Repeatability and reproducibility of deep learning features for lung adenocarcinoma subtypes with nodules less than 10 mm in size: a multicenter thin-slice computed tomography phantom and clinical validation study
Introduction
Although strides have been made toward decreasing lung cancer mortality in the form of thin-slice computed tomography (TSCT) screening, lung cancer persists as a major global healthcare problem. As a consequence, early identification and intervention are necessary to improve survival rates (1). Central to accomplishing this task is the differentiation of lung adenocarcinoma subtypes, which substantially influence prognostic outcomes. For instance, there is a stark contrast between patients with adenocarcinomas in situ and minimally invasive adenocarcinoma (AIS-MIA)—which involves a nearly pristine 5-year disease-free recurrence rate—and those diagnosed with invasive adenocarcinoma (IAC) (2). Thus, establishing the subtype of lung adenocarcinoma early in the detection process is pivotal to improving patient prognosis.
However, subpulmonary segmental or wedge resection may result in an increased recurrence rate for those with IAC, particularly when lymphatic and vascular invasion occurs, even if the tumor is stage IA1 (T1aN0M0, with T1a denoting a tumor not larger than 10 mm in diameter) (3). Therefore, the differential diagnosis of AIS-MIA and IAC is necessary even for small lung adenocarcinomas (less than 10 mm in diameter) manifesting as ground-glass nodules (GGNs) with a hazy increase in attenuation of the lung and preservation of the bronchial and vascular margins (4). However, the diagnostic efficacy for differentiating subtypes of IA1 lung adenocarcinoma appearing as GGNs using radiological features from TSCT images is limited [with the area under curve (AUC) value for the diagnostic logistic model being reported to be 0.847 in the training dataset] (5). Thus, differentiating between AIS-MIA and IAC with sizes less than 10 mm and appearing as GGNs remains a challenge in clinical practice.
Traditional radiomics features (RFs) are high-throughput quantitative features derived from radiologic images and serve as useful adjuncts in informing key clinical decisions (6), including those related to lung cancer diagnosis (7-10). However, the AUC for the differential diagnostic efficacy based on the RF model for the subtype differentiation of lung adenocarcinoma (in GGN lesions measuring less than 10 mm in diameter) is still below 0.85 (11). Moreover, the lack of repeatability and reproducibility of RFs may hinder their generalizability in clinical practice. The RF model, selected through multicenter phantom experiments due its robustness, provides an AUC in diagnostic efficacy of 0.732 in the validation dataset (12). Recent radiomics analyses have proven ineffective in improving the differential diagnosis of early lung adenocarcinoma subtypes measuring less than 10 mm in diameter. Therefore, conducting large-scale end-to-end artificial intelligence (AI) studies is challenging due to the low occurrence of stage IA1 lung adenocarcinoma.
The fusion of deep learning with radiomics, resulting in the emergence of deep learning features (DLFs), has yielded improved outcomes in clinical studies (13,14). Deep learning, unlike traditional radiomics algorithms such as Fourier or wavelet transform-based feature extraction, can automatically learn and extract hierarchical features from raw imaging data, which may lead to superior generalization and predictive performance. Extensive research on other medical conditions has demonstrated that the integration of deep learning with imaging significantly enhances differential diagnostic efficacy (15). However, similar to RF, DLFs have been shown to have certain limitations, including issues with repeatability and reproducibility (13,16). For instance, Ziegelmayer et al. (17) found that for fruits, DLFs from convolutional neural networks were more stable compared with RFs. However, due to the different attenuation value of fruits and human tissues, further research is needed to confirm the applicability of these findings. It is thus essential to evaluate the stability of DLR via a thorax-lung phantom with an radiology absorption and attenuation number approximate to the human body and with standardized pulmonary nodules.
In the field of deep learning, unbiased learning is a broader research area that encompasses a variety of methods aimed at improving the robustness of the learned features during training. One such method is dropout, which randomly deactivates a subset of neurons during each training iteration to prevent overfitting and improve generalization (18). Another technique is batch normalization, which standardizes the inputs to a layer for each minibatch, stabilizing the learning process and reducing the number of training epochs (19). These methods are crucial in enhancing the performance of DLFs, potentially making them a superior alternative to RFs.
In this context, our study is novel by virtue of its focus on lung adenocarcinoma subtypes, specifically those measuring less than 10 mm in diameter and appearing as GGNs. We investigated the factors associated with DLFs by assessing their repeatability and reproducibility and developed a robust model for DLFs through multicenter phantom experiments. This work represents the refinement of the differential diagnosis of small lung cancer subtypes measuring less than 10 mm in diameter. Our aim is not only to substantially improve differential diagnosis but to open new possibilities in the sphere of early lung cancer detection. We present this article in accordance with the STARD reporting checklist (available at https://qims.amegroups.com/article/view/10.21037/qims-24-77/rc).
Methods
This study was conducted in accordance with the Declaration of Helsinki (as revised in 2013) and was approved by institutional review boards of Shanghai Public Health Clinical Center (No. 2022-S042-02), Zhongshan Hospital (No. B2020-429R) and Ruijin Hospital (No. 176 in 2022). The requirement for individual consent was waived due to the retrospective nature of the analysis. All participating hospitals were informed of and agreed to this phantom and clinical study (Figure 1).
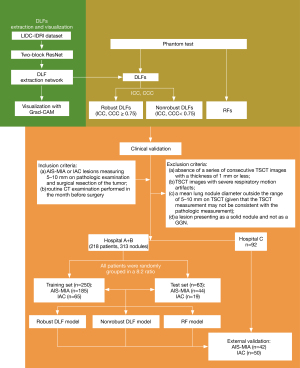
DLF extractor network pretraining
A deep learning network called two-block residual net (two-block ResNet) was designed for extracting DLFs (20). The network comprises two residual blocks, an average pooling layer, and a fully connected layer. The network was trained on a public pulmonary nodule dataset, the Lung Image Database Consortium and Image Database Resource Initiative (LIDC-IDRI) (20), which consists of 1,018 CT scans from 1,010 patients, and was used solely for pretraining for our deep learning network. For this binary classification task, we employed an Adam optimizer (21) with a learning rate of 0.001 and a batch size of 256, using binary cross-entropy as the loss function. The training process reached convergence after 50 epochs (Figure 2). Following training, the parameters of the two-block ResNet were frozen, and it was employed as a feature extractor.
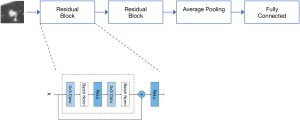
DLF extraction with a phantom
Our phantom study included an anthropomorphic thorax phantom (Kyoto Kagaku Co., Kyoto, Japan) with simulated nodules, which has been described previously (12). The phantom comprised nine spherical nodules, classified into three types of attenuation (–800, –630, and 100 Hounsfield units) and with three varying sizes (8, 10, and 12 mm in diameter). Based on the phantom model, we used multiple CT scanning protocols for testing and retesting from six widely used CT manufacturers. To account for the inconsistency in parameters of chest CT across different manufacturers, we selected one scanner to conduct test and retest scanning using different parameters, as described previously (12) (Table S1).
The identification and segmentation of phantom nodules were performed by two radiologists with nearly 10 years of experience in chest radiology (Yi Zhan and F.S.). They manually segmented the region of interest in all two-dimensional sections of the nodules using in-house software and segmentation tools (22). The region of interest was defined as a 32×32 square pixel segmentation at the center of the nodules, as shown in Figure 3. This size was chosen to maintain consistency with previous traditional radiomics analyses of phantom datasets and to facilitate comparison with previous results (12).
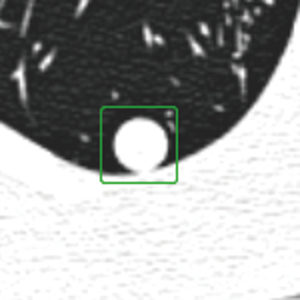
The two-block-ResNet was fed with the segmented CT images from the phantom dataset, and 64-dimensional DLFs were extracted from the average pooling layer of the network through forward propagation. For each nodule, we manually chose the slice with the largest nodule area and used its DLFs as a representation of the nodule’s DLFs.
Intergroup consistency evaluation of DLFs
To evaluate the robustness of the DLFs, we conducted experiments involving various sources of variability, including test-retest, inter-CT, and intra-CT protocol. In each experiment, we extracted the DLFs from both scans of every nodule of the phantom and calculated the concordance correlation coefficient (CCC) and the intraclass correlation coefficient (ICC) for each DLF. ICC values exceeding 0.75 were considered reliable (23). More importantly, a higher threshold could eliminate informative yet not fully stable features. Therefore, a DLF was considered stable if its CCC or ICC value exceeded 0.75, which could aid in the comparison with other studies (12).
Feature visualization of DLFs
To enhance the interpretability of DLFs, we use gradient-weighted class activation mapping (Grad-CAM) (24) to visualize them and gain insights into their relationship with nodule lesions. The clinical dataset’s CT images were input into the two-block-ResNet model, and the algorithm generated a saliency map, pinpointing the regions of the nodule that the DLFs emphasized.
Clinical validation
Classification training and testing dataset
The dataset was obtained from a previously reported dataset consisting of 313 GGNs from 288 patients, with diameters ranging from 5 to 10 mm [as reported previously (5)]. These GGNs were retrospectively reviewed from two hospitals: hospital A (Shanghai Public Health Clinical Center, Shanghai, China) and hospital B (Zhongshan Hospital, Shanghai, China). The inclusion criteria for the dataset were as follows: (I) GGNs with a diameter of 5–10 mm confirmed as either AIS-MIA or IAC through pathological examination and surgical resection and (II) availability of routine CT examination results from the month preceding the surgery. Nodules were excluded from the dataset if they met any of the following criteria: (I) absence of a series of consecutive TSCT images with a thickness of 1 mm or less; (II) presence of severe respiratory motion artifacts in TSCT images; (III) a mean lung nodule diameter outside the range of 5–10 mm on TSCT (since TSCT measurements may not align with pathologic measurements); and (IV) a lesion presenting as a solid nodule rather than as a GGN. The TSCT images were acquired as previously described (5) using five different scanners: Brilliance (Philips, Amsterdam, the Netherlands), SOMATOM Definition AS (Siemens Healthineers, Erlangen, Germany), Emotion 16 (Siemens Healthineers), Scenaria (Hitachi, Tokyo, Japan), and Aquilion ONE (Canon Medical Systems, Otawara, Japan). The dataset was randomly split into a training set (80%) and a test set (20%).
Classification model training and development
We developed different models to identify AIS-MIA and IAC. Three random forest classification models were created: (I) a robust DLF model, which included DLFs with a CCC or ICC value exceeding 0.75; (II) a non-robust DLF model, which included DLFs with a CCC or ICC value less than 0.75; and (III) an RF model, which included 91 RFs extracted using PyRadiomics (version 3.0.1). These RFs included first-order statistics (18 features), gray-level coordination matrix (22 features), gray-level run-length matrix (16 features), gray-level size zone matrix (16 features), neighboring gray-tone difference matrix (5 features), and gray-level dependence matrix (14 features). All three models were independently established, and their features did not overlap with one another. Morphological RFs were excluded due to the adoption of fixed-shape segmentation.
To optimize the models’ performance, a grid search technique was employed during the training process. Grid search is a method that systematically assesses different combinations of model parameters to identify the optimal configuration. Additionally, to mitigate the risk of overfitting, a fivefold cross-validation approach was applied within the grid search process. By combining the grid search technique and fivefold cross-validation, we could automatically identify the most effective features for accurate classification while reducing the likelihood of the model overfitting the training data.
External validation dataset
We tested our model on an external validation dataset retrospectively reviewed from another institution, hospital C (Ruijin Hospital, Shanghai, China). This dataset consisted of 92 GGNs with diameters ranging from 5 to 10 mm, including 42 AIS-MIA cases and 50 IAC cases. The inclusion and exclusion criteria for this external dataset were the same as those used for the training and testing dataset. All TSCT images from the external validation dataset were extracted from the respective institutional picture archiving and communication systems in Digital Imaging and Communications in Medicine (DICOM) format and were deidentified. No images from the external institutions’ picture archiving and communication systems were used for model training or hyperparameter fine-tuning. Table 1 provides a summary of the dataset details.
Table 1
Characteristic | Hospital A + B | Hospital C | ||||||
---|---|---|---|---|---|---|---|---|
Training set | Testing set | External validation | ||||||
AIS-MIA (n=185) | IAC (n=65) | AIS-MIA (n=44) | IAC (n=19) | AIS-MIA (n=42) | IAC (n=50) | |||
Type of nodule | ||||||||
GGN | 144 | 23 | 31 | 10 | 42 | 38 | ||
MGGN | 41 | 42 | 13 | 9 | 0 | 12 | ||
Nodule size (mm)* | 7.372 (1.329) | 8.265 (1.046) | 7.137 (1.364) | 7.615 (1.170) | 7.108 (1.088) | 7.206 (0.979) |
*, data are provided as the mean (standard deviation). GGN, ground-glass nodule; MGGN, mixed ground-glass nodule; AIS-MIA, adenocarcinoma in situ and minimally invasive adenocarcinoma; IAC, invasive adenocarcinoma; Hospital A, Shanghai Public Health Clinical Center, Shanghai, China; Hospital B, Zhongshan Hospital, Shanghai, China; Hospital C, Ruijin Hospital, Shanghai, China.
Statistical analysis
To assess the repeatability and reproducibility of the DLFs and traditional RFs, we employed a CCC (25) with a cutoff value of 0.85 and an ICC (26,27) with a cutoff value of 0.75 (23). Features surpassing these thresholds were deemed robust. During the clinical validation, accuracy and AUC were used to evaluate model performance.
Results
Visualization of DLFs
Visualization of the DLFs of pulmonary lesions in the clinical dataset was conducted, as depicted in Figure 4. Deep learning networks not only focused on the internal information features of the nodules but also on the information at the tumor-lung interface in a fashion similar to the description of radiological signs.
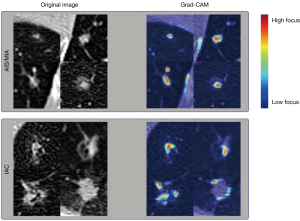
Evaluation of the robustness of DLFs
The robustness of each DLR feature was quantified by calculating the CCC and ICC for the three sources of variability (Table 2). Test-retest variability analysis revealed that 71.88% (46/64) of the DLFs demonstrated stability, with a CCC value of 0.88±0.04. Inter-CT variability analysis indicated that 87.50% (56/64) of the DLFs exhibited stability, with an ICC value of 0.86±0.09. Intra-CT protocol variability analysis demonstrated that all DLFs (64/64), including pitch, rotation time, tube voltage, tube current, field of view, and iteration level, exhibited stability, with an ICC value greater than 0.91. Further analysis indicated that the parameters of slice thickness and slice interval (ICC, 0.79±0.18) and reconstruction kernel (ICC, 0.82±0.07) were significantly associated with the robustness of the DLFs. In addition to the two-block-ResNet employed as our feature extractor, we conducted experiments with various DLR approaches (see Table S2 for details). The findings indicated that using a network with fewer layers tends to yield more robust image features. Therefore, our recommendation is to select a network model with this characteristic when a feature extractor is used.
Table 2
Variable | Measurement | Value* | Ratio† |
---|---|---|---|
Test-retest | CCC | 0.88±0.04 | 46 (71.88) |
Inter-CT | ICC | 0.86±0.09 | 56 (87.50) |
Pitch | ICC | 0.92±0.03 | 64 (100.00) |
Rotation time | ICC | 0.95±0.03 | 64 (100.00) |
Tube voltage | ICC | 0.93±0.04 | 64 (100.00) |
Tube current | ICC | 0.97±0.02 | 64 (100.00) |
Field of view | ICC | 0.91±0.07 | 64 (100.00) |
Slice thickness and slice interval | ICC | 0.79±0.18 | 33 (51.56) |
Reconstruction kernel | ICC | 0.82±0.07 | 57 (89.06) |
Iteration level | ICC | 0.98±0.01 | 64 (100.00) |
*, data are provided as the mean ± standard deviation; †, data are expressed as the numerator/denominator (percentage). The cutoff values were 0.75 for CCC and ICC. CT, computed tomography; CCC, concordance correlation coefficient; ICC, intraclass correlation coefficient.
Clinical validation
The robust DLF model was based on 28 DLF whose CCC or ICC value exceeded 0.75. A non-robust DLF model was based on 36 DLFs whose CCC or ICC value was less than 0.75. In the test set, the following performance outcomes for the classification of IAC and AIS-MIA were observed: the AUC of robust DLF model was 0.67 [95% confidence interval (CI): 0.51–0.81], and the AUC of non-robust DLF model was 0.47 (95% CI: 0.30–0.64); meanwhile, the AUC of the RF model was 0.49 (95% CI: 0.32–0.68). In the external validation, the following performance outcomes for all three models were observed: the AUC of the robust DLF model was 0.65 (95% CI: 0.53–0.76), the AUC of the non-robust DLF model was 0.51 (95% CI: 0.39–0.63), and the AUC of RF model was 0.55 (95% CI: 0.42–0.66) (Table 3, Figure 5).
Table 3
Model | Accuracy | AUC (95% CI) |
---|---|---|
Robust DLF with random forest | ||
Training set | 0.97 | 0.99 (0.99–1.00) |
Test set | 0.68 | 0.67 (0.51–0.81) |
External validation | 0.57 | 0.65 (0.53–0.76) |
Non-robust DLF with random forest | ||
Training set | 0.77 | 0.90 (0.85–0.95) |
Test set | 0.70 | 0.47 (0.30–0.64) |
External validation | 0.46 | 0.51 (0.39–0.63) |
RF with random forest | ||
Training set | 0.79 | 0.87 (0.81–0.91) |
Test set | 0.76 | 0.49 (0.32–0.68) |
External validation | 0.48 | 0.55 (0.42–0.66) |
DLF, deep learning feature; RF, radiomics feature; AUC, area under curve; CI, confidence interval.
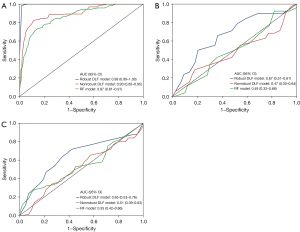
Discussion
The objective of our study was to evaluate the repeatability and reproducibility of DLFs in pulmonary nodules from CT images while also enhancing their interpretability and clinical validity. The results indicated that the DLFs had improved repeatability and reproducibility compared to the traditional RFs. Furthermore, the differential diagnosis model based on robust DLFs enhanced the diagnostic effectiveness for differentiating between IAC and AIS-MIA within the size range of 5–10 mm and appearing as GGNs in the test set and in the external validation. In addition, visual analysis emphasized the importance of the nodule edge in differential diagnosis and underscored the need for further research on the tumor-lung interface.
Generalizability is a common challenge in clinical trials, within both machine learning and radiomics research (28). Our study evaluated the repeatability and reproducibility of DLFs, along with the influencing factors. DLFs exhibited susceptibility to specific variations, such as test-retest, inter-CT, slice thickness, slice interval, and reconstruction kernel, which differed from other variations and were similar those of RFs (12). Compared with RFs (12), DLFs showed higher stability in each variable (test-retest, inter-CT, pitch, rotation time, tube voltage, tube current, field of view, slice thickness and slice interval, reconstruction kernel, and iteration level). Compared to RFs, the DLFs we extracted were mainly from the processing and learning of the original image with deep learning. The original images provide a comprehensive representation of the underlying anatomical structures and pathological features, enabling the extraction of more informative and discriminative features. Filtering techniques alter the original information and introduce artifacts or biases that can impact the performance and generalizability of the DLFs. By utilizing original images, DLF models can directly exploit the rich spatial and textural information present in the data, allowing for more accurate and robust feature extraction. Previous research supports the use of original images for DLF extraction (29), while in other work, for instance, that of Peng et al. (12), the traditional RFs included the results from filter-transformed images. In our study, despite the competitive advantage that was gained from extracting features of filter-transformed images, both the models using robust and non-robust DLFs outperformed the model using traditional RFs. Hence, we speculate that the filters may lead to the loss of information from the original image or a reduction in feature repeatability.
Moreover, despite our smaller sample size and imbalanced data compared to Peng et al. (12), our study achieved comparable model performance in different datasets from multiple centers. We further identified the influencing factors of DLFs from which we could propose the following paradigm for improving the robustness of DLF: first, establish pretrained DLFs models using appropriate databases; second, screen them through a phantom experiment; and finally, establish models through independent datasets to verify their effectiveness and generalization. This paradigm can assist future DLF research in establishing more generalized classification models and aid in laying the foundation for data standardization in subsequent multicenter DLF studies.
Additionally, in the external validation from another hospital, the robust DLF model demonstrated a better estimation of the generalizability of DLFs for image-based radiologic diagnosis compared to the non-robust DLF model and RF model; however, all three models in this study were relatively preliminary. Therefore, it is reasonable to presume that the DLF model may exhibit greater robustness than the RF model in multicenter studies or when an imbalance in data is present.
Despite some studies suggesting difficulties in the interpretability of DLFs, we demonstrated their interpretability through visualization. Grad-CAM is one such technique that has gained popularity in medical image visualization (30). Grad-CAM generates heatmaps by highlighting regions in the image that are most influential in the decision-making process of the DLF model. Overlaying the Grad-CAM heatmaps onto the original image allows for the visual identification of areas that significantly contribute to the model’s predictions, providing valuable insights into the interpretability of DLFs. In our study, the DLF model was primarily centered on the edge region, which is consistent with studies on radiological features (5) and RFs (11), underscoring the importance of tumor-lung interface in differential diagnosis. More importantly, this finding is relevant to the challenges encountered in clinical practice. As it pertains to small lung nodules (lesions less than 10 mm in diameter), the tumor-lung interface of nodules has been found to be more challenging for radiologists to distinguish (31). Therefore, in future research on small lung nodules, greater focus on the tumor-lung interface may be fruitful. Moreover, in studies involving the semiautomatic or manual delineation of the region of interest, the area of the tumor-lung interface should be more carefully distinguished and its consistency more intently scrutinized.
Our study involved several limitations that should be mentioned. First, the phantom experiment did not include all mainstream CT scanners and their scanning parameters although the majority were covered. Second, our findings could have been influenced by bias from the datasets. In the LIDC-IDRI database, the scanner type for each image is not explicitly indicated, which might have had an impact on our DLF extraction. Moreover, the clinical validation datasets were imbalanced and did not include population characteristics (such as ethnicity and gender) for consideration, which could have altered the reproducibility of the RFs and DLFs and limit the effectiveness of the DLFs model. In order to establish a model more suitable for clinical validation, we chose a dataset with an incidence rate consistent for permanent model training (IAC:AIS-MIA ~1:3, less than 10 mm) (32). More balanced data were used for external verification to further confirm the potential of robust DLFs in multicenter research or with data imbalance. Third, the use of two-dimensional DLF extraction and the segmentation method limited the amount of extracted information, thereby influencing the extracted features. Additionally, we acknowledge that data inclusion and patient characteristics such as ethnicity and sex could alter the reproducibility of RFs and DLFs, and this was not accounted for in our study. Nonetheless, we were able to examine the robustness of DLFs, the influencing factors, and their potential to improve the differential diagnostic efficiency of small lung adenocarcinoma subtypes. Future work will aim to address these limitations and further refine our results.
Conclusions
The DLFs demonstrated superior robustness and improved differential diagnostic efficacy compared to RFs when distinguishing between IAC and AIS-MIA within the size range of 5–10 mm and appearing as GGNs. Therefore, DLF models may have promise in enhancing robustness and performance in in multicenter, clinical trials. Additionally, DLF visualization can aid clinicians in gaining a deeper understanding of the decision-making process of the DLF model.
Acknowledgments
We would like to thank the staff at the Radiology Department of the Shanghai Public Health Clinical Center for their contributions to this study.
Funding: This work was supported by
Footnote
Reporting Checklist: The authors have completed the STARD reporting checklist. Available at https://qims.amegroups.com/article/view/10.21037/qims-24-77/rc
Conflicts of Interest: All authors have completed the ICMJE uniform disclosure form (available at https://qims.amegroups.com/article/view/10.21037/qims-24-77/coif). F.L. is an employee of Lianren Digital Health Technology Co., Ltd., but the employer has no financial interests, conflicts, or other connections related to the manuscript or the research it encompasses. The other authors have no conflicts of interest to declare.
Ethical Statement: The authors are accountable for all aspects of the work in ensuring that questions related to the accuracy or integrity of any part of the work are appropriately investigated and resolved. This study was conducted in accordance with the Declaration of Helsinki (as revised in 2013) and was approved by institutional review boards of Shanghai Public Health Clinical Center (No. 2022-S042-02), Zhongshan Hospital (No. B2020-429R), and Ruijin Hospital (No. 176 in 2022). The requirement for individual consent was waived due to the retrospective nature of this study. All participating hospitals were informed of and agreed to the study.
Open Access Statement: This is an Open Access article distributed in accordance with the Creative Commons Attribution-NonCommercial-NoDerivs 4.0 International License (CC BY-NC-ND 4.0), which permits the non-commercial replication and distribution of the article with the strict proviso that no changes or edits are made and the original work is properly cited (including links to both the formal publication through the relevant DOI and the license). See: https://creativecommons.org/licenses/by-nc-nd/4.0/.
References
- Siegel RL, Miller KD, Wagle NS, Jemal A. Cancer statistics, 2023. CA Cancer J Clin 2023;73:17-48. [Crossref] [PubMed]
- Detterbeck FC, Boffa DJ, Kim AW, Tanoue LT. The Eighth Edition Lung Cancer Stage Classification. Chest 2017;151:193-203.
- Donington JS. Survival After Sublobar Resection Versus Lobectomy for Clinical Stage IA Lung Cancer: Analysis From the National Cancer Database. J Thorac Oncol 2015;10:1513-4. [Crossref] [PubMed]
- Bankier AA, MacMahon H, Colby T, Gevenois PA, Goo JM, Leung ANC, Lynch DA, Schaefer-Prokop CM, Tomiyama N, Travis WD, Verschakelen JA, White CS, Naidich DP. Fleischner Society: Glossary of Terms for Thoracic Imaging. Radiology 2024;310:e232558. [Crossref] [PubMed]
- Zhan Y, Peng X, Shan F, Feng M, Shi Y, Liu L, Zhang Z. Attenuation and Morphologic Characteristics Distinguishing a Ground-Glass Nodule Measuring 5-10 mm in Diameter as Invasive Lung Adenocarcinoma on Thin-Slice CT. AJR Am J Roentgenol 2019;213:W162-70. [Crossref] [PubMed]
- Gillies RJ, Kinahan PE, Hricak H. Radiomics: Images Are More than Pictures, They Are Data. Radiology 2016;278:563-77. [Crossref] [PubMed]
- Li Y, Liu J, Yang X, Xu H, Qing H, Ren J, Zhou P. Prediction of invasive adenocarcinomas manifesting as pure ground-glass nodules based on radiomic signature of low-dose CT in lung cancer screening. Br J Radiol 2022;95:20211048. [Crossref] [PubMed]
- Wang T, She Y, Yang Y, Liu X, Chen S, Zhong Y, Deng J, Zhao M, Sun X, Xie D, Chen C. Radiomics for Survival Risk Stratification of Clinical and Pathologic Stage IA Pure-Solid Non-Small Cell Lung Cancer. Radiology 2022;302:425-34. [Crossref] [PubMed]
- Wu FZ, Wu YJ, Tang EK. An integrated nomogram combined semantic-radiomic features to predict invasive pulmonary adenocarcinomas in subjects with persistent subsolid nodules. Quant Imaging Med Surg 2023;13:654-68. [Crossref] [PubMed]
- Ren H, Xiao Z, Ling C, Wang J, Wu S, Zeng Y, Li P. Development of a novel nomogram-based model incorporating 3D radiomic signatures and lung CT radiological features for differentiating invasive adenocarcinoma from adenocarcinoma in situ and minimally invasive adenocarcinoma. Quant Imaging Med Surg 2023;13:237-48. [Crossref] [PubMed]
- Shi L, Shi W, Peng X, Zhan Y, Zhou L, Wang Y, Feng M, Zhao J, Shan F, Liu L. Development and Validation a Nomogram Incorporating CT Radiomics Signatures and Radiological Features for Differentiating Invasive Adenocarcinoma From Adenocarcinoma In Situ and Minimally Invasive Adenocarcinoma Presenting as Ground-Glass Nodules Measuring 5-10mm in Diameter. Front Oncol 2021;11:618677. [Crossref] [PubMed]
- Peng X, Yang S, Zhou L, Mei Y, Shi L, Zhang R, Shan F, Liu L. Repeatability and reproducibility of computed tomography radiomics for pulmonary nodules: a multicenter phantom study. Invest Radiol 2022;57:242-53. [Crossref] [PubMed]
- Afshar P, Mohammadi A, Plataniotis KN, Oikonomou A, Benali H. From handcrafted to deep-learning-based cancer radiomics: challenges and opportunities. IEEE Signal Process Mag 2019;36:132-60. [Crossref] [PubMed]
- Zheng X, Yao Z, Huang Y, Yu Y, Wang Y, Liu Y, Mao R, Li F, Xiao Y, Wang Y, Hu Y, Yu J, Zhou J. Deep learning radiomics can predict axillary lymph node status in early-stage breast cancer. Nat Commun 2020;11:1236. [Crossref] [PubMed]
- Zhang J, Liu J, Liang Z, Xia L, Zhang W, Xing Y, Zhang X, Tang G. Differentiation of acute and chronic vertebral compression fractures using conventional CT based on deep transfer learning features and hand-crafted radiomics features. BMC Musculoskelet Disord 2023;24:165. [Crossref] [PubMed]
- Zhang X, Zhang Y, Zhang G, Qiu X, Tan W, Yin X, Liao L. Deep Learning With Radiomics for Disease Diagnosis and Treatment: Challenges and Potential. Front Oncol 2022;12:773840. [Crossref] [PubMed]
- Ziegelmayer S, Reischl S, Harder F, Makowski M, Braren R, Gawlitza J. Feature Robustness and Diagnostic Capabilities of Convolutional Neural Networks Against Radiomics Features in Computed Tomography Imaging. Invest Radiol 2022;57:171-7. [Crossref] [PubMed]
- Srivastava N, Hinton GE, Krizhevsky A, Sutskever I, Salakhutdinov R. Dropout: a simple way to prevent neural networks from overfitting. J Mach Learn Res 2014;15:1929-58.
Ioffe S Szegedy C. Batch Normalization: Accelerating Deep Network Training by Reducing Internal Covariate Shift. 2015 ;arXiv:1502.03167.- Armato SG 3rd, McLennan G, Bidaut L, McNitt-Gray MF, Meyer CR, Reeves AP, et al. The Lung Image Database Consortium (LIDC) and Image Database Resource Initiative (IDRI): a completed reference database of lung nodules on CT scans. Med Phys 2011;38:915-31. [Crossref] [PubMed]
- Kingma DP, Ba J. Adam: A Method for Stochastic Optimization. CoRR 2014;abs/1412.6980.
- Li J, Zhou L, Zhan Y, Xu H, Zhang C, Shan F, Liu L. How does the artificial intelligence-based image-assisted technique help physicians in diagnosis of pulmonary adenocarcinoma? A randomized controlled experiment of multicenter physicians in China. J Am Med Inform Assoc 2022;29:2041-9. [Crossref] [PubMed]
- Koo TK, Li MY. A Guideline of Selecting and Reporting Intraclass Correlation Coefficients for Reliability Research. J Chiropr Med 2016;15:155-63. [Crossref] [PubMed]
- Selvaraju RR, Cogswell M, Das A, Vedantam R, Parikh D, Batra D. editors. Grad-CAM: Visual Explanations from Deep Networks via Gradient-Based Localization. 2017 IEEE International Conference on Computer Vision (ICCV); 2017 22-29 Oct. 2017.
- Lin LI. A concordance correlation coefficient to evaluate reproducibility. Biometrics 1989;45:255-68.
- Shrout PE, Fleiss JL. Intraclass correlations: uses in assessing rater reliability. Psychol Bull 1979;86:420-8. [Crossref] [PubMed]
- McGraw KO, Wong SP. Forming inferences about some intraclass correlation coefficients. Psychol Methods 1996;1:30-46.
- Yu AC, Mohajer B, Eng J. External Validation of Deep Learning Algorithms for Radiologic Diagnosis: A Systematic Review. Radiol Artif Intell 2022;4:e210064. [Crossref] [PubMed]
- Kheradpisheh SR, Ghodrati M, Ganjtabesh M, Masquelier T. Deep Networks Can Resemble Human Feed-forward Vision in Invariant Object Recognition. Sci Rep 2016;6:32672. [Crossref] [PubMed]
- Nam JG, Park S, Park CM, Jeon YK, Chung DH, Goo JM, Kim YT, Kim H. Histopathologic Basis for a Chest CT Deep Learning Survival Prediction Model in Patients with Lung Adenocarcinoma. Radiology 2022;305:441-51. [Crossref] [PubMed]
- Xu DM, van der Zaag-Loonen HJ, Oudkerk M, Wang Y, Vliegenthart R, Scholten ET, Verschakelen J, Prokop M, de Koning HJ, van Klaveren RJ. Smooth or attached solid indeterminate nodules detected at baseline CT screening in the NELSON study: cancer risk during 1 year of follow-up. Radiology 2009;250:264-72. [Crossref] [PubMed]
- Kato F, Hamasaki M, Miyake Y, Iwasaki A, Iwasaki H, Nabeshima K. Clinicopathological characteristics of subcentimeter adenocarcinomas of the lung. Lung Cancer 2012;77:495-500. [Crossref] [PubMed]