Multidimensional quantitative characterization of periocular morphology: distinguishing esotropia from epicanthus by deep learning network
Introduction
The medial epicanthus is a semilunar skin fold descending over the lacrimal caruncle to attach the medial aspect of the eyelid. The existence of this fold gives the optical illusion of hypertelorism and binocular misalignment (1-4). In Asians, the incidence rate is estimated to range from 40% to 90% (5).
Epicanthus usually vanishes with age, while the persistent prominent epicanthus could be indicative of some developmental abnormalities, especially for young children. As a typical symptom of certain congenital diseases, epicanthus appears in Blepharophimosis-ptosis-epicanthus inversus syndrome (6), Turner syndrome (7), and Noonan syndrome (8). Quantitating the eyelid morphologic differences could prompt the early identification of these epicanthus-related diseases. In addition, prominent epicanthus tends to coexist with other ocular abnormalities that require surgical corrections like severe epiblepharon or blepharoptosis (9).
Thus, it is necessary to evaluate and track their oculoplastic surgery outcomes in an objective way. Moreover, prominent epicanthal folds also serve as a common feature in pseudo-esotropia for the decreasing exposure of the nasal sclera. In these cases, non-professionals may perceive them as esotropia, a condition that causes eye misalignment requiring timely intervention (4). There is a high risk for amblyopia resulting from esotropia if untreated in developing children (10). Given their completely different treatment strategies, accurate discrimination between the epicanthus and esotropia during the initial examination process is also crucial.
Currently, the clinical evaluation of epicanthus is based on ordinary visual observation which is highly dependent on physicians’ experience. This subjective and transient assessment is not sufficient for comparisons across time and individuals. With the development of artificial intelligence (AI), various automated measurement models for periocular morphological parameters have been proposed (7,11-18). Bahçeci Şimşek et al. (19) applied computer vision technology to obtain pupillary distance, eye area, and average eyebrow height for analyzing the surgical outcome after blepharoplasty. Huang et al. (20) proposed an automatic strabismus screening method based on the iris positional similarity. However, the usage of the facial landmark model and edge-detection algorithm showed limited accuracy and robustness which restricted the comparison of subtle operative adjustments. Furthermore, previous work mainly focused on the limited linear metrics and trained with adults’ data. For children, multidimensional morphological characterization of epicanthus with great accuracy could serve as a great benefit in preliminary screening for concomitant esotropia.
Therefore, we proposed an automatic measurement system based on the deep learning network. We aim to efficiently quantify the periocular features and distinguish concomitant esotropia subjects from epicanthus ones. This system is expected to provide specific reference values for preliminary identification of the concomitant esotropia and consecutive observations of eyelid status. We present this article in accordance with the STARD reporting checklist (available at https://qims.amegroups.com/article/view/10.21037/qims-24-155/rc).
Methods
This observational study was conducted adhering to the tenets of the Declaration of Helsinki (as revised in 2013). The study was approved by the Medical Ethics Committee of The Second Affiliated Hospital of Zhejiang University, School of Medicine (Approval No. 2020-583). Informed consent for this study’s publication was obtained from all participants’ parents.
Participants
All 300 participants aged 7–18 years, including 100 children with simple epicanthus, 100 children with concomitant esotropia, and 100 healthy volunteers matched in age and gender, were recruited for this prospective study in the Eye Center at The Second Affiliated Hospital of Zhejiang University, School of Medicine between November 2022 and January 2023. The eyelid morphologies and ocular alignment of participants were evaluated by the same senior ophthalmologist with more than 15 years of clinical experience. The epicanthus was diagnosed in terms of the characteristic epicanthal fold overlying the inner canthus (21). Ocular alignment was tested with the Hirschberg test, cover test, cover-uncover test and alternate cover test, and the deviation was measured with the prism cover test for near (33 cm) and distance (6 m) fixation. Associated strabismus could be diagnosed according to the observed deviation in these tests (22). The concomitant esotropia manifests the same deviation in all directions of gaze within physiologic limits (23). Patients with abnormal eyelid position (e.g., blepharoptosis, myasthenia gravis), coexisting periocular diseases (e.g., blepharon deformities, blepharocoloboma), and a history of ocular trauma or surgery at the periocular regions were excluded. The inclusion and exclusion criteria above were implemented for collection. Consecutive participants were assigned to the following three groups based on the pre-evaluation after comprehensive ophthalmologic examinations. (I) Epicanthus group comprised subjects diagnosed as bilateral epicanthus. (II) Normal group comprised healthy controls without epicanthus. Participants with refractive errors that do not impact eyelid position or morphology were also included. (III) Concomitant esotropia group consisted of subjects diagnosed as concomitant esotropia.
Photograph acquisition
All 300 photographs (600 eyes) were prospectively obtained from the involved participants. Their frontal view images were captured using a Sony FDR-AX60 camera (SONY China Co., Ltd., Beijing, China). When the patient demonstrated esotropia, the front-facing photograph was selected in which at least one eye was in the primary position. The images were stored as JPG files with a resolution of 3,840 by 2,160.
Manual measurement
Anthropometric landmarks in periocular regions were clarified in the beginning. With the benchmark of periocular landmarks, there are 20 parameters defined in this study: including 10 linear, 3 angular, 2 area measurements, and 5 further derived analytical indices to depict the periocular morphological feature. The schematic illustrations are shown in Figure 1. The detailed definitions are presented in Tables 1,2.
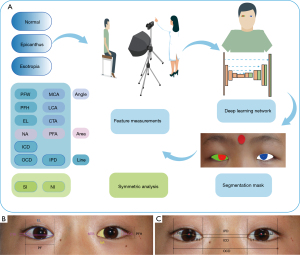
Table 1
Parameters | Abbreviation | Illustration |
---|---|---|
Outer canthal distance | OCD | The horizontal distance between the exocanthion of binoculars |
Inner canthal distance | ICD | The horizontal distance between the endocanthion of binoculars |
Interpupillary distance | IPD | The distance between the center of the pupils of binoculars |
Palpebral fissure width | PFW | The horizontal distance between endocanthion and exocanthion |
Palpebral fissure height | PFH | The vertical distance between the lowest point of the lower eyelid and the highest point of the upper eyelid |
Eyelid length | EL | The marginal length of upper and lower eyelids |
Medial canthus angle | MCA | The angle between the medial upper and lower eyelid, endocanthion acting as the apex |
Lateral canthus angle | LCA | The angle between lateral upper and lower eyelid, exocanthion acting as the apex |
Canthus tilt angle | CTA | The inclination of the horizontal axis of the eye, between endocanthion and exocanthion |
Nasal sclera area | NA | Area of the nasal region of the sclera |
Palpebral fissure area | PFA | The area of the upper and lower eyelid edges outlined |
R1, L1 | – | Distance between the center of the pupil and the internal canthus |
L2, R2 | – | Distance between the center of the pupil and the outer canthus |
OCD, outer canthal distance; ICD, inner canthal distance; IPD, interpupillary distance; PFW, palpebral fissure width; PFH, palpebral fissure height; EL, eyelid length; MCA, medial canthus angle; LCA, lateral canthus angle; CTA, canthus tilt angle; NA, nasal sclera area; PFA, palpebral fissure area; r, right eyes; l, left eyes.
Table 2
Abbreviation | Illustration | Formula |
---|---|---|
CI | Canthal index; the percentage of the intercanthal distance over the outer canthal distance | |
CP | The percentage of the intercanthal distance over the interpupillary distance | |
NR | The ratio of nasal sclera area | |
NI | The area symmetry index of nasal sclera | |
SI (20) | Symmetry index of eye position |
ICD, inner canthal distance; OCD, outer canthal distance; IPD, interpupillary distance; NA, nasal sclera area; PFA, palpebral fissure area; r, right eyes; l, left eyes.
Thirty percent of photographs are randomly selected to be blind measured concurrently by 2 junior ophthalmologists with more than 3 years of clinical experience for 4 linear indices and 3 angular indices: inner canthal distance (ICD), outer canthal distance (OCD), palpebral fissure height (PFH), palpebral fissure width (PFW), medial canthus angle (MCA), lateral canthus angle (LCA), and canthus tilt angle (CTA) using ImageJ (version 1.52; National Institutes of Health, Bethesda, USA). Then, the pixel distance of white-to-white (WTW) referring to the horizontal corneal diameter was measured to derive a conversion factor between pixels and millimeters. With the scale of average WTW of 12 mm (24,25) among Chinese children, all linear pixel measurements were calculated by 12/distance of WTW in pixels. The actual distances were set as the average value from 2 examiners. Meanwhile, the contour of each image’s palpebral fissure and corneal limbus were outlined manually as the ground truth. Any dispute will be arbitrated by a senior specialized ophthalmologist with more than 15 years of clinical experience.
Automated measurement
Segmentation of eyelid and cornea using deep learning
The deep learning model was applied to segment the eyelid and cornea. In this study, 30,000 facial images from the CelebFaces Attributes Dataset were used to train the eye location network (26), and facial images with annotations of 2,069 volunteers from The Second Affiliated Hospital of Zhejiang University were used to train, validate, and test the segmentation network. Thirty percent of 300 facial images from 3 specific groups were selected randomly for an extra test.
First, periocular regions were localized with bounding boxes to extract the regions of interest (ROI). Then, ROI was sent to Attention Recurrent Residual Convolutional Neural Network based on U-Net (Attention R2U-Net) (27). The eyelid and cornea segmentation masks were output to measure periocular morphologic parameters further.
Measurement of periocular morphological parameters
The periocular morphological parameters were measured based on the eyelid and cornea segmentations. The mean shift algorithm with a Gaussian kernel was employed to localize the pupil center. Then, binocular coordinate localizations of each region are stored separately.
First, the location of the inner canthus of both eyes was determined to rotate the image until the two points were on the same horizontal line. All periocular parameters were measured in pixels including canthal distance (including OCD and ICD), interpupillary distance (IPD), palpebral fissure liner features [including PFW, PFH, and eyelid length (EL)], palpebral fissure angular features (including MCA, LCA, and CTA), and palpebral fissure area features [including nasal sclera area (NA) and palpebral fissure area (PFA)], as illustrated in Table 1. To distinguish esotropia from epicanthus, the symmetry index (SI) (20) and the area symmetry index of nasal sclera (NI) were introduced to depict the binocular similarity derived from the linear and area metrics respectively. The values of them are closer to 1, two eyes are more likely in a symmetrical position, otherwise, they may be misaligned. Referring to the scale of WTW, all linear measurements were converted as actual distance. The final measurement takes the average of the first and second return values.
The MCA was calculated with endocanthion as the apex and another two landmarks defined as the intersection of a vertical line with the upper and lower eyelid margins located 2 millimeters from the endocanthion on the lateral side. The LCA was calculated with exocanthion as the apex and another two landmarks defined as the intersection of a vertical line with the upper and lower eyelid margins located 2 millimeters from the exocanthion on the medial side.
Statistical analysis and sample size calculation
The accuracy of corneal and scleral segmentation was evaluated using dice coefficients (Dice) calculated as:
Where A is the ground truth area, and B is the prediction of the model.
The agreement between the two examiners and two measurements was evaluated using intraclass correlation coefficients (ICCs) (28), which with higher values indicated less variation within the methods: moderate agreement (0.44< ICC ≤0.60), substantial agreement (0.60< ICC ≤0.80), excellent agreement (0.80< ICC ≤1.00).
The distributions of the parameters were evaluated with Kolmogorov-Smirnov normality test. The relationship between actual measurements and automated measurements was evaluated by Pearson’s correlation analysis, simple linear regression analysis, and Bland-Altman analysis using GraphPad Prism 8 (GraphPad, San Diego, USA). We applied the student’s t-test to compare the morphological differences between the epicanthus and normal groups. Kruskal-Wallis H test was employed to evaluate the symmetry indexes among three groups and Bonferroni correction was utilized for post-hoc analysis.
Receiver operating characteristic (ROC) curves were generated to assess the discriminatory ability of binocular symmetry indexes for concomitant esotropia by the area under the curves (AUC). Optimal cut-off values were determined as the points with the maximal Youden index (sensitivity + specificity − 1). These statistical assessments were conducted by SPSS 26 (IBM, Chicago, IL, USA). A two-sided P value less than 0.05 was considered statistically significant.
The sample size of consistency assessment was calculated with a two-sided 0.05 Type 1 error and 0.10 Type 2 error. When the expected ICC was prespecified to be 0.90, the calculated sample size was 63 (29). Taking the 10% dropout rate into consideration, the smallest sample size was 70. According to the preliminary experiments, the expected sensitivity of SI and NI to distinguish esotropia were 0.90 and 0.80, respectively. The expected specificity of SI and NI were 0.90 and 0.60, respectively. Thus, sample sizes of the esotropia group and control group were 97 and 145 with a 0.08 permissible error. To ensure a sufficient sample for analysis, we enlarged the total sample size to 100 patients and 200 controls. The sample size calculation was conducted using PASS (NCSS, Kaysville, UT, USA).
Results
Patient characteristics
Three groups of 300 individuals with an age range of 7–18 years were involved in this study for automatic analyses (Figure S1). The median age of the three groups was 9.5 years. In the esotropia group, there are 41 esotropia subjects affected with right eyes and 59 subjects affected with left eyes. Among them, 57 concomitant esotropia subjects had epicanthus at the same time. The demographic characteristics of the included individuals are listed in Table S1.
Performance of segmentation and measurement task
The segmentation mask of the eyelid and cornea based on the proposed model is shown in Figure 1. The dice coefficients for eyelid and cornea segmentation in the extra test set were 0.949 and 0.944, respectively.
A total of 4 linear and 3 angular parameters were measured manually to compare with the automatic method. Inter-examiner reliability was verified with excellent agreement. The ICCs between 2 examiners ranged from 0.914 [95% confidence interval (CI): 0.869–0.943] for ICD to 0.987 (95% CI: 0.980–0.991) for CTA. The ICCs between actual and automated measurements ranged from 0.898 (95% CI: 0.850–0.932) for PFH to 0.983 (95% CI: 0.975–0.989) for OCD. Details about the comparison are shown in Table 3. In Figure 2, Pearson’s correlation analysis and simple linear regression analysis revealed that automated measurements were strongly related to actual measurements, with R2 being 0.9681 for OCD and 0.9417 for ICD. As demonstrated in Figure 3, the Bland-Altman plots also indicated its excellent reliability, with the bias being 0.16 mm for PFW and 0.43 mm for PFH. The R2 of the remaining parameters range from 0.8460 for PFH to 0.9774 for MCA as shown in Figure S2. The biases of the remaining parameters were from 0.33 to 0.69 as presented in Figure S3. In esotropia subjects with epicanthus, the ICCs between two measurements ranged from 0.931 (95% CI: 0.854–0.967) for PFW to 0.989 (95% CI: 0.976–0.995) for ICD as shown in Table S2.
Table 3
Metrics | Automated (mean ± SD) | Actual (mean ± SD) |
Pearson’s r | Manual (mean ± SD) | ICC (95% CI) | |||
---|---|---|---|---|---|---|---|---|
Examiner 1 | Examiner 2 | Examiner 1 vs. examiner 2 | Actual vs. automated | |||||
OCD (mm) | 83.45±6.07 | 82.86±5.85 | 0.984 | 82.58±5.84 | 83.13±6.10 | 0.961 (0.960–0.974) | 0.983 (0.975–0.989) | |
ICD (mm) | 37.63±4.87 | 36.90±3.24 | 0.970 | 37.25±3.37 | 36.55±3.38 | 0.914 (0.869–0.943) | 0.970 (0.954–0.980) | |
PFWr (mm) | 23.13±2.07 | 23.14±2.04 | 0.934 | 22.96±2.15 | 23.33±2.08 | 0.922 (0.881–0.949) | 0.934 (0.901–0.956) | |
PFWl (mm) | 22.67±2.01 | 22.97±2.11 | 0.942 | 22.82±2.17 | 23.12±2.15 | 0.956 (0.933–0.971) | 0.940 (0.911–0.960) | |
PFHr (mm) | 9.49±1.32 | 9.06±1.41 | 0.939 | 8.94±1.38 | 9.18±1.50 | 0.954 (0.930–0.969) | 0.937 (0.906–0.958) | |
PFHl (mm) | 9.47±1.28 | 9.05±1.31 | 0.899 | 9.11±1.39 | 9.00±1.31 | 0.941 (0.910–0.961) | 0.898 (0.850–0.932) | |
MCA (°) | 53.45±13.28 | 55.57±13.39 | 0.988 | 54.14±13.46 | 56.99±13.44 | 0.985 (0.977–0.990) | 0.977 (0.962–0.992) | |
LCA (°) | 66.39±11.68 | 67.06±11.40 | 0.974 | 66.73±11.58 | 64.71±11.47 | 0.947 (0.904–0.969) | 0.972 (0.957–0.982) | |
CTA (°) | 6.33±3.22 | 6.01±3.08 | 0.982 | 5.97±3.10 | 6.06±3.07 | 0.987 (0.980–0.991) | 0.976 (0.950–0.987) |
SD, standard deviation; ICC, intraclass correlation coefficients; CI, confidence interval; OCD, outer canthal distance; ICD, inner canthal distance; PFW, palpebral fissure width; r, right eyes; l, left eyes; PFH, palpebral fissure height; MCA, medial canthus angle; LCA, lateral canthus angle; CTA, canthus tilt angle.
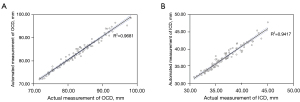
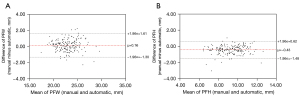
Evaluation of periocular morphological features
The values of 8 monocular eyelid parameters in a dataset of 600 eyes from 3 groups measured by the automatic algorithm were listed in Table S3 respectively. All monocular eyelid parameters except for the ratio of nasal sclera area (NR) in the concomitant esotropia group were similar between the right eyes and left eyes within the group. The reasonable similarity implies the reliability and robustness of the automatic algorithm. In the concomitant esotropia group, the average NR of gaze eyes was 0.16±0.05, while the average NR of esotropia-affected eyes was 0.07±0.04. The significant differences in the NA of the two eyes were consistent with the actual ocular misalignment.
The multi-dimensional features of periocular morphology between the normal eyes and the epicanthus eyes are listed in Table S4. A radar diagram was employed to characterize intuitively as shown in Figure 4. Notably, the PFW, PFH, and EL of the eyes in the epicanthus group were 21.41±1.53, 8.91±1.37, and 53.80±4.11 mm, respectively. The eyes with epicanthus also present a smaller medial canthal angle compared with that of the normal group (51.14°±13.31° vs. 54.87°±12.28°, P<0.01). These linear and angular characteristics of the eyes with epicanthus were consistent with its smaller PFA (137.93±27.57 vs. 168.66±27.07 mm2, P<0.01). As to the derived indexes, the eyes with epicanthus have higher CI (intercanthal distance over the OCD; 0.46±0.02 vs. 0.43±0.02; P<0.01), CP (intercanthal distance over the IPD; 0.59±0.03 vs. 0.56±0.03; P<0.01) and lower NR (0.14±0.05 vs. 0.19±0.04, P<0.01).
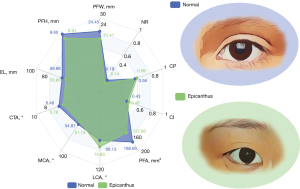
The analysis of positional similarity revealed that the concomitant esotropia group has a significantly higher SI of 1.68 (1.44, 2.04) (P<0.0001, Table S4) and a significantly lower NI of 0.90 (0.86, 0.93) (P<0.0001, Table S4) compared with the other two groups as shown in Table S5. We use violin plots to depict two symmetry indexes distribution of three groups in Figure 5. For further validating the feasibility of two symmetry indexes in case of coincidence of epicanthus and esotropia, we separated the concomitant esotropia group as esotropia alone (Es+Ep−) and esotropia with epicanthus (Es+Ep+) according to the initial evaluation records. As shown in Figure S4, the ocular symmetry indexes of the Es+Ep− subgroup were not significantly different from those of the Es+Ep+ subgroup (P=0.66, Figure S4). However, both of them were significantly different from the epicanthus group in terms of ocular symmetry (P<0.0001, Figure S4). The ROC analysis yielded AUC of 0.971 (95% CI: 0.950–0.991) with SI and 0.821 (95% CI: 0.766–0.877) with NI to distinguish esotropia subjects, respectively. Based on the best performance, the optimal cut-off value for SI was 1.296 with 0.920 sensitivity and 0.910 specificity. The related ROC curves are shown in Figure S5. Furthermore, based on the optimal cut-off value of SI, we validated the applicability of our method in different age ranges with an average accuracy of more than 0.90. The details are presented in Table S6.
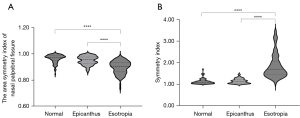
Discussion
In the present study, we established a deep learning system to characterize the periocular morphological features of epicanthus eyes, concomitant esotropia eyes, and control eyes. This automatic method presented excellent agreement with manual measurements. The findings suggested that epicanthus eyes tend to have a shorter and smaller appearance with symmetrical lower exposure of the nasal sclera. The higher CI and CP also explained the visual impression of a wider binocular distance in the epicanthus group. Based on the binocular symmetry, both SI and NI could facilitate initial discrimination of concomitant esotropia subjects, even in case of coincidence of epicanthus. Compared with NI’s performance, the ROC analysis showed that SI had better performance owing to its sensitivity to subtle positional deviations.
Precisely quantifying the periocular characteristics is crucial for early screening of various ocular abnormalities. Among them, epicanthus is always regarded as a normal variation without considering the potential developmental disturbances and epicanthus-related ocular diseases (2,30). In children over 5 years old, except for the risk of occluding the visual field, the tangential vector from persistent epicanthus could result in severe epiblepharon requiring corrective surgery (31,32). In these cases, quantifying the trend of morphological changes in epicanthus eyes with age has important cue value. Moreover, asymmetric exposure of nasal and temporal sclera in children with epicanthus may be deceptive for their parents (4). The incorporation of positional symmetry evaluation in our model could effectively alleviate these unnecessary concerns and anxiety before further examinations during the first visit. Concomitant esotropia is a phenomenon that involves both eyes. When one eye gazes, the deviation is fully reflected in the other eye. Some patients could exhibit alternating binocular gaze. In addition, large negative angle Kappa will give the illusion of esotropia if only evaluated based on a single-eye image. Therefore, we evaluated binocular symmetry for initial discrimination. The application of our measurement model not only enables early identification of eyelid-related diseases but also enhances the efficiency of medical visits and optimizes the allocation of healthcare resources.
Previously, various anthropometric measurements in the periocular region have been conducted to evaluate the subpopulation eyelid appearance (33,34). However, few investigations exist for children and adolescents. Farkas et al. (3) conducted an anthropometric examination in the Caucasian population and revealed that the palpebral fissure is shorter and more tilted in children with major epicanthus than in those without. In our study, multi-feature comparisons demonstrated that epicanthus eyes also appear shorter PFW and PFH, but with less tilted eye fissures. This conflict could be attributed to the age, gender, ethnicity differences, and limited sample size (35-37), which needs to be further validated in a large-scale investigation. In the Chinese population, Cai et al. (36) compared the periocular features of children and young adults and revealed age-related morphological differences. Nevertheless, the interference from epicanthus was not taken into account. The aforementioned anthropometric analyses were measured manually with limited operability and replicability. With the emergence of AI technology, the measurement of periorbital parameters from ocular images becomes easier. Van Brummen et al. (12) developed a deep learning semantic segmentation network to automatically depict eyelid profiles based on facial data of adult Caucasians. Several linear metrics were not comprehensive for quantitative characterization of periocular morphology. Therefore, given the age-related or race-related periocular morphologic differences and the need for discrimination between subjects with epicanthus and ones with concomitant esotropia, a multi-task deep learning system is warranted for the multidimensional characterization of the periocular regions.
There are some limitations to our study. First, our periocular parameters were measured based on two-dimensional (2D) images which means they had discrepancies with the actual eyelid condition, especially in the lateral regions. The disappearance of the depth structure in the lateral constricts the accurate measurement. Therefore, our further work focuses on the analysis of three-dimensional (3D) facial data to reach higher accuracy. In addition, the prior assumption that WTW distance is 12 mm as a scale forms the basis of the conversion from pixels to actual distances. However, the deviations decreased considerably since we collected the images from children of similar age ranges. Finally, our study is based on a small sample size from a single-center dataset with limited generalization, and any application based on the cut-off values should be cautious. Considering the degree of cooperation during ocular alignment tests and image acquisition, only school-aged children with relatively high levels of comprehension and cooperation were recruited for initial validation. In the future, we will optimize the data acquisition using a standardized circle marker as a scale to achieve precise conversion. We will also explore the potential of our system on 3D facial data analysis and further validate our model in a multicenter large-scale test scenario involving a wider range of ages.
Conclusions
In conclusion, this work is the first to describe the periocular morphological features of the epicanthus eyes using a deep learning-based method with high accuracy. The derived symmetry index showed great potential to distinguish concomitant esotropia during initial evaluation. This standardized protocol is expected to be employed in the establishment of a comprehensive anthropometry database and be generalized to other applications in periocular morphological assessment cross-time and cross-individuals.
Acknowledgments
Funding: This work was supported by
Footnote
Reporting Checklist: The authors have completed the STARD reporting checklist. Available at https://qims.amegroups.com/article/view/10.21037/qims-24-155/rc
Conflicts of Interest: All authors have completed the ICMJE uniform disclosure form (available at https://qims.amegroups.com/article/view/10.21037/qims-24-155/coif). L.L. receives funding from the National Natural Science Foundation of China (No. 82000948). J.Y. receives funding from the National Natural Science Foundation of China (Nos. U20A20386 and 81870635), National Key Research and Development Program of China (No. 2019YFC0118400), and Zhejiang Provincial Key Research and Development Plan (No. 2019C03020). The other authors have no conflicts of interest to declare.
Ethical Statement: The authors are accountable for all aspects of the work in ensuring that questions related to the accuracy or integrity of any part of the work are appropriately investigated and resolved. The study was conducted in accordance with the Declaration of Helsinki (as revised in 2013). The study was approved by the Medical Ethics Committee of The Second Affiliated Hospital of Zhejiang University, School of Medicine (Approval No. 2020-583), and informed consent for this study’s publication was obtained from all participants’ parents.
Open Access Statement: This is an Open Access article distributed in accordance with the Creative Commons Attribution-NonCommercial-NoDerivs 4.0 International License (CC BY-NC-ND 4.0), which permits the non-commercial replication and distribution of the article with the strict proviso that no changes or edits are made and the original work is properly cited (including links to both the formal publication through the relevant DOI and the license). See: https://creativecommons.org/licenses/by-nc-nd/4.0/.
References
- Saonanon P. The new focus on epicanthoplasty for Asian eyelids. Curr Opin Ophthalmol 2016;27:457-64. [Crossref] [PubMed]
- Liu N, He A, Wu D, Gong L, Song N. Skin redraping for correction of lower eyelid epiblepharon combined with medial epicanthal fold: a retrospective analysis of 286 Asian children. Eye (Lond) 2022;36:844-9. [Crossref] [PubMed]
- Farkas LG, Cheung G. Orbital measurements in the presence of epicanthi in healthy North American Caucasians. Ophthalmologica 1979;179:309-15. [Crossref] [PubMed]
- Wei N, Qian X, Bi H, Qi X, Lu H, Wei L, Li X, Sun F, Zhang B. Pseudoesotropia in Chinese Children: A Triphasic Development of the Interepicanthal Folds Distance-to-Interpupillary Distance Ratio and Its Changing Perception. Aesthetic Plast Surg 2019;43:420-7. [Crossref] [PubMed]
- Park JI. Modified Z-epicanthoplasty in the Asian eyelid. Arch Facial Plast Surg 2000;2:43-7. [Crossref] [PubMed]
- Sa HS, Lee JH, Woo KI, Kim YD. A new method of medial epicanthoplasty for patients with blepharophimosis-ptosis-epicanthus inversus syndrome. Ophthalmology 2012;119:2402-7. [Crossref] [PubMed]
- Song W, Lei Y, Chen S, Pan Z, Yang J. Multiple facial image features-based recognition for the automatic diagnosis of turner syndrome. Comput Ind 2018;100:85-95. [Crossref]
- Papadopoulos G, Papadopoulou A, Kosma K, Papadimitriou A, Papaevangelou V, Kanaka-Gantenbein C, Bountouvi E, Kitsiou-Tzeli S. Molecular and clinical profile of patients referred as Noonan or Noonan-like syndrome in Greece: a cohort of 86 patients. Eur J Pediatr 2022;181:3691-700. [Crossref] [PubMed]
- SWAN KC. The syndrome of congenital epiblepharon and inferior oblique insufficiency. Am J Ophthalmol 1955;39:130-6. [Crossref] [PubMed]
- Birch EE, Duffy KR. Leveraging neural plasticity for the treatment of amblyopia. Surv Ophthalmol 2024; Epub ahead of print. [Crossref] [PubMed]
- Guo Y, Schaub F, Mor JM, Jia R, Koch KR, Heindl LM. A Simple Standardized Three-Dimensional Anthropometry for the Periocular Region in a European Population. Plast Reconstr Surg 2020;145:514e-23e. [Crossref] [PubMed]
- Van Brummen A, Owen JP, Spaide T, Froines C, Lu R, Lacy M, Blazes M, Li E, Lee CS, Lee AY, Zhang M, Periorbit AI. Artificial Intelligence Automation of Eyelid and Periorbital Measurements. Am J Ophthalmol 2021;230:285-96. [Crossref] [PubMed]
- Lou L, Sun Y, Huang X, Jin K, Tang X, Xu Z, Zhang Q, Wang Y, Ye J. Automated Measurement of Ocular Movements Using Deep Learning-Based Image Analysis. Curr Eye Res 2022;47:1346-53. [Crossref] [PubMed]
- Cao J, Lou L, You K, Gao Z, Jin K, Shao J, Ye J. A Novel Automatic Morphologic Analysis of Eyelids Based on Deep Learning Methods. Curr Eye Res 2021;46:1495-502. [Crossref] [PubMed]
- Lou L, Cao J, Wang Y, Gao Z, Jin K, Xu Z, Zhang Q, Huang X, Ye J. Deep learning-based image analysis for automated measurement of eyelid morphology before and after blepharoptosis surgery. Ann Med 2021;53:2278-85. [Crossref] [PubMed]
- Jin K, Ye J. Artificial intelligence and deep learning in ophthalmology: Current status and future perspectives. Adv Ophthalmol Pract Res 2022;2:100078. [Crossref] [PubMed]
- Shao J, Huang X, Gao T, Cao J, Wang Y, Zhang Q, Lou L, Ye J. Deep learning-based image analysis of eyelid morphology in thyroid-associated ophthalmopathy. Quant Imaging Med Surg 2023;13:1592-604. [Crossref] [PubMed]
- Bao XL, Sun YJ, Zhan X, Li GY. Orbital and eyelid diseases: The next breakthrough in artificial intelligence? Front Cell Dev Biol 2022;10:1069248. [Crossref] [PubMed]
- Bahçeci Şimşek İ, Şirolu C. Analysis of surgical outcome after upper eyelid surgery by computer vision algorithm using face and facial landmark detection. Graefes Arch Clin Exp Ophthalmol 2021;259:3119-25. [Crossref] [PubMed]
- Huang X, Lee SJ, Kim CZ, Choi SH. An automatic screening method for strabismus detection based on image processing. PLoS One 2021;16:e0255643. [Crossref] [PubMed]
- Johnson CC. Epicanthus. Am J Ophthalmol 1968;66:939-46. [Crossref] [PubMed]
- Mooss VS, Kavitha V, Ravishankar HN, Heralgi MM, Aafreen S. Presence and development of strabismus in children with telecanthus, epicanthus and hypertelorism. Indian J Ophthalmol 2022;70:3618-24. [Crossref] [PubMed]
- Spierer A. Acute concomitant esotropia of adulthood. Ophthalmology 2003;110:1053-6. [Crossref] [PubMed]
- Jiang WJ, Wu H, Wu JF, Hu YY, Lu TL, Sun W, Guo DD, Wang XR, Bi HS, Jonas JB. Corneal diameter and associated parameters in Chinese children: the Shandong Children Eye Study. Clin Exp Ophthalmol 2017;45:112-9. [Crossref] [PubMed]
- Flynn TH, Rose GE, Shah-Desai SD. Digital image analysis to characterize the upper lid marginal peak after levator aponeurosis repair. Ophthalmic Plast Reconstr Surg 2011;27:12-4. [Crossref] [PubMed]
- Liu Z, Luo P, Wang X, Tang X. Deep Learning Face Attributes in the Wild. Proceedings of the IEEE International Conference on Computer Vision (ICCV), 2015:3730-8.
- Alom MZ, Yakopcic C, Taha TM, Asari VK. Nuclei Segmentation with Recurrent Residual Convolutional Neural Networks based U-Net (R2U-Net). NAECON 2018 - IEEE National Aerospace and Electronics Conference, Dayton, OH, USA, 2018:228-33.
- Shrout PE, Fleiss JL. Intraclass correlations: uses in assessing rater reliability. Psychol Bull 1979;86:420-8. [Crossref] [PubMed]
- Bujang MA, Baharum N. A simplified guide to determination of sample size requirements for estimating the value of intraclass correlation coefficient: A review. Arch Orofac Sci 2017;12:1-11.
- Park DH, Park SU, Ji SY, Baik BS. Combined epicanthoplasty and blepharoptosis correction in Asian patients. Plast Reconstr Surg 2013;132:510e-9e. [Crossref] [PubMed]
- Oh J, Lee K. Medial lower lid epiblepharon repair solely by skin-redraping medial epicanthoplasty. Br J Ophthalmol 2014;98:1437-41. [Crossref] [PubMed]
- Shin DH, Woo KI, Kim YD. Relationship between lower eyelid epiblepharon and epicanthus in Korean children. PLoS One 2017;12:e0187690. [Crossref] [PubMed]
- Shao J, Cao J, Wang C, Xu P, Lou L, Ye J. Automatic Measurement and Comparison of Normal Eyelid Contour by Age and Gender Using Image-Based Deep Learning. Ophthalmol Sci 2024;4:100518. [Crossref] [PubMed]
- Udodaira K, Yokoyama E, Zhu T, Wang Y, Zhao L. Upper eyelid morphology and age-related changes in Japanese and Chinese females. Skin Res Technol 2024;30:e13604. [Crossref] [PubMed]
- Kunjur J, Sabesan T, Ilankovan V. Anthropometric analysis of eyebrows and eyelids: an inter-racial study. Br J Oral Maxillofac Surg 2006;44:89-93. [Crossref] [PubMed]
- Cai X, Chen Y, Li Q, Ma H, Tang Z, Nie C, Lu R. Anthropometric Analysis on the Ocular Region Morphology of Children and Young Adults in Chinese Han Population. Ophthalmic Plast Reconstr Surg 2019;35:326-32. [Crossref] [PubMed]
- Price KM, Gupta PK, Woodward JA, Stinnett SS, Murchison AP. Eyebrow and eyelid dimensions: an anthropometric analysis of African Americans and Caucasians. Plast Reconstr Surg 2009;124:615-23. [Crossref] [PubMed]