Holmes DR 3rd, Carter R, McCollough CH. Observer Performance with Varying Radiation Dose and Reconstruction Methods for Detection of Hepatic Metastases. Radiology 2018;289:455-64. [Crossref] [PubMed]
- Jiang B, Li N, Shi X, Zhang S, Li J, de Bock GH, Vliegenthart R, Xie X. Deep Learning Reconstruction Shows Better Lung Nodule Detection for Ultra-Low-Dose Chest CT. Radiology 2022;303:202-12. [Crossref] [PubMed]